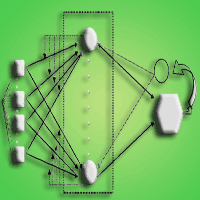
The use of tree crown variables in over-bark diameter and volume prediction models
iForest - Biogeosciences and Forestry, Volume 7, Issue 3, Pages 132-139 (2014)
doi: https://doi.org/10.3832/ifor0878-007
Published: Jan 13, 2014 - Copyright © 2014 SISEF
Research Articles
Abstract
Linear and nonlinear crown variable functions for 173 Brutian pine (Pinus brutia Ten.) trees were incorporated into a well-known compatible volume and taper equation to evaluate their effect in model prediction accuracy. In addition, the same crown variables were also incorporated into three neural network (NN) types (Back-Propagation, Levenberg-Marquardt and Generalized Regression Neural Networks) to investigate their applicability in over-bark diameter and stem volume predictions. The inclusion of crown ratio and crown ratio with crown length variables resulted in a significant reduction of model sum of squared error, for all models. The incorporation of the crown variables to these models significantly improved model performance. According to results, non-linear regression models were less accurate than the three types of neural network models tested for both over-bark diameter and stem volume predictions in terms of standard error of the estimate and fit index. Specifically, the generated Levenberg-Marquardt Neural Network models outperformed the other models in terms of prediction accuracy. Therefore, this type of neural network model is worth consideration in over-bark diameter and volume prediction modeling, which are some of the most challenging tasks in forest resources management.
Keywords
Crown Variables, Taper, Back-Propagation ANNs, Levenberg-Marquardt ANNs, Generalized Regression Neural Networks
Introduction
Crown characteristics are an important component of growth and yield models. Tree crown research contributes to several key forest ecosystem attributes: biodiversity, productivity, forest management, forest environment, and wildlife ([1]). The crown of a tree has a strong influence on stem shape, as foliage provides carbohydrates for tree growth and development of the whole tree and their vertical distribution influences stem shape ([21], [23]). Crown ratio (CR) is considered as an expression of the tree’s photosynthetic potential, and therefore commonly included as a key variable in growth and yield models.
Tree stem shape has been commonly modeled using taper models ([26]). Taper models are used to estimate diameter along the bole at any given height, so tree volume can then be determined based on these diameters and corresponding heights. The auxiliary variables used to increase the accuracy of existing taper equations include: (1) crown dimensions; (2) stand and site variables; and (3) upper stem diameter measurements ([38]). Larson ([18]) reported that within the crown, stem diameters at particular heights are generally smaller when compared with trees of the same dimensions but shorter crowns. As a result, tree boles cannot be completely described as a function of bole length and diameter. In attempts to describe tree taper, numerous models of varying complexity have been advanced. In most mathematical models, taper is modeled in terms of dbh (diameter at breast height) and total height. A few researchers have considered using crown variables (e.g., crown length - CL, CR, and crown height - CH) as covariates ([28], [21], [15]) for describing tree profiles because of the relationship between crown and stem form development, but previous studies have shown mixed results on the benefit of adding crown variables in taper models. The main crown variable utilized in taper and volume models was CR ([32], [15], [23], [13]). However, CL is an interesting variable, which may influence the prediction of diameter and volume in combination with CR ([27]). For this reason, some forms of CR and CL functions were incorporated into the tree-stem taper and volume prediction models in this study.
For stem taper and volume predictions using regression analysis, an appropriate nonlinear function must first be identified, which is a very difficult task. The main reason that artificial neural network (ANN) applications have received attention is that the methodology is comparable to statistical modeling and ANNs can be seen as a complementary effort (without the restrictive assumption of a particular statistical model) or as an alternative approach to fitting nonlinear models to data. Due to the fact that Neural Networks (NNs) attempt to find the best nonlinear function based on the network’s complexity, without the constraint of pre-specified nonlinearity, we investigated their applicability in over-bark diameter and stem volume predictions using the same crown variables previously described.
ANNs have been successfully applied in the field of forest modeling. Among others, ANNs have been used for: (a) prediction of diameter distribution ([19]); (b) forest attributes prediction ([7]); (c) bark volume prediction for standing trees ([8]); and (d) prediction of inside-bark diameter and heartwood diameter ([20]). While ANNs have been applied to the prediction of tree volume ([29]), inclusion of crown variables into ANN models has not been reported.
The objective of this study was to investigate the level of improvement in diameter over bark (dob) and stem volume predictions through the incorporation of crown variables. An additional purpose of this study was to test the performance of different ANNs that can be employed for diameter and volume predictions through the incorporation of crown variables. For this purpose, a modified segmented polynomial taper equation ([6]) and ANN models were utilized with Brutian pine (Pinus brutia Ten.) data collected from southern Turkey.
Material and methods
Data
One hundred seventy three sample trees of Brutian pine were selected from natural even-aged managed stands in Bucak Forest Enterprise, southern Turkey, on lands owned by the Forest Service. Trees were felled through the clear-cutting of areas of the Bucak Forest Enterprise and were systematically sampled to cover the range of diameters within a stand, with emphasis on dominant and codominant individuals. Trees possessing multiple stems, broken tops, obvious cankers or crooked boles were not included in the sample. Total height was measured to the nearest 0.05 m. Diameter over-bark (D) at breast height (1.3 m) was measured and recorded to the nearest 0.1 cm using digital calipers. Diameter over-bark was measured at 0.3, 1.3, 2.3 m and then at intervals of 1 m along the remainder of the stem. In each section, two perpendicular diameters over-bark were measured and then arithmetically averaged. The height to base of the live crown was determined by identifying that point along the bole where the lowest live branch or branch whorl was attached to the main bole as indicated by Jiang et al. ([15]). Finally, CL and CR were derived from crown measurements. Crown ratio was defined as the ratio between length of the crown and total tree height. Actual volumes and sectional volumes in cubic meters were calculated using the overlapping bolts method as described by Bailey ([2]).
A scatter plot of relative diameter against relative height was examined visually to detect possible anomalies in data. Extreme data points were observed; therefore the systematic approach proposed by Bi ([4]) for detecting abnormal data points was applied to increase the efficiency of the process. For this reason, a nonparametric taper curve was fitted by local regression, using the LOESS procedure. This involved local quadratic fitting with a smoothing parameter of 0.25 for the dataset, which was selected after iterative fitting and visual examination of the smoothed taper curves overlaid on the data. The number of extreme values accounted for less than 0.2% for dataset. The plot of relative height against relative diameter used in this study, together with the LOESS regression line, is shown in Fig. 1.
Fig. 1 - Plot of relative height (h/H) vs. relative diameter (dob/D) over-bark for the Brutian pine trees studied.
All trees with total height less than 5.3 m were eliminated, as they could not be used to fit the modified Clark et al. ([6]) model. Sample trees were selected to adequately represent the distribution of these trees in the population in terms of their respective diameter and height classes. Approximately 25% of sample trees were selected at random and used as the validation data set, while the rest was used for model fitting. Summary statistics for both data sets are shown in Tab. 1.
Tab. 1 - Summary of Brutian pine tree attributes for model fitting and for the validation data. (SD): Standard deviation.
Data set | Parameter | Unit | Mean | SD | Min | Max |
---|---|---|---|---|---|---|
Fitting data (measurements from 131 trees, n = 2173) |
Over-bark dbh (D) | cm | 39.85 | 12.16 | 9 | 64 |
Total height (H) | m | 18.77 | 3.9 | 8.8 | 26.8 | |
Disk diameter (dob) | cm | 26.34 | 13.27 | 2 | 73 | |
Disk height (h) | m | 8.63 | 5.57 | 0.3 | 24.3 | |
Stem volume (V) | m3 | 1.06 | 0.77 | 0.02 | 3.31 | |
Validation data (measurements from 42 trees, n = 729 ) |
Over-bark dbh (D) | cm | 42.39 | 13.8 | 11 | 72 |
Total height (H) | m | 19.35 | 4.13 | 9.5 | 26.6 | |
Disk diameter (dob) | cm | 27.72 | 14.13 | 2 | 80 | |
Disk height (h) | m | 8.95 | 5.72 | 0.3 | 24.3 | |
Stem volume (V) | m3 | 1.23 | 0.98 | 0.03 | 4.14 |
Taper and volume equations
The modified form of the segmented polynomial model published by Clark et al. ([6]) was used for this study, taking into account the conclusions of the work by Jiang et al. ([14]). As noted by Jiang et al. ([14]) segmented polynomial models appear to be more accurate than other model formulations for estimating diameter, height, and volume.
Two crown variables, CL and CR were included into the best fitted model identified for Brutian pine. Leites & Robinson ([21]) incorporated both linear and nonlinear CR and CL functions. The forms of CR and CL functions used in this study include (eqn. 1 to 5):
where λi are the parameters to be estimated from data. eqn. 1 through 5 were incorporated into the existing taper and volume models ([14], [15]) for parameters b1, b2, b3, and b4 to ascertain the effects of incorporating crown variables into the existing model forms for Brutian pine. The parameters (b1, b2, b3 and b4) in the taper and volume equations were replaced with all combinations of CR, CL and CR with CL functions and were evaluated for model improvement.
In addition to the evaluation of the entire stem, model performance was examined using sectional relative height classes from 10% to 90% of total height. For the taper and volume model forms used in this study, the upper stem diameter at 5.30 m is a required input variable. Diameters at 5.30 m were obtained through actual field measurements.
Neural network models
Known advantages of ANNs over traditional approaches ([3], [37], [12], [9]) accelerated their use in this research as an alternative approach to regression analysis for fitting nonlinear data. Due to their advantages and limitations ([3], [9]), two different NN architectures (Fig. 2) were used: (a) the multilayer-perceptron (MLP); and (b) the generalized regression neural network (GRNN).
Fig. 2 - (a) The multilayer-perceptron (MLP) and (b) the generalized regression neural network (GRNN) architectures.
Furthermore, in the multilayer-perceptron learning step two different optimization algorithms were used: (1) the back-propagation (BP), which produces the back-propagation artificial neural network (BPANN) models ([33], [10], [11], [31]); and (2) the Levenberg-Marquardt (LM) algorithm which produces the Levenberg-Marquardt artificial neural network (LMANN) models ([22], [25]). The latter algorithm was presented as an intermediate optimization technique between Gauss-Newton and gradient descent methods in order to address the limitations of each of these algorithm.
The generalized regression neural network (GRNN) is one type of NN which was devised by Speckt ([36]). This regression network architecture uses Bayesian techniques to estimate the expected mean value of the output variable.
Appropriate input variables to the NN models can be selected in advance based on a priori knowledge of the physical problem, an approach that is commonly utilized in the field of geotechnical engineering ([24]). In order for the generated NN models to be comparable to the modified form-class segmented taper equations of Clark et al. ([6]), the developed neural network models (BPANN, LMANN and GRNN models) used diameter at breast height over-bark (D), total height of the tree (H), the stem height above the ground to the measurement point (h), the diameter over-bark at 5.3 m above the ground (F) as input variables, and tree diameter (dob) over-bark at any given height (h) as the output variable. Moreover, in order to investigate the level of improvement in dob and stem volume predictions, the additional crown variables, CL and CR, were incorporated as input variables to the NN models.
For the development of the BPANN models, the effectiveness and convergence of training depends significantly on the values of learning rate (LR) and momentum factor (M). The numbers of neurons in the hidden layer of the ANNs were finalized after a trial and error procedure using different combinations of learning rates and momentum factors. Each combination of LR and M was tested for different numbers of hidden neurons. For the LMANN models development, the effectiveness and convergence of training depends significantly on the adjustment of the damping factor (μ
). This was achieved by starting with the value of 0.1 and then using an adjustment factor (v
), which when multiplied by μ
provides an increment or when μ
is divided by v
provides a decrement, until the lowest sum of square errors value was obtained. For the GRNN models, the network was trained for smoothing coefficient values (σ
) equal to 0.001, 0.002, …, 1.0, …, 2.0. The best combinations of all parameters that provide for the best learning of the BPANN, LMANN, and GRNN models are given in Tab. 2.
Tab. 2 - The best combinations of all parameters that conduct to the best learning of the BPANN, LMANN and GRNN models for the prediction of the diameter over-bark (dob) to a measurement point, and the prediction of the stem volume (V) over-bark. (OM): the model without incorporation of the crown variables; (MCR): the model with the CR variable inclusion; (MCRCL): the model with CR and CL variables inclusion; (D): diameter at breast height over-bark (cm); (dob): diameter over-bark (cm) to measurement point at height h; (H): total tree height (m): (h): height above the ground to the measurement point (m); (F): diameter over-bark (cm) at 5.3 m above ground; (V): stem volume over-bark from stump (m3); (CL): crown length; (CR): crown ratio.
BPANN models that resulted to the best learning | ||||||
---|---|---|---|---|---|---|
Model | Number of nodes | Number of Epochs | Learning rate | Momentum factor |
||
Input layer | Hidden layer | Output layer | ||||
OM | 4 : (D, H, h, F) | 8 | 1 : (dob) | 1000 | 0.10 | 0.30 |
MCR | 5 : (D, H, h, F, CR) | 10 | 1 : (dob) | 1000 | 0.10 | 0.30 |
MCRCL | 6 : (D, H, h, F, CR, CL) | 13 | 1 : (dob) | 1000 | 0.10 | 0.30 |
OM | 5 : (D, H, dob, h, F) | 6 | 1 : (V) | 1000 | 0.09 | 0.20 |
MCR | 6 : (D, H, dob, h, F, CR) | 10 | 1 : (V) | 1000 | 0.07 | 0.30 |
MCRCL | 7 : (D, H, dob, h, F, CR, CL) | 11 | 1 : (V) | 1000 | 0.05 | 0.30 |
LMANN models that resulted to the best learning | ||||||
Model | Number of nodes | Number of Epochs |
Initial (μ) value |
Adjustment factor (v) |
||
Input layer | Hidden layer | Output layer | ||||
OM | 4 : (D, H, h, F) | 8 | 1 : (dob) | 3000 | 0.1 | 10 |
MCR | 5 : (D, H, h, F, CR) | 10 | 1 : (dob) | 1000 | 0.1 | 10 |
MCRCL | 6 : (D, H, h, F, CR, CL) | 13 | 1 : (dob) | 1000 | 0.1 | 10 |
OM | 5 : (D, H, dob, h, F) | 6 | 1 : (V) | 2000 | 0.1 | 10 |
MCR | 6 : (D, H, dob, h, F, CR) | 10 | 1 : (V) | 2000 | 0.1 | 10 |
MCRCL | 7 : (D, H, dob, h, F, CR, CL) | 11 | 1 : (V) | 2000 | 0.1 | 10 |
GRNN models that resulted to the best learning | ||||||
Model | Number of nodes | Smoothing coefficient (σ) |
||||
Input layer | 1st Hidden layer | 2nd Hidden layer | Output layer | |||
OM | 4 : (D, H, h, F) | 1956 | 2 | 1 : (dob) | 0.041 | |
MCR | 5 : (D, H, h, F, CR) | 1956 | 2 | 1 : (dob) | 0.049 | |
MCRCL | 6 : (D, H, h, F, CR, CL) | 1956 | 2 | 1 : (dob) | 0.049 | |
OM | 5 : (D, H, dob, h, F) | 1956 | 2 | 1 : (V) | 0.041 | |
MCR | 6 : (D, H, dob, h, F, CR) | 1956 | 2 | 1 : (V) | 0.041 | |
MCRCL | 7 : (D, H, dob, h, F, CR, CL) | 1956 | 2 | 1 : (V) | 0.039 |
Criteria of model evaluation
The statistics used to compare the models included the average bias (B
), the standard error of the estimate (SEE
), the mean absolute error (MAE
), and a fit index (FI
). These evaluation statistics are defined as follows ([35] - eqn. 6 to 9):
where Y
i is the observed value for the i-th observation, Ŷ
i is the predicted value for the i-th observation, ‾Yi
is the mean of the Y
i, df
are the degrees of freedom of the model, n
is the number of observations in the dataset, and SEE
is the standard error of the estimate.
To concurrently minimize taper and volume errors, both equations were fitted simultaneously using SAS PROC MODEL ([34]). All parameters were shared by both the taper and volume equations. Correlated error structure in the data was not taken into account in the SAS MODEL procedure. Prediction accuracy is little affected by the correlated error structure, even when the correlated errors structure is accounted for in the equation fitting process ([17]). The effects of autocorrelation were ignored.
Results
Approximation through taper and volume equations system
Crown ratio (CR) and crown length (CL) were incorporated into the existing taper and volume equations. The parameters (b
1, b
2, b
3 and b
4) for the existing taper and volume equations ([6], [14]) were replaced utilizing both the linear and non-linear functions that incorporate CR, CL, and both crown variables together (eqns. 1 - 5). The full model failed to converge when employing either the linear (eqns. 1 - 3) or nonlinear form of the crown variables (eqns. 4 - 5). All combinations of CR, CL and CR with CL functions with each parameter were tested, but only the replacement of b
4 with the linear eqn. 1 and eqn. 3 resulted in significant parameter estimates (P<0.0001). Parameters estimates for over-bark (dob) taper and volume equations ([6], [14]) with and without the linear CR and linear CR with CL are listed in Tab. 3.
Tab. 3 - Parameter estimates for the compatible taper and volume equations based on the model fitting data. (OM): the original model forms ([6]); (MCR): the original model form with CR; (MCRCL): the original model form with CR and CL.
Model | b 1 |
b 2 |
b 3 |
b 4 |
λ 1 |
λ 2 |
λ 3 |
---|---|---|---|---|---|---|---|
OM | 85.9076 | 6.7407 | 0.6977 | 2.3021 | - | - | - |
MCR | 84.8311 | 6.7349 | 0.6973 | - | 2.9278 | -1.4063 | - |
MCRCL | 85.90755 | 6.7201 | 0.7034 | - | 2.913 | 0.0988 | -3.3829 |
Model OM represents the original model forms without the addition of the crown variable functions, while models MCR and MCRCL represent the modified model after incorporating eqn. 1 and eqn. 3 for the b
4 parameter, respectively. Based on the fit statistics for the OM, MCR, and MCRCL model forms (Tab. 4), inclusion of the linear CR (eqn. 1) and linear CR with CL (eqn. 3) functions improved the fit for over-bark taper equations for Brutian pine.
Tab. 4 - Stem fit statistics for the compatible volume and taper equation systems for Brutian pine based on the model fitting data. (OM): the original model forms ([6]); (MCR): the original model form with CR; (MCRCL): the original model form with CR and CL.
Model | Taper (cm) | Volume (m3) | ||||||
---|---|---|---|---|---|---|---|---|
Bias | SEE | MAE | FI | Bias | SEE | MAE | FI | |
OM | 0.045 | 1.7535 | 1.1907 | 0.9825 | 0.001 | 0.0072 | 0.0043 | 0.9846 |
MCR | 0.0163 | 1.7328 | 1.1815 | 0.983 | 0.0009 | 0.0071 | 0.0043 | 0.9848 |
MCRCL | 0.0872 | 1.696 | 1.1602 | 0.9837 | 0.0009 | 0.007 | 0.0042 | 0.985 |
For dob prediction, the inclusion of crown variables (both CR and CR with CL) had a positive effect for all fit statistics except average bias (Tab. 4) when using the actual upper diameter measurement at 5.30 m. A lower bias does not guarantee good model performance, since large positive and negative values may algebraically counterbalance. Since it indicates the spread of the biases (residuals), the overall standard error of estimate is a better single indicator of goodness of fit ([16]). The additions of linear functions of CR and CR with CL improved model performance by reducing SEE by 1.18-3.28 % and 1.39-2.78 % for diameter and volume estimation, respectively.
For stem volume prediction, significant improvements were observed in the modified Clark et al. ([6]) equation using actual upper stem diameter measurements due to the inclusion of only CR and CR with CL, for the species studied. For the volume function, the MCRCL model performed slightly better than the MCR model (Tab. 4).
Approximation through neural network models
The BPANN, LMANN and GRNN model fit statistics for dob and cubic meter volume prediction, with and without the crown variables, are provided in Tab. 5.
Tab. 5 - Fit statistics for the BPANN, LMANN and GRNN models for diameter over-bark (dob) to a measurement point and the prediction of stem volume (V) over-bark, based on model fitting data (n = 2173). (OM): the model without incorporation of the crown variables; (MCR): the model with the CR variable inclusion; (MCRCL): the model with CR and CL variables inclusion.
Model | dob (cm) | V (m3) | ||||||
---|---|---|---|---|---|---|---|---|
Bias | SEE | MAE | FI | Bias | SEE | MAE | FI | |
BPANN_OM | -0.0089 | 1.588 | 1.1418 | 0.9857 | 0.00079 | 0.00279 | 0.002 | 0.9977 |
BPANN_MCR | -0.0072 | 1.534 | 1.1252 | 0.9865 | 0.00073 | 0.00259 | 0.0018 | 0.998 |
BPANN_MCRCL | -0.005 | 1.5045 | 1.0867 | 0.9871 | -0.00015 | 0.00247 | 0.0017 | 0.9981 |
LMANN_OM | -0.0154 | 1.5196 | 1.0859 | 0.9869 | -1.0·10-6 | 0.00227 | 0.0016 | 0.9985 |
LMANN_MCR | -0.0184 | 1.496 | 1.08 | 0.9872 | 1.5·10-7 | 0.00212 | 0.0015 | 0.9987 |
LMANN_MCRCL | 0.0025 | 1.444 | 1.0336 | 0.9881 | -4.8·10-6 | 0.00204 | 0.0014 | 0.9988 |
GRNN_OM | -0.0221 | 1.5254 | 1.0712 | 0.9868 | 5.7·10-5 | 0.0028 | 0.0018 | 0.9977 |
GRNN_MCR | -0.0269 | 1.496 | 1.012 | 0.9873 | 7.5·10-5 | 0.00268 | 0.0015 | 0.9979 |
GRNN_MCRCL | -0.0211 | 1.4661 | 0.9833 | 0.9878 | 8.7·10-5 | 0.0026 | 0.0014 | 0.9979 |
Results indicate that the inclusion of both crown variables (CR and CL) had positive effects in dob and volume predictions, since their use reduced the standard error of estimate, the bias and the mean absolute errors, and increased the values of the fit index for all types of NN. According to the fitness capability of the different types of NN models used, the Levenberg-Marquardt models of Tab. 2 gave the most accurate results (Tab. 5). Specifically, with regards to the dob predictions, the inclusion of the CR and CL crown variables into the LMANN model resulted in the reduction of SEE values by 4.02% and 1.51%, according to the BPANN_MCRCL and GRNN_MCRCL models, respectively. Further, reductions of the SEE values were observed for volume predictions, as well. Namely, SEE values were reduced by 17.41% and 21.54% according to the BPANN_MCRCL and GRNN_MCRCL models, respectively (Tab. 5). In order to validate the fitted NN models, the same fit statistics (B, SEE, MAE
and FI
) were calculated for the predictions of dob and cubic meter volume based on the validation data set (Tab. 6).
Tab. 6 - Fit statistics for the compatible volume and taper equation systems and for the BPANN, LMANN and GRNN models for diameter over-bark (dob) to a measurement point and the prediction of stem volume (V) over-bark, based on model validation data (n = 729). (OM): the model without incorporation of the crown variables; (MCR): the model with the CR variable inclusion; (MCRCL): the model with CR and CL variables inclusion.
Model | dob (cm) | V (m3) | ||||||
---|---|---|---|---|---|---|---|---|
Bias | SEE | MAE | FI | Bias | SEE | MAE | FI | |
OM | 0.0751 | 1.9673 | 1.3238 | 0.9806 | 0.0006 | 0.0075 | 0.0048 | 0.9883 |
MCR | 0.0686 | 1.9236 | 1.2885 | 0.9813 | 0.0007 | 0.0071 | 0.0046 | 0.989 |
MCRCL | 0.0841 | 1.9635 | 1.3307 | 0.9814 | 0.0004 | 0.0076 | 0.005 | 0.989 |
BPANN_OM | 0.0935 | 1.871 | 1.3066 | 0.9824 | 0.0043 | 0.0055 | 0.0044 | 0.9933 |
BPANN_MCR | 0.0707 | 1.863 | 1.3291 | 0.9826 | 0.0043 | 0.0054 | 0.0043 | 0.9936 |
BPANN_MCRCL | -0.0178 | 1.857 | 1.3188 | 0.9827 | 0.0038 | 0.005 | 0.0038 | 0.9945 |
LMANN_OM | 0.0788 | 1.8093 | 1.2721 | 0.9836 | 0.0035 | 0.0047 | 0.0036 | 0.995 |
LMANN_MCR | -0.0787 | 1.7908 | 1.2976 | 0.9839 | 0.0035 | 0.0046 | 0.0036 | 0.9952 |
LMANN_MCRCL | 0.0552 | 1.7873 | 1.2717 | 0.984 | 0.0033 | 0.0044 | 0.0034 | 0.9956 |
GRNN_OM | 0.5644 | 2.7244 | 1.9538 | 0.9628 | 0.0032 | 0.0058 | 0.0041 | 0.9926 |
GRNN_MCR | 0.4131 | 2.7221 | 2.0848 | 0.9628 | 0.0026 | 0.0056 | 0.0034 | 0.9931 |
GRNN_MCRCL | 0.3122 | 2.7211 | 2.113 | 0.9629 | 0.002 | 0.0054 | 0.0034 | 0.9934 |
Similarly to what noted with the model fitting data, the inclusion of both crown variables as input variables resulted in the most accurate dob and cubic meter volume predictions for all the NN models tested (Tab. 6). Further, the LMANN_MCRCL model showed consistent performance, with better dob and volume predictions than the predictions obtained from the BPANN and GRNN models based on the validation data set.
The SEE for predicting dob and cubic meter volume for the 10 relative height classes for both the compatible taper and volume equation system and for the LMANN models are shown in Fig. 3.
Fig. 3 - The standard errors of estimate (SEE) for estimating diameter over-bark for the taper compatible volume system (a) and for the Levenberg-Marquardt models (c) and volume over-bark along the stem for the taper compatible volume system (b) and for the Levenberg-Marquardt models (d), by relative height classes, using the fitting data.
As seen in Fig. 3a and Fig. 3c, the dob SEE values are significantly lower for the LMANN_MCRCL model across all relative height class except for 10-50% of relative height, where SEE values are slightly lower than the corresponding values of the OM taper model. For volume estimations, SEE values show the same pattern as the dob errors and are clearly lower for the LMANN models across all relative height classes than the corresponding values of the nonlinear regression models (Fig. 3b and Fig. 3d).
Validation results for all the models are shown in Fig. 4. The models were found to generalize for data that was not used in the fitting process and showed consistent performance for both dob and volume prediction.
Fig. 4 - The standard errors of estimate (SEE) and fit indexes (FI) for predicting diameter over-bark and volume over-bark along the stem for the taper and compatible volume system (OM) and for the back-propagation (BPANN_OM, BPANN_MCR and BPANN_MCRCL), Levenberg-Marquardt (LMANN_OM, LMANN_MCR and LMANN_MCRCL), and the generalized regression (GRNN_OM, GRNN_MCR and GRNN_MCRCL) models, using the validation data set.
The results obtained for the validation data set for all models were in agreement with their performance for the model fitting data set (Tab. 4, Tab. 5 and Tab. 6).
Discussion
One of the underlying goals for efficient timber resources management is that of optimizing the prediction accuracy of constructed forest-data models. The performance of nonlinear regression models and NN models (BPANN, LMANN and GRNN models) were compared for the estimation of diameter over-bark and cubic meter volume based on Brutian pine data from southeast Turkey.
The incorporation of crown variables to regression and NN modeling procedures showed improvements in the accuracy for diameter and stem volume predictions in Brutian pine. Slightly better results were obtained for estimating stem form than stem volume, when employing taper and volume equations. As indicated by Weiskittel et al. ([40]), crown variables often explain very little of the variation in stem volume. The level of improvement is likely a function of the natural variation in crown variables. Evaluation of sectional performance showed an improvement in stem form predictions for relative height classes over 50% of total height. The relative size of these improvements was similar to those found by Burkhart & Walton ([5]) and Valenti & Cao ([39]). As Jiang et al. ([15]) pointed out, this is not a surprising result given the fact that only the b
4 parameter was changed.
For environmental issues, such as forest modeling where the complexity of the natural problem is faced, it is very difficult to suggest a specific approach for a given problem. As pointed out by Özçelik et al. ([29]), convenience and economics play the most important role when choosing the method to be used for forest inventory. The determination of the proper approach should take into account both the advantages and limitations of each method. Because of the ability for NNs to automatically fit complex nonlinear models when the complexity of the problem cannot be fully examined, or when the prediction accuracy is the most important element in a survey, NN models appear to be the best option. Our findings are consistent with previous studies by Diamantopoulou ([8]), Pao ([30]), Özçelik et al. ([29]), Leite et al. ([20]), where ANN models generated a better fit when compared to regression models.
In practical forestry, the application of NN models by practitioners can be achieved through the use of trained models that have been constructed by experts for this purpose. However, their use by practitioners requires computational skills but not a priori analytical knowledge when the constructed NN models are being provided. Under the above limitation, NN models are accurate and easy to apply. On the other hand, although regression analysis is based on rules or equations that must be explicitly programmed, nonlinear regression models give reasonable and accurate results. Furthermore, regression models are a tested methodology that provide acceptable results which can easily be applied, and thus worth considering as a solution to a given estimation problem. In this case there is a trade-off between selecting a model which is quite simple to understand and easy to apply (regression model), and one which is more accurate but more difficult to comprehend (NN model).
Conclusions
Accurate estimation of over-bark diameter and stem volume is crucial for the efficient management of forest resources. The inclusion of linear CR and linear CR with CL functions in existing segmented taper and cubic meter volume equations for Brutian pine in Turkey resulted in significant reduction of model sum of squared error. Prediction improvements for upper stem diameter and volume were greater for model forms with CR and CL than model forms with CR alone, though overall improvements were small. Similar results were obtained using the back-propagation, Levenberg-Marquardt and generalized regression neural network models. The incorporation of the crown variables to these models also exhibited improved performance.
Our results indicate that the nonlinear regression model had larger SEE and smaller FI values than the three types of NN models tested, when evaluating both dob and volume predictions (Tab. 4 and Tab. 5). Moreover, the performance of the fitted Levenberg-Marquardt artificial neural network models, where both crown variables were embedded, provided superior performance when compared to nonlinear regression, BPANN and GRNN models for both the fitting and the validation data sets (Tab. 4, Tab. 5 and Tab. 6).
Implementation of the NN approaches does offer a number of advantages over the more traditional regression method of forest-data modeling and should be viewed as a useful alternative to this technique ([29]). The major advantage of NNs for over-bark diameter and stem volume modeling is that when they are used, the underlying relationships between the input and output variables are automatically assimilated into the connection weights of the network. Therefore, they are able to fit complex nonlinear models not specified in advance, unlike other nonlinear modeling techniques such as regression analysis. In spite of NN’s advantages over regression modeling techniques, the discussed accuracy-convenience trade-offs have to be seriously considered in order to determine the best method to apply. When prediction accuracy is the most important element in a survey, then NN models seem to be the best option. However, when convenience is the limiting factor, or if additional accuracy is not most important, nonlinear models can be utilized.
Funding
This study was supported by the Scientific and Technological Research Council of Turkey (TUBITAK-BIDEB).
References
Gscholar
Gscholar
Gscholar
Gscholar
Gscholar
Gscholar
Gscholar
Gscholar
Gscholar
Gscholar
Gscholar
Gscholar
Authors’ Info
Authors’ Affiliation
Faculty of Forestry, Süleyman Demirel University, East Campus, TR-32260, Isparta (Turkey)
Faculty of Forestry and Natural Environment, Aristotle University of Thessaloniki, GR-54124 Thessaloniki (Greece)
Division of Forestry and Natural Resources, West Virginia University, 322 Percival Hall, 26506-6125 Morgantown (WV - USA)
Corresponding author
Paper Info
Citation
Özçelik R, Diamantopoulou MJ, Brooks JR (2014). The use of tree crown variables in over-bark diameter and volume prediction models. iForest 7: 132-139. - doi: 10.3832/ifor0878-007
Academic Editor
Luca Salvati
Paper history
Received: Nov 12, 2012
Accepted: Aug 08, 2013
First online: Jan 13, 2014
Publication Date: Jun 02, 2014
Publication Time: 5.27 months
Copyright Information
© SISEF - The Italian Society of Silviculture and Forest Ecology 2014
Open Access
This article is distributed under the terms of the Creative Commons Attribution-Non Commercial 4.0 International (https://creativecommons.org/licenses/by-nc/4.0/), which permits unrestricted use, distribution, and reproduction in any medium, provided you give appropriate credit to the original author(s) and the source, provide a link to the Creative Commons license, and indicate if changes were made.
Web Metrics
Breakdown by View Type
Article Usage
Total Article Views: 54151
(from publication date up to now)
Breakdown by View Type
HTML Page Views: 45107
Abstract Page Views: 2789
PDF Downloads: 4795
Citation/Reference Downloads: 22
XML Downloads: 1438
Web Metrics
Days since publication: 4168
Overall contacts: 54151
Avg. contacts per week: 90.94
Article Citations
Article citations are based on data periodically collected from the Clarivate Web of Science web site
(last update: Mar 2025)
Total number of cites (since 2014): 29
Average cites per year: 2.42
Publication Metrics
by Dimensions ©
Articles citing this article
List of the papers citing this article based on CrossRef Cited-by.
Related Contents
iForest Similar Articles
Research Articles
Total tree height predictions via parametric and artificial neural network modeling approaches
vol. 15, pp. 95-105 (online: 21 March 2022)
Research Articles
Analyzing regression models and multi-layer artificial neural network models for estimating taper and tree volume in Crimean pine forests
vol. 17, pp. 36-44 (online: 28 February 2024)
Research Articles
Exploring machine learning modeling approaches for biomass and carbon dioxide weight estimation in Lebanon cedar trees
vol. 17, pp. 19-28 (online: 12 February 2024)
Research Articles
Artificial intelligence associated with satellite data in predicting energy potential in the Brazilian savanna woodland area
vol. 13, pp. 48-55 (online: 05 February 2020)
Research Articles
Analysis of factors influencing deployment of fire suppression resources in Spain using artificial neural networks
vol. 9, pp. 138-145 (online: 19 July 2015)
Research Articles
Estimation of stand crown cover using a generalized crown diameter model: application for the analysis of Portuguese cork oak stands stocking evolution
vol. 9, pp. 437-444 (online: 02 December 2015)
Research Articles
Comparative analysis of taper models for Pinus nigra Arn. using terrestrial laser scanner acquired data
vol. 17, pp. 203-212 (online: 22 July 2024)
Research Articles
Identification of wood from the Amazon by characteristics of Haralick and Neural Network: image segmentation and polishing of the surface
vol. 15, pp. 234-239 (online: 14 July 2022)
Research Articles
Modelling taper and stem volume considering stand density in Eucalyptus grandis and Eucalyptus dunnii
vol. 14, pp. 127-136 (online: 16 March 2021)
Research Articles
Compatible taper-volume models of Quercus variabilis Blume forests in north China
vol. 10, pp. 567-575 (online: 08 May 2017)
iForest Database Search
Search By Author
Search By Keyword
Google Scholar Search
Citing Articles
Search By Author
Search By Keywords
PubMed Search
Search By Author
Search By Keyword