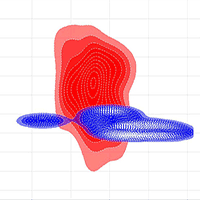
Distribution factors of the epiphytic lichen Lobaria pulmonaria (L.) Hoffm. at local and regional spatial scales in the Caucasus: combining species distribution modelling and ecological niche theory
iForest - Biogeosciences and Forestry, Volume 17, Issue 2, Pages 120-131 (2024)
doi: https://doi.org/10.3832/ifor4406-017
Published: Apr 30, 2024 - Copyright © 2024 SISEF
Research Articles
Abstract
For the rare epiphytic lichen Lobaria pulmonaria (L.) Hoffm., there is a lack of data on ecological niche parameters and distribution factors in the Caucasus, which are necessary to develop an effective system of the species preservation during forest management. The aim of this study was to identify the influence of abiotic, biotic and movement factors on the potential distribution of Lobaria pulmonaria in the Caucasus forests, depending on the spatial scale. We combined species distribution modelling and ecological niche theory based on the BAM (Biotic-Abiotic-Movement) concept. A total of 174 occurrence data were retained in the modelling using Maxent ver. 3.4.3 in R. The distribution models of the main lichen phorophytes in the Caucasus (Pinus sylvestris L. and Fagus orientalis Lipsky) were used as biotic layers in models. The raster of distances from optimal sites, where the probability of the lichen occurrence remained above 0.5, was used as a movement-layer. Different abiotic predictors were significant in the lichen distribution in the Central Caucasus (terrain) and throughout the Caucasus (macroclimate). Interspecific relationships (lichen-phorophyte) were more significant at the local scale. The movement factor contributed most to the local model (80% of the contribution) and limited the lichen distribution to a radius of 20 m in the Central Caucasus and 30 m throughout the Caucasus. Field verification of the local model showed an 85.7% success rate of presence prediction with cutoff values of 0.8. The combination of SDM modelling and ecological niches theory is an effective method for studying the potential localisation and the ecological niches of epiphytic lichens.
Keywords
Lobaria pulmonaria, Caucasus Forest, Species Distribution Modelling, Ecological Niche, Biotic-Abiotic-Movement Concept, Spatial Scale
Introduction
The tree lungwort Lobaria pulmonaria (L.) Hoffm. is a cosmopolitan epiphytic lichen, widespread in old-growth forests of boreal, oceanic, temperate and mountainous regions ([40], [32], [27]). This large foliose cyanolichen serves as a flagship species that draws attention to lichen preservation ([65], [15], [27]). Lobaria pulmonaria is mainly confined to intact forests with long ecological continuity and is considered an old-growth forest indicator ([30], [45], [10], [34], [32]) and an umbrella species ([10], [34], [15], [27]). In old-growth forests, Lobaria pulmonaria colonises the bark of a wide spectrum of phorophytes, mostly deciduous tree ([40], [65], [27]), and Castanea sativa Mill. ([45]). It was also recorded on the bark of Pinus sylvestris L., Picea abies (L.) H. Karst., and Abies nordmanniana (Steven) Spach ([30], [70], [65], [37], [27]).
Many authors have highlighted unsustainable silvicultural practices, habitat fragmentation, air pollution and climate change as major threats to the world population of Lobaria pulmonaria ([65], [46], [15], [27]). This species, sensitive to anthropogenic influence, is endangered in many European countries and is included in national red lists ([45], [32], [27]). It is also red-listed in Russia, including many regions of the Caucasus ([33]). In the Caucasus Lobaria pulmonaria seems to be a rare species that occurs mainly on beech (Fagus orientalis Lipsky), hornbeam (Carpinus betulus L.), linden (Tilia begoniifolia Steven), ash (Fraxinus excelsior L.), oak (Quercus sp.), and maple (Acer sp.) ([70], [25]), rarely on fir (Abies nordmanniana Spach) and pine (Pinus sylvestris L.) ([70], [37]). Although the lungwort lichen distribution patterns are widely studied in European forest landscapes ([15], [27]), they have not been sufficiently known in the Caucasus. The forest cover in the region is steadily decreasing ([67]), and knowledge of the factors limiting the local and regional distribution of Lobaria pulmonaria is therefore essential to develop an effective system of the species preservation during forest management.
The issue can be addressed by species distribution modelling (SDM) combined with ecological niche theory. SDM, based on statistical processing of ground data (e.g., geographic records of a species) using data obtained from environmental models (geographic layers of climatic and topographic information), is considered an effective method of studying the potential distribution of species ([51], [7]). This method identifies the importance of environmental predictors for species distribution by illustrating how the spatial probability of an organism presence depends on variables. Habitats with the highest presence probability are considered most suitable for the target species. Projecting the presence probability over a specific area provides a probability distribution map of species.
Meanwhile, SDM models often consider only abiotic determinants of species distribution and do not include biotic factors (competition, mutualism, predation, etc.), which are known to have a significant influence on species distribution. Accounting for biotic variables in SDM models is possible in the framework of the Biotic-Abiotic-Movement (BAM) concept ([61], [51], [50]). This ecological niche concept considers three groups of factors determining the species distribution, including abiotic predictors (A-factor), biotic determinants (B-factor: interactions between species), and species dispersal capability (M-factor: movement, accessibility of areas - [61], [51]). The BAM concept provides for the possibility of studying the effects of the three factors separately ([61]). The A-model is a geographic expression of the fundamental niche of the species, the BA-model is a geographic expression of the realised niche, and the BAM-model represents an occupied distributional area that is closest to the real species distribution ([61], [51]). The BAM concept assumes a nested (hierarchical) relationship between the fundamental niche, the realised niche and the occupied distributional area, as well as the possibility of integrating “the geography of other species in single-species models” ([61], [50]). Accordingly, a hierarchical modelling approach that represents the hierarchy of the conceptual model is appropriate within this framework. The approach includes means to describe hierarchical relationships between model elements, e.g., by embedding some items within others ([41]). Consequently, we first obtained the A-model of Lobaria pulmonaria distribution based on abiotic variables only. To integrate the biotic factor into the BA-model, we re-run the model with abiotic and biotic factors. We used previously generated probability distribution maps of the phorophytes (in the range from 0 to 1) as separate biotic covariates (biotic layers - [55]). It is known that epiphytic lichens have to follow their phorophytes, and the dynamic of epiphytic lichen populations is significantly determined by the population dynamic of their phorophyte trees ([32]). In the Central Caucasus, the main lungwort lichen phorophyte is Pinus sylvestris, while in the Caucasus region it is Fagus orientalis ([37]). We therefore used distribution models of Pinus sylvestris and Fagus orientalis as biotic layers in the local and regional BA-models of Lobaria pulmonaria. The main assumption of this method is a limited number of modelled predictor species, while the influence of biotic relationships on the spatial distribution of Lobaria pulmonaria may be much more complex.
Movement factor, which determines the dispersal ability of a species, can be formalised through the set of territories accessible to the species ([61], [50]). At present, there is no unified conceptual approach to the spatial specification of movement factor. As reviewed by Barve et al. ([4]), species-accessible areas are typically delineated: (i) within administrative or geographical units with no biologically significant basis; (ii) within biotic areas (regions with a set of species distinct from other regions); and (iii) within territories of historical distribution of species (including those modelled on the characteristics of the species current ecological niches). Accessible areas were also restricted to sites with previously defined climatic classes suitable for the target species ([3]) or regions with fossilised species remains ([44]). To consider the movement factor in the BAM-model, we represented the accessibility of areas through the distance from optimal areas (plots with 0.8 threshold of habitat suitability) at which the probability of species occurrence was above 0.5 ([55]). We rerun the model with abiotic variables, biotic factors, and a raster of distances from optimal sites that were calculated from the BA model. The main assumptions of the approach are: (i) areas with an occurrence probability of Lobaria pulmonaria above 0.8 (the highest predicted probability of the lungwort lichen) are considered most accessible for the species; (ii) areas with an occurrence probability of lungwort lichen above 0.5 are considered accessible; (iii) the dispersal capacity of Lobaria pulmonaria depends on the geographical and ecological accessibility of areas. A somewhat similar approach was applied in the recent study of Soberón & Osorio-Olvera ([62]), who estimated region availability using an adjacency matrix. The adjacency matrix represented all cells, eligible or ineligible, that could be reached from eligible cells at a given dispersal parameter (dispersal capacities - [62]).
The BA- and BAM-models constructed in this way are a type of nested models that allow us to represent the hierarchical organisation of Lobaria pulmonaria ecological niche. Nested models are quite widely used in clinical modelling, but are rarely applied in bioecological modelling ([41]). An example is recent work on incorporating food resource distributions into SDM models of the harpy eagle ([66]). Using a hierarchical modelling approach, the authors included predictions of food resource raster as separate biotic covariates along with abiotic variables in abiotic-biotic models ([66]). Perhaps in the future, nested BAM models, which are an example of effective hierarchical modelling, will be used more frequently in bioecological modelling.
As suggested by some authors ([61], [9], [72], [52], [28]), the importance of abiotic variables and movement factors in species distribution is highest at a regional spatial scale, whereas biotic interactions play a greater role at a local scale. Other authors ([73]) postulated a major role of biotic factors in the species distribution at regional scale. Thus, species distribution patterns established for large territorial units may not be applicable to explain species distributions at local scales, and the influence of spatial scale on the significance of distribution predictors remains debatable. For lichen species, especially in mountainous areas, there is also a lack of data on the significance of environmental variables in different-scale distribution models. It was suggested that the distribution of small sedentary species such as lichens is influenced by climatic factors mainly at a small spatial scale ([31], [24], [20], [15]). Due to its small living space, size and mobility, Lobaria pulmonaria, for example, is sensitive to local high light and temperature stress, which strongly affects the surface temperature of the thallus, enhancing water loss ([15]). In turn, insufficient hydration reduces turgor pressure, hyphae growth, photosynthetic activity and diaspore number of Lobaria pulmonaria ([13], [42], [15]), affecting the reproductive potential and dispersal capability of the species. However, despite the ecological relevance of microclimatic gradients (light levels, humidity, bark pH, etc.), they cannot be mapped at a regional scale to predict species distribution and conservation ([18]). For SDM purposes, large-scale variables that capture the influence of micro-scale predictors are more appropriate. Such variables are easier to record and map, which increases their value for SDM ([18]). At the same time, the relevance of the macroclimatic variables in lichen distribution modelling was challenged because of the unclear relationship of lichen ecological performance to coarse-grained macroclimate ([20]). On the other hand, Eaton & Ellis ([17]) found that macroclimatic variables such as precipitation and mean annual temperature affect the growth of Lobaria pulmonaria. Epiphytic lichens were also shown to prefer regions with more humid climates ([24]). Hence SDM is effective and justified for predicting lichen distribution when macroclimatic data correlate with indicators of the local “ecological success” of species ([18], [17]), and distribution models should be supported by knowledge of the lichen functional ecology ([19]).
The significance of biotic factors may also vary with spatial scale. At the local level, important biotic predictors of Lobaria pulmonaria distribution are pH and bark structure ([13], [42], [34], [32]), trunk diameter ([13], [10], [34]), bryophyte coverage ([5]), phorophyte age and lifespan ([35]). At the landscape level, forest type (species composition), age, area and degree of stand fragmentation are most important for lungwort lichen distribution ([13], [45], [10], [65], [32], [15]). We hypothesized that in the Caucasus, the relevance of the phorophyte distribution factor in SDM models of Lobaria pulmonaria may also vary depending on the spatial scale.
At the regional scale, the study covered the Caucasus, a vast ecoregion (about 390 thousand km2 between 38° to 47° N and 36° to 50° E) with a combination of lowlands, uplands and high mountain ridges. The prevailing mountainous relief determines the distribution of air masses and altitudinal zonality of climate, and, as a consequence, the great diversity of climate and vegetation types in the Caucasus. The ecoregion comprises a variety of natural complexes from semi-deserts and steppes to alpine meadows and permafrost, including a belt of coniferous and deciduous forests. The Central Caucasus, which was our study area at the local scale, on a relatively small territory (about 20.5 thousand km2) includes the main natural complexes characteristic of the entire ecoregion. At the same time, this is the most highland part of the Caucasus (from 200 to 5642 m a.s.l.), which may influence the patterns of L. pulmonaria distribution.
In this study, we aimed to identify the patterns of influence of abiotic, biotic and movement factors on the potential distribution of L. pulmonaria in the Caucasus forests, depending on the spatial scale. We hypothesised that the relative contribution of the different predictors in the lungwort lichen distribution models depends on the scale of the study area. We also wanted to investigate whether species distribution modelling based on the BAM concept is an effective method for studying the ecological niches of epiphytic lichens. Such an understanding is essential as it can be the basis for an effective conservation system not only for L. pulmonaria, but also for the main habitats of the lichen, i.e., old-growth forests.
Materials and methods
Study area
The Caucasus territory is divided into several parts based on orography and climate: the Ciscaucasia, the Greater Caucasus (North Caucasus and Transcaucasia), the Lesser Caucasus, the Colchis and Kura-Araks Lowlands, and the Transcaucasian Highland (Fig. 1). The administrative boundaries of the territory include the Russian Federation, Georgia, Azerbaijan, and Armenia. The prevailing climate of the Ciscaucasia is warm continental or Dfa according to the Köppen-Geiger classification ([55]). In most of the Greater Caucasus, the climate is generally warm summer continental (Dfb) from the foothills to the middle mountains and cool summer continental (Dfc) or even alpine (ET) in the highlands. The North-Western Caucasus and the Black Sea coast of Western Transcaucasia also have a humid subtropical (Cfa) and oceanic (Cfb) climate. In the Greater Caucasus as a whole, aridity increases from northwest to southeast. The climate of the Colchis Lowland is humid subtropical and oceanic, similar to that in the northwestern part of the Kura-Araks Lowland. The southeastern part of the Kura-Araks Lowland has a cold semi-arid climate (BSk). In the mountainous areas of the Lesser Caucasus and Transcaucasian Highland, a warm summer continental climate prevails (drier to the southeast). A cold semi-arid climate is prevalent in the southern part of the Transcaucasian Highland ([55]).
Fig. 1 - The geographic location and orography of the study area. 1 - North-Western Caucasus, 2 - Central Caucasus, 3 - Eastern Caucasus, 4 - Western Transcaucasia, 5 - Central Transcaucasia, 6 - Eastern Transcaucasia, 7 - Javakheti Range, 8 - Meskheti Range, 9 - Trialeti Range, 10 - Somkheti Range, 11 - Bazum Range, 12 - Sevan Range, 13 - Karabakh Range, 14 - Samsari Range, 15 - Tsakhkunyat Range.
The Central Caucasus occupies the most highland areas of the northern slopes in the Greater Caucasus (Fig. 1). The climate of the mountainous areas, as throughout the Caucasus, is cool summer continental (Dfc) and alpine (ET) with increasing dryness and continentality as altitude increases. The average annual precipitation is about 900 mm and mean daily temperature ranges from 12.6 °C in July to -6.7 °C in December (data from Terskol weather station, 2150 m a.s.l.).
The most widespread vegetation belts in the Caucasus are subalpine and alpine meadows and nival heaths. The nival belt is a permafrost zone where vegetation consists of mosses, algae and lichens. The alpine belt includes low-grass meadows and alpine heaths. The subalpine belt features fertile soils and a rich vegetation cover with a predominance of mesophytic meadows. The underlying belt of dark coniferous forests of spruce (Picea orientalis [L.] Peterm.) and fir (Abies nordmanniana) passes through the humid western and central regions of the Greater and Lesser Caucasus. A belt of deciduous forests of beech (Fagus orientalis) with hornbeam (Carpinus betulus) extends through the foothills and middle mountains of the entire ecoregion. Only in the Transcaucasian Highland deciduous forests are replaced by oaks (Quercus robur L., Q. iberica Steven ex M. Bieb., etc.) and juniper (Juniperus excelsa M. Bieb., J. foetidissima Willd., and J. oxycedrus L.) forests. Beech forests in this arid region belong mainly to river floodplains. Pine forests of Pinus sylvestris are most widespread in the Greater Caucasus, where they form fragmented stands on the border with subalpine meadows. In the warm humid climate of the Black and Caspian Sea coasts there is a belt of lowland subtropical forests. Semi-deserts form the lower vegetation belts in the relatively arid central and eastern regions of the Caucasus. In the most humid western regions, the lower belt is formed by steppes.
In the Central Caucasus, the belt spectrum includes semi-deserts, steppe meadows and forest-steppes, beech forests, subalpine and alpine meadows, and nival heaths. The beech forest belt is significantly fragmented due to intensive logging during the 20th century ([67], [59]). Pine forests do not form a vegetation belt, but constitute large undisturbed stands of Pinus sylvestris on steep slopes in middle mountains and highlands, mostly within protected areas (Prielbrusye National Park and Kabardino-Balkar High Mountain State Natural Reserve).
Geographic records and environmental variables
The geographic records of Lobaria pulmonaria were sourced from the expedition research in 2012-2022 in the Greater and Lesser Caucasus (27 records) and from the Global Biodiversity Information Facility ([26] - see Fig. S1 in Supplementary material). Presence points of the species in the Transcaucasian Highland were obtained from the GBIF. A total of 157 species occurrence data from the GBIF (⇒ https://doi.org/10.15468/dl.gf6mxy) were used in the analysis. All samples are stored in the herbarium collection of the Tembotov Institute of Ecology of Mountain Territories of the Russian Academy of Science. To address the problem of spatial clustering of geographic records and sampling bias, we applied spatial thinning. This popular correction method, based on the removal of presence points, provides an occurrence dataset from which efficient SDM models can be constructed ([2], [60]). Accordingly, the species occurrence data for the regional SDM model, were checked for duplicates and spatially rarefied to one record per 1 km2 grid cell using the “clean duplicate” function of the R package “ntbox” ([48]). A total of 174 species occurrence data were retained in the analysis after spatial thinning. The local SDM model (Central Caucasus) was built from 32 geographic records with density and location that did not suggest the necessity for spatial thinning.
We used 16 climatic and two topographic variables from the ENVIronmental Rasters for Ecological Modeling, i.e., the ENVIREM dataset ([68], [22] - Tab. 1). A number of ENVIREM variables (potential evapotranspiration of different quarters, annual potential evapotranspiration, Emberger’s pluviothermic quotient, Thornthwaite aridity index, monthly variability in potential evapotranspiration) based on the evapotranspiration, which is directly linked to physiological and ecological processes in vegetation cover ([68]). These variables, therefore, are effective for predicting the distribution of biological objects ([1], [69]). Second, in mountainous areas, many direct environmental predictors (temperature, precipitation, evapotranspiration, and slope steepness) vary consistently with altitude, leading to an increase in their correlation. However, some ENVIREM variables combine direct predictors such as temperature and potential evapotranspiration (Emberger’s pluviothermic quotient), precipitation and potential evapotranspiration (Thornthwaite aridity index), slope height and steepness (terrain roughness index), slope steepness and moisture (topographic wetness index). Their use, in our opinion, avoids the application of highly correlated direct variables that have consistent variability along an altitude gradient in mountains. To prevent model overfitting, we used the VIF (Variance Inflation Factor) test in R to select uncorrelated environmental layers (VIF threshold ≤ 3), also excluding latent correlations. As a result, five climatic and topographic variables were involved in the analysis (Tab. 1).
Tab. 1 - Results of VIF test used to select noncorrelated environmental variables. Abbreviation, descriptions, and units were based on Title & Bemmels ([68]). (*): ENVIREM variables included in the analysis after Variance Inflation Factor (VIF) test.
Variable | Description, units | VIF |
---|---|---|
PETWettestQuarter * | Mean monthly PET (potential evapotranspiration) of wettest quarter, mm month-1 | 1.78 |
EmbergerQ * | Emberger’s pluviothermic quotient | 1.91 |
PETColdestQuarter * | Mean monthly PET of coldest quarter, mm month-1 | 2.06 |
TRI * | Terrain roughness index | 2.24 |
PETDriestQuarter * | Mean monthly PET of driest quarter, mm month-1 | 2.37 |
aridityIndexThornthwaite | Thornthwaite aridity index | 4.80 |
PETWarmestQuarter | Mean monthly PET of warmest quarter, mm month-1 | 6.37 |
PETseasonality | Monthly variability in potential evapotranspiration, mm month-1 | 8.37 |
minTempWarmestMonth | Minimum temperature of the coldest month, °C×10 | 9.88 |
maxTempColdestMonth | Maximum temperature of the coldest month, °C×10 | 10.57 |
annualPET | Annual potential evapotranspiration, mm year-1 | 11.42 |
thermInd | Compensated thermicity index, °C | 11.78 |
climaticMoistureIndex | A metric of relative wetness and aridity | 13.22 |
growingDegDays5 | Sum of mean monthly temperature for months with mean temperature > 5 °C multiplied by number of days | 15.63 |
continentality | Difference between the average temp. of the warmest month and the average temp. of the coldest month, °C | 16.48 |
topoWet | SAGA-GIS topographic wetness index | 16.97 |
growingDegDays0 | Sum of mean monthly temperature for months with mean temperature greater than 0 °C multiplied by number of days | 17.22 |
monthCountByTemp10 | Number of months with mean temp. > 10 °C | 18.34 |
To build BA-models of Lobaria pulmonaria, we re-modeled the spatial distribution of lungwort lichen using the abiotic environmental variables and previously obtained BAM-models of Pinus sylvestris and Fagus orientalis ([55]) as biotic layers. This method is consistent with the correlative approach to ecological niche modeling, which involves incorporating the geography of other species into single-species models ([61]). Movement factor in BAM-models characterizes a species dispersal capacity or accessible areas ([61], [51], [50]). Geographically, the movement factor indicates “the regions that are accessible to dispersal by the species from some original area over a relevant period of time” ([61], [50]). At present, there is no unified framework for the geographic specification of these regions. In our study, the movement factor indicated the part of the Caucasus that was most accessible to Lobaria pulmonaria at regional and local scales. Sites with a species occurrence probability of 0.8-1 in the BA-models were considered optimal areas. The raster of distances from optimal sites, where the probability of the lungwort lichen occurrence remained above 0.5, was used as a movement-layer in the BAM-models of Lobaria pulmonaria.
For the regional SDM model, ready-to-use environmental layers were downloaded ([22]) with a resolution of 1 km per pixel. For the local model, environmental layers (except TRI values) were downscaled in resolution to 30 m per pixel by the cubic spline interpolation ([16]) and cropped by the mask of the Central Caucasus in the R “dismo” package ([29]). The basic assumption of this method is that when the resolution of the environmental layers is downscaled to 30 m per pixel by cubic spline interpolation, the amount of information also increases. The TRI values for the local model were calculated in the R package “spatialEco” ([63]) using data from the Shuttle Radar Topography Mission ([64]) digital elevation model.
Maxent model development and evaluation
We used the Maxent software ver. 3.4.3 ([53]) in the R “dismo” package ([29]) as an efficient modelling method based on presence-only data ([54]). Optimal model parameters were determined in the R “ENMeval” package ([43]). We applied 10.000 background samples and feature types L, Q, H, LQ, and LQH. Regularization multiplier ranged from 0.5 to 2 in increments of 0.5 for local models and from 0.5 to 5 in increments 0.5 for regional models. A total of 20 local and 50 regional models were generated. We used the Akaike’s information criterion corrected (AICc), the difference between the AICc and its minimum value (ΔAICc), the continuous Boyce index (CBI), and the area under receiver operating characteristic curve AUC from the training data (AUCtrain) to select the models with optimal combinations of regularization multiplier and feature types (Tab. 2). We preferred models with the lowest AICc, ΔAICc, BIC and highest CBI values, which balance model complexity and accuracy/goodness-of-fit ([8], [12]). The higher the AUCtrain values, the better the specificity and sensitivity of model in distinguishing occurrence data from random (background) data ([23]). For the resulting A-, BA- and BAM-models of Lobaria pulmonaria, we applied a five-fold cross-validation, using 20% of occurrence data as test samples and 80% as training samples ([54]). Accordingly, the AUC values of models were averaged over five replicates.
Tab. 2 - Parameters and evaluation of optimal Maxent models of Lobaria pulmonaria distribution in the Caucasus at local and regional spatial scales. AICc: Akaike’s information criterion corrected; AUC: area under the curve; CBI: continuous Boyce index; RM: regularization multiplier.
Spatial scale | Model | AICc | ΔAICc | CBI | AUCtrain | Feature type | RM |
---|---|---|---|---|---|---|---|
Local | A-Model | 205.6 | 25.7 | 0.95 | 0.98 | LQH | 0.5 |
BA-Model | 195.14 | 0.00 | 0.82 | 0.99 | LQH | 1.5 | |
BAM-Model | 190.4 | 0.00 | 1.00 | 0.99 | L | 0.5 | |
Regional | A-Model | 2638.6 | 17.40 | 0.99 | 0.97 | LQH | 0.5 |
BA-Model | 2613.8 | 6.25 | 0.99 | 0.97 | LQH | 0.5 | |
BAM-Model | 2538.6 | 3.48 | 1.00 | 0.98 | LQH | 0.5 |
There is no optimal method for determining the habitat suitability threshold and various thresholds values were used in Maxent modelling ([38]). In this study, we applied a fixed high habitat suitability threshold of 0.8 for optimal localities ([11]), which reduces the risk of false positives, and a habitat suitability threshold of 0.5 for potentially suitable localities.
The significance of environmental predictors in Lobaria pulmonaria distribution was analyzed using percentage contribution (PC, %) and permutation importance (PI, %) ([53]). We also analysed the optimal values of variables from the response curves, which demonstrate the relationship between predictors and the probability of optimal (0.8 thresholds of habitat suitability) conditions.
After model development and evaluation, we conducted field verification of the results. Points for field verification were randomly generated using the “randomPoints” function of the R package “dismo” ([29]). The presence points were exluded from background and the predicted probabilities were used as probability weights. A total of 49 locations were surveyed in the Central Caucasus with predicted probability values ranging from 0.07 to 0.98.
Visualisation and assessment of ecological niche overlap
We used the KDE (Kernel density estimation) method of Blonder et al. ([6]) to visualise ecological niches (Hutchinson hypervolumes) as agglomerations of points in an n-dimensional space of environmental variables (biologically important independent axes), where the points represent acceptable values of these variables. The KDE method is conceptually simple, requires no absence data and is suitable for SDM modelling ([6]). It is particularly effective in studying the realised ecological niches of species ([56]). In our study, the lungwort lichen ecological niches were visualised in the orthogonal space of PCA (Principal Component Analysis) axes that integrated the ENVIREM variables selected by the VIF test. We used the the “FactoMineR” package in R ([39]) for principal component analysis and the “factoextra” ([36]) and “ggplot2” ([71]) packages in R for axis extraction and visualisation.
Results
BAM-models of Lobaria pulmonaria distribution
AUC values of the A-models as well as the BA- and BAM-models of Lobaria pulmonaria indicated their good predictive success (Tab. 3). The main abiotic predictor for the species distribution in the Central Caucasus was the Terrain roughness index (TRI), which quantifies the local vertical orographic heterogeneity of landscapes ([57] - Tab. 3). Optimal TRI values (0.8 thresholds of habitat suitability) at the local scale ranged from intermediately rugged (162-239) to highly rugged (498-958) mountain slopes as classified by Riley et al. ([57]). In terms of percentage contribution, PETDriestQuarter and embergerQ contributed less to the local A-model. According to the optimal values of these parameters ([21], [14]), the lichen occurred mostly in humid areas of the Central Caucasus. At the regional scale, the main environmental predictor was embergerQ, which characterizes humidity and temperature of climate with increasing values for wetter conditions ([21], [14] - Tab. 3). In the Caucasus region, Lobaria pulmonaria also preferred humid areas with embergerQ of at least 100. At the same time, the optimal habitats of the lungwort lichen were more gentle slopes, ranging from nearly level (81-116) to intermediately rugged (162-239) according to Riley et al. ([57]). The percentage contribution of TRI in the regional A-model was only 17.5%.
Tab. 3 - Contribution of environmental variables to the Maxent distribution models of Lobaria pulmonaria in the Caucasus. PC (percentage contribution): variable contribution in the models; PI (permutation importance): the permutation coefficient. Optimal values: optimal values of variables (0.8 thresholds of habitat suitability) from the response curves. Species occurrence: probability of tree species occurrence in sites with 0.8 habitat suitability threshold for Lobaria pulmonaria.
Spatial scale | Environmental variable | A-Model | BA-Model | BAM-Model | ||||||
---|---|---|---|---|---|---|---|---|---|---|
PC, % | PI, % | Optimal values | PC, % | PI, % | Optimal values | PC, % | PI, % | Optimal values | ||
Local | TRI | 49.9 | 21.4 | 200-580 | 8 | 18.2 | 200-580 | 0.1 | 0.4 | 200-650 |
PETDriestQuarter, mm month-1 |
24.2 | 15.5 | 20-25 | 1.8 | 0.7 | 20-25 | 0 | 0 | 20-25 | |
embergerQ | 21.6 | 59.8 | 115-140 | 8.7 | 20.3 | 115-140 | 0.8 | 0.5 | 115-140 | |
PETColdestQuarter, mm month-1 |
2.1 | 1.8 | 15-17 | 1.6 | 4.6 | 15-17 | 0.2 | 0.2 | 15-17 | |
PETWettestQuarter, mm month-1 |
1.2 | 1.4 | 80-115 | 0.3 | 1.7 | 80-115 | 0 | 0.1 | 80-115 | |
Pinus sylvestris occurrence | - | - | - | 70.7 | 42.1 | 0.8-1 | 17.6 | 1.1 | 0.8-1 | |
Fagus orientalis occurrence | - | - | - | 8.7 | 10.3 | 0-0.1 | 1.3 | 0 | 0-0.1 | |
Movement factor, km |
- | - | - | - | - | - | 79.9 | 97.6 | 0.02 | |
AUC ± SD | 0.96 ± 0.08 | 0.90 ± 0.18 | 0.94 ± 0.11 | |||||||
Regional | embergerQ | 59.4 | 48.2 | 100-150 | 34.7 | 33.8 | 100-150 | 3.2 | 11 | 100-150 |
TRI | 17.5 | 10.9 | 100-200 | 0.3 | 1.4 | 100-200 | 0.5 | 2.1 | 100-200 | |
PETDriestQuarter, mm month-1 |
14.3 | 18.7 | 20-40 | 12.1 | 18.2 | 20-40 | 2.1 | 17 | 20-40 | |
PETWettestQuarter, mm month-1 |
8 | 20.2 | 110-130 | 3.4 | 3.9 | 110-130 | 1.5 | 6.5 | 110-130 | |
PETColdestQuarter, mm month-1 |
0.9 | 2 | 18-20 | 0 | 0 | 18-20 | 0.5 | 2.1 | 18-20 | |
Fagus orientalis occurrence | - | - | - | 35.1 | 21.7 | 0.7-1 | 28 | 13.3 | 0.7-1 | |
Pinus sylvestris occurrence | - | - | - | 14.5 | 21 | 0.8-1 | 8.6 | 1.5 | 0.8-1 | |
Movement factor, km |
- | - | - | - | - | - | 55.6 | 46.7 | 0.03 | |
AUC ± SD | 0.95 ± 0.01 | 0.96 ± 0.01 | 0.96 ± 0.01 |
The mapped regional A-model illustrated the concentration of the lichen optimal habitats in the wettest areas of the Caucasus, specifically in the middle mountains and highlands of the North-Western Caucasus, the Western and Central Transcaucasia and the north-western ridges of the Lesser Caucasus (Fig. 2b). The Colchis and Kura-Araks Lowlands, the Ciscaucasian plains as well as the mountainous areas with a dry continental climate (Eastern Caucasus, Transcaucasian Highland, and most of the Lesser Caucasus) had the least suitable abiotic conditions for Lobaria pulmonaria. Optimal areas in the Central Caucasus with a relatively dry continental climate were limited to the valleys of large rivers (Fig. 3b).
Fig. 2 - Distribution maps of Lobaria pulmonaria potential habitats in the Caucasus: A-Model (a), BA-Model (b) and BAM-Model (c). 0-1 is the probability of the species occurrence in the Maxent standard palette colour gradations. Distribution maps were obtained by converting the Maxent output file into a netCDF file with visualisation in PanoplyWin ([49]).
Fig. 3 - Distribution maps of Lobaria pulmonaria potential habitats in the Central Caucasus. The geographic location of the Central Caucasus (a); A-Model (b), BA-Model (c) and BAM-Model (d) of the lichen distribution.
In the BA-models, the most significant environmental predictors of the lichen distribution were biotic variables (phorophyte occurrence), which masked the effects of abiotic factors, particularly at the local scale (Tab. 3). Climate type (embergerQ) remained an important habitat characteristic of the species at the regional scale. In the Central Caucasus, habitats were considered optimal for Lobaria pulmonaria if the probability of Pinus sylvestris occurrence at the sites was 0.8-1.0. Fagus orientalis occurrence was more important for the lichen distribution at the regional scale. The optimal habitats of the lungwort lichen were those with a probability of beech forest occurrence of 0.7-1.0.
Comparing A- and BA-models, the biotic factor reduced the area of suitable and optimal lungwort lichen habitats by 3.0-3.3 times at the local scale and by 1.3-1.8 times at the regional scale (Tab. 4). In the Central Caucasus, Lobaria pulmonaria habitats were mostly predicted in the Kabardino-Balkarian Republic, where the main pure pine stands of the Caucasus region are concentrated (Fig. 3c). In the Caucasus region, areas of the potential lichen habitats decreased evenly throughout the entire predicted range (Fig. 2b). The lesser reduction in the lungwort lichen range in the regional BA-model was probably due to the remaining influence of the macroclimatic embergerQ factor.
Tab. 4 - Areas of acceptable and optimal habitats of Lobaria pulmonaria in the Caucasus by the Maxent distribution models.
Spatial scale | Model | Local | Regional |
---|---|---|---|
Acceptable areas, thousand km2 | A-Model | 0.76 | 25.34 |
BA-Model | 0.23 | 19.28 | |
BAM-Model | 0.06 | 14.83 | |
Optimal areas, thousand km2 | A-Model | 0.06 | 7.3 |
BA-Model | 0.02 | 3.94 | |
BAM-Model | 0.01 | 2.32 |
Movement factor was the most significant environmental predictor of Lobaria pulmonaria distribution in the BAM-models (Tab. 3). However, the percentage contribution of Fagus orientalis occurrence in the regional BAM-model was not much less than that in the BA-model. The distance of suitable areas from the optimal lichen habitats was only 20 m in the Central Caucasus and 30 m within the Caucasus region. Twenty-metre accessibility of suitable areas reduced the potential range of Lobaria pulmonaria in the Central Caucasus by 3.8 times (suitable areas) and 2.5 times (optimal areas) compared to BA-models (Tab. 4, Fig. 3d). The area of species potential habitat at the regional scale decreased only by 1.3 times (suitable areas) and 1.7 times (optimal areas - Tab. 4, Fig. 2c).
Most of the occurrence points used for local model construction were within sites with a high (above 0.8) predicted probability of Lobaria pulmonaria occurrence (Fig. 4). Of the 49 locations visited during the field verification in the Central Caucasus, seven points had predicted occurrence probabilities above 0.8. Of these, Lobaria pulmonaria presence was confirmed at six locations (presence in field validation - Fig. 4), which accounted for 85.7% of correct predictions. The absence of the species was observed in 43 locations (absence in field validation). Only 11 of them had relatively high (0.5-0.8, 10 locations) and high (above 0.8, one location) predicted probability of occurrence (false positives). The remaining 33 locations with confirmed absence of the lungwort lichen had low, up to 0.5, predicted occurrence probabilities (Fig. 4).
Fig. 4 - Results of field verification of Lobaria pulmonaria SDM model in the Central Caucasus. Dotted and solid lines indicate cut-offs at predicted occurrence probabilities of 0.5 and 0.8, respectively (n = 49 localities).
Differentiation of the lungwort lichen ecological niches at local and regional scales
The PCA analysis revealed four main complex factors with a cumulative variation of about 92% in environmental variables (Tab. 5). The climate predictor PETDriestQuarter mostly formed the first main axis of the PCA (about 45% of variable variance). Pinus sylvestris occurrence had the greatest factor loading on the second PCA axis. The two correlated variables of the third PCA factor were embergerQ and Fagus orientalis occurrence. The fourth PCA axis was formed by a single orographic variable TRI. Visualisation of the lungwort lichen ecological niches at local and regional spatial scales using the KDE method showed their significant differentiation along all four PCA axes (Fig. 5).
Tab. 5 - Results of a principal component analysis (Varimax normalized) of environmental variables in Lobaria pulmonaria occurrence points from the Caucasus. (*): Factor loadings > 0.7.
Environmental variables | Factor 1 | Factor 2 | Factor 3 | Factor 4 |
---|---|---|---|---|
embergerQ | -0.31 | 0.04 | 0.86* | -0.18 |
Fagus orientalis occurrence | 0.05 | 0.01 | 0.81* | 0.51 |
PETColdestQuarter, mm month-1 | -0.66 | -0.10 | 0.57 | 0.29 |
PETDriestQuarter, mm month-1 | 0.97* | -0.06 | -0.10 | 0.12 |
Pinus sylvestris occurrence | 0.07 | 0.99* | 0.02 | -0.15 |
TRI | -0.23 | 0.18 | -0.06 | 0.91* |
Eigenvalue | 2.71 | 1.25 | 0.91 | 0.67 |
Explained variance, % | 45.11 | 20.79 | 15.17 | 11.12 |
Fig. 5 - Visualization of Lobaria pulmonaria ecological niches at the local (blue) and regional (red) spatial scales in the orthogonal space of PCA axes.
Discussion
Our approach to identifying the distribution factors of the rare epiphytic lichen Lobaria pulmonaria in the Caucasus forests was based on the use of species distribution modelling and ecological niche theory. Such an approach allowed us not only to reveal the main environmental predictors and to map the lichen possible distribution, but also to assume a significant influence of spatial scale of research on the modelling results. In contrast to conventional SDM studies, we based our models on the BAM concept ([61], [51], [50]) and considered not only abiotic factors, but also interspecific interactions (occurrence of phorophyte species) and movement factor (accessibility of areas).
Main predictors of Lobaria pulmonaria distribution
Considering that the distribution of epiphytic lichens strongly depends on the localization of their phorophytes, the TRI values in the optimal localities of Lobaria pulmonaria probably characterised the orographic distribution of its main epiphytes. Pine forests, which are the main phorophyte of the lungwort lichen in the Central Caucasus, grows on steeper slopes (up to highly rugged) than beech forests, which are the main lichen phorophytes in the Caucasus ecoregion and grow mainly from nearly level to intermediately rugged slopes ([55]). Accordingly, the optimal habitats for Lobaria pulmonaria at the local scale were mountain slopes from intermittently rugged to highly rugged, while at the regional scale the optimal slopes ranged from nearly level to intermediately rugged (Tab. 3).
The significance of embergerQ and PETDriestQuarter in Lobaria distribution models is consistent with previous studies, which showed the important role of climatic factors in physiological processes and distribution of the lichen. Being macroclimatic large-scale parameters, embergerQ and PETDriestQuarter characterize climate temperature and humidity. Fos et al. ([24]) emphasized the relationship of Lobaria pulmonaria distribution with climate humidity in the Iberian Peninsula. As in the Caucasus ecoregion, the species mainly occurred in the northern and western parts of the peninsula with a wetter climate ([24]). The bioclimatic models of Eaton & Ellis ([17]) demonstrated that a macroclimatic variable such as precipitation positively affects the Lobaria pulmonaria growth rate in North America. Considering that there is a positive relationship between thallus size and the number of the diaspores ([13], [42]), precipitation also affects the distribution of lungwort lichen. At the local scale, this factor influences the microclimatic characteristics of habitat humidity, which in turn determine indicators of Lobaria pulmonaria distribution efficiency such as soredia maturation, germination and attachment to the substrate ([13], [42]).
Due to the complex interactions of epiphytic lichens with phorophyte species, tree parameters and forest stand characteristics, the analysis of abiotic predictors of Lobaria pulmonaria distribution alone is insufficient. At the local scale, the probability of the lichen occurrence was highest (80-100%) in areas optimal for pine forests (Tab. 3). In fact, the lungwort lichen localities in the Central Caucasus were strictly confined to pine forests ([37]). In beech stands of the Central Caucasus with a predicted probability of the lichen occurrence of only 10% (Tab. 3), we did not find Lobaria pulmonaria despite long-term careful field investigations. In contrast, the spatial localisation of beech forests was a significant predictor of the lichen distribution at the region scale (Tab. 3). The overlapping ranges of Lobaria pulmonaria (Fig. 2) and Fagus orientalis ([55]) occurred mainly in the western and central parts of the Greater and Lesser Caucasus. This observation supports previous studies that in the Mediterranean region, the lungwort lichen was mainly distributed in humid oceanic areas also optimal for broadleaved forests ([24]). The fragmented more arid eastern part of beech range (Eastern Caucasus and Transcaucasia, south-eastern Lesser Caucasus and Transcaucasian Highland) also was least suitable for the lichen. However, the eastern range of Fagus orientalis ([55]) still included some areas unsuitable for Lobaria pulmonaria populations. Although the beech forest distribution contributed greatly to the regional BA-model (percentage contribution about 35%), the climatic parameter embergerQ remained equally important (Tab. 3). Therefore, the predicted absence of lungwort lichen in beech forests of the Eastern Caucasus and Transcaucasia, south-eastern Lesser Caucasus and Transcaucasian Highland was most probably caused by unsuitable climatic conditions, which were as important for the lichen distribution as the biotic factor.
It is commonly known that Lobaria pulmonaria usually colonised old trees with a large trunk diameter ([13], [10], [34]). At the landscape level, the lungwort lichen populations did best in undisturbed old-growth forests with a large area and low fragmentation ([13], [45], [10], [32]). Moreover, fertile populations of Lobaria pulmonaria were most commonly found in low canopy forests or within “gaps” in the canopy layer ([42], [45], [34]). In dense forests, the lichen preferred the upper parts of tree trunks if there was sufficient humidity ([45]). Based on the above, we hypothesised that the absence of Lobaria pulmonaria in beech forests of the Central Caucasus was probably due, firstly, to unsuitable microclimatic conditions for the species. In this area, the main beech forest type is dense stands (canopy density of 0.8-0.9) with insolation and moisture deficit in the understorey ([67], [59]). Secondly, intensive clear-cutting of beech forests in the Kabardino-Balkarian Republic alone decreased their area by 53.4% between 1957 and 2007 ([67], [59]). Fragmentation and extensive loss of old-growth forests has probably reduced the suitability of beech stands for Lobaria pulmonaria in the Central Caucasus.
The moisture availability in the undergrowth of pine forests in the Central Caucasus varies considerably according to their type. Slope pine forests with rhododendron (Rhododendron caucasicum Pall.) in the undergrowth, as well as valley pine green-moss and bilberry (Vaccinium myrtillus L.) forests have a relatively humid microclimate ([59]). Canopy density of such forests rarely exceeds 0.6-0.8. The pine forests of the Central Caucasus are generally distributed on steep slopes in the middle mountains and highlands, which make logging and skidding difficult. The last incidents of intensive destruction of mountain pine forests in Kabardino-Balkaria occurred in the late 1910s, during the civil war, then the forests were protected ([59]). Thus, sufficient insolation, humidity and extent of pine forests, their relative undisturbedness and the presence of old-growth stands probably explain the occurrence of Lobaria pulmonaria in pine forests of the Central Caucasus.
According to the BAM-models, the most important predictor of Lobaria pulmonaria distribution at the local and regional scales was the dispersal capability of the species (accessibility of areas), which was only 20 m in the Central Caucasus and 30 m throughout the Caucasus (Tab. 3). This observation supports previous studies that dispersal capability mainly limited the lungwort lichen local distribution in southern Sweden ([47]). The established average dispersal distance of vegetative diaspores of the species was 15-30 m ([47], [42], [35]). At the same time, vegetative reproduction by thallus fragments, soredia and/or isidia dominates the lichen life cycle ([40], [10]). Vegetative diaspores are spread by wind, snails, insects ([58]) and have a low survival rate ([42]). Sexual reproduction by small ascospores ensures dispersal of Lobaria pulmonaria over hundreds of metres, but occurs rarely even in optimal habitats of the species ([13], [10], [34]). According to Carlsson & Nilsson ([13]), sexual reproduction of the lungwort lichen does not occur if the entire lichen population comprises a single genotype.
Overlap of the lungwort lichen ecological niches at local and regional scales
KDE methods revealed a significant differentiation of Lobaria pulmonaria ecological niches formed at the local and regional spatial scales (Fig. 5). The niche divergence by Factor 2 (Pinus sylvestris occurrence) and by Factor 3 (embergerQ and Fagus orientalis occurrence) is probably related to different substrate preferences of the lichen, i.e., pine forests in the Central Caucasus and beech forests throughout the Caucasus. The localisation of phorophytes also probably determines the niche divergence by orographic Factor 4 (TRI). Pine forests of the Caucasus are distributed on steep slopes in the middle mountains and highlands, while beech stands occur mainly on gentler slopes in the lowlands and middle mountains.
Thus, the spatial localisation patterns and ecological niche parameters of Lobaria pulmonaria strongly depended on the scale of the analysed area. According to the theoretical framework of the BAM-diagram ([61], [4], [52]), in large-scale studies at a continental or regional level, the importance of bioclimatic variables and the movement factor in species distribution should be particularly high; in local studies interspecific interactions become more important. In this study, we confirmed that the importance of bioclimatic variables in the spatial distribution of biological objects is higher in large-scale studies, while interspecific interactions are more significant in local studies. On the other hand, the contribution of the movement factor to the local BAM-model of Lobaria pulmonaria distribution reached 80%, which contradicts the theory on the relative insignificance of area accessibility in local studies ([61], [4], [52]). In our opinion, these contradictions were related to the specificity of research in the mountains, where species dispersal is primarily hampered by the factor of geographical isolation, appearing first of all at the local scale.
Conclusions
Spatial scale affected the potential localisation and ecological niche of the epiphytic lichen Lobaria pulmonaria. Therefore, the patterns of the lichen spatial distribution at the regional scale are not applicable to explain the species distribution at the local scale. Different abiotic predictors determined the potential lichen distribution in the Central Caucasus (terrain) and throughout the Caucasus (macroclimate). The influence of interspecific relationships (lichen-phorophyte) was more important at the local scale, whereas the regional model retained a significant macroclimate contribution. In the Central Caucasus, the highest probability of the lungwort lichen occurrence (80-100%) was predicted in valley pine forests. In the Caucasus region, the lichen occurrence was most probable in humid beech forests (70-100%) of the North-Western Caucasus, the Western and Central Transcaucasia and the north-western ridges of the Lesser Caucasus. Due to the strong dependence of Lobaria pulmonaria distribution on the biotic factor (suitable phorophytes), protection of pine and beech forests in the Caucasus is necessary to preserve the species. According to the final BAM-models, the most significant predictor of the lichen spatial distribution was the movement factor (accessibility of areas), which limited species dispersal from optimal habitats within a radius of 20 m in the Central Caucasus and 30 m through the Caucasus.
The resulting BAM-models had a high predictive accuracy in accordance with the AUC values. Field verification of the local BAM-model showed that using a cut-off value of 0.8, the presence prediction success rate was 85.7%. We identified six new occurrences of Lobaria pulmonaria at predicted locations in the Central Caucasus. Therefore, in our opinion, the combination of SDM modelling and ecological niches theory based on the BAM concept is an effective method for studying the potential localisation and the ecological niches of epiphytic lichens.
Acknowledgements
The study was supported in part by the Russian Science Foundation, projects no. 23-24-10075, and was conducted within the framework of the State Assignment, project no. 075-00347-19-00 “Patterns of the spatiotemporal dynamics of meadow and forest ecosystems in mountainous areas (Russian Western and Central Caucasus)”.
Author contributions
RP and VCh planned the study. ZKh carried out the data collection, wrote the first draft. RP performed the statistical analysis and designed the figures. VCh contributed to data analysis, reviewed and edited the manuscript.
References
Gscholar
Gscholar
Gscholar
Gscholar
Online | Gscholar
Gscholar
Gscholar
CrossRef | Gscholar
CrossRef | Gscholar
Gscholar
Gscholar
Gscholar
Gscholar
Gscholar
CrossRef | Gscholar
Supplementary Material
Authors’ Info
Authors’ Affiliation
Zalim Khanov 0000-0003-3090-2534
Victoria Chadaeva 0000-0002-0788-1395
Tembotov Institute of Ecology of Mountain Territories of Russian Academy of Science, 37a I. Armand Street, 360051 Nalchik (Russia)
Corresponding author
Paper Info
Citation
Pshegusov R, Khanov Z, Chadaeva V (2024). Distribution factors of the epiphytic lichen Lobaria pulmonaria (L.) Hoffm. at local and regional spatial scales in the Caucasus: combining species distribution modelling and ecological niche theory. iForest 17: 120-131. - doi: 10.3832/ifor4406-017
Academic Editor
Michele Carbognani
Paper history
Received: Jun 22, 2023
Accepted: Jan 29, 2024
First online: Apr 30, 2024
Publication Date: Apr 30, 2024
Publication Time: 3.07 months
Copyright Information
© SISEF - The Italian Society of Silviculture and Forest Ecology 2024
Open Access
This article is distributed under the terms of the Creative Commons Attribution-Non Commercial 4.0 International (https://creativecommons.org/licenses/by-nc/4.0/), which permits unrestricted use, distribution, and reproduction in any medium, provided you give appropriate credit to the original author(s) and the source, provide a link to the Creative Commons license, and indicate if changes were made.
Web Metrics
Breakdown by View Type
Article Usage
Total Article Views: 2585
(from publication date up to now)
Breakdown by View Type
HTML Page Views: 670
Abstract Page Views: 611
PDF Downloads: 1227
Citation/Reference Downloads: 1
XML Downloads: 76
Web Metrics
Days since publication: 205
Overall contacts: 2585
Avg. contacts per week: 88.27
Article Citations
Article citations are based on data periodically collected from the Clarivate Web of Science web site
(last update: Feb 2023)
(No citations were found up to date. Please come back later)
Publication Metrics
by Dimensions ©
Articles citing this article
List of the papers citing this article based on CrossRef Cited-by.
Related Contents
iForest Similar Articles
Research Articles
Local ecological niche modelling to provide suitability maps for 27 forest tree species in edge conditions
vol. 13, pp. 230-237 (online: 19 June 2020)
Research Articles
Impact of forest management on threatened epiphytic macrolichens: evidence from a Mediterranean mixed oak forest (Italy)
vol. 12, pp. 383-388 (online: 12 July 2019)
Research Articles
Retaining unlogged patches in Mediterranean oak forests may preserve threatened forest macrolichens
vol. 12, pp. 187-192 (online: 10 April 2019)
Research Articles
Some refinements on species distribution models using tree-level National Forest Inventories for supporting forest management and marginal forest population detection
vol. 11, pp. 291-299 (online: 13 April 2018)
Research Articles
Environmental niche and distribution of six deciduous tree species in the Spanish Atlantic region
vol. 8, pp. 214-221 (online: 28 August 2014)
Research Articles
Spatial modeling of the ecological niche of Pinus greggii Engelm. (Pinaceae): a species conservation proposal in Mexico under climatic change scenarios
vol. 13, pp. 426-434 (online: 16 September 2020)
Research Articles
Analysing species abundance distribution patterns across sampling scales in three natural forests in Northeastern China
vol. 13, pp. 482-489 (online: 01 November 2020)
Research Articles
Spatio-temporal modelling of forest monitoring data: modelling German tree defoliation data collected between 1989 and 2015 for trend estimation and survey grid examination using GAMMs
vol. 12, pp. 338-348 (online: 05 July 2019)
Research Articles
Fire occurrence zoning from local to global scale in the European Mediterranean basin: implications for multi-scale fire management and policy
vol. 9, pp. 195-204 (online: 12 November 2015)
Short Communications
Dynamic modelling of target loads of acidifying deposition for forest ecosystems in Flanders (Belgium)
vol. 2, pp. 30-33 (online: 21 January 2009)
iForest Database Search
Search By Author
Search By Keyword
Google Scholar Search
Citing Articles
Search By Author
Search By Keywords
PubMed Search
Search By Author
Search By Keyword