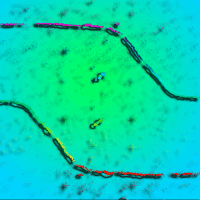
Identification and characterization of gaps and roads in the Amazon rainforest with LiDAR data
José Augusto Spiazzi Favarin (1) , Mateus Sabadi Schuh (2), Juliana Marchesan (2), Elisiane Alba (3), Rudiney Soares Pereira (4)
iForest - Biogeosciences and Forestry, Volume 17, Issue 4, Pages 229-235 (2024)
doi: https://doi.org/10.3832/ifor4295-017
Published: Aug 03, 2024 - Copyright © 2024 SISEF
Research Articles
Abstract
Gap formations in the forest canopy have natural causes, such as bad weather, and anthropic ones, such as sustainable selective extraction of trees and illegal logging, which can already be detected through orbital remote sensing. However, the Amazon region is under frequent cloud cover, which makes it challenging to detect gaps using passive sensors. This study aimed to identify and delimit gaps in the Amazon forest canopy through airborne LiDAR (Light Detection and Ranging) sensor application while testing six different return densities. LiDAR and forest inventory data were obtained over an Amazon rainforest region, defining the minimum area as a forest canopy gap. The point cloud was processed to obtain six return densities with the generation of their respective CHM (Canopy Height Model), which were applied for segmentation and subsequent identification of gap areas and roads. The minimum gap area found was 34 m2, and the Kruskal Wallis test showed no significant difference among the six densities in gap detection; however, road identification decreased as the return density decreased. We concluded that LiDAR data proved promising as point clouds with low return density can be used without impairing gap identification. However, reducing the return density for road identification is not recommended.
Keywords
Forest Canopy Gaps, Aerial Laser Scanning, Point Density, Remote Sensing
Authors’ Info
Authors’ address
Postgraduate Program in Forest Sciences, Federal University of Paraná /UFPR, Lothário Meissner Avenue, 632, Jardim Botnico, 80210-170, Curitiba, PR (Brazil)
Juliana Marchesan 0000-0002-2167-5862
Postgraduate Program in Forest Engineering, Federal University of Santa Maria/UFSM, Roraima Avenue, 1000, Camobi, 97105-900, Santa Maria, RS (Brazil)
Academic Unity of Serra Talhada, Federal Rural University of Pernambuco/UFRPE, Gregório Ferraz Nogueira Av., 56909-535, Serra Talhada, PE (Brazil)
Rural Engineering Department, Federal University of Santa Maria/UFSM, Roraima Avenue, 1000, Camobi, 97105-900, Santa Maria, RS (Brazil)
Corresponding author
Paper Info
Citation
Spiazzi Favarin JA, Sabadi Schuh M, Marchesan J, Alba E, Soares Pereira R (2024). Identification and characterization of gaps and roads in the Amazon rainforest with LiDAR data. iForest 17: 229-235. - doi: 10.3832/ifor4295-017
Academic Editor
Matteo Garbarino
Paper history
Received: Dec 27, 2022
Accepted: Jun 11, 2024
First online: Aug 03, 2024
Publication Date: Aug 31, 2024
Publication Time: 1.77 months
Copyright Information
© SISEF - The Italian Society of Silviculture and Forest Ecology 2024
Open Access
This article is distributed under the terms of the Creative Commons Attribution-Non Commercial 4.0 International (https://creativecommons.org/licenses/by-nc/4.0/), which permits unrestricted use, distribution, and reproduction in any medium, provided you give appropriate credit to the original author(s) and the source, provide a link to the Creative Commons license, and indicate if changes were made.
Web Metrics
Breakdown by View Type
Article Usage
Total Article Views: 8235
(from publication date up to now)
Breakdown by View Type
HTML Page Views: 5697
Abstract Page Views: 1029
PDF Downloads: 1298
Citation/Reference Downloads: 0
XML Downloads: 211
Web Metrics
Days since publication: 331
Overall contacts: 8235
Avg. contacts per week: 174.15
Citation Metrics
Article Citations
Article citations are based on data periodically collected from the Clarivate Web of Science web site
(last update: Mar 2025)
(No citations were found up to date. Please come back later)
Publication Metrics
by Dimensions ©
Articles citing this article
List of the papers citing this article based on CrossRef Cited-by.
References
Analysis and prediction of gap dynamics in a secondary deciduous broadleaf forest of Central Japan using airborne multi-LiDAR observations. Remote Sensing 13: 100.
Gscholar
Treefalls: frequency, time, and consequences. In: “The Ecology of a Tropical Forest: Seasonal Rhythms and Long-Term Changes” (Leight EG, JR, Rand AS, Windsor DM eds). Smithsonian Institute Press, Washington, DC, USA, pp. 101-108.
Gscholar
Efeitos do manejo florestal na estrutura da avifauna na floresta Amazônica de Paragominas (Pará) [Effects of forest management on the structure of the avifauna in the Amazon forest of Paragominas (Pará)]. PhD thesis, Escola Superior de Agricultura Luiz de Queiroz, Universidade de São Paulo, Piracicaba, SP, Brazil, pp. 108. [in Portuguese]
Gscholar
Renascimento de florestas: regeneração da era do desmatamento [Rebirth of forests: regeneration from the era of deforestation]. Oficina de Textos, São Paulo, Brazil, pp. 430. [in Portuguese]
Gscholar
Construção de estradas florestais e transporte florestal rodoviário na região amazônica. Circular Técnica 6 [Construction of forest roads and road forest transport in the Amazon region. Technical Circular 6]. EMBRAPA/CPATU, Belém, PA, Brazil, pp. 30. [in Portuguese]
Gscholar
Applying high-resolution UAV-LiDAR and quantitative structure modelling for estimating tree attributes in a crop-livestock-forest system. Land 11 (4): 507.
CrossRef | Gscholar
Levantamento de recursos minerais. Folha SA. 23 - São Luís e parte da folha SA. 24 - Fortaleza. Geologia, geomorfologia, solos, vegetação e uso potencial da terra - vol. 3 [Survey of mineral resources. Sheet SA. 23 - São Luís and part of sheet SA. 24 - Fortaleza. Geology, geomorphology, soils, vegetation, and potential land use - vol. 3]. Departamento Nacional de Produção Mineral - DNPM, Ministério de Minas e Energia, Rio de Janeiro, Brazil. [in Portuguese]
Gscholar
Programa internacional reúne cientistas em evento sobre uso de dados LiDAR e inventários florestais [International program brings together scientists at an event on the use of LiDAR data and forest inventories]. Empresa Brasileira de Pesquisa Agropecuária - EMBRAPA, Brasília, Brazil, web site. - [in Portuguese]
Online | Gscholar
Sucessão florestal e as florestas brasileiras: Conceitos e problemas [Forest succession and Brazilian forests: Concepts and problems]. In: Proceedings of the “VIII Congresso de Ecologia do Brasil”. Caxambu (MG, Brazil) 23-28 Sept 2007. Sociedade de Ecologia do Brasil, Caxambu, MG, Brazil, pp. 2. [in Portuguese]
Online | Gscholar
Sobrevivência de espécies arbóreas plantadas em clareiras causadas pela colheita de madeira em uma floresta de terra firme no município de Paragominas na Amazônia brasileira [Survival of seedlings planted in gaps after harvesting in a terra firme rain forest in Paragominas region in the Brazilian Amazon]. Acta Amazonica 40 (1): 171-178.
CrossRef | Gscholar
An international comparison of individual tree detection and extraction using airborne laser scanning. Remote Sensing 4 (4): 950-974.
CrossRef | Gscholar
FUSION/LDV: software for LIDAR data analysis and visualization. USDA Forest Service, Washington, DC, USA.
Gscholar
Automated method for measuring the extent of selective logging damage with airborne LiDAR data. ISPRS Journal of Photogrammetry and Remote Sensing 139: 228-240.
CrossRef | Gscholar
Conhecendo o R - Uma visão mais que Estatística [Knowing R - A vision more than Statistics]. UFV, Viçosa, MG, Brazil, pp. 222. [in Portuguese]
Gscholar
Using high-density UAV-LiDAR for deriving tree height of Araucaria angustifolia in an urban Atlantic rain forest. Urban Forestry and Urban Greening 63: 127197.
CrossRef | Gscholar
Mudanças climáticas e Amazônia [Climate change and the Amazon]. Ciência e Cultura 59: 22-27. [in Portuguese]
Gscholar
Levantamento de Recursos Naturais - Folhas SF. 23/24 Rio de Janeiro/Vitória: geologia, geomofologia, pedologia, vegetação e uso potencial da terra [Survey of Natural Resources - Sheets SF. 23/24 Rio de Janeiro/ Vitória: geology, geomorphology, pedology, vegetation, and potential land use]. Ministério de Minas e Energia, Rio de Janeiro, Brazil, pp. 775. [in Portuguese]
Gscholar
Assessing forest gap dynamics and growth using multi-temporal laser-scanner data. International Archives of Photogrammetry, Remote Sensing and Spatial Information Sciences 34 (8-W2): 173-178.
Gscholar
Canopy gap detection and analysis with airborne laser scanning. In: “Forestry Applications of Airborne Laser Scanning”, vol. 27 (Maltamo M, Næsset E, Vauhkonen J eds). Springer, Dordrecht, Netherlands, pp. 419-437.
Gscholar