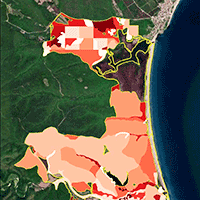
Modeling aboveground carbon in flooded forests using synthetic aperture radar data: a case study from a natural reserve in Turkish Thrace
Can Vatandaslar (1-2) , Ferhat Bolat (3), Saygin Abdikan (4), Pinar Pamukcu-Albers (5), Caner Satiral (6)
iForest - Biogeosciences and Forestry, Volume 17, Issue 5, Pages 277-285 (2024)
doi: https://doi.org/10.3832/ifor4527-017
Published: Sep 27, 2024 - Copyright © 2024 SISEF
Research Articles
Abstract
Flooded forests are rare and highly dynamic ecosystems, yet they can store a significant amount of carbon because of their ability to produce biomass rapidly. Estimation and mapping of the carbon that is stored in flooded forests are challenging tasks through the use of optical remote sensing because these ecosystems are often located in moist regions where clouds can interfere with data acquisition and image interpretation. This study models the aboveground carbon (AGC) stocks of a flooded forest in Turkish Thrace with synthetic aperture radar (SAR) data, which are less affected by weather and illumination conditions compared to optical imagery. Forest management plan data, including inventory records of 229 sample plots, a detailed forest cover map, and stand tables of the 2.119-ha Igneada Longoz Forest, were used to calculate AGC and to develop spatially explicit models based on ALOS/PALSAR-2 (Advanced Land Observing Satellite/Phased Array L-band Synthetic Aperture Radar) and Landsat-8 images. The results indicated that the horizontally transmitted and horizontally received (HH) and cross-polarization ratio (CPR) bands of ALOS/PALSAR were the most influential variables in the linear and nonlinear regression models. The models did not include any variables from either radar- or optical-based vegetation indices. While the estimation accuracies of the two models were similar (root mean square percentage error ≈ 26%), the linear model yielded negative estimations in several land cover classes (e.g., dune, forest opening, degraded forest). AGC stock was estimated and mapped using the nonlinear model in these cases. The density map revealed that Igneada Longoz Forest stored 279,258.9 t AGC, with a mean and standard deviation of 124 ± 115.4 t C ha-1. AGC density varied significantly depending on stand types and management units across the forest, and carbon hotspots accumulated in the northern and southern sites of the study area, primarily composed of ash and alder seed stands. The models and maps that this study developed are expected to help in the rapid and cost-effective assessment of AGC stored in flooded forest ecosystems across the temperate climate zone.
Keywords
SAR Mosaics, Landsat-8, Normalized Difference Vegetation Index (NDVI), Aboveground Biomass and Carbon Stocks, Carbon Density Maps, Bottomland Forests, National Parks, Igneada
Authors’ Info
Authors’ address
Faculty of Forestry, Artvin Coruh University, 08100 Artvin (Turkey)
Warnell School of Forestry and Natural Resources, University of Georgia, Athens 30602, GA (USA)
Faculty of Forestry, Çankiri Karatekin University, 18200 Çankiri (Turkey)
Department of Geomatics Engineering, Hacettepe University, Sihhiye, Ankara (Turkey)
Department of Geography, University of Bonn, 53115 Bonn (Germany)
Forestry Research and Application Center, Artvin Coruh University, 08100 Artvin (Turkey)
Corresponding author
Paper Info
Citation
Vatandaslar C, Bolat F, Abdikan S, Pamukcu-Albers P, Satiral C (2024). Modeling aboveground carbon in flooded forests using synthetic aperture radar data: a case study from a natural reserve in Turkish Thrace. iForest 17: 277-285. - doi: 10.3832/ifor4527-017
Academic Editor
Matteo Garbarino
Paper history
Received: Nov 24, 2023
Accepted: Jun 28, 2024
First online: Sep 27, 2024
Publication Date: Oct 31, 2024
Publication Time: 3.03 months
Copyright Information
© SISEF - The Italian Society of Silviculture and Forest Ecology 2024
Open Access
This article is distributed under the terms of the Creative Commons Attribution-Non Commercial 4.0 International (https://creativecommons.org/licenses/by-nc/4.0/), which permits unrestricted use, distribution, and reproduction in any medium, provided you give appropriate credit to the original author(s) and the source, provide a link to the Creative Commons license, and indicate if changes were made.
Web Metrics
Breakdown by View Type
Article Usage
Total Article Views: 8699
(from publication date up to now)
Breakdown by View Type
HTML Page Views: 7120
Abstract Page Views: 671
PDF Downloads: 840
Citation/Reference Downloads: 0
XML Downloads: 68
Web Metrics
Days since publication: 161
Overall contacts: 8699
Avg. contacts per week: 378.22
Citation Metrics
Article Citations
Article citations are based on data periodically collected from the Clarivate Web of Science web site
(last update: Mar 2025)
Total number of cites (since 2024): 1
Average cites per year: 0.50
Publication Metrics
by Dimensions ©
Articles citing this article
List of the papers citing this article based on CrossRef Cited-by.
References
Evaluating the accuracy of spectral indices from Sentinel-2 data for estimating forest biomass in urban areas of the tropical savanna. Remote Sensing Applications: Society and Environment 22 (7): 100484.
CrossRef | Gscholar
Correlation between NDVI and Sentinel-1 derived features for maize. In: Proceedings of the “IEEE International Geoscience and Remote Sensing Symposium”. Brussels (Belgium) 11-16 July 2021. The Institute of Electrical and Electronics Engineers, Inc., Brussels, Belgium, pp. 6773-6776.
CrossRef | Gscholar
Qualitative and quantitative evaluation of water resources that feed into the National Park Igneada longoz forests (Turkish Thrace) by using physico-chemical and biological analyses. Hidrobiológica 25 (2): 231-237.
Gscholar
Ecosystem-based multifunctional forest management plan of Igneada Longoz Forests National Park (2014-2033). Turkish General Directorate of Forestry, OGM Publishing, Ankara, Turkey, pp. 245. [In Turkish]
Gscholar
Using satellite estimates of aboveground biomass to assess carbon stocks in a mixed- management, semi-deciduous tropical forest in the Yucatan peninsula. Geocarto International 37 (25): 7659-7680.
CrossRef | Gscholar
Süleymaniye ormani sivri meyveli disbudaklari (Fraxinus oxycarpa Willd.) odununun bazi fiziksel ve mekanik özellikleri ve degerlendirme imkanlari hakkinda arastirmalar [Research on physical and mechanical features of Caucasian ash (Fraxinus oxycarpa Willd.) in the Süleymaniye forest]. Forestry Research Institute, Technical Bulletin Series no. 47, Cihan Publishing, Ankara, Turkey, pp. 187. [in Turkish with German abstract]
Gscholar
The flooded forest: guidance for policy makers and river managers in Europe on the restoration of floodplain forests. University of Cambridge Press, Cambridge, UK, pp. 96.
Gscholar
Good practice guidance for land use, land-use change and forestry. IPCC/OECD/IEA/IGES, The Institute for Global Environmental Strategies, Hayama, Japan, pp. 590.
Gscholar
IPCC Guidelines for national greenhouse gas inventories, prepared by the National Greenhouse Gas Inventories Programme. The Institute for Global Environmental Strategies, Hayama, Japan, pp. 747.
Gscholar
Applied linear statistical models. McGraw-Hill/Irwin Professional Publishing, New York, USA, pp. 1408.
Gscholar
Total carbon stocks and carbon accumulation in living tree biomass in forest ecosystems of Turkey. Turkish Journal of Agriculture and Forestry 35: 265-279.
Gscholar
Coefficients that can be used to calculate biomass and carbon amounts from increment and growing stock in Turkey. In: Proceedings of the “International Symposium for the 50th Anniversary of the Forestry Sector Planning in Turkey”. Ankara (Turkey) 26-28 November 2013. CTA Press, Ankara, Turkey, pp. 240-251. [in Turkish with English abstract]
Gscholar
Integrating Sentinel-1A SAR data and GIS to estimate aboveground biomass and carbon accumulation for tropical forest types in Thuan Chau district, Vietnam. Remote Sensing Applications: Society and Environment 14: 148-157.
CrossRef | Gscholar