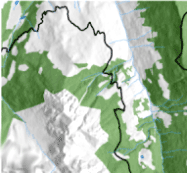
Remote sensing-supported vegetation parameters for regional climate models: a brief review
iForest - Biogeosciences and Forestry, Volume 3, Issue 4, Pages 98-101 (2010)
doi: https://doi.org/10.3832/ifor0543-003
Published: Jul 15, 2010 - Copyright © 2010 SISEF
Review Papers
Collection/Special Issue: NFZ Summer School 2009 - Birmensdorf (Switzerland)
Long-term ecosystem research: understanding the present to shape the future
Guest Editors: Marcus Schaub (WSL, Switzerland)
Abstract
Land surface plays a key role in a climate system. Thus, the land surface description will become increasingly important for climate modelling by its feedbacks on the climate. Various forms of active/passive remotely sensed data are nowadays being used to provide continuous and up-to-date information on the earth’s surface on both global and regional scales. This information is useful to be included in climate models. This review summarizes how LAI and albedo, two of the most important land surface parameters, could be derived from remote sensing. Whereas the high acquisition frequency, accessibility, and spatial continuality are referred to potential advantages, the scaling is still a drawback which may cause further problems such as incompatibility of different remote sensing data sources for a specific climate model. Moreover, issues like shadow and atmospheric effects are often problematic, especially when optical remote sensing is applied. Here, suggestions for improvement are made and open questions are pointed out.
Keywords
Regional climate models, Forest and vegetation parameters, Active and passive remote sensing
Introduction
Climate projections for the 21st century show an increase in near surface temperatures across Europe ([9], [7]). The probability of extreme events, i.e., floods, heat waves, and droughts, are also predicted to increase near in the future (e.g., [5], [35], [36]). Modelling studies have shown the influence of land surface characteristics on climate simulations ([30], [4]) that can alter the climate change signal. On a finer scale, the land surface description will become increasingly important due to feedback effects on the local climate. Information about land cover can be gathered from either terrestrial measurements or remote sensing.
Following the launch of the first earth observation satellite in 1972, information extracted from remote sensing has been applied by environmental scientists as a continuous and up-to-date source of spectral/spatial data to represent different attributes of the earth surface in general, as well as vegetation cover in particular. The present review focuses on integrating monitoring and remote sensing methods for improved representation of forest cover in climate models. Two important vegetation-related land-cover attributes including Leaf Area Index (LAI) and background albedo (i.e., the albedo of a snow-free surface) have been selected to illustrate how they could be derived using methods based on airborne/space borne remote sensing data. In addition, an example is given of their practical application in a regional climate model.
The role of LAI and Albedo in the land surface processes
Climate determines the spatial distribution of forests, which is affected by climate change (e.g., [27]). In turn, forests modify water and energy exchanges between land and atmosphere due to their Leaf Area Index (LAI), lower albedo and higher roughness length. Here, LAI and albedo have been selected as two key parameters in climate models which can be routinely derived from remote sensing data products. According to NASA definition, LAI is the one-sided green leaf area per unit ground area in broadleaf vegetation canopies, which is approximated by half the total needle surface area in coniferous canopies (see NASA-LAI - ⇒ http://modis-land.gsfc.nasa.gov/lai.htm). In climate models, LAI influences evapotranspiration and the precipitation interception capacity, which are the major components of the surface thermal and moisture balance of the hydrological cycle. It is a driver for many other vegetation exchange processes such as photosynthesis ([39]) and carbon flux ([14]). LAI is also a major indicator of stress in forests.
Solar radiation is the main source of energy for the global climate system. After interacting with the atmosphere, where the radiation is partly absorbed and partly scattered, a fraction of the incoming solar radiation is absorbed at the earth’s surface. The remainder is reflected back to the atmosphere. The ratio of this reflected energy to the incoming radiation is called the surface albedo ([31]). Albedo describes radiation processes at the atmosphere-land surface boundary and determines the energy budget of the earth’s surface in the models.
Deriving LAI from remote sensing
Remote sensing data and techniques can complement existing ground-based measurement of LAI. In other words, measurements extracted from remotely sensed data are generally referred to as “indispensable components”, which are necessary for modelling and simulating ecological variables and processes ([41]). The consistency of LAI over different spatial resolutions makes it an ecologically-relevant parameter for multi-scale research that ranges from leaf to landscape, and to regional scales ([40]).
Both active (detection of the reflected or emitted electromagnetic radiation from natural energy sources) and passive (detection of reflected responses from objects that are irradiated from artificially-generated energy sources) remote sensing forms have been used to retrieve LAI on various spatial scales. Despite the possible biases in estimating LAI as a result of shadow effects in forest canopies, airborne optical sensors have been widely used for LAI extraction from fine-scale airborne imagery, either for modelling the time-course of LAI (e.g., [20], [10]) or to correlate the remotely sensed spectral features and LAI measured on the ground (e.g., [38], [28], [22]).
To relate LAI and different pixel-based vegetation indices (e.g., Normalized Difference Vegetation Index), various regression techniques have been applied. These techniques estimate LAI from the spectral indices like NDVI on a regional and landscape scale, mainly using visible/infrared Landsat data ([6], [37]). Though the use of satellite data in LAI retrieval may be affected by problems such as the nonlinearity of the LAI/vegetation index relationship (which forces the use of non- linear models) or background reflections in pixel values (which might cause biased LAI estimation), medium resolution satellite data are extensively used as a valuable LAI source for regional climate models.
Active remote sensing forms, i.e., Radio Detection and Ranging (RADAR) and more recently Light Detection and Ranging (LiDAR), have also been used to obtain useful information about the structural and physical dimensions of forests and other types of land cover. To ensure the independence of active remote sensing systems from cloud cover and the saturation of vegetation index, they are generally used instead of (or together with) optical remote sensing forms. Only occasionally, RADAR data ([3]) as well as LiDAR ([21], [29]) have been used in LAI retrieval on regional scales. Pang et al. ([29]) found that different forest types (needle leaf and broadleaf) show different relationships between LiDAR return ratio and LAI. Yet, they evaluated the data as a suitable source for large scale mapping of LAI in forests, as it was strongly correlated to field-measured LAI.
Background albedo from remote sensing
Although point measurements can be made with field observations, remote sensing offers a reliable and accurate way to estimate albedo on a regional scale. Given appropriate atmospheric modelling tools, optical remote sensing instruments can measure the surface reflectance in particular directions and illumination angles dictated by the scanning mechanism and the trajectory characteristics of the platform. Lewis et al. ([23]) used the data from an Airborne Solid-state Imaging Spectrometer (ASAS) to estimate integrated reflectance properties related to albedo. The results were compared with the results of a linear model of surface bi-directional reflectance and validated using the field-recorded albedo measurements. The ASAS-derived albedo agreed well with those collected in the field.
Surface albedo has also been derived from medium-resolution remote sensing features (mainly in the visible/near infrared domain). This has been tested, optimized and calibrated by other studies to be used in regional climate models (e.g., [25], [18], [26], [34]).
Albedo has already been estimated on micro scale. However, its values tend to vary as the scale becomes coarser. This makes it difficult to model it on macro scales, particularly when terrestrial ecosystems are monitored. For example, Liu et al. ([24]) derived course resolution albedo on a 1-km scale Advanced Very High Resolution Radiometer (AVHRR) data using the information extracted from 30-m resolution Thematic Mapper (TM) by two methods including arithmetic averaging of the albedo values retrieved from TM data and direct retrieval from AVHRR data. Using a corrective algorithm, they managed to considerably reduce the scaling errors on a regional course scale.
Land surface representation in the regional climate model REMO: a practical example
The REgional climate MOdel (REMO) is a three dimensional hydrostatic model for the atmosphere ([15], [16]). The model’s prognostic variables include surface pressure, horizontal wind components, temperature, water vapour and cloud water content. REMO has been successfully tested and validated for several countries and regions (e.g., [32], [17], [13]) with horizontal resolutions of 10 km, 20 km, and 50 km.
Land surface processes in REMO are controlled by physiological vegetation properties such as LAI, fractional vegetation cover, background albedo, surface roughness length due to vegetation, forest ratio, and soil water holding capacity. For each land cover type, these vegetation fields are allocated in the global dataset of Land Surface Parameters ([11], [12]). This information is aggregated to the model grid scale, by averaging the vegetation parameters of all land cover types located in each grid cell, weighted by their fractional areas within the cells.
Whereas albedo, LAI, and fractional vegetation cover display an annual cycle, the other parameters remain constant throughout the year. The seasonal variation in the LAI between its minimum and maximum value is provided using the monthly growth factor ([12]), which is defined by the fraction of absorbed Photosynthetically Active Radiation (FPAR) in low and mid-latitudes. A global distribution of FPAR has been derived from Berthelot et al. ([1]), based on NOAA-AVHRR data ([19]).
For the parameterization of background albedo, global distributions of soil and vegetation albedo have been produced ([33]). They are derived from remotely sensed MODIS (Moderate-Resolution Imaging Spectroradiometer) products of the albedo for total short-wave broad bands and the FPAR. The separated soil and vegetation albedo database can be applied in climate modelling to describe the annual background albedo cycle as a function of the monthly-varying LAI ([33]).
As well as many other regional climate models, REMO works with fixed vegetation parameters per month, which have to be tested by comparing against periodical field observations or fine-scale remote sensing data. For example, Blümel & Reimer ([2]) calculated high-resolved LAI values of NOAA data for validation and compared them to the fixed LAI values which were used in the REMO model. The results demonstrated the vegetation development within the land-use classes well. In addition to the seasonal variations and the influence of various weather conditions on vegetation growth was also reflected in the calculated LAI values.
Discussion and conclusion
The practical importance of adequate representation of forest cover in regional climate models is increasing with land cover change through climate change, natural vegetation dynamics, or land use that have been shown to greatly affect climate.
Field observations play a crucial role in understanding water and energy exchange processes and validating the results of climate models. But measuring albedo and LAI on longer time scales and over large regions requires a great effort. Most observed data in a specific area are hardly adaptable for other regions. Alternatively, the basic advantages of remote sensing techniques against field observations are the following:
- They can provide continuous information over large areas, whereas field observations are carried out only at selected stations.
- Depending on the type of commercial sensor and spatial resolution, collection intervals of remote sensing data range from daily to monthly, whereas field measurements cover often short-term periods.
However, there are some uncertainties which may cause biases in LAI estimation using remote sensing data, including shadow and atmospheric effects in forest canopies (particularly in case of optical data), non- linearity of LAI/vegetation index relationships, and background reflections in NDVI values.
The spectral and radiometric problems can be reduced integrating the new active remote sensing. High-density 3D topographic information (e.g., Airborne Laser Scanning) and sub-canopy and cloud-independent information on stand structure (e.g., RADAR systems) could be the most important areas for further development in this field. Although LiDAR height metrics improve the LAI estimation in forested areas ([29]), the use of them is still associated with high acquisition costs, especially when gathering small footprint data.
To overcome the possible non-linearities in regression analysis, the application of distribution-free models, i.e., non-parametric methods, could be effective when spatial prediction of LAI from remote sensing predictors is of particular interest. The moderate-resolution imagery, when combined with relevant indices (e.g., Soil Reflectance Index), were shown to be accurate predictors for non-parametric modelling of LAI in regional scales ([8]).
For the regional climate model REMO, the following remote sensing-supported improvements would be useful for forest cover description:
- Forest-types could be distinguished in detail using commercial remote sensing data featuring higher spectral/spatial resolution. As a consequence it may also be possible to allocate the annual cycle of albedo and LAI to each forest type. Therefore, regional differences in LAI and albedo of the different forest types could be shown.
- Annual cycles of other important vegetation parameters, e.g., roughness length due to vegetation, could be described.
As a drawback, the scaling (resolution) problem is one of the existing challenges to find the most suitable remote sensing data for climate modelling purposes. For example, LAI is consistent over different spatial resolutions ([41]), whereas albedo values show a relatively high variability in coarser scales ([24]) and depend on various factors (e.g., solar zenith angle, satellite sensors, imaging time, local soil characteristics, and soil moisture content).
Thus, a synthesis of research methods, i.e., to obtain the best data/area compatibility at the least costs, seems a future challenge to develop an effective remote sensing-supported characterization of vegetation attributes for climate models. More intense international collaboration in improving remote sensing and incorporating the resulting information into climate models is therefore essential.
Acknowledgements
This review has been written as final assignment of the International Summer School “HOWTOPC” organized by the “Nancy-Freiburg-Zürich” forest network and the Swiss Federal Research Institute of Forest, Snow and Landscape (WSL), in summer 2009, in Zürich-Switzerland. The authors would like to thank the reviewers for their valuable comments on the manuscript.
References
Gscholar
CrossRef | Gscholar
CrossRef | Gscholar
Gscholar
Gscholar
Gscholar
Gscholar
CrossRef | Gscholar
Gscholar
Gscholar
Gscholar
Gscholar
Gscholar
Gscholar
Gscholar
Authors’ Info
Authors’ Affiliation
Dept. of Remote Sensing and Landscape Information Systems, University of Freiburg, Tennenbacher straße 4, D-79106 Freiburg (Germany)
Institute of Environment and Earth Sciences, Faculty of Forestry, University of West Hungary, 9400 Sopron (Hungary)
Corresponding author
Paper Info
Citation
Latifi H, Galos B (2010). Remote sensing-supported vegetation parameters for regional climate models: a brief review. iForest 3: 98-101. - doi: 10.3832/ifor0543-003
Academic Editor
Marcus Schaub
Paper history
Received: May 25, 2010
Accepted: May 31, 2010
First online: Jul 15, 2010
Publication Date: Jul 15, 2010
Publication Time: 1.50 months
Copyright Information
© SISEF - The Italian Society of Silviculture and Forest Ecology 2010
Open Access
This article is distributed under the terms of the Creative Commons Attribution-Non Commercial 4.0 International (https://creativecommons.org/licenses/by-nc/4.0/), which permits unrestricted use, distribution, and reproduction in any medium, provided you give appropriate credit to the original author(s) and the source, provide a link to the Creative Commons license, and indicate if changes were made.
Web Metrics
Breakdown by View Type
Article Usage
Total Article Views: 57643
(from publication date up to now)
Breakdown by View Type
HTML Page Views: 48264
Abstract Page Views: 3840
PDF Downloads: 4280
Citation/Reference Downloads: 44
XML Downloads: 1215
Web Metrics
Days since publication: 5483
Overall contacts: 57643
Avg. contacts per week: 73.59
Article Citations
Article citations are based on data periodically collected from the Clarivate Web of Science web site
(last update: Mar 2025)
Total number of cites (since 2010): 7
Average cites per year: 0.44
Publication Metrics
by Dimensions ©
Articles citing this article
List of the papers citing this article based on CrossRef Cited-by.
Related Contents
iForest Similar Articles
Review Papers
Accuracy of determining specific parameters of the urban forest using remote sensing
vol. 12, pp. 498-510 (online: 02 December 2019)
Review Papers
Remote sensing of selective logging in tropical forests: current state and future directions
vol. 13, pp. 286-300 (online: 10 July 2020)
Technical Reports
Detecting tree water deficit by very low altitude remote sensing
vol. 10, pp. 215-219 (online: 11 February 2017)
Review Papers
Remote sensing support for post fire forest management
vol. 1, pp. 6-12 (online: 28 February 2008)
Research Articles
Assessing water quality by remote sensing in small lakes: the case study of Monticchio lakes in southern Italy
vol. 2, pp. 154-161 (online: 30 July 2009)
Research Articles
Afforestation monitoring through automatic analysis of 36-years Landsat Best Available Composites
vol. 15, pp. 220-228 (online: 12 July 2022)
Technical Reports
Remote sensing of american maple in alluvial forests: a case study in an island complex of the Loire valley (France)
vol. 13, pp. 409-416 (online: 16 September 2020)
Research Articles
Mapping the vegetation and spatial dynamics of Sinharaja tropical rain forest incorporating NASA’s GEDI spaceborne LiDAR data and multispectral satellite images
vol. 18, pp. 45-53 (online: 01 April 2025)
Review Papers
Analysis of full-waveform LiDAR data for forestry applications: a review of investigations and methods
vol. 4, pp. 100-106 (online: 01 June 2011)
Research Articles
Identification and characterization of gaps and roads in the Amazon rainforest with LiDAR data
vol. 17, pp. 229-235 (online: 03 August 2024)
iForest Database Search
Search By Author
Search By Keyword
Google Scholar Search
Citing Articles
Search By Author
Search By Keywords
PubMed Search
Search By Author
Search By Keyword