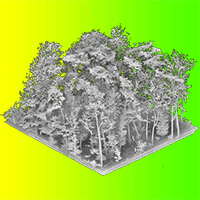
How biomass and other tree architectural characteristics relate to the structural complexity of a beech-pine forest
iForest - Biogeosciences and Forestry, Volume 16, Issue 6, Pages 368-376 (2023)
doi: https://doi.org/10.3832/ifor4305-016
Published: Dec 19, 2023 - Copyright © 2023 SISEF
Research Articles
Abstract
The provision of ecosystem functions and services in forests is closely linked to the presence of complex structures. One such service is the ability to store carbon. It has recently become possible to quantify both structural complexity and biomass of forests (as proxy of carbon storage) using light detection and ranging (LiDAR). The objective of this study was to analyze how the community-level complexity of a forest stand relates to structural characteristics, and biomass in particular, of the trees comprising the stand. To do so, we virtually assembled 30 forests (3D models), all representing different versions of a beech-pine forest in Germany, based on real world 3D LiDAR scan data of all trees in the forest. At the individual tree level, various structural characteristics, including wood volume and biomass were derived using both voxel models and quantitative structure models (QSM). Basal area and biomass, as well as to a lower degree also the mean height of maximum crown projection area, significantly affected the structural complexity at stand level. Among the different forest models, the variation in complexity could best be described using a combination of basal area, mean height of the maximum crown projection area, and the coefficient of variation of total tree height. Biomass alone explained 54% of the variation in stand-level complexity, while the multivariate model based on measures addressing the amount and vertical distribution of plant material explained 86% of the variability in complexity. Using a laser-based and holistic approach of assessing the structural complexity, namely the box-dimension, allowed identifying key structural attributes that promote aboveground structural complexity of the forest studied here.
Keywords
LiDAR, 3D Forest Model, Mobile Laser Scanning, Pine-beech Forest, Mixed Forest, Structural Complexity
Introduction
In the context of increasing socio-political demands on forest ecosystems, the challenge for forest management is to ensure that ecosystem services are provided and ecosystem multifunctionality is maintained ([20]). As forests with a high vertical and horizontal structural diversity can generate a multitude of ecosystem functions and services ([28], [14]), research increasingly demands that forests should be understood as complex adaptive systems ([4], [40]) and that their management should be adapted accordingly to promote structural complexity ([35]).
Structural complexity can be defined as the sum of all dimensional, architectural and distributional characteristics of all plant individuals in a given space and at a given point in time ([50]). With light detection and ranging (LiDAR), it has become possible to record this holistic forest characteristic directly and efficiently, not focusing on individual trees but the forest as a community structure. Novel LiDAR-based measures proved useful for the quantification of structural complexity, for example the canopy rugosity with a focus on the spatial heterogeneity of foliage ([19]), the stand structural complexity index (SSCI - [11]), or the box-dimension (Db - [49]).
Similarly, from laser scanning data it is today possible to automatically reconstruct accurate 3D models of trees to estimate aboveground biomass ([42], [3], [7], [6]). With so-called quantitative structure models (QSMs), representing trees as a hierarchical collection of cylinders and other building blocks, the volume of individual tree compartments has become available with no need for destructive methods ([41]). The use of LiDAR to measure biomass in forest ecosystems offers two distinct advantages to allometric derivation: it allows continuous monitoring of biomass dynamics ([2], [27]) and mapping of biomass heterogeneity ([61]), both extremely important characteristics, as environmental factors and silvicultural measures are known to influence growth relationships between individuals ([22]) while climate change alters the metabolism of individual trees ([31], [56]).
Structural complexity and biomass are two forest characteristics that are seemingly interlinked, but a forest rich in biomass is not necessarily highly complex in structure as well. Most biomass is stored in the tree trunks, while structural complexity is largely driven by overall space occupation ([24]), individual tree architecture and branching pattern ([51]), and pattern of overall vertical layering ([55]). If the relationship between forest ecosystem structure and biomass is in the research focus, it is therefore evident that LiDAR sensors hold great potential to assist.
The objective of this case study was to identify key structural parameters for promotion of structural complexity and to analyze the relationship between biomass and structural complexity in particular. For this purpose, 3D data of a mixed pine-beech forest in the leaf-less state (winter) were obtained from mobile laser scanning and thirty virtual 3D forest models were created by combining the segmented individual trees of the real forest. The resulting stand models varied according to various structural parameters.
We hypothesized that (i) structural characteristics of the trees comprising a stand can explain the community complexity of a stand. We also hypothesized that (ii) stand biomass, if all wooden above-ground plant organs (stem, branches and twigs) are considered, is positively related to the complexity of a stand.
Materials and methods
Study sites
The forest studied here is involved in a long-term study program within the International Cooperative Program on Integration Monitoring (ICP IM) to investigate the effects of air pollution on ecosystems. It is located at the site “Neuglobsow” in the catchment area of the Lake Stechlin in the State of Brandenburg, North-East Germany (53° 08′ N, 13° 02′ E - Fig. 1). The site is located in a lowland area (approx. 65 m above sea level) on a gentle slope (median = 4.5%) and characterized by a temperate, subcontinental climate that shows strong annual variations. The average annual precipitation is 620 mm but varied between 400 and 820 mm per year during the period of 2000-2014. Most of the precipitation falls during winter. Furthermore, the site is characterized by weakly podzolized sandy soils, which are classified as arenosols according to the World Reference Base for Soil Resources. These soils possess a low capacity for plant-available water. The stocking of the study area consists mainly of deciduous beech (> 110 years old) and Scots pine (> 160 years old) and the stand has been managed for conservation purposes since 1936 ([45], [44], [10]). The investigated intensive-measurement site in the forest area is fenced and has an area of 0.25 ha (50 × 50 m).
Fig. 1 - General map of the study site at the station “Neuglobsow“ with a 3D representation of the mixed beech-pine stand (3D point cloud from laser scanning) on the left.
Laser scanning and point cloud processing
The forest stand was surveyed using a GeoSLAM ZEB® Horizon (GeoSLAM Ltd., Nottingham, UK) handheld mobile 3D laser scanner. During scanning, a maximum distance of 100 m can be measured between the laser scanner and the objects to be measured with a positional accuracy of around 3 cm. The LiDAR sensor of the device, a Velodyne VLP-16® (Velodyne Lidar, San Josém, CA, USA), measures distances using the time-of-light method. A total of 300.000 points can be captured per second ([15]).
For data collection, the study area was first circled with the laser scanner and later crossed several times without a fixed pattern, simply to ensure good coverage of the site. The duration of the recording was approx. 20 minutes and the stand was recorded in winter 2020 to 2021 (leaf-off state). The scan was processed using GeoSlam Hub v. 6.1 (GeoSlam Ltd.) and exported as xyz-file (point cloud) for further post-processing. Slope correction of the point cloud was performed using LIDAR360 (Greenvalley International, CA, USA). To reduce noise in the 3D point cloud, sub-sampling (0.01 m) and noise filtering (spherical: 0.1 m) were applied in CloudCompare (version 2.12 beta - ⇒ http://www.danielgm.net/). To address the individual trees, they were segmented manually from the point cloud for maximum quality. All trees located with their stem base inside the fence and with at least 4 m in height were considered in our study (n = 100 trees).
At the individual tree level, a series of structural parameters were derived using the methods introduced in the literature (Tab. 1). A fully automatic determination of the tree diameter was not possible for some of the very small tree trees (<10 cm in diameter), therefore manual measurement on the computer was performed in CloudCompare using two opposing points on a cross-sectional disc selected from the point cloud at breast height (1.3 m).
Tab. 1 - Structural attributes determined at single tree level.
Parameter | Abbrev. | Type | Reference |
---|---|---|---|
Total tree height (m) | TTH | cloud-based | Seidel et al. ([47]) |
Diameter at breast height (cm) | DBH | cloud-based | Seidel et al. ([47]) |
Maximum crown projection area (m2) | MaxArea | cloud-based | Seidel et al. ([48]) |
Height of maximum crown projection area (m) | HMaxarea | cloud-based | Seidel et al. ([48]) |
Crown volume (m3) | CrVo | cloud-based | Metz et al. ([36]) |
Crown surface area (m2) | CSA | cloud-based | Metz et al. ([36]) |
Box-dimension | Db | cloud-based | Seidel ([49]) |
Wooden tree volume | WTV | QSM-based | Hackenberg et al. ([16], [17], [18]) |
Wooden tree volume (WTV) was determined using the CompuTree software with the SimpleForest plugin ([17]). Here, QSM-models were built with the same parameter settings for each tree: 0.10 m clustering tolerance and a maximum of 600-point clusters with 400 points each. At least 0.5% of all points had to be contained in a cluster for it to be created ([8]).
To determine the aboveground biomass (AGB), WTV was multiplied by the species-specific functions for the conversion of volume to dry wood substance. A density of 558 kg m-3 was assumed for beech and 431 kg m-3 for pine ([29], [30], [59]).
3D Forest models
For the analysis of the biomass-complexity relationship, thirty virtual models of the forest stand were “assembled” in CloudCompare using the trees identified and segmented from the real stand (Tab. 2). The individual trees of each model forest were loaded into a single project point cloud and the point clouds were saved as a merged 3D forest model point cloud.
Tab. 2 - Overview of the varied structural parameters within the 30 forest models.
No. | Description | Group | No. trees |
---|---|---|---|
1 | Current stand situation | Original | 100 |
2 | pure beech stand (all pine trees removed, without understory) | Mixture | 47 |
3 | pure pine stand (all beech trees removed, without understory) | Mixture | 16 |
4 | 50% beech, 50% pine (no understory, randomly selected trees) | Mixture | 32 |
5 | 80% beech, 20% pine (no understory, randomly selected trees) | Mixture | 59 |
6 | 70% pine, 30% beech (no understory, randomly selected trees) | Mixture | 23 |
7 | 20% of the tallest trees with respect to TTH (both species) | TTH | 21 |
8 | 60% symmetrically distributed around mean TTH (both species) | TTH | 59 |
9 | 20% of the tallest trees removed | TTH | 79 |
10 | All diameters represented once (classes of 1 cm) | DBH | 43 |
11 | developmental stage: immature timber (15 cm to 37 cm DBH) | DBH | 29 |
12 | developmental stage: intermediate timber (38 cm to 50 cm DBH) | DBH | 21 |
13 | developmental stage: mature timber (> 50 cm DBH) | DBH | 12 |
14 | trees with the highest DBH removed (upper 10%) | DBH | 90 |
15 | trees with highest crown volume (upper 10%) | Crown structure | 10 |
16 | 30% symmetrically distributed around the mean of CrVo | Crown structure | 30 |
17 | of trees with greatest CSA (upper 20%) | Crown structure | 20 |
18 | 60% symmetrically distributed around the mean of CSA | Crown structure | 60 |
19 | trees with highest HMaxarea (upper 40%) | Crown structure | 40 |
20 | trees with lowest HMaxarea (lowest 60%) | Crown structure | 60 |
21 | trees with highest Maxarea (upper 50%) | Crown structure | 50 |
22 | least complex trees (lower 35%) | Complexity | 35 |
23 | 30% symmetrical distributed around the mean of Db | Complexity | 30 |
24 | most complex trees (upper 35%) | Complexity | 35 |
25 | pure beech stand, evenly distributed, without understory (copies of an identical, randomly selected single tree) | Plantation | 49 |
26 | pure pine stand, evenly distributed, without understory (copies of an identical, randomly selected single tree) | Plantation | 25 |
27 | row-wise mixture of beech and pine, without understory (two identical, randomly selected individuals of each tree species) | Plantation | 52 |
28 | stand with single tree mixture of two trees with the highest Db of the respective tree species | Plantation | 47 |
29 | stand with strip-wise mixture of two randomly selected trees from the upper and lower layer | Plantation | 78 |
30 | stand with troop mixture of randomly selected trees from all stand layers | Plantation | 187 |
The current stand situation in the real world was represented as model 1. The additional models varied in different aspects, such as tree species mixture (pure stands of Fagus sylvatica and Pinus sylvestris in model 2 and 3, respectively), different mixture proportions (model 4-6), different vertical layering (only tallest trees: model 7), only trees with intermediate height (model 8) and models with only small and intermediate trees (model 9).
In model 10, random representatives of each diameter class (one individual per class) were combined to form a forest stand and in model 11-13 the trees were assigned to their developmental stages (immature, intermediate, mature). In model 14, the trees with the largest diameter (10% of the highest DBH) were removed from the stand. In model 15-24 different architectural parameters like crown volume (CrVo), crown surface area (CSA), height of the greatest crown projection area (HMaxarea), and the maximum crown projection area (MaxArea) were varied, selecting the trees with the highest and medium expressions of these parameters, respectively. In addition, model 22-24 contained trees with only low, medium, and high individual tree complexity (Db), respectively.
In model 25 to 30 tree positions were not based on the real-world location anymore. Instead, plantation-like forests were created in which identical, randomly selected individual trees of F. sylvatica (model 25) and P. sylvestris (model 26) were replicated and evenly arranged in the stand area. In subsequent models, two randomly selected representatives of both tree species were mixed in rows (model 27), then the two most complex individuals were mixed tree by tree (model 28), two randomly selected trees of the upper and lower stand were mixed in stripes (models 29), and finally, single trees from each stand layer were arranged in troops (model 30). In these models, the stem base of the individual trees was not allowed to cross the fence area and the crowns were not allowed to overlap or touch. Basal area was not allowed to exceed less than 20% and more than 120% of the initial value. For an overview on all tested forest models see Tab. 2.
For each 3D forest, the structural complexity was determined based on the box-dimension of the final model. The box-dimension (Db) is a holistic measure of structural complexity that can be obtained from laser scanning in an efficient manner for single trees ([49], [8], [46], [9]) as well as entire forest plots ([21], [37]), including mobile, tripod-based and airborne approaches ([52]). For those 3D forest models whose areal extent decreased, the box-dimension was reduced on an area weighted basis to take account for the new areas without trees present. This was necessary to correct because the potential areas without trees at the plot margin remain unaccounted for. This would result in a Db calculation for the area with trees only, which would correctly determine the box-dimension of this area, but ignore the fact that there is a partial clear-cut in the plot without any complexity. To avoid this, we used the approach above (area-weighted Db), practically reducing the measured Db by the “empty” parts of the plot.
Statistical analysis
For each forest model, the mean and coefficient of variation of the parameters of the individual trees were determined. The relationship between this data and the complexity (Db) of the whole 3D forest model (study stand) was tested using simple linear regression. The best multiple linear regression model explaining stand-level structural complexity was selected using stepwise variable selection (stepAIC). The independent variables in the model were also tested for multicollinearity using variance inflation factor (VIF) values. Analysis of residuals of the statistical model was then carried out to check for validity. All statistical analyses, models and graphs were performed with RStudio Desktop v. 2022.07.2+576 ([43]).
Results
Tree and stand characteristics
A total of 100 individual trees were segmented from the original point cloud, of which 63 trees were in the upper stand layer (dominant) and 37 in the lower and intermediate stand (co-dominant or suppressed). In the dominant stand layer, 47 (75%) European beech and 16 (25%) Scots pine trees were recorded. In the understory, mainly individuals of F. sylvatica were found. A large range of tree sizes were observed in the studied stand (Tab. 3).
Tab. 3 - Statistical measures of the structural parameters of the individual trees (n = 100) in the studied forest. (SD): standard deviation.
Parameter | Min | Max | Mean ± SD |
---|---|---|---|
TTH (m) | 4.04 | 33.83 | 20.83 ± 11.49 |
DBH (cm) | 2.4 | 73.26 | 27.11 ± 20.00 |
HMaxarea (m) | 1.6 | 30 | 16.18 ± 10.67 |
Maxarea (m) | 0.66 | 99.56 | 24.83 ± 22.83 |
CrVo (m3) | 0.95 | 961.28 | 150.40 ± 19.07 |
CSA (m2) | 12.43 | 952.28 | 257.91 ± 222.44 |
Db | 1.45 | 2.03 | 1.76 ± 0.10 |
AGB (t) | 0.01 | 10.65 | 1.19 ± 1.58 |
Structural complexity of the tested 3D forest models
In the real-world condition (model 1, the reference), the investigated forest stand reached a Db value of 2.17. If the beech and pine percentages (model 4) were set equal, the Db value decreased slightly (2.11) and if only the pines were represented (model 3), the lowest complexity (Db: 1.18) was achieved. When the tallest trees in the study stand (model 7) were presented together, here resulting in a higher proportion of pines being present, structural complexity decreased. In contrast, the stand created in model 8, in which medium tree heights were present, reached a relatively high complexity (Db: 2.07). In the subsequent models, the highest Db was achieved when the trees with the highest DBH (10%; model 14) were not included. Moreover, the scenario that contained of mature trees had a low complexity of only 1.4 units in Db.
Model 21 referred to the upper half of the trees with a high MaxArea and resulted in a rather high Db value (Db: 2.03). In the plantation-like stands, model 25 (pure beech stand) showed an identical Db value as the reference stand, while the simulated pure pine stand (model 26) had a lower Db value (2.03). The tree by tree mixture in model 28 achieved a Db value of 2.16. An overview on the structural complexity of all modelled forests is given in Fig. 2, including a two-dimensional representation of the point clouds as seen from the side.
Fig. 2 - Box-plots showing the variation of Db in the respective silvicultural models depending on the grouping variables mixture, tree height (TTH, m), diameter at breast height (DBH, cm), crown structure, structural complexity (Db) and plantation. The individual tree parameters were varied in their expression (high, medium, low) and the modelled stands were compiled accordingly. Model assignment to the groups was according to Tab. 2. The dashed line shows the Db of the real stand.
For a visual comparison of the complexity of the forests created in the different model groups (Tab. 2) the results are also visualized in Fig. 3, with the real-world forest (model 1 - Fig. 3, upper left) indicated as a reference.
Fig. 3 - 3D forest models. Representation of the point clouds of the thirty forest models with their respective characteristic values of structural complexity (Db). The assignment of each model to the different model groups is reported in Tab. 2.
Explaining structural complexity of the stand
From step-wise variable selection, the best regression model explaining structural complexity was identified using AIC comparison. It contained stand basal area, mean HMaxarea and the coefficient of variation of TTH as combined predictors of Db. Multicollinearity of the independent variables could be excluded (VIF < 10). The respective regression coefficients of the variables (Tab. 4) were all significant and the adjusted coefficient of determination (R2adj) of the regression model was 0.86. The residuals of the constructed model were homoscedastic. Thus, the requirement of constant error variance could be fulfilled. Furthermore, they were normally distributed, so that the model could be considered valid.
Tab. 4 - Coefficients of the “best” multiple linear regression model for estimating Db as a result of stepAIC. The model goodness of fit is R2adj = 0.86. All coefficients are significant (significance level: *** = p < 0.001).
Parameter | Estimate | Pr(>|t|) |
---|---|---|
Intercept | 3.036 | 2.50e-14 *** |
Basal area | 0.024 | 4.97e-12 *** |
Mean of HMaxarea | -0.068 | 6.98e-09 *** |
CV of TTH | -0.01 | 2.34e-06 *** |
Among all tested bivariate relationships between complexity at stand-level and statistical measures describing the structural characteristics of the trees in the stand, the Db-biomass relationship had the highest coefficient of determination (R2 = 0.54 - Fig. 4). Basal area as well as mean Hmaxarea of the trees were also significantly related to the stand-level structural complexity, with R² being 0.45 and 0.14, respectively. All other tested tree characteristics were not related to the stand-level complexity.
Fig. 4 - Scatterplots showing the relationship between Db and the mean and coefficient of variation of height (TTH), diameter at breast height (DBH), height of maximum crown projection area (Hmaxarea, m), Maximum crown projection area (m2), crown surface area (CSA), crown volume (CrVo), as well as basal area, biomass and the structural complexity (Db) on single tree level. R2 is the coefficient of determination of the linear regression models and the dashed lines indicate the confidence interval at 95% for significant relationships.
Discussion
We investigated thirty different 3D forest models created from real-world trees in terms of their structural complexity to gain a deeper understanding of the geometric drivers of complexity at stand level.
As we used the box-dimension as a holistic measure of complexity ([33]), it is theoretically possible that 3D forest models created artificially result in higher complexity than the initial real-world stand (model 1, reference). However, in our study, the complexity of the reference stand (model 1) was only reached by a plantation (model 25 - Fig. 2, Tab. 2, Fig. 3) with highly artificial structures (identical copy of a randomly selected beech tree, no admixed pine, dense planting scheme). One would expect lower complexities for models with fewer trees than in the reference if the remaining trees are located on their real-world positions (model 2-24), since such models resemble basically a virtual harvest. This was in fact confirmed by the data. However, models 25-30 (the plantations) with trees positioned on artificial locations in the stand or with greater number of trees, could theoretically result in greater complexity than that observed for the reference due to artificial tree positions and higher tree numbers than those of the reference. In case of model 30, with 187 trees instead of the 100 in the reference, one would almost expect an increased complexity. As shown in Fig. 2, the model group “plantations” in fact did not exceed the reference but possessed the highest average complexity of all groups. This can likely be explained by the set of rules we established before model construction. By not being allowed to touch the neighboring crowns, space occupation was clearly limited if trees in the plantation where identical copies, since each tree copy filled the same vertical layer. A homogenous vertical filling created by a pronounced multi-layeredness is however known to be decisive for a large box-dimension ([55], [57]). Furthermore, by limiting the basal area to more than 20% (all models) and less than 120% (plantation models) of the reference, we also set boundaries as to how unrealistic a model could be.
If only the tallest trees were selected for building a forest model, the structural complexity was comparatively low. In contrast, models that mainly included the dominated trees in addition to the less dominant ones showed a higher structural complexity. Models 8 and 9 additionally differed in the height of the understory trees added to the scene, which had a rather small influence on structural complexity. The predominant single-layered structure of the studied stand in its natural form (model 1) can be seen in the regression coefficient of the multiple regression model (Tab. 4), as the coefficient of variation of the TTH had a negative effect. This means that the Db here reacts sensitively to the increase in height variation. The main part of the structural complexity of the stand is therefore achieved via the dominant stand layer. In stands with more pronounced vertical structuring, the coefficient of variation of height would be expected to influence the Db positively. While literature points towards the importance of large trees for a high stand-level complexity ([13], [50]), we like to add that this is not necessarily true for tall individuals. In our case study, the tall pine trees did not result in a high Db at stand level, simply due to their poor complexity at the scale of the individual. This does not mean that admixing pines to the beech trees did not have positive effects. Mixing effects observed here basically confirmed earlier findings. The Db of forest model 5 (80% F. sylvatica, 20% P. sylvestris) differed only slightly from that of the initial stand, likely due to a similar mixture ratio. If the mixing proportions of pine were increased (e.g., model 4 and 6), and the number of individuals of F. sylvatica was consequently reduced, the Db was considerably decreased. The pure stand of F. sylvatica (model 2), on the other hand, had a significantly higher Db than the mixed stands from models 4 and 6, and the pure stand of P. sylvestris showed the lowest structural complexity. Juchheim et al. ([25]) also showed that increasing the proportion of beech in mixtures with pine (as well as spruce) is related to increased structural complexity. The decisive factor is likely the interspecific variations in crown architecture at the individual tree level, which enabled a complementary use of the crown space ([58], [1]). Furthermore, structural heterogeneity is increased when species with different shade tolerance are mixed ([39]). These two mechanisms lead to a more efficient use of canopy space in mixed stands than in pure stands ([26]). Likely for this reason, and despite the fact that beech stands can grow extremely complex structures if naturally grown or managed accordingly ([55]), the admixture of pine was still beneficial to stand complexity in our case study.
As we found three of the tested structural characteristics of the individual trees to relate significantly to the stand-level complexity, namely the sum of the trees’ biomass, the basal area, and the mean height of maximum crown projection area (Fig. 4), we accepted our starting hypothesis (i). For single tree parameters, earlier studies showed that measures of tree size (height, crown radius) as well as descriptors of internal architecture (branching) explain the complexity of the individual tree ([51]). However, here we were interested in explaining the stand-level complexity. So far, this has only been done for smaller stands ranging from 10 × 10 m to 20 × 20 m with only very few parameters being related to stand-level complexity for the 20 × 20 m, and those few only weakly (R²< 0.2 - [50]). It is likely that single tree characteristics more and more lose importance for the complexity of a 50 × 50 m stand. Individual tree features are simply overridden by stand-level features. At larger scales synergetic interactions might give rise to properties at the stand level that can no longer be detected at the individual tree level when viewed in isolation ([34], [51]). Therefore, the effect of favorable crown parameters, which increase structural complexity at the individual tree level, may become less important at the stand level. It is hence not surprising that we did not observe any relationship with complexity for many of the tested measures (Fig. 4).
Our data also support hypothesis (ii), stating that the Db is sensitive to the amount of biomass in a forest. It is important to mention here, that this relationship has been shown before on the tree-level scale, but not on stand scale. The strong explanatory power of biomass in our study (R2 = 0.54 - Fig. 4) is likely explained by the fact that biomass was here derived from very detailed QSM models depicting the trees up to smallest branches. This also supports the finding that structural complexity relates positively to increased space filling with plant material ([24], [54]). Biomass is often estimated using basal area, since the latter is one of the most convenient variables one can measure in a stand with traditional means ([5]). Basal area is also a stand characteristic that can be straightforwardly managed (increased or decreased). Although a functional relationship between a tree’s diameter and its crown shape exists ([38], [23]), the relationship between stem diameter and detailed crown architecture is often overridden by other influences during tree development, such as branch breakages, asymmetric growth as well as physiological adaptations to shade, competition and other biotic and abiotic environmental drivers of tree shape ([32]). Interestingly, despite these constraints, a linear relationship between stand structural complexity (Db) and stand basal area was found for the forest models tested here (R2 = 0.45 - Fig. 4). Earlier studies could either not find a relationship between stand basal area and the structural complexity in managed forests ([52]) or hump-shaped relationships in case of primary forests ([12], [53]). We argue that in our formerly managed forest, the derived positive relationship was linear because the maximum basal area observed in any of our models was still below 50 m2 per ha. In this range, earlier studies did also not observe hump-shaped pattern ([12]). Given the relationship between space filling and complexity ([24]) it can be expected that basal area, being a proxy for volume and thereby to some degree also space filling, is also related to the structural complexity. However, if we imagine a forest full of dead, branchless stems, there would be no relationship between basal area and the structural complexity anymore, since the complexity depends largely on the branching structures. Therefore, the strength of the relationship between Db and basal area depends on how well the stems’ cross-sectional areas actually relate to the number and distribution of branches. In our data, as well as in primary forests ([12]) this was obviously given, while in managed forests ([52]) this relationship might be overruled by management effects on crown development.
Finally, the mean height of the maximum crown projection area was significantly but weakly related to Db. The negative direction of the correlation seems plausible, as a lower mean height of maximum crown projection area basically indicates the presence of trees with deeper crowns, greater vertical layering and overall increased space filling, all factors associated with greater forest complexity ([24], [50], [55], [54]), as we have already stated above.
Despite overall confirmation of hypothesis (ii), the identified significant relationship between biomass and complexity also shows quite some unexplained variation (46%). For example, the initial stand (model 1) had a lower biomass than the forest stand built by model 25, but these two stands possessed the same structural complexity. Increasing biomass therefore not necessarily results in an increased complexity. The quantitative structure models here used for biomass quantification are considered the most reliable non-destructive method available ([6]). Still, we argue that biomass should not be used as sole proxy to estimate the structural complexity of a forest. Biomass is mostly located in the stems, which contribute little to the complexity of a forest. This is supported by the statistical modelling approach applied here. Using a combined model of basal area, mean Hmaxarea and the coefficient of variation of TTH the best prediction of the structural complexity was achieved (Radj = 0.86). This model basically combines a proxy for the amount of plant material (basal area) with a proxy for the vertical layering (mean Hmaxarea) and a proxy for the overall variability in tress sizes (CV of TTH). We already discussed the basal area - complexity relationship earlier in the context of the individual correlations. For mean Hmaxarea, it is not surprising to observe a negative coefficient in the multiple regression model (Tab. 4), as low-hanging crowns are contributing to the multi-layeredness of the stands. The last element, the CV of the tree heights, is known to be useful when it comes to addressing stand structural complexity, e.g., it is the core element of the structural complexity index by Zenner & Hibbs ([60]). However, in our multivariate model the effect of the coefficient of variation of TTH was significant but small and also negative, indicating that the modelled stands with less variation in tree heights were also more complex. This is likely an artefact caused by the plantations modelled here which were rather homogenous in height (models 25-30 - Fig. 2,) but still quite complex in structure.
Conclusion
In this case study, the variation of structural complexity (Db) as a function of stand structural parameters was investigated in thirty different 3D forest models of a mixed European beech and Scots pine stand using real-world structural information from hand mobile laser scanning. The Db of the stands in the individual forest models was most appropriately described (R²adj = 0.86) by a combination of stand basal area, mean height of the greatest crown projection area and the coefficient of height variation. Among the tested single structural variables, biomass of the stand was most closely related to the stands’ complexity but with a much lower explained variation (R² = 0.54).
As predicted by the theoretical derivation of the box-dimension, biomass alone is unlikely to explain structural complexity observed in a forest to a level where it could be justified using biomass alone as a solid proxy. The three-dimensional character of structural complexity is better reflected if additional measures addressing the vertical distribution of plant material are used in addition.
Conflict of Interest
The authors declare no conflict of interest.
Acknowledgements
This work was funded through grant SE2383/8-1 provided by the German Research Foundation (DFG).
References
CrossRef | Gscholar
CrossRef | Gscholar
CrossRef | Gscholar
Gscholar
Gscholar
Gscholar
CrossRef | Gscholar
CrossRef | Gscholar
Authors’ Info
Authors’ Affiliation
Friederike Anna Böttger
Department for Spatial Structures and Digitization of Forests, Faculty of Forest Sciences and Forest Ecology, Georg August University of Göttingen, Büsgenweg 1, Göttingen, 37077 (Germany)
Corresponding author
Paper Info
Citation
Seidel D, Böttger FA (2023). How biomass and other tree architectural characteristics relate to the structural complexity of a beech-pine forest. iForest 16: 368-376. - doi: 10.3832/ifor4305-016
Academic Editor
Francesco Ripullone
Paper history
Received: Jan 12, 2023
Accepted: Nov 04, 2023
First online: Dec 19, 2023
Publication Date: Dec 31, 2023
Publication Time: 1.50 months
Copyright Information
© SISEF - The Italian Society of Silviculture and Forest Ecology 2023
Open Access
This article is distributed under the terms of the Creative Commons Attribution-Non Commercial 4.0 International (https://creativecommons.org/licenses/by-nc/4.0/), which permits unrestricted use, distribution, and reproduction in any medium, provided you give appropriate credit to the original author(s) and the source, provide a link to the Creative Commons license, and indicate if changes were made.
Web Metrics
Breakdown by View Type
Article Usage
Total Article Views: 13050
(from publication date up to now)
Breakdown by View Type
HTML Page Views: 10849
Abstract Page Views: 1286
PDF Downloads: 788
Citation/Reference Downloads: 4
XML Downloads: 123
Web Metrics
Days since publication: 468
Overall contacts: 13050
Avg. contacts per week: 195.19
Article Citations
Article citations are based on data periodically collected from the Clarivate Web of Science web site
(last update: Mar 2025)
Total number of cites (since 2023): 1
Average cites per year: 0.33
Publication Metrics
by Dimensions ©
Articles citing this article
List of the papers citing this article based on CrossRef Cited-by.
Related Contents
iForest Similar Articles
Research Articles
Are we ready for a National Forest Information System? State of the art of forest maps and airborne laser scanning data availability in Italy
vol. 14, pp. 144-154 (online: 23 March 2021)
Research Articles
Integrating area-based and individual tree detection approaches for estimating tree volume in plantation inventory using aerial image and airborne laser scanning data
vol. 10, pp. 296-302 (online: 15 December 2016)
Research Articles
Do different indices of forest structural heterogeneity yield consistent results?
vol. 15, pp. 424-432 (online: 20 October 2022)
Technical Advances
Forest stand height determination from low point density airborne laser scanning data in Roznava Forest enterprise zone (Slovakia)
vol. 6, pp. 48-54 (online: 21 January 2013)
Research Articles
Optimizing line-plot size for personal laser scanning: modeling distance-dependent tree detection probability along transects
vol. 17, pp. 269-276 (online: 07 September 2024)
Research Articles
Three-dimensional forest stand height map production utilizing airborne laser scanning dense point clouds and precise quality evaluation
vol. 10, pp. 491-497 (online: 12 April 2017)
Research Articles
Determining basic forest stand characteristics using airborne laser scanning in mixed forest stands of Central Europe
vol. 11, pp. 181-188 (online: 19 February 2018)
Research Articles
Comparison of wood stack volume determination between manual, photo-optical, iPad-LiDAR and handheld-LiDAR based measurement methods
vol. 16, pp. 243-252 (online: 23 August 2023)
Research Articles
Oak often needs to be promoted in mixed beech-oak stands - the structural processes behind competition and silvicultural management in mixed stands of European beech and sessile oak
vol. 13, pp. 80-88 (online: 01 March 2020)
Research Articles
Efficient measurements of basal area in short rotation forests based on terrestrial laser scanning under special consideration of shadowing
vol. 7, pp. 227-232 (online: 10 March 2014)
iForest Database Search
Search By Author
Search By Keyword
Google Scholar Search
Citing Articles
Search By Author
Search By Keywords
PubMed Search
Search By Author
Search By Keyword