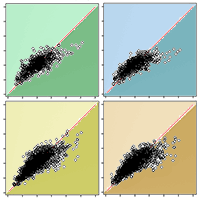
Prediction of stem diameter and biomass at individual tree crown level with advanced machine learning techniques
Salim Malek (1-3), Franco Miglietta (1), Terje Gobakken (2), Erik Næsset (2), Damiano Gianelle (3), Michele Dalponte (3)
iForest - Biogeosciences and Forestry, Volume 12, Issue 3, Pages 323-329 (2019)
doi: https://doi.org/10.3832/ifor2980-012
Published: Jun 14, 2019 - Copyright © 2019 SISEF
Research Articles
Abstract
Knowledge about the aboveground biomass (AGB) and the diameters at breast height (DBH) distribution can lead to a precise estimation of carbon density and forest structure which can be very important for ecology studies especially for those concerning climate change. In this study, we propose to predict DBH and AGB of individual trees using tree height (H) and crown diameter (CD), and other metrics extracted from airborne laser scanning (ALS) data as input. In the proposed approach, regression methods, such us support vector machine for regression (SVR) and random forests (RF), were used to find a transformation or a transfer function that links the input parameters (H, CD, and other ALS metrics) with the output (DBH and AGB). The developed approach was tested on two datasets collected in southern Norway comprising 3970 and 9467 recorded trees, respectively. The results demonstrate that the developed approach provides better results compared to a state-of-the-art work (based on a linear model with the standard least-squares method) with RMSE equal to 81.4 kg and 92.0 kg, respectively (compared to 94.2 kg and 110.0 kg) for the prediction of AGB, and 5.16 cm and 4.93 cm, respectively (compared to 5.49 cm and 5.30 cm) for DBH.
Keywords
Aboveground Biomass, Diameter at Breast Height, Airborne Laser Scanning (ALS), Remote Sensing (RS), Support Vector Machine for Regression (SVR), Random Forests (RF)
Authors’ Info
Authors’ address
Franco Miglietta 0000-0003-1474-8143
Institute of Biometeorology, CNR, 50145 Firenze (Italy)
Erik Næsset 0000-0002-2460-5843
Faculty of Environmental Sciences and Natural Resource Management, Norwegian University of Life Sciences, P.O. Box 5003, NO-1432 s (Norway)
Damiano Gianelle 0000-0001-7697-5793
Michele Dalponte 0000-0001-9850-8985
Dept. of Sustainable Agro-ecosystems and Bioresources, Research and Innovation Centre, Fondazione E. Mach, v. E. Mach 1, 38010 San Michele all’Adige, TN (Italy)
Corresponding author
Paper Info
Citation
Malek S, Miglietta F, Gobakken T, Næsset E, Gianelle D, Dalponte M (2019). Prediction of stem diameter and biomass at individual tree crown level with advanced machine learning techniques. iForest 12: 323-329. - doi: 10.3832/ifor2980-012
Academic Editor
Carlotta Ferrara
Paper history
Received: Oct 22, 2018
Accepted: Apr 06, 2019
First online: Jun 14, 2019
Publication Date: Jun 30, 2019
Publication Time: 2.30 months
Copyright Information
© SISEF - The Italian Society of Silviculture and Forest Ecology 2019
Open Access
This article is distributed under the terms of the Creative Commons Attribution-Non Commercial 4.0 International (https://creativecommons.org/licenses/by-nc/4.0/), which permits unrestricted use, distribution, and reproduction in any medium, provided you give appropriate credit to the original author(s) and the source, provide a link to the Creative Commons license, and indicate if changes were made.
Web Metrics
Breakdown by View Type
Article Usage
Total Article Views: 42898
(from publication date up to now)
Breakdown by View Type
HTML Page Views: 35793
Abstract Page Views: 3357
PDF Downloads: 3111
Citation/Reference Downloads: 5
XML Downloads: 632
Web Metrics
Days since publication: 2119
Overall contacts: 42898
Avg. contacts per week: 141.71
Citation Metrics
Article Citations
Article citations are based on data periodically collected from the Clarivate Web of Science web site
(last update: Mar 2025)
Total number of cites (since 2019): 14
Average cites per year: 2.00
Publication Metrics
by Dimensions ©
Articles citing this article
List of the papers citing this article based on CrossRef Cited-by.
References
Improved allometric models to estimate the aboveground biomass of tropical trees. Global Change Biology 20: 3177-3190.
CrossRef | Gscholar
Allometric equations for integrating remote sensing imagery into forest monitoring programmes. Global Change Biology 23: 177-190.
CrossRef | Gscholar
Estimating aboveground carbon density and its uncertainty in Borneo’s structurally complex tropical forests using airborne laser scanning. Biogeosciences 15: 3811-3830.
CrossRef | Gscholar
Biomass functions for pine, spruce and birch in Sweden. Report 45, Department of Forest Survey, Swedish University for Agricultural Sciences, Uppsala, Sweden, pp. 73.
Gscholar
Environmental correlates of tree biomass, basal area, wood specific gravity and stem density gradients in Borneo’s tropical forests. Global Ecology and Biogeography 19: 50-60.
CrossRef | Gscholar