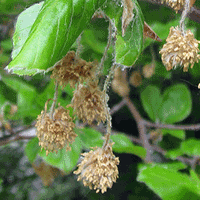
Predicting phenology of European beech in forest habitats
iForest - Biogeosciences and Forestry, Volume 11, Issue 1, Pages 41-47 (2018)
doi: https://doi.org/10.3832/ifor1820-010
Published: Jan 09, 2018 - Copyright © 2018 SISEF
Research Articles
Abstract
Reliable phenological observations are important for studying the response of trees to climate and climate change. National phenological networks were not specifically established to monitor tree phenology within forests, yet they are often used to generalise tree phenological phases at national or regional scales. Our objective was to investigate whether a phenological monitoring network using trees in open areas can accurately predict phenology of European beech (Fagus sylvatica L.) located within forests by using two models: one with correlates of environmental variables and one with interpolated monthly air temperature and sun hours. The first leaf unfolding, general leaf colouring and leaf fall dates from 2004 through 2010 were modelled using data from 47 Slovene National Phenology Network (NPN) stations in open areas and tested on phenological observations within forests using data from the UNECE CRLTAP ICP Forests network. Good agreement was found between predicted and observed first leaf unfolding in the forest, while slightly lower agreement was detected for general leaf colouring and leaf fall. Suggestions for the improvement of national phenological network are discussed in order to better predict beech phenology in forest habitats.
Keywords
Leaf Unfolding, Leaf Colouring, Leaf Fall, Modelling, Fagus sylvatica, Slovene National Phenology Network, ICP Forests
Introduction
The life cycle of many plant and animal species are triggered by environmental signals, such as temperature and light ([15]). As a consequence, any change in temperature could impact the phenological phases of both plants and animals, which are therefore very important indicators of climate change ([35]). The mean global temperature has increased over the last decade, as well as the occurrence of temperature extremes ([19]). Consequently, shifts of species’ ranges and phenology were observed in many regions ([28], [3], [13], [23], [7]) with possible important impacts on species interactions. For instance, different rates in temporal shifts cause disruptions in plant-animal relationships ([2], [36], [35], [16]).
To anticipate the effect of climate change on the phenology of certain species, the reliability of observations from phenological monitoring is of paramount importance. In Europe, several long-term phenological monitoring networks have been established and run by the national meteorological services ([43], [28]). The longest records of direct phenological observations are for the flowering of cherry trees Prunus jamasakura in Japan ([1]) and for grape harvests in Western Europe ([8]). In phenological monitoring networks, different plant and animal groups have been considered, including trees, lower plants, birds and butterflies ([43], [32]). However, phenological observations of tree phenological phases have proven to be one of the most effective indicators of the impact of climate change ([15]). For instance, leaf unfolding is strongly influenced by temperature ([27], [28], [9]) and photoperiod ([6]). Apart from the global climate change, site characteristics (altitude, slope, aspect etc.) also have strong influences on temperature and therefore on the phenology of lower plants and trees ([33]). Altitude has an inverse relationship with temperature, and therefore phenological events occur later at higher altitudes ([12], [9], [33]). In the Northern Hemisphere, sites with steeper slopes and with south-western aspects become warmer earlier in the season, and consequently, the phenological events there occur earlier than on northern slopes ([33]). Furthermore, the effect of local climate is buffered in forests, e.g., the temperature extremes measured outside the forest are not so pronounced within forest stands or in the canopy ([29]). Consequently, the tree phenology within and outside forests could be different.
To describe and model the spatial variability of tree phenological phases at national or regional scales, a phenological monitoring network should maximise the accuracy of the definitions and techniques used and the quality of the data ([43]). Although many national phenological monitoring networks have their phenological stations equally distributed over altitude, slope, and aspect, most stations are either outside the forest or on its edge ([28], [20]). This could have implications for the observed tree phenological phases and the generalisations resulting from these observations at national or regional scales.
Our objective was to evaluate whether tree phenological phases within forests can be predicted based on tree phenological phases observed at national phenological stations. To this purpose, we used two different models to assess first leaf unfolding, general leaf colouring and leaf fall of European beech (Fagus sylvatica L.) in forests. The models were simple enough to be applied for the major tree species over a large range of possible weather conditions: one model involves correlates of environmental variables and the other model uses interpolated monthly air temperature and sun hours. The first model is based on on-site data, readily available in any phenological network but with regionally limited application, while the second model is appropriate for wider application (e.g., regional, European), but based on interpolated data. Suggestions for national phenological network improvements are discussed, aiming to enhance the predictions of tree phenological phases in forests at national or regional scales.
Methods
Phenological observations
We used data on manual phenological observations of the timing of first leaf unfolding, general leaf colouring and leaf fall of beech in Slovenia. Slovenia is characterised by fairly large gradients of climatic factors due to its position between the Alps, the Mediterranean and continental Europe ([9], [25]). Consequently, a great variety of forest habitats, from the lowlands to the high mountains, can be found in the country ([21]). The phenological stations are distributed throughout Slovenia, with altitudes ranging from 55 to 1050 m a.s.l. and with different site and climatic characteristics (Fig. 1 - see also Tab. S1 and Tab. S2 in Supplementary material). The period of observation was from 2004 until 2010. The data originated from two monitoring networks, described below.
Fig. 1 - Phenological stations of the Slovenian National Phenological Network of the Slovenian Environment Agency (NPN - red cicles) and the Level II phenological monitoring network of the UNECE International Co-operative Programme on Assessment and Monitoring of Air Pollution Effects on Forests (ICP Forests - blue triangles) in Slovenia.
(A) The monitoring network of the National Phenological Network of the Slovenian Environment Agency (NPN). The selection of trees and the observations were made in accordance with national guidelines ([44]), which in general follow the World Meteorological Organization (WMO) Guidelines for phenological observations ([20]). Monitoring of different phenological phases, as suggested for perennial plants, was performed on individual adult trees in natural populations. The monitored trees were located in the forest, at forest edge and in open areas. Observations were made at 47 phenological stations on a single tree per station. If one of the selected trees died or was removed, it was replaced with a nearby tree, in accordance with the criteria for phenological monitoring. In spring, the observed period of leaf unfolding and leaf development spans approximately 4 weeks, during which the observations were carried out every day. The day of leaf unfolding was recorded when the first regular leaf surface became visible in 3-4 buds on the observed tree. This phase corresponds to the Biologische Bundesanstalt, Bundessortenamt und Chemische Industrie code 11 (BBCH11 - [26]). The day of leaf colouring was recorded when at least 50% of leaves had turned yellow on the observed tree (BBCH code 94). The day of leaf fall was recorded when at least 50% of the leaves had fallen from the tree crown (BBCH code 95).
(B) The Level II phenological monitoring network of the UNECE International Co-operative Programme on Assessment and Monitoring of Air Pollution Effects on Forests (ICP Forests - Fig. 1, blue triangles) in Slovenia ([38]), where the selection of trees and the observations were made in accordance with the harmonised guidelines ([4], [37]). Phenological monitoring was performed on 20 individual trees from each of 5 monitoring plots (phenological stations, 75 × 75 m). The assessments were always performed on the same trees. If one of the selected trees died or was removed, it was replaced with a nearby tree, in accordance with the criteria for phenological monitoring. All the selected trees were dominant or co-dominant, and the upper part of tree crowns was monitored using binoculars. If the upper part of the crown was not visible, the middle part of the crown was monitored. The same part of the crown was considered for subsequent phenological observations throughout the observation years. Biotic (pests and/or diseases) and abiotic damage (e.g., frost, wind, or hail) relevant to phenological development of trees was also considered. The observations during leaf unfolding, leaf colouring and leaf fall were performed weekly. The week of leaf unfolding was recorded when the first regular leaf surface became slightly visible in up to 33% of the observed part of the crown. The week of leaf colouring was recorded when coloured leaves in autumn became abundant in more than 66% of the monitored part of the crown. The week of leaf fall was recorded when leaves fell in autumn from the entire (100%) observed part of the crown.
Analysis
It is worth emphasizing that NPN protocols were not specifically established to monitor tree phenology within the forest. Direct comparison of phenological observations from the two monitoring networks is unfeasible because of differences in spatial distribution of phenological stations and the number of observed trees per station. Therefore, two different models were tested to predict first leaf unfolding, general leaf colouring and leaf fall of beech in forests, using NPN phenological observations as the training dataset and the ICP Forests phenological observation as validation dataset. To overcome the differences in the observation time-step of the first leaf unfolding, general leaf colouring and leaf fall from the two phenological networks, the week of the year (WOY) was calculated from the daily phenological observations in the NPN dataset, similarly to the ICP Forests weekly observation dataset.
In order to predict WOY for selected phenological phases, two models with different sets of variables were built based on the NPN phenological observations data using a general linear mixed model (GLMM):
- Model 1 was developed based on correlates of environmental variables at the NPN stations: altitude, slope (square root transformed), aspect (N: north; NE: north-east; E: east; SE: south-east; S: south; SW: south-west; W: west; and NW: north-west) and year (2004 to 2010).
- Model 2 included the following independent variables: interpolated moving average of air temperature and number of sun hours (one, two or three months) at the NPN stations based on gridded meteorological data at 1 km resolution ([14]). The months refer to the months before and the month during the phenological phase.
Model selection was performed as follows: for each of the models (model 1 and model 2) all combinations of the selected variables in a model were built. The Akaike Information Criterion (AIC) was used to select the best model ([5]), and the combinations of variables that were within four units of the AIC from the best performing combination of variables were averaged to one final model. As there were more phenological observations in each station, the station code was included in the GLMM as a random effect.
For the predictions of the phenological phases in forests, final models 1 and 2 were run using the data of the ICP Forests stations on slope, altitude, aspect, and observation year (model 1) or interpolated average monthly temperature and number of sun hours (model 2). The tree number (1 to 20) was nested into the ICP Forests station and included as a random effect. The predicted WOY of models for the phenological phases in forests were compared with the observed WOY of ICP Forests data using another GLMM. Models predictions were also compared to the NPN dataset to test the robustness of the models.
The performance of the models was evaluated based on the following statistics: R2; Pearson correlation coefficient (r), which describes the degree of correspondence between observed and predicted values; index of agreement (D), which is a descriptive measure of relative error; and the root mean square error (RMSE), which expresses the error between the observed and predicted values ([34]). By comparing the indices (R2, r, D, RMSE) of the models 1 and 2, which predict phenological phases at NPN stations and in forests, respectively, we can evaluate how well the NPN data can predict tree phenological phases in forests.
Results
First leaf unfolding
On average, the first leaf unfolding was recorded in the 16th week of the year (16.4 WOY, mid-April). The earliest first leaf unfolding was recorded in 2007 (14.9 WOY), while the latest in 2005 (17.2 WOY). For model 1, the first leaf unfolding showed high positive correlation with altitude in all models (weight Wi = 1.00 - Tab. 1). A trend was detected between the slope and the date of first leaf unfolding, though it was non-significant and has been found only in a few models (Wi = 0.21). The year of observation was an important variable explaining the date of leaf unfolding in all models (Wi = 1.00). For model 2, the first leaf unfolding showed high negative correlation with the average temperature from the months February, March and April (Wi = 1) and the number of sun hours in April (Wi = 0.28 - Tab. 2). As expected, both models predicted well the date of first leaf unfolding in open areas (Tab. 3). Predictions of the date of first leaf unfolding in the forest were slightly better by model 2 compared to model 1 (Fig. 2, Tab. 3) with relatively higher R2 (0.45 vs. 0.40 for model 2 vs. model 1, respectively), higher r (0.78 vs. 0.63) and lower D (0.75 vs. 0.70, respectively). The RMSE between the observed and predicted first leaf unfolding in the forest was similar for both models (1.1 WOY). Furthermore, there were no significant differences between the observed date of first leaf unfolding in the forest and model predictions (for model 1: t = 0.344, P = 0.731; for model 2: t = -0.912, P = 0.362).
Tab. 1 - Independent variables included in model 1 explaining the first leaf unfolding, general leaf colouring and leaf fall in European beech at the NPN phenological stations from 2004-2010. The estimates were averaged from the models that were four Akaike Information Criterion (AIC) units from the best model. The classes were compared with the first class in the variable (year=2004; aspect=East). Slope was square root transformed. (WOY): week of the year; (SE): standard error.
Dependent variable |
Independent variable |
Estimate | SE | Adjusted SE |
z value |
Pr(>|z|) |
---|---|---|---|---|---|---|
First leaf unfolding (WOY) |
(Intercept) | 16.16 | 0.24 | 0.24 | 66.54 | <0.001 |
year: 2005 | 0.15 | 0.13 | 0.13 | 1.14 | 0.250 | |
year: 2006 | -0.13 | 0.13 | 0.13 | 0.98 | 0.327 | |
year: 2007 | -2.19 | 0.13 | 0.13 | 16.84 | <0.001 | |
year: 2008 | -1.15 | 0.14 | 0.14 | 8.40 | <0.001 | |
year: 2009 | -1.69 | 0.13 | 0.13 | 12.81 | <0.001 | |
year: 2010 | -0.40 | 0.13 | 0.13 | 2.97 | 0.003 | |
altitude | 0.00 | 0.00 | 0.00 | 5.64 | <0.001 | |
sqrt(slope) | -0.10 | 0.05 | 0.06 | 1.84 | 0.066 | |
General leaf colouring (WOY) |
(Intercept) | 42.16 | 0.42 | 0.43 | 99.05 | <0.001 |
year: 2005 | 0.01 | 0.21 | 0.21 | 0.07 | 0.944 | |
year: 2006 | 0.31 | 0.21 | 0.21 | 1.49 | 0.136 | |
year: 2007 | -1.82 | 0.22 | 0.22 | 8.20 | <0.001 | |
year: 2008 | -1.59 | 0.27 | 0.27 | 5.94 | <0.001 | |
year: 2009 | -0.22 | 0.22 | 0.22 | 1.01 | 0.314 | |
year: 2010 | -0.49 | 0.23 | 0.23 | 2.17 | 0.030 | |
aspect: flat | -0.74 | 0.64 | 0.66 | 1.12 | 0.263 | |
aspect: N | -1.19 | 0.62 | 0.64 | 1.85 | 0.065 | |
aspect: NE | 0.71 | 0.76 | 0.78 | 0.91 | 0.363 | |
aspect: NW | -0.30 | 0.74 | 0.76 | 0.39 | 0.693 | |
aspect: S | -1.26 | 0.55 | 0.57 | 2.22 | 0.027 | |
aspect: SE | -0.60 | 0.60 | 0.62 | 0.97 | 0.332 | |
aspect: SW | -0.12 | 0.69 | 0.71 | 0.18 | 0.861 | |
aspect: W | -0.24 | 0.57 | 0.59 | 0.41 | 0.684 | |
sqrt(slope) | 0.11 | 0.12 | 0.13 | 0.89 | 0.372 | |
Leaf fall (WOY) |
(Intercept) | 43.63 | 0.25 | 0.25 | 174.74 | <0.001 |
year: 2005 | 0.00 | 0.19 | 0.19 | 0.03 | 0.980 | |
year: 2006 | 0.54 | 0.19 | 0.19 | 2.80 | 0.005 | |
year: 2007 | -1.26 | 0.24 | 0.24 | 5.14 | <0.001 | |
year: 2008 | -1.26 | 0.27 | 0.27 | 4.64 | <0.001 | |
year: 2009 | 0.44 | 0.20 | 0.20 | 2.16 | 0.031 | |
year: 2010 | -0.22 | 0.21 | 0.21 | 1.06 | 0.289 | |
aspect: flat | -0.31 | 0.58 | 0.60 | 0.51 | 0.607 | |
aspect: N | -0.44 | 0.58 | 0.60 | 0.75 | 0.455 | |
aspect: NE | 1.28 | 0.7 | 0.72 | 1.78 | 0.075 | |
aspect: NW | 0.15 | 0.67 | 0.70 | 0.22 | 0.825 | |
aspect: S | -0.66 | 0.5 | 0.52 | 1.26 | 0.206 | |
aspect: SE | 0.39 | 0.54 | 0.56 | 0.69 | 0.488 | |
aspect: SW | 0.45 | 0.63 | 0.65 | 0.70 | 0.486 | |
aspect: W | -0.08 | 0.52 | 0.54 | 0.14 | 0.885 |
Tab. 2 - Independent variables included in model 2 explaining the first leaf unfolding, general leaf colouring and leaf fall of European beech at the NPN phenological stations from 2004-2010. The estimates were averaged from the models that were four Akaike Information Criterion (AIC) units from the best model. (WOY): week of the year; (SE): standard error.
Dependent variable | Independent variable | Estimate | Std. Error |
Adjusted SE |
Z value |
Pr(>|z|) |
---|---|---|---|---|---|---|
First leaf unfolding (WOY) | (Intercept) | 19.58 | 0.21 | 0.21 | 93.67 | <0.001 |
Average temperature in April, March and February | -0.55 | 0.04 | 0.04 | 12.35 | <0.001 | |
No of sun hours in April | -0.004 | 0.001 | 0.001 | 3.56 | <0.001 | |
General leaf colouring (WOY) | (Intercept) | 36.46 | 0.64 | 386.00 | 56.80 | <0.001 |
Average temperature in September and October | 0.43 | 0.05 | 386.00 | 8.62 | <0.001 | |
Leaf fall (WOY) | (Intercept) | 37.91 | 0.67 | 208.00 | 56.84 | <0.001 |
Average temperature in September | 0.39 | 0.04 | 208.00 | 8.66 | <0.001 |
Tab. 3 - Performance assessment of model 1 and model 2 based on R2, Pearson correlation coefficient (r), index of agreement (D) and the root mean square error (RMSE) between model predictions and observed phenological phases in European beech at phenological stations (NPN) and in the forest (ICP Forests). (WOY): week of the year.
Phenological phases |
Model | R2 | r | D | RMSE (WOY) | ||||
---|---|---|---|---|---|---|---|---|---|
NPN | ICP Forests |
NPN | ICP Forests |
NPN | ICP Forests |
NPN | ICP Forests |
||
First leaf unfolding | Model 1 | 0.56 | 0.40 | 0.77 | 0.63 | 0.85 | 0.75 | 0.8 | 1.1 |
Model 2 | 0.68 | 0.45 | 0.83 | 0.78 | 0.89 | 0.70 | 0.7 | 1.1 | |
General leaf colouring | Model 1 | 0.33 | -1.12 | 0.58 | 0.20 | 0.70 | 0.47 | 1.3 | 1.8 |
Model 2 | 0.50 | 0.15 | 0.71 | 0.60 | 0.79 | 0.54 | 1.2 | 1.6 | |
Leaf fall | Model 1 | 0.31 | 0.23 | 0.56 | 0.51 | 0.66 | 0.66 | 1.2 | 1.4 |
Model 2 | 0.61 | -0.12 | 0.80 | 0.63 | 0.86 | 0.57 | 0.9 | 1.6 |
Fig. 2 - Comparison between the predicted and observed week of the year (WOY) of the phenological phases in European beech at phenological stations in the forest (ICP Forests). (a) First leaf unfolding date; (b) general leaf colouring date; (c) leaf fall date; (*): P<0.05; (**): P<0.01; (***): P<0.001.
General leaf colouring
On average, the general leaf colouring was recorded in the 41st week (41.4 WOY - early October). In model 1, the general leaf colouring was highly influenced by the year of observation (Wi = 1 - Tab. 1). The earliest date of leaf colouring was observed in 2007 (40.0 WOY), while the latest was observed in 2006 (42.2 WOY). Aspect was another important variable explaining general leaf colouring, but contributed little to the averaged model (Wi = 0.52 - Tab. 1). In general, the earliest leaf colouring occurred at stations with southern aspect (40.7 WOY), while it was latest at stations with a north-eastern aspect (42.8 WOY). The general leaf colouring was not significantly related to the slope (z = 0.893, P = 0.372), and did not contribute significantly to the averaged model (Wi = 0.07). For model 2, the general leaf colouring showed positive correlation to average monthly temperature in September and October (Tab. 2). Both models satisfactorily predicted general leaf colouring dates in open areas (Tab. 3), with slightly better predictions for model 2 compared to model 1. However, the fit was lower compared to the first leaf unfolding. The leaf colouring in the forest was not predicted satisfactorily by model 1 (Tab. 3). The difference between the predicted general leaf colouring in forest by model 1 and that observed was not statistically significant (t = 0.515, P = 0.607). Model 2 predictions were better compared to model 1, but still not satisfactory (Tab. 3). Model 2 predicted the leaf colouring in forest later than it was observed (t = 17.48, P < 0.001 - Fig. 2b).
Leaf fall
On average, the leaf fall started at the 43th week of the year (43.46 WOY, late October). In model 1, the leaf fall was strongly affected by the year of observation (Wi = 1 - Tab. 1). The earliest leaf fall was in 2007, occurring in the 41st week (41.95), while the latest was in 2009 (43.95 WOY). The earliest leaf fall dates were at the stations with southern aspects in the 42nd week (42.77 WOY). The latest leaf fall was at the stations with north-eastern aspects (44.83 WOY). Leaf fall at phenological stations with an eastern aspect was later when compared to either flat areas or other aspects, though not significantly different. However, aspect scarcely contributed to the averaged model (Wi = 0.22). For model 2, the leaf fall date showed a positive correlation to the average temperature in September (Tab. 2).
Both models satisfactorily predicted leaf fall in open areas (Tab. 3), with better predictions for model 2 compared to model 1. However, the fit was lower compared to the first leaf unfolding. The leaf fall in the forest was satisfactorily predicted by model 1 (Tab. 3). However, model 1 predictions of leaf fall in the forest were earlier than that observed (t = -8.287, P < 0.001). Model 2 predictions of leaf fall in the forest were worse compared to model 1 and unsatisfactory (Tab. 3). Model 2 predicted leaf fall in the forest even earlier than model 1 (t = -31.94, P < 0.001 - Fig. 2c).
Discussion
Beech phenology is generally considered to be less sensitive to environmental variability than some other tree species ([18], [10], [42]). This study demonstrates that first leaf unfolding dates in beech can be satisfactorily predicted based on tree phenological phases observed at national phenological stations. For general leaf colouring the predictions were not satisfactory, whereas for the leaf fall only predictions of the model using correlates of environmental variables at phenological stations were satisfactory; however, the fit was lower compared to the first leaf unfolding.
First leaf unfolding in beech is strongly related to temperature ([27], [28]) and photoperiod ([6]). It seems to be less sensitive to micro-topographical factors, as reported by Davi et al. ([10]) in France where the first leaf unfolding of beech did not significantly differ between northern and southern aspects in 2007, though showing a high year-to-year variability. This suggests that factors at a macroecological scale, such as altitude, are mainly affecting the beech first leaf unfolding ([9]). The results of this study show that first leaf unfolding of beech in forest habitats could be predicted based on phenological observations at national phenological stations. The developed model, based on interpolated monthly air temperature and sun hours, allows application over a large range of possible weather conditions (e.g., regional, European). Nevertheless, when only on-site data on environmental correlates at phenological stations are available (e.g., altitude, slope, aspect), regionally-limited but satisfactory predictions of first leaf unfolding of beech in forest could be expected.
In contrast to first leaf unfolding, the environmental factors driving autumn phenological events, such as leaf colouring or leaf fall, are less well understood ([11], [22], [42]). The main drivers of general leaf colouring of beech are supposed to be both photoperiod and low temperatures, contributing to the smaller year-to-year variability observed ([17], [42]). The results of this study, however, indicate a high sensitivity of general leaf colouring to the year of observation. General leaf colouring of beech in forests could not be satisfactorily predicted by any of the models applied. Predictions of the model based on interpolated monthly air temperature and sun hours at phenological stations were slightly better compared to predictions of the model based on environmental correlates; however, the predicted leaf colouring in forest was later than that observed. One of the reasons might be the difficulty to accurately acquire leaf senescence observations ([18]). Indeed, the phenological phase “leaf colouring” is obviously more problematic to be recognised than the first three green leaves in spring, as the definition refers to 50% of all coloured leaves, including leaves both on branches and on the ground. The variation among individual trees at comparable or identical sites is much higher than in first leaf unfolding. Moreover, in this study local site conditions at phenological stations related to soil properties and radiation were not undertaken due to the lack of relevant auxiliary data.
The leaf fall in the forest was satisfactorily predicted only by the model based on site-dependent environmental correlates, revealing a weak sensitivity to site aspect. However, leaf fall in the forest was predicted to occur 1.4 WOY (about 10 days) later than that observed. Nonetheless, our results show that beech leaf fall in forest habitats could be predicted based on phenological observations at national phenological stations using the model based on environmental correlates at phenological stations (e.g., altitude, slope, aspect). Contrastingly, predictions of the model based on interpolated monthly air temperature and hours of sun were not satisfactory, though they could be improved by on-site measurements of climatic data (e.g., air temperature, solar radiation, etc. - [39]).
Further factors affecting predictions of beech phenology in forests can be explained by the two phenological networks and protocols which were different in several features. First, the phenological phases of the NPN network were recorded daily, and the whole tree crown was monitored ([20]). In contrast, the phenological phases of the ICP Forests network were monitored weekly, and only the upper or middle part of the crown was observed ([4]). In this study, the differences in the observation time-step were overcome by synchronising the observations through WOY. Another difference between the NPN and the ICP Forests datasets was the number of trees observed per phenological station. In the NPN network, monitoring of different phenological phases is performed on a single tree, whereas 20 trees are observed in the ICP Forests stations. Our results show a large variation in the weekf of the year (WOY) observed for the trees within the ICP Forests station for the phenological phases investigated. Thus, the NPN network reflects the phenological behaviour of a single tree, which may not be necessarily representative of the larger region surrounding the phenological station, as suggested by the national Guidelines for Phenological Observations ([44]). Vitasse et al. ([40]) reported high variation in the sensitivity to temperature of beech leaf phenology within populations. On the other hand, the phenological observations from 20 trees at the ICP Forests stations are likely to better represent the phenology of the local tree population. Another important difference between the monitoring networks were the local site characteristics of the phenological stations. Indeed, the NPN phenological monitoring network uses trees in open areas and on the forest edge, whereas ICP Forests monitors trees within forest stands in larger forest complexes. Due to the direct influence of open-field temperatures and solar radiation in open areas or at forest edges ([30]) and less pronounced temperature extremes within forest stands or in the canopy ([29]), lower agreement between beech phenology at phenological stations in open areas or in the forest would be expected.
Interestingly, the differences between the phenological observations in forests and the model predictions were not consistent among phenological phases and years. High inter-annual variation in beech phenology has been reported by several authors ([24], [10]). As beech shows a high phenotypic plasticity in leaf phenology, it reacts directly to temperature changes ([41]), which could be reflected in changes in the phenological phases among years. However, the inter-annual differences in the phenological observations in forests and the model predictions could be due to inaccurate prediction by model 1. In Sweden, inter-annual variation in tree phenology was also modelled for different bioclimatic regions ([31]), with most of the models inaccurately predicting the inter-annual variation. In this study, the combination of local site characteristics (e.g., weather, temperature) with differences among beech provenances across sites could partially account for the unsatisfactory predictions of the model and the variation of phenological observations among years.
Conclusions
This study shows that the models satisfactorily predicted the first leaf unfolding and leaf fall dates of beech in selected forest habitats, while general leaf colouring was less accurately predicted. Especially in areas with complex terrain, models are prone to incorrectly predict phenological phases ([31]). Therefore, we recommend combining ICP Forests and NPN methodologies to improve the national phenological network. First, more trees should be monitored per phenological station to decrease the effect of individual variability on the phenological assessment. Second, observations should be carried out every day to improve the temporal resolution of datasets. Third, the forest phenological stations should be more evenly distributed along the altitudinal range of the natural forest habitats of the tree species under study. Finally, measurement of climatic data should be performed at phenological stations established in complex terrains to overcome the limitations of spatial interpolated data. The results of the models presented in this study could also be tested on other tree species at a larger scale.
Acknowledgements
The authors would like to acknowledge the financial support from the following sources: the Slovenian Research Agency (research core funding No. 0404-501; the Programme Group “Forest biology, ecology and technology”), ICP Forests and the Forest monitoring in Slovenia, the ManFor C.BD project “Managing forests for multiple purposes: carbon, biodiversity and socio-economic wellbeing” (LIFE09ENV/IT/00 0078) and EUFORINNO “European Forest Research and Innovation” (Reg. Pot No. 315982). We would like to thank Jean McCollister for proofreading the manuscript. In addition, we thank the reviewers for their helpful comments on the manuscript.
The first two authors (U.V. and M.D.G.) have contributed equally to the article.
References
CrossRef | Gscholar
Gscholar
Gscholar
Gscholar
CrossRef | Gscholar
Online | Gscholar
CrossRef | Gscholar
Gscholar
Authors’ Info
Authors’ Affiliation
Maarten De Groot
Mitja Skudnik
Primož Simončič
Slovenian Forestry Institute, Večna pot 2, 1000 Ljubljana (Slovenia)
Slovenian Environment Agency, Vojkova 1b, 1000 Ljubljana (Slovenia)
Corresponding author
Paper Info
Citation
Vilhar U, De Groot M, Zust A, Skudnik M, Simončič P (2018). Predicting phenology of European beech in forest habitats. iForest 11: 41-47. - doi: 10.3832/ifor1820-010
Academic Editor
Giorgio Matteucci
Paper history
Received: Aug 20, 2015
Accepted: Nov 08, 2017
First online: Jan 09, 2018
Publication Date: Feb 28, 2018
Publication Time: 2.07 months
Copyright Information
© SISEF - The Italian Society of Silviculture and Forest Ecology 2018
Open Access
This article is distributed under the terms of the Creative Commons Attribution-Non Commercial 4.0 International (https://creativecommons.org/licenses/by-nc/4.0/), which permits unrestricted use, distribution, and reproduction in any medium, provided you give appropriate credit to the original author(s) and the source, provide a link to the Creative Commons license, and indicate if changes were made.
Web Metrics
Breakdown by View Type
Article Usage
Total Article Views: 40093
(from publication date up to now)
Breakdown by View Type
HTML Page Views: 34206
Abstract Page Views: 1996
PDF Downloads: 3027
Citation/Reference Downloads: 23
XML Downloads: 841
Web Metrics
Days since publication: 2391
Overall contacts: 40093
Avg. contacts per week: 117.38
Article Citations
Article citations are based on data periodically collected from the Clarivate Web of Science web site
(last update: Feb 2023)
Total number of cites (since 2018): 3
Average cites per year: 0.50
Publication Metrics
by Dimensions ©
Articles citing this article
List of the papers citing this article based on CrossRef Cited-by.
Related Contents
iForest Similar Articles
Research Articles
Assessing the relative role of climate on litterfall in Mediterranean cork oak forests
vol. 11, pp. 786-793 (online: 14 December 2018)
Research Articles
Phenology of the beech forests in the Western Carpathians from MODIS for 2000-2015
vol. 10, pp. 537-546 (online: 05 May 2017)
Research Articles
Successional leaf traits of monsoon evergreen broad-leaved forest, Southwest China
vol. 10, pp. 391-396 (online: 16 March 2017)
Research Articles
Relationships between leaf physiognomy and sensitivity of photosynthetic processes to freezing for subtropical evergreen woody plants
vol. 12, pp. 551-557 (online: 17 December 2019)
Research Articles
Trends and driving forces of spring phenology of oak and beech stands in the Western Carpathians from MODIS times series 2000-2021
vol. 16, pp. 334-344 (online: 19 November 2023)
Research Articles
A comparative study of growth and leaf trait variation in twenty Cornus wilsoniana W. families in southeastern China
vol. 10, pp. 759-765 (online: 02 September 2017)
Research Articles
Salinity strongly drives the survival, growth, leaf demography, and nutrient partitioning in seedlings of Xylocarpus granatum J. König
vol. 10, pp. 851-856 (online: 26 October 2017)
Research Articles
Effect of environmental gradients on leaf morphological traits in the Fandoghlo forest region (NW Iran)
vol. 13, pp. 523-530 (online: 13 November 2020)
Research Articles
Leaf morphology of progenies in Q. suber, Q. ilex, and their hybrids using multivariate and geometric morphometric analysis
vol. 11, pp. 90-98 (online: 31 January 2018)
Research Articles
A comparative study of four approaches to assess phenology of Populus in a short-rotation coppice culture
vol. 9, pp. 682-689 (online: 12 May 2016)
iForest Database Search
Search By Author
Search By Keyword
Google Scholar Search
Citing Articles
Search By Author
Search By Keywords
PubMed Search
Search By Author
Search By Keyword