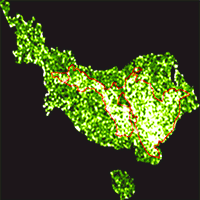
COSMO-SkyMed potential to detect and monitor Mediterranean maquis fires and regrowth: a pilot study in Capo Figari, Sardinia, Italy
iForest - Biogeosciences and Forestry, Volume 11, Issue 3, Pages 389-395 (2018)
doi: https://doi.org/10.3832/ifor2623-011
Published: May 15, 2018 - Copyright © 2018 SISEF
Research Articles
Abstract
Mediterranean maquis is a complex and widespread ecosystem in the region, intrinsically prone to fire. Many species have developed specific adaptation traits to cope with fire, ensuring resistance and resilience. Due to the recent changes in socio-economy and land uses, fires are more and more frequent in the urban-rural fringe and in the coastlines, both now densely populated. The detection of fires and the monitoring of vegetation regrowth is thus of primary interest for local management and for understanding the ecosystem dynamics and processes, also in the light of the recurrent droughts induced by climate change. Among the main objectives of the COSMO-SkyMed radar constellation mission there is the monitoring of environmental hazards; the very high revisiting time of this mission is optimal for post-hazard response activities. However, very few studies exploited such data for fire and vegetation monitoring. In this research, Cosmo-SkyMed is used in a Mediterranean protected area covered by maquis to detect the burnt area extension and to conduct a mid-term assessment of vegetation regrowth. The positive results obtained in this research highlight the importance of the very high-resolution continuous acquisitions and the multi-polarization information provided by COSMO-SkyMed for monitoring fire impacts on vegetation.
Keywords
Introduction
The Mediterranean maquis is mostly characterized by evergreen woody shrubs (0.5-5 m tall) with sclerophyllous leaves; often isolated trees or small trees patches are present, as well as gaps with bare soil and rocks. Differences in aspect, slope and soil depth can generate large variation in species presence, vegetation cover and height. In the Mediterranean climate, rainfalls are mainly concentrated in fall and winter. It is during the very dry summer that most of the fire events take place, some of which can be extremely severe and very dangerous also for the neighboring population. The Mediterranean maquis co-evolved with disturbance caused by human activities, including fire which can promote regeneration, and after which rapid regrowth (~5-10 years) usually take place ([33], [37]). However, strong anthropic pressure and fragmentation of the landscape, together with increased temperatures and prolonged droughts as a result of climate change, have increased fire risk and events severity ([34]). Thus any destructive event, such as natural or human-induced fire, needs to be carefully monitored for the caused damages and the recovery response ([10]).
Remote sensing has a fundamental role in the provision of fire-related information and different optical and microwave sensors are used in hazard monitoring, with a key factor being the satellite revisiting time to minimize the time lag between pre- and post-event data and to conduct efficient follow up ([20], [21]). For vegetation monitoring, usually image acquisition is conducted during a pre-fire phase to characterize the area (e.g., classification of vegetation types, or fuel load prediction), a short-term post-fire phase to record the extent and severity of fire, and a mid/long-term post-fire phase to monitor the regeneration of vegetation ([16]).
Post-fire assessments have been conducted prevalently using optical data; however some studies also report the use of Synthetic Aperture Radar (SAR) data, or combination of optical and SAR ([4], [5], [13], [15], [25], [27], [30], [31], [39], [40], [41], [43], [46]). Possibly, the wider use of optical data is related to their broader diffusion in previous decades and their easier processing with respect to SAR, though in recent years both data types are equally available and supported by image processing software. The larger use of optical data also allowed to establish strong relationships, such as the linear relation between the Composite Burnt Index ([23]), designed to ecologically define burn severity, and optical-derived indices such as the differential Normalized Burn Ratio (ΔNBR - [22]).
Mid/long-term assessments to monitor vegetation regrowth have been extensively reviewed by Gitas et al. ([16]), who concluded that medium resolution optical satellites have been mostly used, due to the need of time-series. For regrowth monitoring, the use of a vegetation index derived from optical data has been proposed by Bastos et al. ([3]). Using higher resolution satellite optical data in synergy (Landsat and WorldView-2), Chu et al. ([8]) were able to detect post-fire regeneration in a larch forest, developing a new Forest Recovery Index. Similarly, Arnett et al. ([1]) showed that RapidEye optical image was able to differentiate various level of canopy damage due to fire better than Landsat, thanks to its higher spatial resolution. However, cloud-cover presence often hampers the possibility to collect dense time series optical images over forested regions.
Although SAR data are known to have different potential for ecological applications ([22]), its use in fire monitoring is less frequent. These data can be used to estimate not only the burnt severity but also the fuel load ([35]). In the case of fire monitoring, SAR insensitiveness to cloud cover and haze is definitively an advantage with respect to optical data, which often are unavailable due to smoke and cloud presence during and after fire occurrence. Changes of the vegetation volumetric structure can be also detected by SAR, thanks to the sensitivity of microwaves to woody structure presence and water content. SAR time-series have been used by Minchella et al. ([29]) and Tanase et al. ([42]) for monitoring the vegetation recovery in Mediterranean and boreal ecosystems. While different frequencies can be used according to vegetation type and size of branches, the incidence angle is an important parameter to take into account, as steeper angles increase the contribution from soil while at large angles the effect of volumetric scattering is dominant ([2]). In all cases, to avoid seasonal effects in time-series analysis of vegetation regrowth patterns, the use of unburned control points in proximity of the burnt area is suggested ([12]).
COSMO-SkyMed (CSK) is a constellation composed of four satellites equipped with Synthetic Aperture Radar operating at X-band. The first satellite has been launched by the Italian Space Agency (ASI) on June 2007, and the full constellation is operational since mid-2010. CSK is designed to provide products/services for environmental monitoring and surveillance and for military purposes. CSK has the capability of acquiring a large amount of daily images; it has all weather and day/night acquisition capabilities, and very short interval between the acceptance of the user request and the release of the remote sensing product ([11]). CSK has high spatial and radiometric resolution, with different acquisition modes, including CSK Stripmap products, that are the base of the Map Italy archive, and provide very frequent imagery over the whole Italian territory, with optimal potential for hazard events monitoring.
This research explores for the first time the use of CSK data for post-fire monitoring of Mediterranean vegetation. Specifically, the Map Italy archive has been used for the short-term assessment, while a specific acquisition campaign for mid-term assessment has been realized over the study site, a protected areas located in coastal Sardinia, Italy, covered by a large fire in 2013. The study is based on the hypothesis that CSK data can efficiently map burnt area and monitor the regrowth of maquis vegetation at least at an initial phase of recovery, thanks to the exploitation of dense time-series, of different polarizations, and to the acquisition of data at different incidence angles. To summarize, the objective of the present research is to test the suitability of CSK SAR data for vegetation burnt areas detection and regrowth monitoring, comparing the results with those obtained with commonly used optical freely available data.
Methods
The study includes a short-term and a mid-term assessment phase. The short-term assessment is based on the use of two CSK Map Italy SAR images, collected soon before and after the fire event, in order to map the burnt area extent. SAR results were compared in accuracy with those obtained using Landsat 8 optical data, and both were validated using the fire perimeter data collected by GPS in the field by the Sardinian Forest Service. The mid-term assessment was based on the use of long CSK time series to monitor the amount of vegetation regrowth after the fire damage. The results were evaluated using vegetation field data collected in specific field surveys.
Study area and ground data
The Capo Figari study area (Fig. 1) is a Site of Community Importance (SIC) listed in the European Commission Habitats Directive; this peninsula is located in northeastern Sardinia region (40° 59′ N, 09° 38′ E) and on June 25th 2013 was impacted by a large fire (>100 ha), according to the classification of the European Forest Fire Information System ([36]). The area is characterized by heterogeneous morphology; elevation ranges between 0 and 340 m, with slopes up to 80°. The climate in the region is Mediterranean, with monthly average precipitation in the range 2-41 mm and monthly average temperatures in the range 10-25 °C. The dominant vegetation is composed by Mediterranean shrubs, adapted to the very dry summer months, such as Erica arborea, Ginestra spp., Euphorbia dendroides, Pistacia lentiscus, and Juniperus spp.; Cistus spp. is abundant as a result of regeneration from past fire events. The extent of the burnt area was mapped by the Sardinian Forest Service (CFVA) on October 4th 2013, by means of a Global Positioning System (GPS) field survey: the fire affected 195.22 ha, of which 190 ha composed by Mediterranean maquis and 5.22 by uncultivated areas. Considering that in this coastal area slope and aspect have a strong effect on the vegetation type and development, the study site was stratified in sub-areas according to ancillary information, including: Corine Land Cover 2006 (CLC2006), a 2006 Ikonos very high resolution optical image, 1 m spatial resolution 2006 orthophotos, and a 10 m spatial resolution Digital Elevation Model (DEM).
Fig. 1 - Upper left: Sardinia island in Italy (in the red circle). Lower left: the location of the study area in Sardinia (in the red oval). Larger image: the Capo Figari study area.
On the 4th-5thApril and 16thJuly 2014 two field campaigns were conducted to collect maquis parameters. Ten transect of 15 m each were randomly located in each of the maquis sub-areas previously identified. Vegetation cover data was collected using the point intercept method at every 0.50 m, where a pole was used to identify the height and species of any plant hitting the pole. Percentage cover was calculated as the number of hits for each species or ground cover class divided by the total number of points per transect. Plant height was also recorded by measuring the vertical distance from the soil surface to the top of the branches.
Remote sensing data and analyses
SAR data for short-term assessment are two scenes from CSK Map Italy archive: a pre-fire scene acquired on 18th of June 2013 and a post-fire one dated 4th of July 2013. Single Look Slant Range scenes were acquired in Hi-Image mode, with 3 meters ground resolution, HH single polarization and 24° incidence angle. Intensity images were co-registered, calibrated and orthorectified using a 10 m DEM, with final spatial resolution kept equal to 3 m. Backscattering coefficient was normalized following Ulander ([45]) and a refined Lee filter with 9 × 9 pixel window was used for speckle reduction. A normalised backscattering change index (BCI) was computed as the normalized difference in gamma naught between post-event (γ0post) and pre-event (γ0pre) scenes (eqn. 1):
Negative BCI values were excluded from the analysis, considering that at HH polarization and 24° incidence angle an increase in backscattering is caused by the increased contribution of soil after fire event. A threshold was arbitrary selected to distinguish burnt from non-burnt areas; a morphological filter based on a 5 × 5 kernel was used to improve burnt area delineation in SAR images.
Optical data for short-term assessment are two Landsat 8 L1T images, acquired on 23rd of June and 2nd of July. Images were atmospherically corrected using the ATCOR2 package ([32]). The normalized burnt ratio index (NBR) was computed for each image as the normalized difference between the reflectance at 2.2 µm (Short Wave Infra-Red - SWIR) and 0.86 µm (Near Infra-Red, NIR - eqn. 2):
The ΔNBR, also considered as indicator of fire severity according to Cocke et al. ([9]), was calculated as the difference between the index values in pre- and post-event images. The averaged ΔNBR value for the study site was used as threshold to delineate burnt area in optical imagery.
For the mid-term assessment, considering the steep slopes in Capo Figari, CSK acquisitions were planned at incidence angles included in the 40°- 50° range, in order to reduce layover and shadowing distortion effects, and realized between December 2013 and August 2014. CSK constellation can provide an average of 6 scenes in a 16-day orbital cycle, with 3 ascending and 3 descending acquisitions. StripMap Single Look Complex Ping-Pong HH-HV scenes, at 15 meters ground resolution, were acquired at 43° in descending pass (43D, 47 total scenes) and 49° in ascending pass (49A, 39 total scenes).
Intensity images were co-registered, calibrated and orthorectified using a 10 m DEM. Backscattering coefficient was normalized following Ulander ([45]) and a refined Lee filter with 5 × 5 pixel window was used for speckle reduction. The combination of ascending and descending acquisitions allowed the exploitation of multi-angle views, as the terrain slope modifies the actual local incidence angle (LIA).
Backscattering values at HH, HV and HV/ HH polarization ratio were analyzed as function of 5° increasing LIA. Values around each 5° step were grouped and averaged, following the approach proposed by Tanase et al. ([40]).
Linear trends in backscattering time-series at each 5° step have been computed for each strata. The slopes obtained from linear time-series trends were used to derive a regression model for vegetation parameters prediction.
Results
In Capo Figari few areas are characterized by very steep zones (> 35°), where bare soil and rocks are dominant with almost absence of vegetation; thus areas having a slope > 35° were excluded from the analysis. Three slopes classes were considered: No_Slope (< 10°), Low_Slope (10°-20°), Medium_Slope (20°-35°). Three main vegetation types were present according to CLC2006: Mediterranean Maquis (M), Gariga (GA), and Sparse Vegetation (AR). The Mediterranean Maquis type was subdivided by visual interpretation of orthophotos and Ikonos imagery in Dense Maquis (MD), Intermediate Maquis (MI) and Sparse Maquis (MS). The Sparse Vegetation type was further subdivided according to aspect into: eastward Sparse Vegetation (AR1) and westward Sparse Vegetation (AR2). An area not affected by fire was finally delineated and used as control: Unburnt Maquis (UM). Thus, the stratification of the study area based on interpretation of ancillary information resulted in the identification of 7 homogeneous sub-areas, as summarized in Tab. 1. The three maquis classes (MD, MI, MS) were sampled with 10 transects per each maquis type in April and 7 transects in July field campaigns.
Tab. 1 - Homogeneous sub-areas characteristics.
Class | Vegetation type |
Vegetation density |
Aspect | Slope |
---|---|---|---|---|
MD | Mediterranean Maquis | Dense | North | Low/Medium |
MI | Mediterranean Maquis | Intermediate | West | Medium |
MS | Mediterranean Maquis | Sparse | South/East | Flat/Low |
GA | Gariga | Intermediate | East | Medium |
AR1 | Sparse Vegetation | Sparse | West | Medium |
AR2 | Sparse Vegetation | Sparse | East | Medium |
UM | Mediterranean Maquis | Intermediate/Dense | West | Low/Medium |
Ground data analysis indicate that the percentage of vegetation cover did not show any significant increase between April and July (t-test, p > 0.05), during which regrowth started. MD and MS sub-areas showed similar composition, with high vegetation (50-60%) and low bare soil (11-15%) values, while MI sub-area showed higher bare soil (39-46%) coverage. Vegetation height significantly increased in all the sub-area (t-test, p < 0.05): in MD the average increase from April to July resulted equal to 75 % (from 17.54 to 31.44 cm), in MI equal to 70 % (from 15.44 to 26.81 cm), in MS equal to 98% (from 17.25 to 35.05 cm).
Short-term assessment
For the short-term assessment, the burnt areas identified by means of a threshold applied to BCI derived from CSK scene and the averaged ΔNBR derived from Landsat 8 images were compared to the area detected by the GPS survey of the Sardinian Forest Service (Fig. 2). Results showed that optical and SAR correctly identified the 78% of the ground delineated burnt area (169 ha), while Landsat 8 correctly identified the 77% (176 ha). Commission error from SAR-derived burnt area resulted slightly higher with respect to the optical derived (21 ha or 10% for SAR; 14 ha or 6% for optical), while omission error was larger from optical-derived burnt area than the SAR-derived one (27 ha or 12% for SAR; 38 ha or 17% for optical).
Fig. 2 - Comparison of burnt areas detected by GPS survey (red), SAR data (orange), and Landsat 8 (green).
Pre- and post-event backscattering, ΔNBR and BCI values were explored at 5° LIA steps. The results show that backscattering variation is dependent on LIA. At angles < 20° and > 40° the difference in backscattering is higher than 2 dB. The Pearson correlation coefficient between ΔNBR and BCI varies between 0.31 and 0.62, with higher values between 15° and 25° angles, and overall averaged correlation coefficient equal to 0.39 (Tab. 2).
Tab. 2 - Backscattering, BCI, ΔNBR and correlation values at increasing 5° LIA steps.
Parameter | 10° | 15° | 20° | 25° | 30° | 35° | 40° | 45° | 50° |
---|---|---|---|---|---|---|---|---|---|
γ0pre (dB) | -8 | -7.46 | -8.67 | -9.83 | -10.36 | -10.44 | -9.82 | -9.68 | -9.95 |
γ0 post (dB) | -5.65 | -4.98 | -6.57 | -8.05 | -9.21 | -8.9 | -7.96 | -7.78 | -8.13 |
BCI | 0.27 | 0.25 | 0.23 | 0.19 | 0.12 | 0.17 | 0.21 | 0.22 | 0.21 |
ΔNBR | 0.6 | 0.62 | 0.64 | 0.55 | 0.6 | 0.61 | 0.53 | 0.57 | 0.57 |
Pearson r | 0.31 | 0.47 | 0.62 | 0.47 | 0.34 | 0.38 | 0.34 | 0.38 | 0.43 |
The backscattering, BCI, ΔNBR, correlation and LIA values were extracted and averaged for each of the sub-areas previously identified (Tab. 3). AR1 and AR2 areas show higher backscattering values in both scenes, while the Maquis vegetated areas the lowest. The BCI shows a very low values in AR1 and AR2, which is thus not effectively recognized as burnt.
Tab. 3 - Backscattering, BCI, ΔNBR and correlation values per sub-area.
Parameter | AR1 | AR2 | GA | MD | MI | MS |
---|---|---|---|---|---|---|
γ0pre (dB) | -5.1 | -5.42 | -8.87 | -11.95 | -10.49 | -11.7 |
γ0 post (dB) | -4.38 | -3.68 | -5.71 | -9.82 | -8.39 | -10 |
LIA | 32.4 | 15.42 | 18.23 | 31.6 | 42 | 24.8 |
BCI | 0.08 | 0.19 | 0.29 | 0.22 | 0.23 | 0.18 |
ΔNBR | 0.41 | 0.49 | 0.74 | 0.74 | 0.53 | 0.77 |
Pearson r | 0.08 | 0.41 | 0.34 | 0.55 | 0.32 | 0.33 |
Mid-term assessment
For mid-term assessment, the three maquis sub-areas and the UM control area for which ground truth was available were considered. The regrowth dynamic was assessed at different 5° LIA steps, but only steps for which at least 10% of the pixels were available were used in the analysis. This resulted in having the 40°-60° range for MD, 25°-40° and 55°-60° for MI, 40°-55° for MS, and 30°-60° for UM. The values for time series for each sub-area are plotted in Fig. 3 for HH, HV, and HV/HH ratio, after applying a 3-acquisition moving average, with corresponding values for HV/HH ratio shown in Tab. 4.
Fig. 3 - Time-Series plots for HH, HV and HV/HH per sub-area. (orange): UM (unburnt control); (red): MD (Dense Maquis); (green): MI (Intermediate Maquis); (blue): MS (Sparse Maquis).
Tab. 4 - Backscattering HV/HH values per sub-area and LIA 5° steps. When pixel number did not reach the 10% of those of the area, the values was not considered (indicated with “n/a”). (UM): unburnt control; (MD); Dense Maquis; (MI): Intermediate Maquis; (MS): Sparse Maquis.
HV/HH [dB] | 30° | 35° | 40° | 45° | 50° | 55° | 60° |
---|---|---|---|---|---|---|---|
MD | n/a | n/a | -8.31 | -8.58 | -7.10 | -7.23 | -7.48 |
MI | -9.40 | -8.71 | -8.28 | n/a | n/a | -7.17 | -7.51 |
MS | n/a | n/a | -8.52 | -8.13 | -8.14 | -7.62 | n/a |
UM | -6.79 | -6.57 | -6.52 | n/a | -6.10 | -5.79 | -5.98 |
Linear trend were also computed to help the identification of best angles and polarizations for regrowth assessment, but only at 40° and 55° information is available for all the sub-areas. The HV/HH ratio shows an increasing trend at different LIA steps in all sub-areas, with higher values for the UM case. Mean slopes for HV/HH were equal to 0.85 for MD, 0.72 for MI, 0.84 for MS, and 0.28 for UM sub-area, with marked differences between burnt and control areas.
With limited ground truth, a preliminary test to evaluate the possibility of using slope values for prediction of vegetation regrowth was done, under the hypothesis that a change in vegetation height can influence the HV/HH ratio at all the angles. The HV/HH slopes at all incidence angles were averaged and this increase was applied to the April vegetation height measurement; results were compared with actual field data from July. The predicted height for MD in July was equal to 33.2 cm, while the observed height to 31.4 cm; for MI the predicted value was 27.2 cm, while the observed one 26.8 cm; for MS the predicted height was 32.5 cm and the observed 35.0 cm. Overall, the differences resulted always below 8% of the observed values.
Finally, 10 scenes were selected from dates close to those of the July field measures, and the average slope values for 40° and 55° LIA for the three maquis sub-areas were computed. The results are illustrated in Fig. 4 in comparison with the observed field values. This HV/HH ratio increase was also calculated for the whole study area to map the vegetation regrowth and is shown in Fig. 5, in which the burnt area according to GPS field measures is overlapped; the average change rate for the HV/HH backscattering was +0.8% day-1 within the burnt area, while it resulted +0.2% day-1 outside the burnt area.
Fig. 4 - Averaged slope values calculated for 40_ and 55_ LIA in proximity of the field measurements. Bright bars represent HV/HH-derived slopes, dark bars represent the measured increases. (MD): Dense Maquis; (MI): Intermediate Maquis; (MS): Sparse Maquis.
Fig. 5 - HV/HH growth rates calculated over Capo Figari area. In red the burnt area perimeter according to field GPS measures.
Discussion and conclusions
Using CSK pre- and post-fire scenes, the burnt area has been accurately delineated. Results indicate that about 78% of the area defined as burnt by the CFVA ground survey has been correctly identified based on CSK data, with a result very similar to that obtained using Landsat 8; comparing the two data sources, CSK shows a larger commission error while Landsat 8 a larger omission error. This result is higher with respect to that from a previous comparison of SAR and optical data for forest fire mapping in the Mediterranean area, which showed that SAR correctly mapped 78% of the area identified by optical data as burnt ([4]). The two sensors detect different features: while ΔNBR is primarily sensitive to vegetation chlorophyll content ([28]), BCI mostly responds to volumetric changes in the target. However, both responses are also influenced by water content in soil and vegetation. Kurum ([24]) suggested that a SAR approach that combines pre- and postfire backscattering could be used to quantify the fire impacts, if the effects induced by geometry, weather, and other human activities are accounted for in the analysis. Tanase et al. ([44]) conducted a comparison of fire severity as detected by Landsat or L-band SAR data for seven sites, concluding that the obtained accuracy is higher with optical data for high biomass forests, but comparable for low-biomass forests.
Different researches highlighted the usefulness of optical and SAR data for burnt area detection, also used in conjunction in the Mediterranean region ([38]). Although optical data have been more frequently used, recent studies have been also conducted with different SAR data types, as shown by the Gitas et al. ([16]) review. As an example, free Sentinel 1 data have been used to detect burnt areas and estimate related carbon emissions at country level in Indonesia ([26]). At local level, in South Africa, RADARSAT-2 and Sentinel 1 C-band SAR polarimetric data showed the capability to detect burnt areas, with results similar to those obtained with Sentinel 2 data ([14]). Our results show that also CSK, which according to our knowledge has not previously tested for monitoring of burnt areas and regrowth, can be exploited for this purpose. SAR insensitiveness to clouds and haze represents an advantage with respect to optical data use, with the high temporal resolution of CSK constituting an especially relevant feature that allows rapid response in hazard emergency. The presented results are in line with the indications provided by Imperatore et al. ([18]), suggesting the use of SAR in Sardinia island for fire scar detection.
The varied topography in the study area allowed to study the SAR response at difference incidence angles, which is a useful information when data acquisition campaigns have to be planned. The results from both BCI and ΔNBR indices were slightly higher in areas characterized by lower incidence angles. In the two Sparse Vegetation sub-areas (AR1, AR2) the indices tended to show lower values, in accordance with the low vegetation density presence. The higher correlation between optical and SAR-based indices was obtained in the Dense Maquis sub-area, indicating a similar response to vegetation foliar changes.
Exploring the different polarizations of CSK data revealed that the polarization ratio is more sensitive to changes in vegetation volume, due to volumetric scattering contribution to the signal, with evident differences between burnt and unburnt maquis. It was recognized that X-band shows a smaller dynamic range in burnt severity compared to longer wavelengths, mainly due to the highest sensitivity to soil roughness and penetration capability ([39], [42]). Usually, when spatial filtering is applied, the effect of incoherent scattering coming from highly rough surfaces can be reduced, but at the expenses of spatial resolution which has to be degraded. In this research the availability of a dense time-series allowed to perform a temporal data processing, limiting the need for filtering and preserving the high spatial resolution which is needed to meet the legislative requirements for burnt area detection ([10]).
The very preliminary results obtained by monitoring the vegetation regrowth indicate that CSK can be useful for this purpose, though additional data are needed to support this initial evidence. The monitoring of vegetation regrowth can be important for post-fire planning interventions, especially in regions affected by repeated drought events. It is expected that combining two different frequencies, e.g., CSK and the freely available Sentinel data, can further support vegetation monitoring even at more advanced stage of regrowth, and at fine scales, as previously shown by Holecz et al. ([17]). Forest regeneration it is important for carbon cycling, but its monitoring is considered difficult, with previous efforts primarily based on optical data ([6]). Recently, L-band SAR data have been successfully employed to monitor regeneration under different restoration treatment in China ([7]). The use of SAR data for monitoring vegetation regrowth is also supported by the indication that the optical signature from burnt area deteriorates rapidly, resulting in fire-disturbed sites begin poorly distinguishable from unburned sites by the end of the post-fire season ([19]). Overall, considering the positive results obtained also in comparison with optical data, and the broad image availability in all-weather conditions, the present study promotes a wider adoption of SAR data for vegetation fire monitoring, at least for short and mid-term assessment stages.
Acknowledgements
We thank the Sardinian Forest Service (Corpo Forestale e di Vigilanza Ambientale - Servizio territoriale dell’Ispettorato dipartimentale di Tempio) for the valuable information and assistance provided during our field visits and sampling.
GVL and DP thank the EU for supporting the BACI project funded by the EU’s Horizon 2020 Research and Innovation Program under grant agreement 640176.
References
CrossRef | Gscholar
Online | Gscholar
Gscholar
CrossRef | Gscholar
Gscholar
Gscholar
CrossRef | Gscholar
Authors’ Info
Authors’ Affiliation
Dario Papale
Department for Innovation in Biological, Agro-Food and Forest Systems (DIBAF), University of Tuscia, I-01100 Viterbo (Italy)
Valentina Bacciu
Fondazione CMCC (Euro-Mediterranean Center on Climate Change), Division on Impacts on Agriculture, Forests and Ecosystem Services, I-07100 Sassari and I-01100 Viterbo (Italy)
Fabio Del Frate
Department of Civil Engineering and Computer Sciences (DICII), University of Rome Tor Vergata, I-00100 Rome (Italy)
Italian Space Agency, I-00100 Rome (Italy)
Corresponding author
Paper Info
Citation
Vaglio Laurin G, Avezzano R, Bacciu V, Frate FD, Papale D, Virelli M (2018). COSMO-SkyMed potential to detect and monitor Mediterranean maquis fires and regrowth: a pilot study in Capo Figari, Sardinia, Italy. iForest 11: 389-395. - doi: 10.3832/ifor2623-011
Academic Editor
Davide Ascoli
Paper history
Received: Sep 03, 2017
Accepted: Mar 05, 2018
First online: May 15, 2018
Publication Date: Jun 30, 2018
Publication Time: 2.37 months
Copyright Information
© SISEF - The Italian Society of Silviculture and Forest Ecology 2018
Open Access
This article is distributed under the terms of the Creative Commons Attribution-Non Commercial 4.0 International (https://creativecommons.org/licenses/by-nc/4.0/), which permits unrestricted use, distribution, and reproduction in any medium, provided you give appropriate credit to the original author(s) and the source, provide a link to the Creative Commons license, and indicate if changes were made.
Web Metrics
Breakdown by View Type
Article Usage
Total Article Views: 38232
(from publication date up to now)
Breakdown by View Type
HTML Page Views: 33117
Abstract Page Views: 2068
PDF Downloads: 2319
Citation/Reference Downloads: 13
XML Downloads: 715
Web Metrics
Days since publication: 2180
Overall contacts: 38232
Avg. contacts per week: 122.76
Article Citations
Article citations are based on data periodically collected from the Clarivate Web of Science web site
(last update: Feb 2023)
Total number of cites (since 2018): 3
Average cites per year: 0.50
Publication Metrics
by Dimensions ©
Articles citing this article
List of the papers citing this article based on CrossRef Cited-by.
Related Contents
iForest Similar Articles
Review Papers
Remote sensing-supported vegetation parameters for regional climate models: a brief review
vol. 3, pp. 98-101 (online: 15 July 2010)
Review Papers
Remote sensing support for post fire forest management
vol. 1, pp. 6-12 (online: 28 February 2008)
Review Papers
Accuracy of determining specific parameters of the urban forest using remote sensing
vol. 12, pp. 498-510 (online: 02 December 2019)
Research Articles
Assessing the performance of MODIS and VIIRS active fire products in the monitoring of wildfires: a case study in Turkey
vol. 15, pp. 85-94 (online: 19 March 2022)
Review Papers
Remote sensing of selective logging in tropical forests: current state and future directions
vol. 13, pp. 286-300 (online: 10 July 2020)
Technical Reports
Detecting tree water deficit by very low altitude remote sensing
vol. 10, pp. 215-219 (online: 11 February 2017)
Research Articles
Evaluation of hydrological and erosive effects at the basin scale in relation to the severity of forest fires
vol. 12, pp. 427-434 (online: 01 September 2019)
Research Articles
Remote sensing of Japanese beech forest decline using an improved Temperature Vegetation Dryness Index (iTVDI)
vol. 4, pp. 195-199 (online: 03 November 2011)
Research Articles
Assessing water quality by remote sensing in small lakes: the case study of Monticchio lakes in southern Italy
vol. 2, pp. 154-161 (online: 30 July 2009)
Technical Reports
Remote sensing of american maple in alluvial forests: a case study in an island complex of the Loire valley (France)
vol. 13, pp. 409-416 (online: 16 September 2020)
iForest Database Search
Search By Author
Search By Keyword
Google Scholar Search
Citing Articles
Search By Author
Search By Keywords
PubMed Search
Search By Author
Search By Keyword