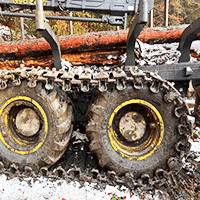
Exploring the reliability of CAN-bus data in assessing forwarder rolling resistance under real working conditions
iForest - Biogeosciences and Forestry, Volume 17, Issue 6, Pages 360-369 (2024)
doi: https://doi.org/10.3832/ifor4687-017
Published: Nov 13, 2024 - Copyright © 2024 SISEF
Research Articles
Abstract
The interaction between off-road vehicles and terrain in forestry operations has been extensively studied to assess machine performance and soil damage, emphasizing the importance of the relationship between machine mobility and terrain conditions. This study assesses the rolling resistance coefficient (μr) using engine data acquired through CAN-bus systems and the J1939 standard. The aim is to determine whether soil-machine interactions can be detected by modeling rolling resistance coefficients with a simple approach based on machine parameters and essential terrain characteristics. The study was conducted on a forwarder (John Deere® 1210G) across different terrain surfaces and load conditions. CAN-bus data were processed, while terrain characteristics and slope were determined using high-accuracy spatial data. The activities consisted of (i) a calibration test to evaluate the model’s sensitivity and (ii) a field test in a real working scenario. The developed methodology demonstrated sufficient sensitivity to detect increasing rolling resistance values on rougher surfaces, highlighting the impact of surface type on forwarder operations. Field tests revealed lower rolling resistance values for the unloaded forwarder (between 0.15 and 0.3) than loaded conditions (from 0.4 to 0.6). The model reliably captured μr changes between consecutive drives and skids, particularly during uphill operations, with significant differences influenced by trail conditions and forwarder interactions rather than just load. By providing a practical methodology for assessing off-road machine performance and its impact on driving surfaces, the study highlights the importance of understanding off-road vehicle dynamics for informed operation planning decisions. This study underscores that integrating real-time mobility data from CAN-bus technology with terrain analysis enhances operational efficiency and helps minimize soil damage, thereby supporting more sustainable forest management practices.
Keywords
Soil, Forest Operations, Rolling Resistance Coefficient, Forwarder, Efficiency, Logging, J1939, CAN-bus
Introduction
Complex terrain, characterized by the presence of obstacles and/or the specific physical-mechanical properties of the forest soil, presents significant challenges for ground-based extraction in forestry, requiring specific equipment and/or best practices to ensure machine performance under diverse environmental conditions ([28]). Therefore, optimizing machine performance while minimizing environmental impact is crucial for sustainable forestry practices ([47]).
Mechanized logging operations with ground-based equipment, prevalent in European production forestry, are known to cause soil impacts ([9], [34], [26]), especially on wet soils with low bearing capacity ([19]). Consequently, the success of these operations relies on the machinery’s ability to navigate challenging environments characterized by deformable soil, uneven terrain, slopes, and various natural features. The intricate relationship between the vehicle and the terrain, which depends on the topography, the soil characteristics, the vehicle itself, and its operation, makes it challenging to forecast the vehicle’s behavior in such circumstances ([53]). Therefore, optimal working conditions and traction machine configurations are essential to address these challenges effectively ([37]). Shorter periods of freeze and thaw cycles and wet or water-saturated soil conditions create vulnerable soils with restricted trafficability ([52]). Consequently, heavy machinery is more likely to cause lasting site damage, including soil rutting or compaction ([40], [32], [27]). On steeper slopes, these damages can lead to channeling, intensifying erosional energy and worsening the loss of valuable topsoil ([49], [46], [25]). These severe soil impacts have numerous adverse effects, including both economic and ecological aspects. For example, operating machinery on soft grounds demands more power, increasing fuel consumption, elevating machine wear, and increasing the risk of technical failures ([3], [31]). Additionally, soil compaction caused by heavy machinery can delay seedling growth by shortening root development and reducing water uptake, which weakens plant physiological performance and disrupts forest regeneration processes ([10], [29]).
Nevertheless, the fully mechanized Cut-to-Length (CTL) timber harvesting system is frequent across most European countries and employed on various soils and topographies ([38]). This widespread application highlights the relevance of addressing the broader implications of forwarder use globally, for instance, enabling machine operators to know which trail segments to avoid during specific operation times to ensure forest stand accessibility ([23]). Anticipating areas and sites highly susceptible to machine impact improves the spatial forecasting of risks for severe damages. This concerns not only extensive off-road traffic during clear-cut operations, prevalent in Nordic European countries but also single-selection silviculture systems, frequently employed in Central Europe, where minimizing soil impacts is crucial, as machine traffic is restricted to permanent operation trails ([19]). Studies from North America ([24]), Asia ([18]), and other regions have also highlighted the critical role of forwarders in diverse environmental conditions. In this context, forestry vehicles play a crucial role in mechanized logging operations in various terrains, emphasizing high mobility’s importance in effectively navigating low-bearing-capacity soil and sloped terrain ([13]). Investigative methods aimed at reducing ground damage caused by forest machinery ([6], [14], [48]), including innovative designs of forest machines ([41], [23], [37]), and predictive planning tools based on terrain indices ([15], [1], [12], [43], [51]) have been reported since the early 2000s. Past research emphasized the importance of considering terrain difficulty, water bodies, and timber extraction directions for effectively planning forest road networks and timber extraction strategies ([11], [13]). Nowadays, combining data from several sources, such as machines, terrain, and weather, allows detecting the impact of operational conditions on machine performance indicators, such as fuel consumption ([17]). Moreover, modern forest machines equipped with CAN-bus, diesel engines, and hydrostatic transmissions provide practical data for trafficability mapping, aiding in route planning and operation optimization ([50], [2], [3], [31]). The rolling resistance coefficient (μr) is one of the most critical metrics in analyzing CAN-bus data ([30], [43]). This coefficient indicates vehicle performance and terrain dynamics, enabling informed decision-making in forest operations. Monitoring rolling resistance over time allows for assessing long-term machine efficiency and supports route planning ([42]). Validating the accuracy of μr data allows a deeper understanding of how operational variables impact rolling resistance dynamics. Moreover, a series of practical advantages to utilizing the rolling resistance coefficient in assessing operation performance can be recognized. Firstly, it explains off-road vehicle performance under varying driving surfaces. Integrating rolling resistance data with spatially detailed models, such as those used in rut depth prediction, can generate accurate, site-specific trafficability assessments, minimizing soil degradation and optimizing operational efficiency ([21]). Furthermore, modeling μr over space and time allows for the dynamic adjustment of operational conditions, thus improving the adaptability and reliability of trafficability forecasts ([43]). Finally, our work investigates the applicability and comparability of the rolling resistance coefficient as a parameter for assessing vehicle driving conditions and soil interaction, specifically of a forwarder, in a real working case scenario. Previous studies ([50], [2]) focused on modeling the relationship between operational machine (i.e., harvester and forwarder) variables and environmental data under controlled conditions. However, our study seeks to expand existing research by examining how rolling resistance behaves during forwarding operations in real-world conditions, specifically with varying weights and repeated passes on the same trail. Moreover, we aim to evaluate the effectiveness of the CAN-bus system as a cost-effective tool for data collection in forest machine operations and its capability to provide valuable data for modeling the rolling resistance coefficient (μr) across diverse terrain conditions. By analyzing rolling resistance in various operational and environmental settings, this study aims to validate the reliability of μr as a practical parameter for assessing machine performance and soil interaction on a broader scale beyond controlled test environments. The methodology developed is intended to provide a representative sample of forest ground and various operations, thereby enhancing the robustness and applicability of the proposed methods across varied scenarios and improving strategic planning of timber extraction activities. The study was conducted in several phases: (i) initially, μr was determined by analyzing CAN-bus data collected on a controlled site; (ii) crucial factors influencing the forwarder’s rolling resistance on different surfaces were identified; and (iii) the reliability of CAN-bus data in a real-case logging operation was tested by applying our methodology on different skid trails under actual working conditions on sloped terrain, thus adding practical relevance to the study. To test the reliability of μr modeling on each skid trail at different forwarder loading statuses and across consecutive cycles on the same skid, we assessed μr changes within and across the different scenarios. Therefore, we hypothesized that: (i) during the first cycle (C1) on uphill trails under unloaded (U) conditions, there would be no significant differences in the average μr values, indicating the stability of the rolling resistance coefficient; (ii) rolling resistance (μr) would exhibit measurable changes between the first (C1) and second (C2) cycles, as well as between unloaded (U) and loaded (L) forwarder conditions.
This would demonstrate the method’s sensitivity to variations in operational contexts and support the use of μr as a reliable indicator of machine-soil interaction in real-world forestry operations.
Materials and methods
Study areas
Two tests at different times and locations were done to evaluate the proposed methodology for quantifying and assessing the forwarder rolling resistance coefficient and its spatial distribution (Fig. 1). The field test (Panel 2) took place in November 2022 (51° 21′ N, 08° 01′ E in WGS84), while the calibration test (Panel 1) in June 2023 (51° 27′ N, 07° 59′ E). Both studies were conducted in North Rhine-Westphalia, central Germany, and the different locations served as study areas for assessing the method’s applicability under various environmental conditions.
Fig. 1 - Maps of the study sites in North Rhine-Westphalia, Germany. Panel 1 (upper right) shows the calibration test site with three different surfaces: asphalt (A), gravel (G), and skid trail (ST). Panel 2 (lower right) displays the field experiment site with three skids (S1, S2, S4) where the forwarder moved during logging operations, L-loaded (yellow points), and U-unloaded (blue points) twice. The dashed lines and triangles represent the transects and points where rut depth and soil moisture content were measured.
Forest machinery setup and CAN-bus
In both research activities, the forwarder was equipped with the specific off-road Alliance Forestry 344 Elit tire, size 710/45-26.5, with a tire air pressure of 2.5 bar. Since the field test campaign was conducted in wintertime, the machine was set up with tracks. The front bogie was fitted with Olofsfors ECOTM tracks, while the rear had Olofsfors BalticTM tracks (Fig. 2).
Fig. 2 - Forwarder bogie track configurations employed during the field test, showcasing differing setups for both front (a) and rear (b) bogies.
Before the study, the weight of the operating forwarder and tracks were measured separately on an industrial scale (Tab. 1) to ensure homogeneity in developing the μr model; for data recording, the CANedge2 module datalogger from CSS Electronics was utilized. This device is designed to interface with the Controller Area Network (CAN) bus of the forwarder (i.e., the primary communication network of the vehicle) and to acquire data from it. Moreover, this module is equipped with a GNSS (Global Navigation Satellite System) antenna, allowing it to determine the geographical position of the vehicle ([20], [7]). This module recorded data on various mobility parameters such as gross power, rotational engine (RPM), ground speed, and GNSS machine position with a frequency of 1 s.
Tab. 1 - Details of the forwarder weights and engine specifications used in the study.
Variable | Value | Units |
---|---|---|
John Deere PowerTech™ Plus 6068 turbocharged | 156 (209) | kW (hp) |
Mass Forwarder1210G | 21.940 | kg |
Mass ECO-Tracks | 1.240 | kg |
Mass Baltic-Tracks | 1.840 | kg |
Mass Forwarder1210G + Tracks | 25.020 | kg |
Site and load characterization
The study areas were located in a hilly region at an average elevation of 400 m a.s.l. Soil types at calibration and field-test sites (Fig. 1) were classified using Heinz Peter Schrey’s classification ([45]). Skid trail terrain from field test felt within the brown-earth soil type, characterized by silty loam and well-drained soils. Therefore, we assumed homogenous soil characteristics among the different skid trails. Since the correlation between soil moisture and rutting is evident ([4], [44], [43], [51]), weather condition assessment is essential to relate μr to precipitation rate. Therefore, we gathered daily precipitation observations on both test areas (i.e., calibration and field, respectively), recorded by the weather station Arnsberg-Neheim of the German Weather Service - DWD (Deutscher Wetterdienst). The day before the calibration test, a precipitation of 6 mm was recorded. However, no evident signs of moisture were found in the soil. Therefore, since we wanted to apply the same methodology among the experiments, driving tests on ST were assumed to happen in dry conditions as on hard surfaces (i.e., A and G). The field-test area recorded an average daily precipitation rate of 4 mm during logging operations. In this case, we also measured soil moisture content (SMC), expressed as a percentage of volume (%vol) in the topsoil beneath the litter layer, using the gravimetric method ([35]). Therefore, five soil samples were collected at 20-meter intervals along each skid, providing information about soil wetness conditions. While the forwarder was always unloaded when performing the calibration test on hard surfaces, during the field test (i.e., logging operations), the load of each forwarder travel had to be determined for each skid (100 m) and cycle using timber volume measurements obtained from the John Deere online portal Timbermatic® ([5], [33]). The forest stand consisted mostly of beech trees (Fagus sylvatica L.) mixed with single trees of spruce (Picea abies [L.] H. Karst.) and European larch (Larix decidua Mill.). For this reason, an average wood density of 750 kg m-3 was used to calculate the weight of the loads ([36]). The conversion from average logged volume into weight was performed following the standard formula in eqn. 1:
Moreover, in the field test, the depth of wheel ruts was manually measured after each vehicle passage. Measurements were taken in five transects along each skid at twenty-meter intervals with a precision of 1 cm using a horizontal hurdle and a measuring rod. To ensure consistency through data collection, the location of rut measurements was marked on the ground using spray paint, allowing measurements from the same spot across consecutive vehicle passages ([44]).
Tests design and data acquisition
The calibration test took place in controlled conditions to ensure a proper dataset for distinct driving surfaces. Three different segments, 100 m in length each, were traveled by the empty forwarder without tracks: (i) an asphalt road (A), (ii) a forest gravel road (G), and (iii) a compacted skid trail (ST). Both A and G road segments were traveled through four times repetitively at different constant speeds of 1, 5, 10, and 15 km h-1. Before each repetition, the forwarder was refilled with gasoline to ensure consistent weight throughout the tests. Data from the test on A served to calibrate the model employed in calculating the rolling resistance, as further described below. The field test setup was conducted in November 2022, observing the forwarder within a real-case logging operation. The traveling test was made of three different and parallel skid trails 100 m long each, namely skid trail 1 (S1), skid trail 2 (S2), and skid trail 4 (S4). Data collection occurred under typical logging conditions, with the forwarder operating uphill (U-unloaded) and downhill (L-loaded). This approach allowed us to eventually capture variations in μr across different skid trail slopes and loading forwarder conditions. The proposed method for μr calculus utilizes CAN-bus data through the so-called Society of Automotive Engineers (SAE) J1939 protocol. The acquired J1939 log files were thus decrypted according to the specific DBC file related to the J1939 protocol and then resampled to a specified time-frequency (e.g., 1 second).
μr calculation
CAN-bus data employed for the μr calculus include engine speed in revolutions per minute (RPM), navigation-based vehicle speed in kilometers per hour (km h-1), and GPS-derived machine positions for deriving slope angle estimation. The calculation of μr involved several steps. Given the average reference engine power of the forwarder (eqn. 1), we started by determining the actual engine power (Peng) by dividing the engine speed in revolutions per minute (derived from CAN-bus) by the forwarder reference one (eqn. 2):
In this context, RPMref represented the reference engine speed, which is the standard or baseline RPM value at which the engine produces the reference power of 156 kW (eqn. 3):
Here, RPMact refers to the actual engine speed recorded by the CAN-bus system. This is the real-time RPM value that varies depending on the current operating conditions of the engine.
By substituting these values, we calculated the actual engine power (Peng) based on the real-time engine speed data and the known reference values.
CAN-bus data recorded during the test drives on A facilitated the assessment of real-time power acting on the wheels since the controlled environment prevented further environmental variables. This power value was achieved by following the methodology proposed by Ala-Ilomäki et al. ([3]) field-based study and Ala-Ilomäki et al. ([2]), with some adjustments concerning the type of machine used in our test. A coefficient of mechanical efficiency (nmech), set at 0.74 by the test from Ala-Ilomäki et al. ([3]), was multiplied by the power engine, to derive the net power (Pnet) from mechanical friction losses (eqn. 4 - eqn. 6):
Successively, GPS data were used to determine the vehicle speed (v), needed to convert the expended power into motion force (eqn. 7):
The slope angle in the direction of travel was then needed to calculate the slope force (eqn. 8). This was achieved using a 1 m digital elevation model (DEM) obtained from a LiDAR flight performed in 2019 of the Bezirksregierung Köln region’s authority, with a vertical accuracy of ± 0.2 m, combined with the GPS path recorded during test drives. When driving downhill, slope force was added to the motive force, while it was subtracted from the latter when driving uphill (eqn. 9). Finally, the rolling resistance coefficient (μr) was calculated by dividing the rolling resistance force by the normal component of the vehicle weight (eqn. 10):
Data processing and analysis
The CANedge2 datalogger’s internal clock was synchronized with the current time before each data acquisition. CAN-bus output data from the calibration test was used to standardize the model to derive μr in the field test. The GPS-based vehicle speed, recorded in km h-1, was converted to m s-1. However, at a 1-second frequency, false speed readings, including 0 km h-1, had to be removed. This issue occurred mainly during tests at a constant speed of 1 km h-1 (i.e., on A, G, and ST), indicating GPS signal accuracy issues at lower speeds. Despite maintaining a constant 1 km h-1 speed, the CAN-bus often underestimated the speed, frequently showing 0 km h-1, while at higher speeds, underestimation caused by GPS inaccuracies was approximately 25%. Therefore, speeds equal to 0 km h-1 were filtered out in both calibration and field tests. Moreover, considering the significant mass of the forwarder (22 t), any accelerations and decelerations during testing were minimal and presumed to offset each other, as supported by previous experimental measurements ([50], [43]). The forwarder GPS positions were isolated for three specific skid trails (S1, S2, and S4), traversing each trail twice. We indicated these two traversals as C1 for the first pass and C2 for the second. During each cycle, the vehicle drove uphill (U-unloaded) and downhill (L-loaded) conditions. Importantly, in C2, the vehicle followed trails that were precisely the same as those of C1. This setup ensured consistency in soil conditions, allowing us to examine any potential differences in rolling resistance between the first and second passes. The analysis evaluated significant differences in the rolling resistance coefficient (μr) values between two driving surfaces, G and ST, in the calibration test. We used the Shapiro-Wilk test to check for departure from the normal distribution of G and ST data groups. Since the data did not meet the assumption of normality, we applied the non-parametric Mann-Whitney U test. After validating the methodology for calculating the rolling resistance coefficient in the controlled tests, we used it to the data of the field test. Statistical analysis followed the same approach proposed by Kabacoff ([22]). Descriptive statistics from the two tests reported averaged values for an overall understanding of the analyzed variables (i.e., slope, forwarder weight, SMC, rut depth, and μr). We examined the distribution and relationships between categorical variables (skid, cycle, forwarder driving direction, and loading status) using Chi-squared tests to establish their independence.
To assess the differences between first (C1) and second (C2) drive conditions across different operational states (U and L), we employed the Wilcoxon Signed-Rank test, a non-parametric statistical method suitable for paired data that does not assume a normal distribution. Specifically, we analyzed each skid dataset corresponding to different skids (S1, S2, S4) under both loaded (L) and unloaded (U) conditions. Non-parametric tests, such as Kruskal-Wallis test, were utilized to compare groups among the skids and the combination of cycle and forwarder status (U and L). This methodology was applied to evaluate the model’s sensitivity (and CAN-bus data) in capturing μr changes across the abovementioned scenarios. Moreover, a correlation analysis among machine and terrain variables was carried out by using the “cor.test” function to assess statistical significance. All data preparation, processing, and analysis were conducted using R ([39]).
Results
General μr observations
The study covered a comprehensive analysis of various driving surfaces and conditions. Calibration tests were conducted on all three surfaces (A, G, and ST) under similar conditions (i.e., an average slope of 5% and a forwarder weight of 22 t). Overall, results indicated varying μr values across surfaces (Tab. 2), with A exhibiting the lowest coefficient at 0.055 - fixed as a reference from the literature - and ST showing the highest at 0.44. Field tests extended the investigation to skid trails labeled S1, S2, and S4, characterized by steeper slopes and different SMC conditions. According to the different skid trails and forwarder driving directions, all recorded tests demonstrated considerably high average μr values, ranging from 0.2 to 0.26 in U forwarder condition (i.e., an average slope around 28% and a forwarder weight of 25 t) and from 0.51 to 0.6 in L forwarder condition (i.e., an average slope around 28% and a forwarder weight ranging from 31 t to 34 t - Tab. 2).
Tab. 2 - Summary of test scenarios and average parameters. (*): Reference μr value from Suvinen & Saarilahti ([50]).
Test scenario |
Driving surface |
Slope (%) |
Forwarder weight (t) |
SMC (%) |
Rut depth (cm) |
μr |
---|---|---|---|---|---|---|
Calibration | A | 5 | 22 | - | - | 0.06* |
G | 5 | 22 | - | - | 0.09 | |
ST | 8 | 22 | - | - | 0.44 | |
Field | S1 (U) | 27 | 25 | 29 | 15.5 | 0.20 |
S1 (L) | 27 | 34 | 29 | 15.6 | 0.51 | |
S2 (U) | 28 | 25 | 32 | 14.0 | 0.25 | |
S2 (L) | 28 | 33 | 32 | 13.2 | 0.60 | |
S4 (U) | 29 | 25 | 40 | 11.4 | 0.26 | |
S4 (L) | 29 | 31 | 40 | 12.4 | 0.56 |
Calibration test results
The results of the calibration test showed essential differences in the rolling resistance coefficients (μr) among the traveled surfaces, specifically gravel road (G) and skid trail (ST). Mean values and summary statistics further highlighted variations in the calculated μr among the test surfaces (Tab. 3). Although the dataset from the test on asphalt (A) was entirely used for building the model, the tests on G and compacted ST showed a significant increase in their average rolling resistance values (Fig. 3). The results of the first analysis of raw data showed a clear increment of μr coefficient as surface roughness increases.
Tab. 3 - Rolling resistance coefficient (µr) per surface (without tracks) during calibration test. (SD): standard deviation; (IQR): interquartile range.
Variable | Calibration | |
---|---|---|
Surface G | Surface ST | |
Mean µr | 0.09 | 0.44 |
SD µr | 0.03 | 0.14 |
Median µr | 0.09 | 0.36 |
IQR µr | 0.02 | 0.1 |
Fig. 3 - Boxplot showing the distribution of μr values for gravel road (G) and skid trail (ST). Boxes show the median (bold line) and first and third quartile (borders), while whiskers represent values below and above. Points identify outliers.
The Shapiro-Wilk normality test revealed a W value of 0.77 with p<0.001 for G, while for the ST group, the W value was 0.67 with p<0.001, indicating a non-normal distribution. The mean coefficient of rolling resistance μr in the ST group (0.44) was significantly higher than in group G (0.09). Since the data did not meet the normality assumption, we applied the Mann-Whitney U test. The low p-value (p<0.001) revealed a significant difference in the rolling resistance values between groups G and ST.
Field test results
The field test enabled us to calculate and determine μr’s main statistics for the three distinct skid trails, S1, S2, and S4, using CAN-bus data (Tab. 4). A general trend in μr distribution among skids, forwarder driving directions, and loading status was observed (Fig. 4).
Tab. 4 - Summary of statistical test results of the Wilcoxon Signed-Rank test and Kruskal-Wallis tests conducted to compare the μr values across different combinations of skid, cycle, and forwarder driving directions and load status.
Test | Group | Comparison | p-value |
---|---|---|---|
Wilcoxon Signed-Rank |
S1_U | C1 vs. C2 | ≤ 0.01 |
S1_L | C1 vs. C2 | ≤ 0.0001 | |
S2_U | C1 vs. C2 | ≤ 0.0001 | |
S2_L | C1 vs. C2 | ≤ 0.001 | |
S4_U | C1 vs. C2 | ≤ 0.0001 | |
S4_L | C1 vs. C2 | ≤ 0.0001 | |
Kruskal-Wallis | C1_U | S1 vs. S2 vs. S4 | > 0.05 |
C2_U | S1 vs. S2 vs. S4 | ≤ 0.0001 | |
C1_L | S1 vs. S2 vs. S4 | ≤ 0.0001 | |
C2_L | S1 vs. S2 vs. S4 | ≤ 0.0001 | |
C1 | S1 vs. S2 vs. S4 | ≤ 0.0001 | |
C2 | S1 vs. S2 vs. S4 | ≤ 0.0001 |
Fig. 4 - Boxplot of μr distributions across skid trails, cycles, and forwarder statuses. Blue indicates unloaded-uphill, yellow indicates loaded-downhill. Boxplot limits indicate the first and third quartiles, while whiskers represent values below and above. The median is depicted by a bold line, with outlier values shown as dots.
The Shapiro-Wilk test yielded a p-value below 0.001, indicating that μr values for skids and cycles do not follow a normal distribution. Observations were categorized by skids (S1, S2, S4), cycles (C1 and C2), and forwarder status (U and L). The distribution of μr values across all skids and cycles was homogeneous, hovering around the 50% mark for each category (e.g., S1_C1_U and S4_C2_L). We further assessed the independence between factors with a Chi-squared test, which revealed no significant association (p = 0.8864) between forwarder status (U, L) and skid nor between skid and cycle (p = 0.119). Moreover, no significant association was detected between forwarder loading status and cycle (p = 0.688).
In U forwarder conditions, the highest rolling resistance values uphill were recorded on S4 during the second cycle (C2) on the second day of the field campaign. Conversely, in L forwarder conditions, the highest rolling resistance values downhill were observed on S2 during the second cycle (C2) on the first day of the field campaign. In the field test, for both C1 and C2, the forwarder average estimated loads (eqn. 10) for S1, S2, and S4 were 9.2, 7.9, and 6.1 t cycle-1, respectively. In S1, despite harvesting the heaviest average load, no significantly higher values of μr were found in downhill conditions. On the other hand, in S4, despite harvesting a lighter average load, relatively higher μr values were observed when traveling loaded. However, the highest values of μr for L were found in S2 with a medium load of the forwarder. Since the data did not meet normality, a Kruskal-Wallis test was performed (Tab. 4).
When analyzing μr differences between the three different skids on the same cycle (C1) and forwarder loading status (U), e.g., S1_C1_U, S2_C1_U, and S4_C1_U, we found no statistically significant difference (p > 0.05) in the distribution of μr. On the other hand, when running the same analysis for C2, e.g., S1_C2_U, S2_C2_U, and S4_C2_U, the p-value was much lower than the standard significance level of 0.05, indicating a significant difference in the distribution of μr across the different skid groups in C2.
Additionally, the Kruskal-Wallis test for increasing μr values between unloaded (U) and loaded (L) forwarder conditions within C1 (i.e., S1_C1_U and S1_C1_L; S2_C1_U and S2_C1_L; S4_C1_U and S4_C1_L) revealed a significant difference among the observed groups (p < 0.05). Similar results were obtained performing the same test for C2 (i.e., S1_C2_U and S1_C2_L; S2_C2_U and S2_C2_L; S4_C2_U and S4_C2_L). Moreover, the Wilcoxon Signed-Rank Test was further performed to test for increasing μr value variations for each skid (S1, S2, S4) between the first and second consecutive drive (C1 and C2) under both loaded (L) and unloaded (U) conditions on the same skid. Results showed significant differences in μr values between two consecutive drives (C1 and C2) on the same skid with the U and L forwarder status (Tab. 4).
Relationship between μr and machine and terrain variables
Building on these findings, we further examined the relationship between μr and rut depth at each cycle on S1, S2, and S4 to understand how these variables interacted (Fig. 5). We began our analysis by examining the descriptive statistics of our dataset to provide an overview of the variables under consideration (Tab. 5).
Fig. 5 - Correlation between average μr values and average rut depths (cm). Bar heights represent μr values, while points indicate scaled rut depths. Blue/yellow colors represent unloaded/loaded forwarder data, and different point shapes relate to rut depth values for each skid trail’s four travels.
Tab. 5 - Descriptive statistics of μr, RPM, FW weight, and rut depth for every combination of skid, cycle, and FW loading status. (SD): Standard deviation.
Skid | Cycle_FW status |
Count | μr | RPM | FW weight | Rut depth | ||||
---|---|---|---|---|---|---|---|---|---|---|
Mean | SD | Mean | SD | Mean | SD | Mean | SD | |||
S1 | C1_U | 132 | 0.16 | 0.17 | 1642 | 59 | 25020 | 0.0 | 14.3 | 2.8 |
C1_L | 119 | 0.45 | 0.06 | 1320 | 47 | 34208 | 0.0 | 15.2 | 4.5 | |
C2_U | 140 | 0.23 | 0.21 | 1631 | 68 | 25020 | 0.0 | 16.6 | 3.7 | |
C2_L | 149 | 0.57 | 0.13 | 1358 | 71 | 34208 | 0.0 | 16.0 | 2.9 | |
S2 | C1_U | 134 | 0.16 | 0.11 | 1652 | 31 | 25020 | 0.0 | 13.5 | 2.1 |
C1_L | 134 | 0.56 | 0.14 | 1346 | 94 | 32933 | 0.0 | 12.5 | 1.8 | |
C2_U | 141 | 0.34 | 0.23 | 1606 | 80 | 25020 | 0.0 | 14.5 | 2.6 | |
C2_L | 142 | 0.64 | 0.19 | 1379 | 100 | 32933 | 0.0 | 13.9 | 2.6 | |
S4 | C1_U | 148 | 0.16 | 0.18 | 1558 | 47 | 25020 | 0.0 | 10.6 | 4.9 |
C1_L | 143 | 0.50 | 0.10 | 1319 | 45 | 31133 | 0.0 | 12.0 | 3.0 | |
C2_U | 136 | 0.36 | 0.27 | 1621 | 66 | 25020 | 0.0 | 12.1 | 3.9 | |
C2_L | 126 | 0.62 | 0.12 | 1381 | 95 | 31133 | 0.0 | 12.7 | 3.0 |
In 50% of the measurements, rut depth increased from C1 to C2, specifically in S1 and S4 (Fig. 5). Particularly, in S4, rut depth values showed a steady increase with each pass of the forwarder. Conversely, in S2, the opposite situation occurred. There was a relative trend of increasing rut depth with the forwarder’s passage under the same conditions (both in U and L), but not an absolute increase. When comparing the rut depth measurements between S2_C1_U - S2_C1_L and S2_C2_U - S2_C2_L, a decrease in rut depth is observed (Fig. 5). In S1, we found an intermediate situation compared to S2 and S4, with an increase in the measurements of rut depth between S1_C1_U and S1_C1_L, but a decrease in rut depth between S1_C2_U and S1_C2_L.
The correlation analysis for skid trails S1, S2, and S4 revealed significant interactions among RPM, forwarder (FW) weight, μr, and rut depth, as detailed in Tab. 6. In S1, a strong negative correlation between RPM and FW weight (-0.918) indicates that increased RPM is associated with decreased FW weight. Similarly, RPM and μr showed a moderate negative correlation (r = -0.600), suggesting a reduction in rolling resistance with higher RPMs. Conversely, FW weight and μr exhibited a strong positive correlation (r = 0.698), indicating that increases in forwarder weight are associated with increased rolling resistance. Additionally, a moderate negative correlation between FW weight and rut depth (r = -0.557) was observed in certain conditions.
Tab. 6 - Pairwise Pearson’s correlation coefficients between four main machine-terrain variables in each skid trail. (*): p ≤ 0.05; (**): p ≤ 0.01; (***): p ≤ 0.001; (****): p ≤ 0.0001.
Skid | Variable | FW weight | μr | Rut depth |
---|---|---|---|---|
S1 | RPM | -0.918**** | -0.600**** | -0.082 |
FW weight | - | 0.698**** | 0.093* | |
μr | - | - | 0.231**** | |
S2 | RPM | -0.846**** | -0.487**** | 0.471**** |
FW weight | - | 0.683**** | -0.557**** | |
μr | - | - | -0.185**** | |
S4 | RPM | -0.859**** | -0.359**** | -0.399**** |
FW weight | - | 0.611**** | 0.650**** | |
μr | - | - | 0.652**** |
In S2, the patterns were similar, with RPM and FW weight showing a robust negative correlation (r = -0.846), while RPM and μr showed a moderate negative correlation (r = -0.487). RPM and rut depth, however, exhibited a weak positive correlation (r = 0.471), indicating differing interactions compared to S1.
A strong negative correlation between RPM and FW weight (r = -0.859) was found in S4. RPM correlated negatively with both μr (r = -0.359) and rut depth (r = -0.399), while FW weight positively correlated with both μr (r = 0.611) and rut depth (r = 0.650). A strong positive correlation between μr and rut depth (r = 0.652) was consistently observed across all trails. Almost all relationships for each skid showed high significance (p ≤ 0.0001). However, a few exceptions occurred in S1 when correlating machine variables (FW weight and RPM) with rut depth.
Discussion
Evaluation of μr calculation in the calibration test
This study represents the first attempt to develop a model for μr assessment of a forwarder in a real working case scenario by using CAN-bus data to validate the methodology previously developed by Ala-Ilomäki et al. ([3]) to assess soil trafficability. Following past studies aimed at defining μr variable in a controlled experimental setup ([6], [50]), we extended the methodology to a real case logging scenario. Our results showed that the forwarder rolling resistance and its spatial variability can be assessed through a CAN bus data logger. This supports previous findings ([50], [2], [3], [43]) and emphasizes a strong correlation between surface roughness and rolling resistance, consistent with our initial hypothesis. Indeed, our findings support the first hypothesis that during the first cycle (C1) on uphill trails under unloaded (U) conditions, there would be no significant differences in the average μr values, indicating the stability of the rolling resistance coefficient. Differences in rolling resistance arose from the physical and mechanical characteristics of the tested surfaces, especially during the calibration test in June 2023. The analysis of data collected on different types of surfaces allowed for an accurate estimation of rolling resistance behavior under varying surface conditions, using the same forwarder setup and measuring methods. The highest μr values were recorded on the compacted skid trail (ST), while the lowest values were observed on the gravel road (G). This is because the skid trail had an irregular surface and a coarser texture, increasing rolling resistance more than G and A. High μr values can be further attributed to the fact that, given the physical characteristics, only the driving test at a constant speed of 1 km h-1 could be performed on ST. In this case, the sensitivity of the employed CAN-bus data logger may have resulted in a final μr value that is unexpectedly much higher on ST than on G. Moreover, this discrepancy was more pronounced when comparing the μr value of ST with the average values obtained on skid trails during the field test, mainly when the forwarder was using tracks and driving on steeper slopes. It is worth noting that each test was both site and machine-specific, making calibration tests fundamental.
Rolling resistance model performance
The field tests conducted with the tracked forwarder allowed us to calculate and determine the average values of μr using CAN-bus data for three distinct skidding tracks (S1, S2, and S4). These results provided a comprehensive overview of the distribution of μr values among the forwarder’s various skids, cycles, and load states. μr values recorded in the three observed skids and in the unloaded forwarder conditions (U) aligned well with previous tests where the forwarder was equipped with tracks ([6]). The comparison of μr values for the unloaded forwarder during the first uphill drive (C1) across S1, S2, and S4 did not reveal significant differences, indicating that the model built on CAN-bus data is reliable in calculating μr when similar forest skid (in terms of slope and SMC) are driven at first passage in same working condition (i.e., uphill driving direction, same forwarder weight). This result supports our first hypothesis, confirming that μr values remain stable during the first cycle on uphill trails under unloaded conditions. This also suggests that during the first uphill drive, for unloaded operational machines, μr values are not significantly affected by slope and soil characteristics but are mainly linked to machine operational dynamics. Such results support our hypothesis about the model’s ability to consistently return comparable μr values when environmental conditions are similar, in line with the findings by Ala-Ilomäki et al. ([3]). Significant differences emerged when comparing μr values between the first and second drive (C1 and C2) within the same skid, particularly for the unloaded forwarder conditions. These findings align with our second hypothesis that rolling resistance (μr) would show measurable changes between the first (C1) and second (C2) cycles, as well as between unloaded (U) and loaded (L) forwarder statuses, demonstrating the method’s sensitivity to variations in operational contexts. These differences are likely due to changing trail conditions or soil compaction effects, as reported by Labelle et al. ([23]). This finding was further supported by the Wilcoxon Signed-Rank test, which highlighted significant μr variation between consecutive drives within the same skid. Moreover, significant differences in μr were observed for the second drive (C2) across different skids, indicating a strong impact of terrain and forwarder interactions over repeated cycles ([37]). Additionally, the Kruskal-Wallis test emphasized the effect of loading on rolling resistance; μr values for the loaded forwarder in downhill conditions (L) did not vary much among the different tests. This indicates that factors other than load only, such as skid trail conditions and forwarder interaction with the terrain, influence μr values, as Hoffmann et al. ([19]) discussed. However, minor μr variations might also be related to slight differences in the timber volumes loaded in each skid. Overall, the implementation of the J1939 standard offered the advantage of a standardized procedure for communication among different ECUs, ensuring compatibility across various manufacturers and enabling broad scalability of the methodology across different forestry machine models and work environments ([8], [5]). Results demonstrated a complex interplay of factors affecting μr values, including load, skid, cycle, and terrain conditions. The observed consistency and balanced distribution patterns suggest that while the load is a significant factor, terrain and forwarder dynamics critically influence rolling resistance in forestry operations, generally increasing it since rougher terrain and heavier loads amplify resistance, while certain terrain conditions and optimized machinery configurations can mitigate this effect ([16]).
Interaction and constraints of μr with terrain and machine variables
The interaction between rolling resistance (μr) and machine variables revealed a critical understanding of the effects on rut depth across different cycles and skidding trails (S1, S2, S4). An important finding was the positive correlation between μr and rut depth, particularly evident in S4, highlighting the critical impact of terrain characteristics on rolling resistance ([19]). The positive relationship observed in S4 can be attributed to the fact that the activities in S4 took place the day after S1 and S2, when a light rain occurred overnight. In 50% of the measurements, rut depth increased from the first to the second cycle (C1 to C2), with S1 and S4 showing consistent increases, while S2 exhibited relative variations.
This trend suggests that soil moisture content and the presence of loose materials significantly influence rut depth ([37]). However, It is crucial to acknowledge the limitations associated with measuring rut depth. According to Starke et al. ([48]), the observed differences in rut depth may vary due to environmental conditions and measurement methods. This trend can be attributed to the movement of loose material from the topsoil, which fills part of the ruts ([3]). This observation highlights the importance of considering driving conditions when assessing terrain impact ([13]). The correlation matrix highlighted strong negative relationships between RPM and FW weight / μr and a moderate positive correlation between RPM and rut depth, contrasting with other negative RPM correlations ([43]). These relationships did not follow a common trend among S1, S2, and S4. This could be linked to machine load and soil characteristics such as SMC ([30]). Initially, we hypothesized a discrepancy between μr values in uphill and downhill drives due to a direct relationship with the engine RPM. We expected higher RPM when driving the forwarder loaded downhill due to the engine brake, which is automatically activated. However, no direct correlation was observed between the increase in RPM and the corresponding increase in μr, as the average aggregated engine RPM values were higher for the six unloaded uphill drives (1615 RPM) than for the six loaded downhill ones (1350 RPM). The increase of μr on downhill slopes is explained by the forwarder that stopped or significantly slowed down to activate the crane and load logs. Therefore, these increases were mainly related to the machine’s operations rather than specific ground conditions. These results suggest that the current form of the model does not fully capture the complexities of forwarder operations, particularly during loading interruptions. Future improvements of the model should consider these operational nuances to enhance the accuracy and reliability of μr measurements. As the forwarder drove uphill, increasing RPM was observed in U configuration, with a decreased FW weight, subsequently reducing rut depth, potentially minimizing terrain impact ([6]). This finding is critical for route planning, emphasizing the importance of managing RPM to optimize machine performance and reduce soil damage ([31]).
Conclusion
Effective forestry operations in rough and steep terrains require technologies adapting to varying environmental conditions. Our study presents a model that utilizes forwarder CAN-bus data and terrain variables to calculate the rolling resistance coefficient, providing a reliable indicator of machine performance, ground impact, and soil damage. Despite some limitations in explaining certain discrepancies, the model is valuable for evaluating off-road vehicles’ effects on terrain, offering significant insights into sustainable forestry practices. Obtaining μr coefficient from real working condition data serves as an operational criterion for trafficability prediction. The straightforward nature of the model ensures its easy implementation by forestry professionals, supporting better planning and the long-term sustainability of forest viability. For instance, integrating μr into operation planning optimizes machine routes and operations, reducing soil compaction and minimizing environmental impact during logging activities.
Acknowledgements and funding
This paper is part of the ETN Skill-For.Action project funded by the European Union’s HORIZON 2020 research and innovation program under the Marie Sklodowska Curie grant agreement No. 936355.
References
CrossRef | Gscholar
Gscholar
CrossRef | Gscholar
CrossRef | Gscholar
CrossRef | Gscholar
Gscholar
Authors’ Info
Authors’ Affiliation
Thilo Wagner
Forest Education Center (FBZ) - Center for Forest and Timber Industry, State Enterprise for Forestry and Timber NRW, Arnsberg (Germany)
Francesco Sforza 0000-0003-3286-0948
Francesco Marinello 0000-0002-3283-5665
Stefano Grigolato 0000-0002-2089-3892
Department of Land, Environment, Agriculture and Forestry, Università degli Studi di Padova, Padova (Italy)
Institute for Alpine Environment, Eurac Research, Bolzano (Italy)
Faculty of Agricultural, Environmental and Food Sciences, Free University of Bolzano/Bozen (Italy)
Department of Forest and Wood Science, Stellenbosch University, Stellenbosch, Matieland 7602 (South Africa)
School of Agricultural, Forest and Food Sciences (HAFL), Bern University of Applied Sciences, Zollikofen (Switzerland)
Corresponding author
Paper Info
Citation
Guerra F, Marzini S, Sforza F, Wagner T, Marinello F, Grigolato S (2024). Exploring the reliability of CAN-bus data in assessing forwarder rolling resistance under real working conditions. iForest 17: 360-369. - doi: 10.3832/ifor4687-017
Academic Editor
Rodolfo Picchio
Paper history
Received: Jul 09, 2024
Accepted: Sep 27, 2024
First online: Nov 13, 2024
Publication Date: Dec 31, 2024
Publication Time: 1.57 months
Copyright Information
© SISEF - The Italian Society of Silviculture and Forest Ecology 2024
Open Access
This article is distributed under the terms of the Creative Commons Attribution-Non Commercial 4.0 International (https://creativecommons.org/licenses/by-nc/4.0/), which permits unrestricted use, distribution, and reproduction in any medium, provided you give appropriate credit to the original author(s) and the source, provide a link to the Creative Commons license, and indicate if changes were made.
Web Metrics
Breakdown by View Type
Article Usage
Total Article Views: 1843
(from publication date up to now)
Breakdown by View Type
HTML Page Views: 609
Abstract Page Views: 524
PDF Downloads: 639
Citation/Reference Downloads: 0
XML Downloads: 71
Web Metrics
Days since publication: 164
Overall contacts: 1843
Avg. contacts per week: 78.66
Article Citations
Article citations are based on data periodically collected from the Clarivate Web of Science web site
(last update: Mar 2025)
(No citations were found up to date. Please come back later)
Publication Metrics
by Dimensions ©
Articles citing this article
List of the papers citing this article based on CrossRef Cited-by.
Related Contents
iForest Similar Articles
Research Articles
Deploying an early-stage Cyber-Physical System for the implementation of Forestry 4.0 in a New Zealand timber harvesting context
vol. 17, pp. 353-359 (online: 13 November 2024)
Research Articles
Fuel consumption comparison of two forwarders in lowland forests of pedunculate oak
vol. 12, pp. 125-131 (online: 11 February 2019)
Research Articles
Effect of forwarder multipassing on forest soil parameters changes
vol. 15, pp. 476-483 (online: 24 November 2022)
Research Articles
Comparison of alternative harvesting systems for selective thinning in a Mediterranean pine afforestation (Pinus halepensis Mill.) for bioenergy use
vol. 14, pp. 465-472 (online: 16 October 2021)
Research Articles
Temporal analysis of the traffic loads on forest road networks
vol. 6, pp. 255-261 (online: 13 June 2013)
Research Articles
Changes in moisture exclusion efficiency and crystallinity of thermally modified wood with aging
vol. 12, pp. 92-97 (online: 24 January 2019)
Research Articles
Estimating machine impact on strip roads via close-range photogrammetry and soil parameters: a case study in central Italy
vol. 11, pp. 148-154 (online: 07 February 2018)
Research Articles
Influence of slope on physical soil disturbance due to farm tractor forwarding in a Hyrcanian forest of northern Iran
vol. 7, pp. 342-348 (online: 17 April 2014)
Research Articles
Use of terrestrial laser scanning to evaluate the spatial distribution of soil disturbance by skidding operations
vol. 8, pp. 386-393 (online: 08 October 2014)
Research Articles
Density, extractives and decay resistance variabilities within branch wood from four agroforestry hardwood species
vol. 14, pp. 212-220 (online: 02 May 2021)
iForest Database Search
Search By Author
Search By Keyword
Google Scholar Search
Citing Articles
Search By Author
Search By Keywords
PubMed Search
Search By Author
Search By Keyword