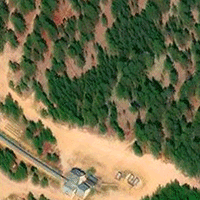
Factors of soil CO2 emission in boreal forests: evidence from Central Siberia
iForest - Biogeosciences and Forestry, Volume 16, Issue 2, Pages 86-94 (2023)
doi: https://doi.org/10.3832/ifor4097-016
Published: Mar 19, 2023 - Copyright © 2023 SISEF
Research Articles
Abstract
Soils of boreal forests are crucial carbon reserves. The response of soil carbon emission to climate change significantly affects the concentration of carbon dioxide in the atmosphere. Soil carbon emission models frequently show a nonlinear response to temperature, but soil moisture is an important limiting factor, often overlooked in energy limited ecosystems. We suggest a statistical model of soil CO2 emission constrained by soil moisture and temperature for different ecosystems in the boreal zone. We tested this modelling strategy using direct measurements of seasonal soil CO2 emission near the research observatory ZOTTO near the Bor settlement, Central Siberia, Russia, in 2012-2017. Soil moisture explained a significant amount of variability of soil emission: the adjusted R2 was twice higher than in the baseline model. Although the temperature-only model describes the annual variability of carbon dioxide emissions quite well, the addition of moisture measurement significantly refines the quality of prediction of the seasonal component dynamics. Models including both temperature and soil moisture could serve as a promising tool to analyze the carbon cycle in boreal forest ecosystems.
Keywords
Boreal Forests, Soil CO2 Emission, Soil Temperature, Soil Moisture, Carbon Cycle, Climate Change, Exponential Model
Introduction
The spatial and temporal controls of variability in soil CO2 emissions are poorly understood and this hampers the development of models of carbon emission ([76], [62], [9]). The ecosystems are usually described by nonlinear, multicomponent and hierarchical models ([37]). When modeling the dynamics of carbon in the ecosystem, one of the most important and commonly used dependencies is the nonlinear temperature sensitivity of soil CO2 emission (Es).
Soil temperature is usually a factor that reliably describes the changes in Es, however, due to the complexity of the soil environment, most researchers rely on empirical models instead of process models ([56], [28]). It is assumed that Q10 function is used as a measure of variability in the annual soil CO2 emission to simulate ecosystem respiration ([41], [11], [54]). However, the use of the well-known Arrhenius and Q10 functions to describe the exponential response of Es to temperature has been criticized ([70], [28], [75]) because of their similar temperature sensitivity over a wide range of soil temperatures ([41]). There is empirical evidence that the temperature sensitivity of Es is declining amid rising the soil temperature when measured within one study area and between different ecosystems ([41], [30], [59], [28]). A decrease in the temperature sensitivity of soil CO2 emissiom during drought was recorded ([7], [70], [59], [28], [74], [35]). Another finding was that higher Q10 is associated with decline of root and leaf decomposition rates ([5]). Seasonal or more detailed temporal resolution analysis is needed to improve understanding of the interactions between environmental variables and Es ([13]).
The dependence of the soil CO2 emission on soil moisture and temperature has been established ([41], [11], [70]). However, it is still unclear how Q10 is influenced by other climatic factors besides temperature ([41], [65], [74], [12]). There are several examples of empirical measurements that demonstrate relationships between Es, temperature, and moisture conditions ([50], [20], [25], [57], [11], [6], [62]). During the growing seasons in the boreal forest ([40]) with current climate change and increasing drought periods, soil moisture is getting the most powerful factor in carbon exchange processes.
The influence of temperature and moisture on Q10 is one of the determining factors to assess the climate change impact on ecosystem carbon fluxes ([31], [45]). Existing global ecosystem models, e.g., DGVMs ([38]), do not incur the different sensitivity of CO2 emission of specific soils related to the temperature and moisture. The errors in predicting annual Es from the global models for boreal and arctic regions are comparatively high as they have low soil CO2 emission rates ([26]).
An important issue in considering the dependence of soil CO2 emission on moisture conditions is the so-called “Birch effect” ([4], [27], [3]), which shows how periodic cycles of drying and rewetting regulate the process of soil organic matter mineralization and, as a result, act as one of the factors modifying soil CO2 emissions.
It was previously noted that for various ecosystems there are moisture conditions leading to an increase in soil CO2 emission; however, as a rule, the range of optimum values of soil moisture is indicated ([67]). In some studies, threshold moisture was employed ([42]) as a specific value of soil moisture for the ecosystem to remove water limitation of the emission process. In these systems, the Es is affected by temperature changes. The reference values of Es are strictly specific to a particular region and ecosystem and cannot be used for other ecosystems.
Our study is aimed at modifying the exponential model of soil CO2 emission by introducing an additional factor, i.e., the threshold soil moisture. The main goal is to test a nonlinear regression model of the seasonal dynamics of soil CO2 emission, accounting for the value of the threshold soil moisture for different ecosystems of the middle taiga region of Central Siberia: lichen pine forest, feathermoss pine forest, mixed forest, destroyed area of lichen pine forest. We focused on solving the following tasks: (i) assess the quality of the model for describing the seasonal dynamics of soil CO2 emission; (ii) conduct a comparative analysis of the model’s performance for different seasons and types of ecosystems; (iii) check the accuracy of the model parameters using the averaged coefficients for all seasons.
Materials and methods
Study area
The research was carried out in the south of the Turukhansk region of the Krasnoyarsk Krai (60° 47′ N, 89° 21′ E) within the Ket-Symskaya lowland near the scientific observatory “ZOTTO” (⇒ http://www.zottoproject.org/).
The territory is located on the border of two climatic zones: subarctic and harsh continental. The average annual long-term temperature is -3.7 °C according to instrumental observations from the Bor (61° 36′ N, 90° 01′ E) weather station (⇒ http://www.meteo.ru/). The amplitude of oscillation of the air temperature within the month can reach 42 °C. The average relative humidity is 76%. The average total precipitation during the year is 590 mm ([53]).
Measurements of soil CO2 emissions, soil temperature and soil moisture
Direct measurements of soil CO2 emission were carried out during the snowless period from June to September in 2012, 2013, 2015, 2016, and from August to September in 2017. The meteorological characteristics of the analyzed growing seasons are significantly different, particularly the amount of precipitation between seasons varied from 119 in 2012 to 328 mm in 2015 (Fig. 1), which is 54% lower and 23% higher respectively compared to the long-term average values from the nearest meteorological station (Bor) for the same period (from June to September).
Fig. 1 - Average temperature and total precipitation over the measurement period (June - September) for a 5-year period and average long-term values of these parameters at the Bor weather station (1936-2017 - air temperature dataset; 1966-2017 - precipitation dataset). Air temperature data is provided with standard errors.
The study sites were selected from the most abundant underline surfaces (Fig. 2): lichen pine forest, feathermoss pine forest, mixed forest, destroyed area (the early stage of recovery after clearcut, until 2005 it was represented by the lichen pine forest). We made one sample plot for each type of underlined surface. Most of them were located under trees except the destroyed area. The measurements were carried out from June to September for every season. The plots were located 250 to 500 m from each other. The main characteristics of the study sites are presented in Tab. 1.
Tab. 1 - Description of the study sites. Average values for soil temperature and moisture are given for a period of all measuring seasons.
Parameter | Lichen pine forest |
Feathermoss pine forest |
Mixed forest |
Destroyed area |
---|---|---|---|---|
Forest age (years) | 73 | 119 | 27 | - |
Forest composition | 10P | 10P | 5B3P2A+S | - |
Mean soil temperature (°C) | 13.81 ± 3.74 | 11.47 ± 2.42 | 12.98 ± 2.55 | 20.04 ± 7.23 |
Mean soil moisture (m3 m-3) | 0.21 ± 0.09 | 0.26 ± 0.12 | 0.25 ± 0.10 | 0.31 ± 0.09 |
Carbon content (g C kg-1 soil) | 13.0 ± 1.5 | 7.0 ± 3.6 | 14.9 ± 1.4 | 7.3 |
Nitrogen content (g N kg-1 soil) | 0.6 ± 0.1 | 0.3 ± 0.1 | 0.6 ± 0.2 | 0.4 |
C/N ratio | 22 | 23 | 25 | 18 |
Measurements were taken once a day between 11:00 and 16:00. Five measuring plastic collars (PVC) were installed in all forested areas, and three collars were installed in the destroyed area. The distance between the collars was determined by the spatial distribution of the living ground cover, the presence of micro-relief, the density of the forest stand, the distance to the nearest tree. The distance did not exceed 2 m which made it possible to more reliably estimate the spatial variation in the soil CO2 emission. Measurements were carried out in three repetitions, on the basis of which the average value was further calculated, the measurement time was 2 minutes with an interval between measurements of 30 seconds.
Direct measurements of soil temperature and moisture were performed directly for each measurement of soil CO2 emission fluxes. Temperature measurements were carried out at three depths (5, 10, and 15 cm from the soil surface) using a Soil Temperature Probe Type E (Omega, USA). A Theta Probe Model ML2x moisture meter (Delta T Devices Ltd., UK) was used to measure the soil moisture content (SWC) at the depth of 5 cm from the soil surface ([43]).
Modeling seasonal soil CO2 emissions
The simulation of soil CO2 emission was carried out according to two models: (i) the classical Lloyd-Taylor exponential model of the dependence of soil emission on the temperature (“T model”); and (ii) a nonlinear regression model (“T-SWC model”) with a newly introduced parameter - the threshold soil moisture for different ecosystems.
The T model is described by the following equation ([41] - eqn. 1):
where E0, and α are parameters reflecting the sensitivity of the flow to a certain initial value of emission and changes in soil temperature, respectively.
The T-SWC model is based on the modification of the exponential model considering the parabolic effect of moisture and the introduction of an additional factor, i.e., the threshold soil moisture (β0), specific for different types of ecosystems (eqn. 2):
The parameter β0 was chosen based on the empirical analysis of several years of the seasonal soil CO2 emission measurements for the different ecosystems.
The threshold soil moisture was defined as the exact soil moisture value (Tab. 2) that corresponds to the minimum soil moisture at which a significant dependence is observed between soil CO2 emission and soil temperature without limitation by moisture conditions. In this study, we used just a linear relationship between soil CO2 emission and soil temperature to find out a significant correlation in comparison to our previous study where we used an exponential relationship between emission and temperature ([44]). The remaining steps of the algorithm for calculating the threshold soil moisture content are identical. The whole procedure consists of five following steps: (i) the soil CO2 emission and soil temperature observations were arranged in a series of increasing soil moisture; (ii) the linear relationship between the CO2 emission and soil temperature was identified for step-by-step selection until the last three measurements on the basis of Fisher’s test - factual correlation coefficient; (iii) the significant correlation coefficient (p < 0.05) was calculated for the different number of observations until last three of them - significant correlation coefficient; (iv) the threshold moisture was identified as a moisture value when the inequality was respected: factual correlation coefficient was higher than significant correlation coefficient.
Tab. 2 - Values of threshold soil moisture (β0) for different types of ecosystems (in m3 m-3).
Ecosystem | β 0 |
---|---|
Lichen pine forest | 0.32 |
Feathermoss pine forest | 0.22 |
Mixed forest | 0.25 |
Destroyed area | 0.26 |
The study of seasonal dynamics of soil CO2 emission was also accompanied with a daily set of soil temperature and moisture data at 8 cm depth from measuring station located close by the study area (⇒ http://www.zottoproject.org/). Continuous measurements for these parameters from June to September for each year were obtained for every ecosystem by a linear transformation of recorded data with 10-minutes resolution. The procedure for calculating daily values of soil temperature and moisture was carried out for each measurement season and type of ecosystem in the period from June 1 to September 30 included (Tab. 3).
Tab. 3 - Equations for calculating daily measurements by temperature (T) and soil moisture (SWC) for the lichen pine forest (p - permanent measurements). (Tp): soil temperature; (SWCp): soil moisture from the depth 8 cm at the measuring station.
Year | Soil temperature (Ts) | Soil moisture (SWC) |
---|---|---|
2012 | Ts= 1.61·Tp - 5.38 (R2= 0.87) | SWC = 4.10·SWCp + 5.10 (R2= 0.83) |
2013 | Ts= 1.21·Tp - 1.17 (R2= 0.94) | SWC = 2.86·SWCp + 4.32 (R2= 0.85) |
2015 | Ts= 1.20·Tp - 0.73 (R2= 0.86) | SWC = 2.74·SWCp + 6.51 (R2= 0.75) |
2016 | Ts= 1.22·Tp - 1.88 (R2= 0.76) | SWC = 3.43·SWCp + 6.75 (R2= 0.74) |
2017 | Ts= 1.19·Tp - 1.63 (R2= 0.98) | SWC = 2.20·SWCp + 14.62 (R2= 0.53) |
Parameterization and estimation of model coefficients
Data processing and further calculations of the model coefficients were obtained by means of the R software environment ([55]) with the “stargazer” ([24]), “tidyverse” ([69]), and “olsrr” ([23]) packages.
The models were linearized to the following forms; the T model as (eqn. 3):
and the T-SWC model as (eqn. 4):
We used the adjusted coefficient of determination (Adj. R2 - [32], [61], [8]) and the Akaike information criterion (AIC - [51]) to estimate the goodness-of-fit and to select the best appropriate regression models.
The value of AIC depends on the number of estimated parameters p and maximum value hat{L}‚ of the model’s likelihood function and was calculated as (eqn. 5):
The higher the value, the higher is the error of the model. An important hint for the modeling results interpretation is that AIC values could be both positive and negative, but the rule is always the same: one should select the model with the lowest value of AIC to get the best fit. Combining the calculation of both R-squared and AIC allows to get a more comprehensive assessment of the goodness-of-fit when dealing with the models with different set of variables. There are cases when a growth of R-squared value is determined by the inclusion of a new variable that does not add any useful information to explain the variance of independent variable. In this case, the use of AIC allows to control for such imaginary overestimates of the explanatory power of the model.
Results
Model coefficients estimation
We estimated the impact of the environmental factors on soil CO2 emission in different ecosystem types based on values of the coefficients α and β (Tab. 4, Tabs. S1-S4 in Supplementary material). In this case, we considered α as an indicator of sensitivity to temperature changes and, accordingly, β to moisture. It was fixed that temperature is mostly affected soil CO2 emission in the feathermoss pine forest (α = 0.111), and moisture in the lichen pine forest (β = 0.365). The values of α coefficients were on average 24%-56% lower than the β values according to the T-SWC model for different ecosystems. Interestingly, in the destroyed area we observed an inverse dependence on soil moisture, since the average value of β coefficient over four seasons of measurement was negative.
Tab. 4 - Models coefficients for all sites averaged over four seasons of measurements. (SE): standard error; (N): number of observations; (Adj. R2): adjusted R2; (AIC): Akaike Information Criterion; (*): p<0.10; (**): p<0.05; (***): p<0.01.
Model | All years | α (SE) | β (SE) | E0 | N | Adj. R2 | AIC |
---|---|---|---|---|---|---|---|
T model | Lichen pine forest | 0.076*** (0.008) |
- | 0.944 | 148 | 0.371 | 153.114 |
Feathermoss pine forest | 0.096*** (0.012) |
- | 1.095 | 136 | 0.325 | 59.203 | |
Mixed forest | 0.062*** (0.010) |
- | 2.018 | 138 | 0.230 | 162.225 | |
Destroyed area | 0.070*** (0.013) |
- | 0.152 | 89 | 0.248 | 211.743 | |
T-SWC model | Lichen pine forest | 0.108*** (0.008) |
0.365*** (0.045) |
0.231 | 148 | 0.566 | 99.391 |
Feathermoss pine forest | 0.111*** (0.010) |
0.200*** (0.027) |
1.143 | 136 | 0.519 | 13.916 | |
Mixed forest | 0.064*** (0.009) |
0.267*** (0.045) |
1.435 | 138 | 0.382 | 132.866 | |
Destroyed area | 0.079*** (0.013) |
-0.157* (0.085) |
1.407 | 89 | 0.267 | 210.315 |
It was possible to form a series of ecosystems’ sensitivities to moisture conditions based on the value of the β coefficient, namely: lichen pine forest > mixed forest > feathermoss pine forest > destroyed area. The introduction of a specific threshold value for the moisture content allowed the analysis of the biological characteristics of the functioning of different ecosystems based on mathematical solutions.
In most cases, adjusted R2 was higher for T-SWC models than for T models. The only exception was the season of 2017 in the forested areas (Tab. S1-S3 in Supplementary material) when both models showed similar results, likely due to the small number of measures. However, in other seasons we recorded a significant difference in the model performances: on average, Adj. R2 for the T-SWC model was higher, depending on the type of ecosystem and season, varying from 0.267 to 0.566, while it varied from 0.230 to 0.371 according to the T model (Tab. 4). During the dry seasons of 2012 and 2013, the largest discrepancy was recorded at the feathermoss pine forest using the T model (Adj. R2 of 0.099 and 0.108, respectively), which was significantly larger than that obtained by T-SWC model for the two seasons (0.315 and 0.470, respectively).
In the season with the highest amount of precipitation (2015), the T and T-SWC models showed the smallest deviation between the calculated and observed CO2 emission, the maximum being about 15-20%, which suggests that the soil CO2 emission flux is not inhibited by moisture conditions in this season at the forested areas. The destroyed area reacted differently: for all the considered seasons the simulation results of the two models showed similar adjusted R2, which indicates a similar models’ implementation for this area.
For a more accurate assessment of the models’ quality the Akaike information criterion (AIC) was calculated. The T-SWC model showed better results than the T model for the forested areas, with the exception of season 2017. The variation in AIC values was significant, both within the same area and among the measurement seasons. The largest scatter of the criterion values was observed for the destroyed area according to the coefficients averaged for all seasons, and among the forested sites, with the mixed forest standing out. Moreover, according to the T model, the variation of the AIC values was always higher than that of the T-SWC model (Tab. 4). This can be explained by a high deviation of the fluxes calculated from the average coefficients of CO2 fluxes from the real values of emissions. At the destroyed site in 2012 (Tab. S4 in Supplementary material), the AIC was non-significantly lower for the T model (AIC = 0.760) than for the T-SWC model (AIC = 0.730). In 2013 and 2015, the AIC values were not significantly different, which may indicate similar performances of two models for calculating emission fluxes from this area.
Modeling CO2 fluxes using the T-SWC model
The T-SWC model showed seasonal fluctuations in the soil CO2 emission among seasons with different precipitation conditions (Fig. 1, Fig. 3). We recorded a maximum differences of about 20% between the simulated fluxes for different ecosystems using the T-SWC model with direct measurements for all considered seasons as a mean seasonal model error. Interestingly, in seasons with different moisture conditions the largest difference between the measured and modeled soil emission fluxes was observed in the same period of the year (beginning-mid June), reaching 60%-80% in some days. The T model often showed the higher difference with the observed soil CO2 emission during the season; however, the average simulated values of emissions over the season are close to the measured ones. Note that in the 2015 season, when heavy rainfalls were recorded, both the T-SWC and the T models demonstrated similar results in soil CO2 emission over the season. This fact may indicate that in the absence of a precipitation deficit, the use of one factor as predictor, i.e., the soil temperature, is sufficient to accurately calculate the soil emission fluxes.
Fig. 3 - Dynamics of soil emission for seasons with different moisture conditions for lichen pine forest. Red squares represent the simulation results for the T model, green rhombuses - direct measurements, blue triangles - the work of the T-SWC model. In the Y-axis is located DOY - Day of year.
All forested areas (lichen pine forest, feathermoss pine forest, mixed forest) shared a general pattern: the largest differences between measured and modeled soil CO2 emission by the T-SWC model were observed mainly in two periods, i.e., the second half of June and the second half of August, reaching 60% error in some days (Fig. 4). The T model did not detect the peak values of soil CO2 emission and during periods of maximum growth of fluxes, the difference between the measured and obtained by the T model CO2 emission was about 25% ± 4%. Regarding the destroyed site, the discrepancies between the CO2 emission rates measured and simulated using the T and T-SWC models throughout the 2013 season were non-significant and amounted to less than 10%, which is presumably related to the origin of the site. The absence of structured soil horizons and ground cover could reduce the sensitivity of this ecosystem to moisture conditions. When comparing the destroyed area with the others, it was evident that the values of both measured and modeled soil emission were 6-10 times lower than in forested areas.
Fig. 4 - Seasonal dynamics of soil emission in different seasons of measurements for different ecosystems in the 2013 season. Red squares represent the simulation results for the T model, green rhombuses - direct measurements, blue triangles - the work of the T-SWC model. In the Y-axis is located DOY - Day of year.
Discussion
In this study the threshold soil moisture was introduced for the first time as an additional parameter in the exponential model of CO2 soil emission from the middle taiga ecosystems of the Central Siberia region. Our results confirm that during the summer period moisture conditions play an important and often decisive role in the dynamics of soil CO2 emission fluxes, as previously emphasized in several studies ([68], [60], [48], [15], [10], [16], [39], [2], [64]). We compared two models for calculating soil emission on seasonal, annual, and interannual scales, of which one is currently widely used, i.e., the Lloyd-Taylor exponential model.
The threshold soil moisture for each ecosystem considered is a specific value that can be calculated by the algorithm previously reported in the study of Makhnykina et al. ([44]). This parameter allows not only to observe the changes in seasonal dynamics of soil CO2 emission more accurately, but also to establish how strongly moisture conditions may limit a specific ecosystem. The lowest threshold moisture value was recorded in the feathermoss pine forest, which indicates its high resistance to changes in the precipitation conditions. The most sensitive was the lichen pine forest which demonstrates a quick response to harsh changes in the amount of available moisture through changes in the soil CO2 emission rates.
T-SWC model: estimates for different ecosystems and different seasons
The model of exponential growth of soil CO2 emission ([41]) predicts a permanent increase in CO2 emission rates with increasing temperature ([77], [72]). In this study, we assumed that the maximum fluxes would occur in the middle of growing season; however, when ecosystems of different origin and different precipitation conditions are to be considered, several shortcomings of this model will become more obvious (Fig. 3, Fig. 4).
The modification of the exponential model was aimed to obtain adequate estimates of the dynamic changes in the soil emission rates along the season. At the beginning of the growing season, many biological and physiological processes are activated ([31], [65], [34]), including soil processes which significantly contribute to the formation of soil CO2 emissions. The introduction of the threshold moisture content (β0) into the exponential model of soil emission makes it possible not only to emphasize the importance of this factor for the biological processes occurring in the soil, but also to account for the peculiarities of a particular ecosystem.
The use of the T-SWC model allowed to identify periods of active growth and decline in soil emission rates along the growing season, which reflect the status of natural ecosystems as either atmospheric CO2 sink or source ([52]). Although seasons differed significantly in terms of precipitation (Fig. 1), the developed model has proven to adequately reflect the changes in soil emission both for seasons with a deficit of precipitation (2012, 2013) and for waterlogged seasons (2015). The same evidence holds for all forested areas.
In the feathermoss pine forest, the soil temperature does not show significant changes along the season, and the soil moisture is maintained at a relatively constant level from June to September, due to the abundant litter and ground cover (here the moss-lichen layer reaches 20-25 cm in thickness). We argue that the thickness of the ground cover in this ecosystem is the main reason for the lowest threshold soil moisture (β0= 0.22). Our results are consistent with previous studies carried out in similar ecosystem in the boreal forests of Canada ([34]) where the average soil temperature in a coniferous forest with a mossy ground cover was lower than in a mixed forest, and reflected the magnitude of seasonal CO2 emission dynamics. By capturing the peculiarities of this ecosystem through the value of the threshold soil moisture in the T-SWC model, it was possible to obtain a high convergence of the modeled emission flux of CO2 with the direct measurements: during periods of maximum emission the discrepancy in the CO2 emission rates did not exceed 15%.
Estimates of the T-SWC model for the mixed forest in this study also showed a high convergence of direct observation and modeled soil emission in all the studied seasons. The largest discrepancy in the peak emission rates (about 15%) was observed in 2013, which was characterized by dry conditions throughout the season (Fig. 1). Interestingly, the soil emission flux in the mixed forest was accurately predicted by means of meteorological parameters solely such as soil temperature and moisture. However, some studies noted that the characteristics of the vegetation cover ([36], [1]), the biomass of roots ([49]) and microorganisms ([14]), as well as the presence of organic matters in the soil ([58]) are significant factors in the formation of the soil CO2 emission.
The highest sensitivity to changes in moisture conditions was recorded in a lichen pine forest. Here, the dynamics of soil CO2 emission along the season was accurately described by the T-SWC model. The largest discrepancy between modeled and measured CO2 fluxes was observed in 2012, when the occurrence of a moisture deficit (Fig. 1) led to the inhibition of respiratory activity until the middle of the season (Fig. 4), likely due to the suppression of many biological processes, such as the decomposition of soil organic matter, the activity of soil heterotrophs ([21]), as well as changes in individual soil properties-aggregate structure, soil water capacity, surface tension, and others ([18]) as a response to water shortage.
The destroyed area, likely due to anthropogenic causes, showed low sensitivity to the variation in both moisture and soil temperature (Fig. 4). Indeed, the forest development at this site had a few significant differences in the rate of post-intervention recovery, including CO2 emission rates ([29]). Seasonal measurements at this site began five years after the disturbance, and now we can assume that the area origin may have an indirect impact on the sensitivity to the environmental variables.
T and T-SWC models comparison
Temperature and moisture conditions and the climatic characteristics of the measuring season have been proven to significantly affect the soil CO2 emission, and should be carefully considered when modeling CO2 efflux. Few studies argued that the annual CO2 emission from the soils is mainly controlled by the temperature and can be predicted based on this environmental factor only. However, research has recently focused on small-scale changes in terms of both temporal and spatial variation of soil CO2 efflux ([63], [22], [77], [2], [17]). The role of moisture conditions along the growing season is especially relevant for forest ecosystems which are sensitive to this effect. Mielnick et al. ([46]) noted that the response of soil emission to changes in moisture conditions can reflect more accurately the dynamic variation in the rate of CO2 fluxes. For prairie region, the mentioned authors obtained estimates that reliably described the seasonal and annual dynamics of soil emission. However, their model had also a drawback, as it focuses on certain growing conditions for which the model was originally created ([46]).
In studies on soil CO2 emissions on a seasonal scale, the inclusion of temperature as the sole predictor in the model has several disadvantages, such as the lack of seasonal fluctuations in soil emission due to variations in the precipitation regime. The inconsistency of models based only on temperature has already been noted ([70], [28], [75]). However, according to several authors, this could also result from the lack of inclusion of other important factors, such as the diffusion of gases in the soil, hydrodynamic soil dispersion ([47]), and the presence of root or mycorrhizal exudates ([66], [33]), among others. This confirms the complexity of the process of soil CO2 emission and its dynamic fluctuations. However, it is worth noting that multicomponent models including additional sets of specific variables tend to be more site- or region-specific, thus losing their general applicability and transferability to other ecosystems
In this study, the seasonal dynamics of soil CO2 emission were poorly described by the T model (Fig. 4) across all forested areas. In the destroyed area with no ground cover, the T model accurately reflects the real CO2 fluxes, since in this case, the temperature was a sufficient factor for obtaining adequate estimates of the seasonal dynamics of soil emission. The development of destroyed areas is one of the most widely discussed at the present time ([73], [19], [29]) due to the rapid decline of natural forests because of anthropogenic actions. However, the intensity of disturbance and the recovery period are often determined by the origin of the ecosystem and by other local environmental factors ([78], [71]). The response of disturbed ecosystems to climatic changes is also specific and is often determined by specific regional features.
Conclusion
The suggested model based on temperature and soil moisture has shown to adequately describe the seasonal dynamics of soil CO2 emission both in different ecosystems and among seasons with different rainfall. The model parameters reflect the influence of each of the considered factors in a specific ecosystem. The model including the temperature as unique predictor can be applied to obtain the average soil CO2 emission over the season but had poor performances for studying the seasonal dynamics.
The model application depends on the microclimatic characteristics of a particular ecosystem, namely, a factor that has a limiting effect on the biological processes in the soil. Further development of the study can expand the number of sites, types of ecosystems with different moisture conditions for further modeling the seasonal soil CO2 emissions. The study of moisture conditions as a crucial factor of forest soil carbon cycle is promising for better understanding the functional role of boreal forest ecosystems, whether they act as sinks or sources of atmospheric CO2.
Authors’ contributions
AM, AP and EV designed the study; IT and AP ran simulations with T and T-SWC models; AM and AP analyzed the data; AM wrote the initial draft of the manuscript. All authors contributed critically to the drafts and gave final approval for publication.
Acknowledgments
The authors thank all the staff members of the international research station “ZOTTO” involved in the measurement process. This study was supported by the Russian Science Foundation (grant no. 19-77-30015). Calculations were made using partial financing from state assignment to the Centre for Forest Ecology and Productivity, Russian Academy of Sciences (project no. AAAA-A18-118052400130-7). Data collection and discussion were made using partial financing from RFBR, Krasnoyarsk Territory and Krasnoyarsk Regional Fund of Science (project no. 20-44-243003).
References
CrossRef | Gscholar
Gscholar
CrossRef | Gscholar
Gscholar
CrossRef | Gscholar
CrossRef | Gscholar
CrossRef | Gscholar
Gscholar
CrossRef | Gscholar
CrossRef | Gscholar
Authors’ Info
Authors’ Affiliation
Anatoly S Prokushkin 0000-0001-8721-2142
Eugene A Vaganov 0000-0001-9168-1152
V.N. Sukachev Institute of Forest, Siberian Branch of the Russian Academy of Sciences 660036, Krasnoyarsk (Russia)
Ivan I Tychkov 0000-0001-5445-1323
Anatoly S Prokushkin 0000-0001-8721-2142
Anton I Pyzhev 0000-0001-7909-3227
Eugene A Vaganov 0000-0001-9168-1152
Siberian Federal University 660041, Krasnoyarsk (Russia)
Eugene A Vaganov 0000-0001-9168-1152
Center for Forest Ecology and Productivity, Russian Academy of Sciences 117997, Moscow (Russia)
Corresponding author
Paper Info
Citation
Makhnykina AV, Tychkov II, Prokushkin AS, Pyzhev AI, Vaganov EA (2023). Factors of soil CO2 emission in boreal forests: evidence from Central Siberia. iForest 16: 86-94. - doi: 10.3832/ifor4097-016
Academic Editor
Ana Rey
Paper history
Received: Mar 09, 2022
Accepted: Jan 02, 2023
First online: Mar 19, 2023
Publication Date: Apr 30, 2023
Publication Time: 2.53 months
Copyright Information
© SISEF - The Italian Society of Silviculture and Forest Ecology 2023
Open Access
This article is distributed under the terms of the Creative Commons Attribution-Non Commercial 4.0 International (https://creativecommons.org/licenses/by-nc/4.0/), which permits unrestricted use, distribution, and reproduction in any medium, provided you give appropriate credit to the original author(s) and the source, provide a link to the Creative Commons license, and indicate if changes were made.
Web Metrics
Breakdown by View Type
Article Usage
Total Article Views: 21670
(from publication date up to now)
Breakdown by View Type
HTML Page Views: 18312
Abstract Page Views: 1841
PDF Downloads: 1186
Citation/Reference Downloads: 0
XML Downloads: 331
Web Metrics
Days since publication: 850
Overall contacts: 21670
Avg. contacts per week: 178.46
Article Citations
Article citations are based on data periodically collected from the Clarivate Web of Science web site
(last update: Mar 2025)
Total number of cites (since 2023): 3
Average cites per year: 1.00
Publication Metrics
by Dimensions ©
Articles citing this article
List of the papers citing this article based on CrossRef Cited-by.
Related Contents
iForest Similar Articles
Research Articles
The manipulation of aboveground litter input affects soil CO2 efflux in a subtropical liquidambar forest in China
vol. 12, pp. 181-186 (online: 10 April 2019)
Research Articles
Effects of tree species, stand age and land-use change on soil carbon and nitrogen stock rates in northwestern Turkey
vol. 9, pp. 165-170 (online: 18 June 2015)
Research Articles
Soil stoichiometry modulates effects of shrub encroachment on soil carbon concentration and stock in a subalpine grassland
vol. 13, pp. 65-72 (online: 07 February 2020)
Research Articles
Soil respiration along an altitudinal gradient in a subalpine secondary forest in China
vol. 8, pp. 526-532 (online: 01 December 2014)
Research Articles
Soil respiration and carbon balance in a Moso bamboo (Phyllostachys heterocycla (Carr.) Mitford cv. Pubescens) forest in subtropical China
vol. 8, pp. 606-614 (online: 02 February 2015)
Research Articles
Different harvest intensity and soil CO2 efflux in sessile oak coppice forests
vol. 9, pp. 546-552 (online: 25 March 2016)
Research Articles
Soil C:N stoichiometry controls carbon sink partitioning between above-ground tree biomass and soil organic matter in high fertility forests
vol. 8, pp. 195-206 (online: 26 August 2014)
Research Articles
Comparison of soil CO2 emissions between short-rotation coppice poplar stands and arable lands
vol. 11, pp. 199-205 (online: 01 March 2018)
Research Articles
Thinning effects on soil and microbial respiration in a coppice-originated Carpinus betulus L. stand in Turkey
vol. 9, pp. 783-790 (online: 29 May 2016)
Research Articles
Potential relationships of selected abiotic variables, chemical elements and stand characteristics with soil organic carbon in spruce and beech stands
vol. 14, pp. 320-328 (online: 09 July 2021)
iForest Database Search
Search By Author
Search By Keyword
Google Scholar Search
Citing Articles
Search By Author
Search By Keywords
PubMed Search
Search By Author
Search By Keyword