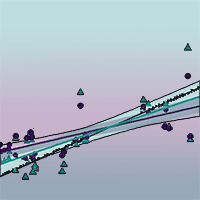
Evaluation of operating cost management models for selection cutting in Scandinavian continuous cover forestry
iForest - Biogeosciences and Forestry, Volume 16, Issue 4, Pages 218-225 (2023)
doi: https://doi.org/10.3832/ifor4204-016
Published: Aug 12, 2023 - Copyright © 2023 SISEF
Research Articles
Abstract
The importance of continuous cover forestry (CCF) is increasing, yet there is lack of data and understanding about many aspects of this management, including the operational costs. Our objectives were to retrieve available harvesting cost models from published studies on selection cutting in Norway-spruce-dominated stands in Scandinavian countries and to evaluate them against real case studies. First, we retrieved three recently published harvesting cost models which provided explicit cost functions. Models 1 and 2, based on rotation forestry (RF) data and adapted for CCF, had separate sub-models for cutting and hauling costs. Model 3 was based on CCF data and produced total harvesting costs, including the cutting and hauling costs combined. Second, we measured cutting costs for 29 harvesting operations on stands with different stages of CCF structure. We then compared the observations with the simulations of Models 1 and 2 cutting cost sub-models for those cases. Third, we expanded the dataset, including a further 34 harvesting operations in stands with more advanced CCF structures (without measured costs). We then simulated the total harvesting costs for all three models in this dataset to investigate their general behaviour. On average, Models 1 and 2 cutting cost sub-models had relatively good and consistent predictions compared with the observed values. However, they differed in total costs due to different estimates for the hauling cost sub-models. Model 3 had predictions comparable to Models 1 and 2 in the more advanced stages of CCF, but much higher in the less advanced. This study provides important data regarding cutting costs in CCF and demonstrates the feasibility of using existing harvesting cost models.
Keywords
Introduction
Continuous cover forestry (CCF) is a form of forest management that avoids the use of clearcutting and thus maintains a continuity of woodland conditions across the site ([39], [26], [27]), unlike the conventional even-aged rotation forestry (RF) management method, which is based on large-scale clearcutting. A common method for CCF management in Norway spruce (Picea abies [L.] Karst.) dominated forests is selection cutting, which results in an unevenly structured stand where trees of various sizes and ages grow together ([22]). Selection cutting can be applied with different methods ([40], [25]), although there are some general common principles ([49], [29], [48]): some of the largest trees, whose relative growth may be decreasing, are removed both to create growth space for the remaining trees and to obtain valuable saw logs; the densest tree groups may be thinned to provide sufficient growth space for the remaining trees; defective and diseased trees may be removed regardless of their size; above all, sufficient undergrowth reserve in the forest is needed to replace felled trees, so special attention is paid to promoting the emergence of new seedlings.
In the 2000s, loss of forest nature diversity and the climate crisis raised the need to improve the ecological sustainability and carbon sequestration capacity of forests ([38]). At the same time, in addition to forest professionals, diverse groups like politicians, news editors, tourism entrepreneurs, and the public are increasing their participation in the public CCF discussion. Their interaction has enlarged the significance of CCF because each supporter group has different arguments to push for increasing the area under this management, including economic profitability, carbon stock, ecosystem services, and social and health impacts. A wide range of studies has shown that CCF increases various ecosystem services such as biodiversity, cultural heritage, and recreational use ([15], [10], [23]). According to Eyvindson et al. ([9]), to maximise multifunctionality at regional level in Fennoscandia while maintaining high timber extraction rates, the area of CCF should increase and RF should decrease (without being completely banned).
However, various authors ([18], [49], [20]) have concluded that knowledge on stand development, harvesting methods, and the economic benefits of CCF in the Nordic countries is too limited to reach definitive conclusions. Above all, there is a need for more studies and data to test CCF in actual working environments.
The forest work environment, defined by characteristics such as stand structure, terrain topography, and soil bearing capacity, affects harvesting activities ([35], [48]). The size and spatial order of trees in RF stands is uniform, and they may have regular strip road networks. They thus offer the optimal working environment for conventional harvesters and forwarders. On the other hand, the importance of the operator’s skills is emphasised when harvesting CCF stands with selection criteria because the risk of damage to regenerating trees increases as large trees are felled ([36]). Well-implemented selection cutting and the timing of future harvestings determine the success of CCF, which is based on the viability of the regeneration stock to provide enough good-quality standing trees in the future ([46]). The most important growth capital for CCF is medium-sized trees (i.e., 5-15 meters in height). Previous studies show that the damage rate in such trees is 10%-20%, and a significant proportion of the damage is in their canopies ([12], [48], [33]). On the other hand, the survival of seedlings (i.e., 0.5-3.0 meters in height) strongly affects the long-term development of the uneven structure. In published studies, the proportion of seedling damage ranged 20%-75% ([13], [50]). The risk of seedling damage depends on the number of removed trees, their height, and their distance from the strip road ([50]). Especially in frost conditions, seedlings and small trees break more easily ([8]). To minimise the damage, the operator’s work planning and the working technique itself (i.e., logging timing, the location and width of strip roads, and the felling direction of trees) plays a key role ([34]).
Laamanen ([21]) studied the structure before the harvesting and removal of eight CCF stands in Finland. The volume before harvesting was in the range of 157-285 m3 ha-1, while the total removal and average volume of harvested trees were 110-231 m3 ha-1 and 251-410 dm3 respectively. Similarly, Andreassen & Oyen ([4]) found that the average size of removed trees in CCF was significantly larger than in clearcutting. Many studies have shown that processing trees of the same size is slower in CCF than in RF due to the higher care needed to avoid damage to the remaining standing trees. However, the overall productivity per total volume removed of CCF was found to be only slightly lower than in RF when in the former stands, the largest trees in the forest were mainly removed ([11], [24], [4], [14]). In the case of the first transformation thinning of an RF stand to CCF, the stand structure before harvesting, the number of the removals, and the thinning method may still ensure good productivity. In subsequent interventions during the transformation process, Jonsson ([16]) found productivity to be 30% slower than in the first. This was due to the trees being thinned again from the finished strip roads of the first selection cutting, meaning the productivity decreased because there were fewer removals. In young dense stands, boom corridor thinning (BCT) has proved to be a cost-effective harvester’s working method, creating uneven structured post-stands that promote the development of CCF forests ([1], [32], [5]). In BCT, trees are harvested continuously in linear corridors within the harvester’s boom reach instead of using traditional selective thinning from below, where the smallest trees are primarily removed one by one.
Net Present Value (NPV) analysis, a system to measure the present value of the future cash flow the forest will produce, is the most common way to compare the profitability of different forest management methods. The method involves the calculation of long-term income and expenditure, discounting them from the present, and calculating the difference. If positive, the given silviculture system is profitable ([51], [54]). When using this method, the harvesting costs of different methods and work environments can be compared. According to Surakka & Sirén ([49]), in mature spruce stands the harvesting cost of selection harvesting in CCF was the same as in RF. In contrast, for Jonsson ([16]) the cost of selection cutting and forwarding was 28% higher in CCF than in RF over one rotation period.
Since most of the articles addressing the profitability of CCF apply roadside prices with harvesting costs subtracted, it would be interesting to compare the underlying harvesting cost models. Our research questions are: (i) What harvesting cost models are available for CCF in the Nordic countries? (ii) Do they correctly predict the costs under a new independent validation? (iii) Do they produce similar outcomes when an identical thinning profile is applied?
Materials and methods
Model selection
We searched the Google Scholar® online repository for papers on profitability in CCF showing total harvesting cost models, including both cutting and hauling. We considered the following criteria: (i) an identical distribution of harvested trees can be fed into all models; (ii) the harvesting cost models result in total costs (expressed in euros); and (iii) recently published (in the last 15 years). Among the potential candidates ([49], [52], [54], [41], [53], [44], [45], [46], [55], [47], [17], [7], [37]), the following models fulfilled our selection criteria.
Model 1 ([53]) includes a component for the cutting and one for the hauling costs (respectively eqn. 1 and eqn. 2) so that the total cost is the sum of the two components:
where C1 is equal to 1.15, n is the total number of trees removed (n ha-1), vt is the volume of tree t (m3), Hsaw is the total volume of sawlogs and Hpulp is the total volume of pulpwood removed (both in m3 ha-1). This model was initially based on the empirical cost functions for RF stands from Kuitto et al. ([19]), which presented operational time studies in several final fellings and thinnings carried out in Finland in 1991-1992. However, the model was adjusted to correspond to CCF management conditions by applying a specific procedure: hauling cost components from the thinning cost function and the logging cost component from the clearcut cost function were multiplied by a factor equal to 1.15. This coefficient is in line with the study of Surakka & Sirén ([49]), which tackles selection harvesting. During model fitting, the logging cost and hauling cost rates were considered to be 82.5 € and 59.5 € per hour.
Model 2 ([46]) also includes a separate component for the cutting and hauling costs (respectively eqn. 3 and eqn. 4):
where C1 is equal to 1.15, n is the total number of trees removed (n ha-1), and vt the volume of tree t (m3). Similarly to Model 1, this model was initially based on the empirical cost functions for RF stands from Nurminen et al. ([30]), which presented operational time studies in several final fellings and thinnings carried out in Finland in 2004. These models were then similarly adjusted for CCF as in Model 1. During model fitting, the logging cost hauling cost rates were considered equal to 126 € and 60 € per hour.
Model 3 was fitted by Juutinen et al. ([17]) based on harvesting cost data retrieved from the CCF study of Surakka & Sirén ([49]), simultaneously estimating the combination of cutting and forest haulage (eqn. 5). The authors build the linear model directly from the data published by Surakka & Sirén ([49]).
where x is the total removals (m3 ha-1), and z the average stem volume of harvested trees (dm3). During model fitting, the logging cost and hauling cost rates were considered to be 70 € and 50 € per hour. Model 3 did not include fixed costs, while models 1 and 2 expected to include them as an additional term to reflect, e.g., administrative and transport costs independent of cutting removal. For this study, they were unnecessary and therefore these costs were excluded.
Harvesting case studies
CCF-BASIS dataset
This dataset consists of 5 spruce-dominated stands, each with 4-7 rectangular plots (size either 800 or 1000 m2) for a total of 29 plots (Fig. 1). The stands were all dominated by mature spruce, subjected in the past to varied silvicultural regimes both across and within stands (either thinning from above, low thinning, or no evidence of recent thinning). Two stands (for a total of 12 plots) were on a site characterised by Oxalis-Myrtillus vegetation, one stand (7 plots) on Myrtillus type, and two stands (for a total of 10 plots) on a herb-rich drained peatland type ([6]).
Field trials were carried out during 2021-2022; two stands during winter harvesting in 2021; two stands during summer harvesting in 2021 and 2022; and one stand during winter harvesting in 2022. Three different single-grip harvester brands (John Deere 1170G, Ponsse Beaver, Komatsu 901 901XC) and four machine operators were used in the test cuttings. However, all the harvesters were medium-size types and intended for both thinnings and clearcuttings. Most operators had several years’ professional experience of CCF selection cutting, while one was extensively trained before the experiment. The strip road was placed and marked out approximately in the middle of the shorter sides of the plots (20 m), so that the harvester’s crane reach could reach across the whole plot. Before the trials, the characteristics of the plots’ growing stock were measured. After thinning, all remaining trees were mapped and measured by species and diameter at breast height (dbh), and the damage of trees and soil due to logging was measured. In the plots, after selection cutting, the basal area of remaining trees was in accordance with the forest management recommendations applied in Finland ([3]).
Four plots were already quite irregular in their structures and were selectively harvested similarly to an ongoing CCF (henceforth “advanced CCF”), thus indicating favourable stand characteristics for CCF management. The majority (14 plots) had more irregular structures than the average RF stand in Finland but still needed some level of transformation (“medium CCF”) to be fully managed according to CCF principles. The rest (11 plots) had a quite regular structure and underwent their first selective thinning for moving towards an irregular structure (“beginning CCF”). In each stand, there may have been plots of different structures, and average values for each category are presented in Tab. 1. The average harvesting profiles, i.e., the distribution per hectare of the dbh of harvested trees against all trees, for each category (advanced, ongoing, and transformation CCF) are shown in Fig. 2.
Tab. 1 - Summary statistics for the plots and harvesting operations in each category. (BA): basal area. Values are mean ± standard deviation. Productivity is calculated using an increased cutting time of 15 minutes per hour.
Statistics | CCF-BASIS (Advanved) |
CCF-BASIS (Medium) |
CCF-BASIS (Beginning) |
ERIKA |
---|---|---|---|---|
No. of harvesting operations | 4 | 14 | 11 | 34 |
Stand BA pre-harvest (m2 ha-1) | 24.1 ± 2.0 | 23.9 ± 10.9 | 33.0 ± 4.2 | 21.5 ± 5.0 |
Stand BA removed (m2 ha-1) | 8.9 ± 2.9 | 17.8 ± 4.0 | 18.7 ± 7.2 | 8.5 ± 3.6 |
Volume removed (m3 ha-1) | 75.0 ± 29.4 | 179.4 ± 63.5 | 207.0 ± 80.6 | 91.9 ± 45.4 |
Average volume of removed trees (m3) | 0.31 ± 0.14 | 0.50 ± 0.30 | 0.63 ± 0.21 | 0.44 ± 0.32 |
Productivity, with delays (m3 h-1) |
20.9 ± 5.1 | 45.5 ± 10.8 | 32.8 ± 11.5 | - |
Cutting costs (€ m-3) | 5.4 ± 1.6 | 3.9 ± 1.4 | 2.1 ± 0.3 | - |
Fig. 2 - Size distribution of total standing trees pre-harvesting (grey) and of the felled trees (red), averaged for each category, per hectare. Both CCF-BASIS (beginning, medium, and advanced) and ERIKA datasets.
The time and motion study ([28], [29]) was conducted by video recording the harvesters’ work performance in each plot, where one action camera was mounted inside the harvester’s cabin. The operation time was recorded using the continuous timing method ([28], [31]). A time and motion study tool developed using Microsoft Visual Basic® language in Excel® software ([29]) was used to record and analyse the time consumption of the video material of the plots. We thus measured the total working time for the cutting (E0h, or Effective Work Time per Hour) and then included estimated work-related delay events of 15 minutes per hour (E15h, increasing by 25% E0h). Using a price of 85 € per E15h, we calculated the costs for m3 removed in each plot. Details on removals and cutting time and costs for each category are shown in Tab. 1.
ERIKA dataset
This dataset consists of 20 spruce-dominated stands with a total area of 1-2 ha each, all in a more advanced CCF stage than CCF-BASIS (i.e., already in or near a steady state - Fig. 1). There was one permanent sample plot (size: 1600 m2) in each stand. The stands belonged to a long-term CCF permanent sample plot experiment involving single-tree selection starting in the 1980s. Most of the stands (16) belonged to the Myrtillus vegetation type; the rest (4) belonged to the mesic Oxalis-Myrtillus type. For more information, please refer to Valkonen et al. ([56]).
Fourteen plots were subject to a selection cutting during the winter of 1996/1997 and then all of them during the winter of 2011/2012, for a total of 34 measurements. In both 1996 and 2011, the harvesting was carried out to enhance uneven-aged structures in the stands and to reset their basal areas to the original values of the 1980s. The emphasis of the removal were the larger diameter classes (dbh > 30 cm). However, some larger trees had to be retained in many stands to achieve the target basal area. On the other hand, a small number of mid-sized trees (20 < dbh ≤ 30 cm) was removed where there was a major surplus. Five stands were not harvested due to their operationally unjustifiable harvestable volumes. In 2011, the cutting was similar in type and execution, but removed volumes were larger, and all stands had an operationally justifiable harvestable volume due to the longer period of growth between harvests (15 years).
Advance tree and strip road marking, manual felling, debranching and cut-to-length culling with chainsaw, and forwarder hauling were employed during the harvesting. The trees to fell were selected and very well marked in advance, and other trees removed or lost in harvesting were considered harvest loss. The stand characteristics, including the details of removals, are shown in Tab. 1, while the average harvesting profile is shown in Fig. 2. Harvesting costs were unavailable for this dataset.
Models’ evaluation
All the analyses were carried out in R ([43]). First, we applied Models 1 and 2 to each plot-specific harvesting profile of the CCF-BASIS dataset and estimated the cutting costs alone, which were compared with the observed ones. We carried out visual analyses of the simulation results (included residuals) and calculated the errors.
Second, we applied all three complete models to the combined CCF-BASIS and ERIKA datasets and estimated the total costs (cutting and hauling) to further analyse their behaviour against an extended dataset, albeit without observed values. In this case, ERIKA plots were assigned to the advanced CCF stage of the CCF-BASIS dataset. We only carried out visual analyses of the simulation results.
Results
Cutting costs
The simulations of both models fitted well with the observed costs, resulting in a root mean square error (RMSE) of 1.05 € and 1.07 € for models 1 and 2 respectively (Fig. 3a) when all categories were considered. Regarding the different category of stand structures, the degree of accuracy (i.e., RMSE) was more divergent between models, and the lowest errors were in the advanced CCF structures (Fig. 3b to Fig. 6d). The results for Models 1 and 2 were not statistically different (p-value > 0.10) for Welch two sample t-tests carried out for all data and within each category (see also the summary in Tab. 2). The residuals were homogeneously distributed according to the simulated values (Fig. 4). Cutting costs tended to decrease with increased cutting removals (Fig. 5).
Tab. 2 - Results of the models’ independent validation for each category, both for the cutting costs only and the total cost analysis. Values are mean and standard deviation, in € m-3. For the total costs, “Advanced” include the respective CCF-BASIS plots plus the ERIKA dataset.
Category | Model | Beginning | Medium | Advanced |
---|---|---|---|---|
Cutting costs only | Model 1 | 3.2 ± 0.3 | 3.9 ± 1.1 | 5.0 ± 1.0 |
Model 2 | 2.8 ± 0.5 | 3.7 ± 1.8 | 5.3 ± 1.4 | |
Total costs | Model 1 | 6.4 ± 0.4 | 7.2 ± 1.3 | 9.6 ± 2.9 |
Model 2 | 4.9 ± 0.6 | 5.8 ± 1.8 | 7.6 ± 3.4 | |
Model 3 | 10.7 ± 2.6 | 11.1 ± 1.8 | 9.5 ± 2.3 |
Total costs
The estimate for the total harvesting costs (cutting and hauling) of the three models had different trends against total removals (Fig. 6a), which are summarised in Tab. 2. Models 1 and 2 showed increasing costs for lower removals (usually corresponding to lower average tree volume felled as well - data not shown). Two extreme cases with costs of 40-60 € m-3 were removed from the ERIKA dataset, where total removals and average tree volume were especially low (for the former, 17.5 and 26.04 m3 and for the latter, 176 and 274 dm3). On the other hand, Model 3 showed slightly increasing costs for increasing total removals, as cutting and hauling were modelled simultaneously, the underlying reason being the capacity limit of the workload in haulage with increasing removal.
On average, for all data, Model 2 estimates were significantly lower than for Model 1, whose estimates in turn was significantly lower than for Model 3 (p-value < 0.01 after Welch two sample t-tests - Fig. 6b to Fig. 6d). This trend also happened within the beginning and medium categories but not in the advanced CCF stages, where Model 3 and Model 1 were not significantly different (p-value > 0.10, Welch two sample t-tests).
Discussion
In this study, we assessed the harvesting costs for CCF in boreal settings, which is crucial for determining the feasibility of this silvicultural approach in the current debate. Given the increasing interest in CCF, it is important to provide stakeholders with the best tools for decision making. Our first objective was to retrieve published harvesting costs models literature that could be applied in simulation studies. We found only three models, all with limitations. Models 1 and 2 were fitted on RF data and adjusted to CCF with some broad assumptions. They were both also used in subsequent studies about the economic assessment and optimisation of CCF management ([54], [37]). Model 3 was fitted explicitly on CCF data but with a simple method and only a few harvesting operations ([49]). It has been used only in the study of Juutinen et al. ([17]). Given the limitation of all the above models, there is an urgent need of better suited models based on a larger number of CCF real case studies.
Our second objective was to evaluate the predictions of the retrieved models during an independent validation. This was limited to the cutting cost part of Models 1 and 2, using only the CCF-BASIS dataset (29 harvesting operations). The simulated values of the two models were not significantly different, and both well fitted the observed values (the root mean square error was around 1 € m-3 in both cases). The two models reached very similar results, even starting from very different hourly cost assumptions for cutting: 86 € for Model 1 (published in 2010) and 120 € for Model 2 (published in 2017), respectively similar to and much higher than the 85 € paid in the observed operations. Estimates were more correct for advanced continuous cover forestry stages than for the beginning and medium stages, although there were only 4 replicates for the former and 25 for the latter. Cutting costs for the advanced stage included a 15% increase compared to rotation forestry, as suggested by the model developers. We found this correction unnecessary for the beginning and medium stages, where the operating conditions and removals were closer to the rotation forestry stands used for the models’ calibration.
Regarding the third objective, we analysed the total cost prediction of all three models, verifying their predictions using identical thinning profiles. We expanded the dataset by including 34 more harvesting operations from the ERIKA dataset, a long-term experiment involving selectively harvested stands, for a total of 63 operations. Models 1 and 2 showed significant differences in the total costs which were not observed after the analysis of the cutting costs only. Model 1 estimates were on average 1.77 € m-3 higher than Model 2. On average, Model 3 predicted values were higher than those of both Models 1 and 2, with a difference of 2.5 and 3.2 € m-3, respectively, considering all the data. The differences decreased moving towards the more advanced continuous cover forestry stage, where the total costs were not significantly different for Models 1 and 3 (9.60 and 9.35 € m-3, respectively) but still significantly lower for Model 2 (7.62 € m-3). The data used for the calibration of Model 3, which are real case studies of selection harvesting in CCF operations, showed an average of 9.44 € m-3. We found only one comparison in the literature ([16]), which presented total costs between 10 and 15 € m-3 for values of the average volume of felled trees of 0.3-0.5 m3 (comparable to our advanced CCF stage), similar to Model 3 but higher than values obtained using both Models 1 and 2. It must be noted that for total costs, the hourly cost assumption for cutting was lowest for Model 3 (70 €), compared to the previously mentioned 86 € and 120 € for Models 1 and 2, respectively. On the other hand, hauling cost assumptions were less different among the three models: 60 € for Models 1 and 2, and 50 € for Model 3. Furthermore, it should be stressed that Model 3 was the only model based on actual CCF logging conditions, while Models 1 and 2 were initially modelled according to logging conditions in rotation forestry.
Since there were differences between the models regarding total costs, this may have a consequence for stand-level optimisation, as even small changes in endogenous variable values could have a profound effect on optimal solutions ([2], [42]).
Our analyses did not include fixed costs. Models 1 and 2 used values of 300 € and 100-500 € respectively, which could remarkably affect the low-intensity operations described in this study. Given an average of 75-90 m3 total removals per ha observed in the advanced continuous cover forestry stage, these fixed cost values could have an impact on the harvested cubic metre, ranging from 1-7 €. It is evident that an adequate estimate of such costs is needed.
Conclusions
Regarding the cutting costs, Models 1 and 2 did not significantly differ in their simulations, and they reliably estimated the observed data in all stages, although we did not use the suggested 15% increase in cutting costs for the first phases of the transformation to continuous cover forestry (here, the beginning and medium stages).
Regarding the total harvesting costs, we found different behaviours for the various stages. For the beginning and medium stages, Models 1 and 2 seemed to provide more adequate harvesting costs than Model 3, which was not calibrated using such conditions. For the initial stages of CCF, we suggest the former models are used, although Model 2 provides consistently lower costs that Model 1. In the more advanced CCF stages, Models 1 and 3 gave similar results, considerably higher than Model 2. The few verification data available seem to suggest the use of Model 1 or Model 3. However, it is necessary to validate these results against a larger number of cases of advanced continuous cover forestry operations or to prepare new models.
Acknowledgements
This study was supported by Luke’s project CCFBASIS “Technological and conceptual basis for Continuous Cover Forestry” (grant no. 41007-00182900).
References
Gscholar
Gscholar
Gscholar
Gscholar
Gscholar
Gscholar
Gscholar
Gscholar
Gscholar
Gscholar
Gscholar
Gscholar
Gscholar
Gscholar
Gscholar
Gscholar
Gscholar
Authors’ Info
Authors’ Affiliation
Eero Holmström
Sauli Valkonen 0000-0002-2879-4821
Natural Resources Institute Finland (LUKE), Helsinki (Finland)
Yrjö Nuutinen 0000-0003-3360-4444
Natural Resources Institute Finland (LUKE), Joensuu (Finland)
Corresponding author
Paper Info
Citation
Bianchi S, Ahtikoski A, Muhonen T, Holmström E, Valkonen S, Nuutinen Y (2023). Evaluation of operating cost management models for selection cutting in Scandinavian continuous cover forestry. iForest 16: 218-225. - doi: 10.3832/ifor4204-016
Academic Editor
Rodolfo Picchio
Paper history
Received: Aug 11, 2022
Accepted: Apr 09, 2023
First online: Aug 12, 2023
Publication Date: Aug 31, 2023
Publication Time: 4.17 months
Copyright Information
© SISEF - The Italian Society of Silviculture and Forest Ecology 2023
Open Access
This article is distributed under the terms of the Creative Commons Attribution-Non Commercial 4.0 International (https://creativecommons.org/licenses/by-nc/4.0/), which permits unrestricted use, distribution, and reproduction in any medium, provided you give appropriate credit to the original author(s) and the source, provide a link to the Creative Commons license, and indicate if changes were made.
Web Metrics
Breakdown by View Type
Article Usage
Total Article Views: 7988
(from publication date up to now)
Breakdown by View Type
HTML Page Views: 6802
Abstract Page Views: 567
PDF Downloads: 550
Citation/Reference Downloads: 1
XML Downloads: 68
Web Metrics
Days since publication: 265
Overall contacts: 7988
Avg. contacts per week: 211.00
Article Citations
Article citations are based on data periodically collected from the Clarivate Web of Science web site
(last update: Feb 2023)
(No citations were found up to date. Please come back later)
Publication Metrics
by Dimensions ©
Articles citing this article
List of the papers citing this article based on CrossRef Cited-by.
Related Contents
iForest Similar Articles
Research Articles
Comparison of alternative harvesting systems for selective thinning in a Mediterranean pine afforestation (Pinus halepensis Mill.) for bioenergy use
vol. 14, pp. 465-472 (online: 16 October 2021)
Research Articles
Soil CO2 efflux in uneven-aged and even-aged Norway spruce stands in southern Finland
vol. 11, pp. 705-712 (online: 06 November 2018)
Research Articles
Assessment of sanitary conditions in stands of Norway spruce (Picea abies Karst.) damaged by spruce bud scale (Physokermes piceae Schrnk.)
vol. 6, pp. 73-78 (online: 07 February 2013)
Research Articles
Ozone fumigation effects on the morphology and biomass of Norway spruce (Picea abies L.) saplings
vol. 2, pp. 15-18 (online: 21 January 2009)
Short Communications
Culturable fungi associated with wood decay of Picea abies in subalpine forest soils: a field-mesocosm case study
vol. 11, pp. 781-785 (online: 28 November 2018)
Research Articles
Changes in moisture exclusion efficiency and crystallinity of thermally modified wood with aging
vol. 12, pp. 92-97 (online: 24 January 2019)
Research Articles
Decomposition of Norway spruce and European larch coarse woody debris (CWD) in relation to different elevation and exposure in an Alpine setting
vol. 9, pp. 154-164 (online: 28 August 2015)
Research Articles
Interaction between planting spacing and wood properties of Eucalyptus clones grown in short rotation
vol. 14, pp. 12-17 (online: 02 January 2021)
Research Articles
Effects of substrate and ectomycorrhizal inoculation on the development of two-years-old container-grown Norway spruce (Picea abies Karst.) seedlings
vol. 8, pp. 487-496 (online: 10 November 2014)
Research Articles
Nine-year monitoring of cambial seasonality and cell production in Norway spruce
vol. 9, pp. 375-382 (online: 16 January 2016)
iForest Database Search
Search By Author
Search By Keyword
Google Scholar Search
Citing Articles
Search By Author
Search By Keywords
PubMed Search
Search By Author
Search By Keyword