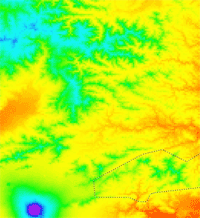
Remote sensing of Japanese beech forest decline using an improved Temperature Vegetation Dryness Index (iTVDI)
iForest - Biogeosciences and Forestry, Volume 4, Issue 5, Pages 195-199 (2011)
doi: https://doi.org/10.3832/ifor0592-004
Published: Nov 03, 2011 - Copyright © 2011 SISEF
Research Articles
Collection/Special Issue: COST Action FP0903 (2010) - Rome (Italy)
Research, monitoring and modelling in the study of climate change and air pollution impacts on forest ecosystems
Guest Editors: E Paoletti, J-P Tuovinen, N Clarke, G Matteucci, R Matyssek, G Wieser, R Fischer, P Cudlin, N Potocic
Abstract
The Tanzawa Mountains, which cover parts of Kanagawa, Yamanashi and Shizuoka prefectures in Japan, are known for their natural beech forests. Since the 1980s, decline of the beech forests, probably caused by air pollution, water stress and insect infestation, has become a serious problem. We estimated the natural beech forest mortality rate in the mountains by using multi-temporal 8-day composite data recorded at the MODIS instrument aboard the Terra satellite, daily air temperature data at meteorological stations (AMeDAS) in 2007, and a global digital elevation model obtained from ASTER aboard the Terra satellite. For the estimation, we used a Normalized Difference Vegetation Index (NDVI) indicating the vegetation density, a Temperature Vegetation Dryness Index (TVDI), and an improved TVDI (iTVDI) indicating the differences in transpiration rates between areas of similar vegetation density. We compared the NDVI, TVDI, and iTVDI maps with an existing mortality map of beech forests in the study area to verify their accuracy. To produce iTVDI maps, we calculated maps of air temperature by using ambient air temperature and elevation data. By interpolation using an environmental lapse rate, we calibrated air temperature maps with good accuracy (RMSE = 0.49 °C). The iTVDI map could detect mortality more accurately than the NDVI and TVDI maps in both spring and summer. Use of iTVDI enabled us to detect forest decline caused by air pollution and water deficits, inducing a reduction in transpiration rates. This index should be useful for monitoring vegetation decline.
Keywords
Decline, Forest, MODIS, Normalized Difference Vegetation Index (NDVI), Improved Temperature Vegetation Dryness Index (iTVDI)
Introduction
Surveys and monitoring of plant responses to environmental stress are important for us to understand plant functions and preserve ecosystems. However, in mountainous areas, it is difficult to conduct regular field surveys and more efficient monitoring methods are needed. Remote-sensing data obtained from sensors aboard satellites or aircraft can be used for surveys in such areas. Thermal sensing is useful for detecting vegetation responses such as stomatal closure, spectral sensing is useful for detecting declines in chlorophyll content, and lidar is useful for detecting changes in growth ([8], [2]). In examples of studies that have used remote-sensing data to estimate vegetation conditions, Omasa et al. ([7]) and Omasa ([6]) examined the effect of temperature differences on transpiration rate and stomatal conductance in areas near urban environments affected by air pollution. Rahimzadeh-Bajgiran et al. ([9]) used MOderate Resolution Imaging Spectroradiometer (MODIS) satellite data to examine drought in semi-arid regions. The spatial resolution of MODIS is only moderate (250, 500, or 1000 m), but it can observe land surfaces frequently and over wide areas. Thus, MODIS data are useful for studying and analyzing wide ranges of land cover and time series changes.
These studies focused not only on land cover classification, but also analyzing information on the physiological and ecological aspects of vegetation by calculating indices correlated with vegetation density or evapotranspiration rate. For example, the Normalized Difference Vegetation Index (NDVI - [11]) evaluates the density of vegetation. The Temperature Vegetation Dryness Index (TVDI - [12]) and the improved Temperature Vegetation Dryness Index (iTVDI - [10]) evaluate the evapotranspiration rate. TVDI is calculated by using a vegetation index (e.g., the NDVI) and the surface temperature. In contrast, iTVDI is calculated by using a vegetation index and the difference between surface and air temperatures. In fully covered vegetation areas in which the leaf surface is not wet, TVDI and iTVDI can be used to evaluate differences in the rates of evapotranspiration over areas of the same vegetation density. iTVDI corrects for the effect of elevation on surface temperature by using air temperature. In the Tanzawa Mountains, which were the focus of this study, the existence of dead trees and trees with discolored leaves and low biomass has been reported ([13]). By using iTVDI, we can expect to detect trees that have normal quantities of leaves but depressed evapotranspiration rates because of stomatal closure caused by lack of water or air pollution. To detect the ongoing forest decline ([14]) that is occurring in the Tanzawa Mountains, we need to evaluate such information on the physiological and ecological condition of vegetation.
Here, we made NDVI, TVDI, and iTVDI maps from MODIS data retrieved frequently in 2007 and compared them with an existing mortality map of beech forests in the study area ([3]). We examined the possibility of estimating the beech forest mortality rate and differences in evapotranspiration related to stomatal closure.
Materials and methods
Study area
The study area covered part of the Tanzawa Mountains of Japan (Fig. 1 - about 6300 km2: 138.62-139.40° E, 35.33-36.00° N). The Tanzawa Mountains include part of Kanagawa, Yamanashi and Shizuoka prefectures. Natural beech forests are preserved in the areas, which has been designated a Quasi-National Park. However, decline of the beech forests has become a serious problem since the 1980s and continues to spread. Koshiji et al. ([3]) reported that the extent of forest containing more than 201 dead trees per 1 km2 was about 55 km2 in 1985. Air pollution (mainly from ozone), water stress, and insect infestation are thought to be factors in this decline, but the main factors have not yet been disclosed yet and the mechanism of the decline remains unknown ([14]). Our study area included Tanzawa National Park where most of the decline has been reported (Fig. 1).
Fig. 1 - Location of the study area. Dotted line in the lower figure indicates Tanzawa-Oyama Quasi-National Park where serious beech forest decline is occurring.
Data
We used remote-sensing data obtained from the MODIS sensor aboard the Terra satellite. MODIS has 36 spectral bands (wavelength ranging from 0.40 to 14.4 μm) and supplies many kinds of data products. We used MOD09A1 data, which provide images of reflectance (8 bands, 459 to 2155 nm, with 500 m pixel resolution) and MOD11A2 data, which provide images of land surface temperature (with 1000 m pixel resolution). Each dataset is a composite of 8 days’ data to reduce the effects of clouds and noise. We used 46 data sets from 2007. In the study area, the number of pixels (with 500 m pixel resolution) was 24 820.
We used daily air temperature and elevation data observed at 10 meteorological stations (Automated Meteorological Data Acquisition System - AMeDAS) in the study area and a Global Digital Elevation Model (GDEM - with 30 m pixel resolution) obtained from the ASTER sensor abroad the Terra to produce air temperature maps.
Methods
The methodology is illustrated in the flow chart in Fig. 2. We made maps of NDVI, TVDI and iTVDI and compared them with a forest mortality map ([3]). For image processing, we reprojected these remote-sensing images and masked them to reduce the effect of cloud and noise. The mortality map showed the number of dead trees per 1 km2. Koshiji et al. ([3]) used aerial photography (with 25 cm pixel resolution) from 1985 and counted the number of dead trees by visual observation. The extent of the mortality was classified into three levels, low, moderate, or high. Low means that there were fewer than 201 dead trees per 1 km2, moderate means 201 to 400, and high means more than 401. Yamane et al. ([13]) conducted a ground survey in the Tanzawa Mountains in 2007 and suggested that the area where there had been more dead trees in 1985 represented stronger decline in 2007. Therefore, we used the 1985 mortality map as ground truth data for 2007.
Fig. 2 - Flow chart of the image processing procedure. MOD09A1 and MOD11A2 are MODIS data. AMeDAS data have daily temperature values and altitudes. GDEM is the Global Digital Elevation Model obtained by using ASTER. Ts is surface (soil and canopy) temperature, Ta is air temperature. Ground-truth data are GIS data on beech forest mortality in the Tanzawa Mountains ([3]).
NDVI maps
NDVI, which uses the reflectance in the near-infrared and red bands, is a popular index for vegetation monitoring. NDVI reflects both chlorophyll content and vegetation amount. MODIS observes these wavelengths as band 2 (841 to 876 nm - near infrared) and band 1 (620 to 670 nm - red). NDVI is calculated as follows (eqn. 1):
where RED
and NIR
represent the spectral reflectance measurements acquired in the red and near-infrared regions, respectively.
Maps of surface temperature and of surface temperature minus air temperature
Generally, surface temperature (Ts) and surface temperature minus air temperature (Ts - Ta) reflect evapotranspiration rates, because the Ts value is reduced by latent heat. Therefore, we can use Ts and (Ts - Ta) as indices of the evapotranspiration rates. (Ts - Ta) is the surface temperature corrected by Ta. We consider that this value works well in mountainous areas, where the effect of elevation on Ts cannot be ignored.
We made (Ts - Ta) maps to produce iTVDI maps, calculating the differences between the Ts and Ta maps. We obtained Ts maps from MODIS data and made the Ta maps from AMeDAS data and the GDEM. We calculated the daily air temperature value at each pixel by weighting the nearest four AMeDAS stations’ temperature values using the inverse distance-weighted algorithm between each pixel and each of the four stations, and we corrected the air temperature values by using the environmental lapse rate. We used 6.49 (°C / 1000 m) as the lapse rate, as defined by the International Standard Atmosphere model. Because the land surface temperature values obtained by MODIS are 8-day composites, we calculated 8-day mean air temperatures. We obtained 46 (Ts - Ta) map scenes and calculated their differences between them.
TVDI and iTVDI maps
TVDI and iTVDI are indices developed to estimate the evapotranspiration per each vegetation amount. These indices were developed from the water deficit index (WDI - [5]). WDI quantifies the rate of evapotranspiration from partial vegetation cover. In a vegetation environment where the potential maximum evapotranspiration rate is LEp and the actual one is LE, WDI is calculated by using eqn. 2. When the evapotranspiration rate is relatively small, the WDI value approaches 1 (eqn. 2):
where (Ts - Ta)obs is the surface temperature minus air temperature at the observation time, and (Ts - Ta)wet and (Ts - Ta)dry are (respectively) the minimum and maximum (Ts - Ta) for the same vegetation index value (e.g., NDVI) in the trapezoidal shape of the vegetation index vs. (Ts - Ta). WDI needs calibration using Penman-Monteith combined with energy balance equation ([4]). Therefore, in the WDI model, some of the inputs required to estimate the vertices of the trapezoid are difficult to assess (e.g., aerodynamic resistance, maximum and minimum canopy resistance, and soil heat flux).
Fig. 3 - Definition of iTVDI. Plots are calculated using the data from 1 to 9 May 2007. This trapezoidal shape shows the differences in potential evapotranspiration under each vegetation density. The iTVDI at point C is given by 1-BC/AB, as shown in eqn. 3.
TVDI and iTVDI were developed to apply the WDI principle to natural ecosystem studies on a large scale. These indices are retrieved by using satellite and meteorological data and by calculating the positions of two lines (Fig. 3, segments 1-2 and 3-4) that define the top and bottom of the plots of the Ts vs. NDVI space (for TVDI) and the (Ts - Ta) vs. NDVI space (for iTVDI). The theoretical shape resulting from (Ts - Ta) vs. NDVI that is used to derive iTVDI is presented in Fig. 3. The n vertices (n = 1, 2, 3, 4) in Fig. 3 indicate well-watered full-cover vegetation, full-cover vegetation suffering high water stress, saturated bare soil, and dry bare soil, respectively. Both TVDI and iTVDI indicate the relative position of Ts or (Ts - Ta) on a line on which NDVI remains constant. iTVDI at point C in Fig. 3 is calculated by using segments AB and BC as in eqn. 3 and TVDI is calculated by using the same principle as in eqn. 4 (eqn. 3, eqn. 4):
We selected a study area that included many characteristic environments, including forest, soil, and cropland. We positioned the right and left lines of the trapezoid to include 95% of all plots (mean of NDVI ± 2 standard deviations [SD]) and the top and bottom lines to delineate 10 plots including 99% of all the original study plots (mean of (Ts - Ta) ± 3 SD) at each step (by dividing the difference between the right and left edges into 10 parts - Fig. 3).
Both TVDI and iTVDI vary between 0 and 1. Values increased in environments where evapotranspiration rates are low. These values can be used to estimate differences in evapotranspiration rates between areas with the same vegetation amount. In mountainous areas such as the Tanzawa Mountains, environmental lapse rate correction should work well on iTVDI.
Results and discussion
Ta map
We made Ta maps from the data from the 10 AMeDAS stations and the GDEM data (example for the period July 28 to August 4, 2007 - Fig. 4). To verify the accuracy of the method, we made maps by using the data from nine of the stations and compared the estimated data with the observations at the 10th, excluded, station. The method calibrated air temperature with good accuracy (RMSE = 0.49 °C).
Fig. 4 - Ta map (average of 28 July to 4 August 2007) calculated from AMeDAS data and GDEM data. The region is the same as in Fig. 1.
Spatial comprehension between NDVI, TVDI and iTVDI
We focused on two temporal datasets, spring (May 1 to May 8, 2007), when the leaves emerge, and summer (July 28 to August 4, 2007) when the amount of leaf cover peaks. We show NDVI, TVDI, and iTVDI maps for summer (Fig. 5). The black pixels are areas where there are no data because of cloud masking. The NDVI values at almost all pixels were greater than 0.8. This suggested that the NDVI was unable to detect dead trees. In contrast, the TVDI and iTVDI maps had spatially varied values. The finding that the NDVI map had uniform values suggested that there were areas where the evapotranspiration rates were different despite the same vegetation amounts.
Fig. 5 - NDVI, TVDI, and iTVDI maps calculated from MODIS composite data obtained from 28 July to 4 August 2007. Black pixels are areas where there are no data because of cloud masking. The dotted region is a magnification of that in Fig. 1.
Statistical evaluation of NDVI, TVDI and iTVDI
We verified the NDVI, TVDI, and iTVDI values at the pixels as Low, Moderate, or High on the beech forest mortality map ([3] - Fig. 6). The data for both spring and summer showed that iTVDI had a better relationship with mortality rate than did NDVI or TVDI. As was found in the comparison of summer data among the three indices, NDVI values in summer were so high (near 0.9) that NDVI did not detect dead trees. TVDI values were unreliable in summer, because areas with low evapotranspiration rates should have had relatively low values.
Fig. 6 - Values of NDVI, TVDI and iTVDI in areas classified as Low, Moderate, and High in the mortality map in spring and summer. Columns marked by different letters differ significantly at P < 0.05 according one-way ANOVA followed by LSD (least significant difference) tests. Error bars show the standard error of the mean.
NDVI was accurate only for the spring data. TVDI did not work well in either season, but iTVDI worked well in both seasons. iTVDI detects differences in evapotranspiration in areas with the same vegetation amount. Thus, iTVDI detected well-covered areas where transpiration rates were low; these areas tended to have dead trees. The difference between TVDI and iTVDI showed that the effect of an elevation in Ts could not be ignored. Reduction in transpiration rates is likely attributable to stomatal closure caused by air pollution and water stress. iTVDI suggested the existence of areas on the map where transpiration rates were reduced by stomatal closure. Judging from the above, we found that indices calculated from the MODIS data and the meteorological data, especially iTVDI, could be used to estimate forest mortality rate in both spring and summer. iTVDI can thus be used to detect areas that are suffering a reduction in transpiration rates and are at risk of future decline. It should therefore be useful as an index for monitoring vegetation decline. Comparison with other remote-sensing studies of forests reveals that vegetation indices tend to be saturated in the high biomass region, and there has been hope for a vegetation index that sensitively reflects the vegetation status in such regions ([1]). We found that iTVDI worked well in high biomass regions such as those in the Tanzawa Mountains.
Conclusions
Diagnosis and monitoring of plant responses to environmental stress are important to our understanding and preservation of plant function. We tried to detect forest mortality rate in the Tanzawa Mountains by calculating NDVI, TVDI and iTVDI maps using MODIS data. NDVI was saturated and TVDI was unreliable in summer. In contrast, iTVDI worked well in both spring and summer and showed a good relationship with forest mortality rate.
iTVDI detects areas where evapotranspiration rates are relatively reduced and the robustness of this index makes it useful for monitoring mountainous areas. We therefore expect that it will be a good index for monitoring vegetation decline.
References
Gscholar
Gscholar
Gscholar
Gscholar
Gscholar
Gscholar
Gscholar
Gscholar
Authors’ Info
Authors’ Affiliation
Y Shimizu
P Rahimzadeh-Bajgiran
K Omasa
Department of Biological and Environmental Engineering, Graduate School of Agricultural and Life Sciences, University of Tokyo, 1-1-1 Yayoi, Bunkyo-ku, 113-8657 Tokyo (Japan)
Corresponding author
Paper Info
Citation
Ishimura A, Shimizu Y, Rahimzadeh-Bajgiran P, Omasa K (2011). Remote sensing of Japanese beech forest decline using an improved Temperature Vegetation Dryness Index (iTVDI). iForest 4: 195-199. - doi: 10.3832/ifor0592-004
Paper history
Received: Nov 30, 2010
Accepted: Jul 20, 2011
First online: Nov 03, 2011
Publication Date: Nov 03, 2011
Publication Time: 3.53 months
Copyright Information
© SISEF - The Italian Society of Silviculture and Forest Ecology 2011
Open Access
This article is distributed under the terms of the Creative Commons Attribution-Non Commercial 4.0 International (https://creativecommons.org/licenses/by-nc/4.0/), which permits unrestricted use, distribution, and reproduction in any medium, provided you give appropriate credit to the original author(s) and the source, provide a link to the Creative Commons license, and indicate if changes were made.
Web Metrics
Breakdown by View Type
Article Usage
Total Article Views: 56203
(from publication date up to now)
Breakdown by View Type
HTML Page Views: 47793
Abstract Page Views: 2807
PDF Downloads: 4194
Citation/Reference Downloads: 28
XML Downloads: 1381
Web Metrics
Days since publication: 4767
Overall contacts: 56203
Avg. contacts per week: 82.53
Article Citations
Article citations are based on data periodically collected from the Clarivate Web of Science web site
(last update: Feb 2023)
Total number of cites (since 2011): 7
Average cites per year: 0.54
Publication Metrics
by Dimensions ©
Articles citing this article
List of the papers citing this article based on CrossRef Cited-by.
Related Contents
iForest Similar Articles
Research Articles
Sensitivity analysis of RapidEye spectral bands and derived vegetation indices for insect defoliation detection in pure Scots pine stands
vol. 10, pp. 659-668 (online: 11 July 2017)
Research Articles
Trends and driving forces of spring phenology of oak and beech stands in the Western Carpathians from MODIS times series 2000-2021
vol. 16, pp. 334-344 (online: 19 November 2023)
Review Papers
Remote sensing-supported vegetation parameters for regional climate models: a brief review
vol. 3, pp. 98-101 (online: 15 July 2010)
Research Articles
Landsat TM imagery and NDVI differencing to detect vegetation change: assessing natural forest expansion in Basilicata, southern Italy
vol. 7, pp. 75-84 (online: 18 December 2013)
Research Articles
Assessing the performance of MODIS and VIIRS active fire products in the monitoring of wildfires: a case study in Turkey
vol. 15, pp. 85-94 (online: 19 March 2022)
Research Articles
Modeling aboveground carbon in flooded forests using synthetic aperture radar data: a case study from a natural reserve in Turkish Thrace
vol. 17, pp. 277-285 (online: 27 September 2024)
Research Articles
Mapping Leaf Area Index in subtropical upland ecosystems using RapidEye imagery and the randomForest algorithm
vol. 7, pp. 1-11 (online: 07 October 2013)
Review Papers
Accuracy of determining specific parameters of the urban forest using remote sensing
vol. 12, pp. 498-510 (online: 02 December 2019)
Research Articles
Fuel type characterization based on coarse resolution MODIS satellite data
vol. 1, pp. 60-64 (online: 28 February 2008)
Technical Reports
Detecting tree water deficit by very low altitude remote sensing
vol. 10, pp. 215-219 (online: 11 February 2017)
iForest Database Search
Search By Author
Search By Keyword
Google Scholar Search
Citing Articles
Search By Author
Search By Keywords
PubMed Search
Search By Author
Search By Keyword