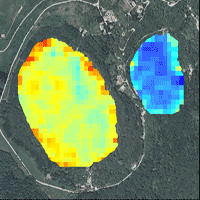
Assessing water quality by remote sensing in small lakes: the case study of Monticchio lakes in southern Italy
iForest - Biogeosciences and Forestry, Volume 2, Issue 4, Pages 154-161 (2009)
doi: https://doi.org/10.3832/ifor0507-002
Published: Jul 30, 2009 - Copyright © 2009 SISEF
Research Articles
Abstract
In order to investigate bio-physical parameters associated with water quality, a model based on calibrated and atmospherically corrected Remotely Sensed data has been implemented. Secchi Disk depth and chlorophyll concentration parameters were estimated in a system of very small lakes at Monticchio (Italy) using Landsat TM data. The model was developed through the use of stepwise multiple regression and gave high coefficients of determination (R2 = 0.82 for Secchi Disk and R2 = 0.72 for chlorophyll). Values for water transparency were strongly correlated with chlorophyll a concentration: a linear relationship between the two parameters showed a high coefficient of determination (R2 = 0.93). The case study shows that the application of this approach on lakes with a small surface area, such as the Monticchio lakes in Southern Italy, is effective and the developed model well describes the water quality parameters.
Keywords
Water quality, Landsat, chlorophyll, Secchi Disk transparency, Remote Sensing, Monticchio Lakes
Introduction
Assessing the quality of surface water in lakes, rivers and reservoirs is a key issue for environmental monitoring and management. In Europe, lakes are classified according to eco-regions ([6]), ranging from alpine to boreal, from continental to sub-arctic. Each class is based on morphological, vegetational and climatic conditions, which influence the water quality parameters such as chlorophyll concentration, turbidity, suspended sediments, and thermal characteristics. Furthermore, lakes are often subjected to sudden environmental changes caused by anthropic activities due to their multiple uses (recreational, industrial, agricultural, water supply, etc.). In order to assess lake water quality, in situ traditional methods are very accurate but have the disadvantage of being invasive, expensive and labour-intensive. In situ methods, therefore, may undersample the spatial variability required for the proper monitoring and management of lake water quality. Remote sensing provides an efficient method to enhance the in situ limnological measurements, allowing the extension of the recorded parameters to the entire lake surface.
Remotely sensed data from satellites have been used for the monitoring of water quality ([32], [47], [48]) since the early ’80s. The Thematic Mapper sensor installed on the Landsat satellite has been the most widely used sensor to monitor internal waters ([45], [40], [30], [29], [54]). Thanks to its good spectral characteristics and its finer spatial resolution (30 m pixels), which allowed even small lakes to be investigated, it was particularly popular in Europe ([26], [2], [14], [58], [56], [5], [6]). Sea and ocean water monitoring also increasingly uses data from remote sensors (i.e., Aqua-MODIS, Envisat-AATSR - [49], [22], [9]), which guarantee repetitiveness, improving the detection of limnological parameter changes. But for lake areas with a small extension, where water monitoring is a crucial issue, the coarse resolution of such kind of sensors is not applicable.
Remote sensing water quality assessment, is usually investigated using the optical bands in the region from blue to near infrared. These data are then used to explore the relation between the reflectance of water bodies and biophysical parameters such as: transparency, chlorophyll concentration (phytoplankton), and the organic and mineral suspended sediments.
The first approach in the lake water quality assessment has been the analysis of chromatic coordinates in the visible part of the electromagnetic spectrum. This approach is based on the idea that an increase of radiance or reflectance at long wavelengths is an indicator of a decrease of spectral response, whereas a reduction of the spectral response towards the shorter wavelengths (blue) is related to an increase in chlorophyll or suspended solid sediments. Although this methodology has been applied by several studies ([7], [38], [26], [57]), the results at high chlorophyll and/or suspended solid sediments concentrations are ambiguous ([21]). Many studies focus on chlorophyll concentration ([45], [14], [19], [5], [6]), Bukata et al. ([8]) demonstrated that increasing chlorophyll concentration causes a decrease in the spectral response at short wavelengths, particularly in the blue band.
Several studies investigated the correlation between spectral response of internal waters with water transparency using single Landsat bands in the visible region ([20]), or some combination thereof ([30], [29]). Water transparency, measured as Secchi Disk (SD), is strongly correlated to the spectral response of Landsat bands ([37]), especially the blue and red ones ([31], [13]).
Common statistical techniques have been used to study the correlation between spectral data and limnological parameters such as chlorophyll concentration ([2], [40], [23], [5]), transparency ([32], [31], [14], [17], [20], [30], [29]) and suspended soil sediments ([45], [47], [54]).
The atmospheric path between the satellite and the water surface affects the remotely sensed reflectance: therefore data need to be adequately corrected before statistical analysis can be carried out ([28]). In quantitative studies the interactions of incident energy with the atmosphere are large enough to significantly affect the incoming radiance, which may compromise the results ([50]). This is particularly important for surfaces like water, where the reflected light fraction is very low: being around 1-10% of the sensor-measured radiance ([24]). As a consequence, the sensor-recorded radiance of the lake surface can be very low compared to the path radiance. Atmospheric correction is also required in cases where multitemporal analysis is used to assess water quality change. Several techniques have been proposed for the atmospheric correction of the remote sensed data ([27], [10], [44], [18], [12], [52]). Many of them are variants of DOS (Dark Object Subtraction) method; more sophisticated techniques (Radiative Transfer Code) usually require in situ measurements of the atmospheric characteristics during the satellite overpass and therefore are more expensive and, above all, not applicable on historical data.
The aim of this study is to assess the reliability of a model based on calibrated and atmospherically corrected remotely sensed data on two adjacent and small volcanic lakes (Lago Grande and Lago Piccolo) in Basilicata Region (Southern Italy) through the analysis of the relationships between Landsat TM data and chlorophyll concentration and water transparency, gathered in a previous study ([15]). Among the Italian volcanic lakes the Monticchio lakes, and in particular the Lago Grande, are characterised by a high concentration of phosphorus and nitrogen on the bottom and on the surface as well ([41]). The raising interest in the study of the eutrophication processes and, in particular in the strategies to reduce them, is also confirmed by a recent study ([34]).
In the present paper the main innovation is represented by the implementation of the proposed Landsat TM based methodology on very small lakes, while so far it has been applied only for very large lakes. In fact, the use of Landsat TM dataset available since the 70s, even if characterised by a coarse resolution, allows a multitemporal analysis of the internal water quality.
The proposed approach leads also to the implementation of a different functional model ([46]) based on the relation of the two Landsat bands TM1 and TM2, which represents the minimum and maximum reflectance of the chlorophyll a in internal waters ([25], [53]).
Materials and Methods
Study area
The Monticchio lakes system consists of two volcanic basins located in the Vulture mountain range in Basilicata, south Italy (40° 55’ 48.29” N; 15° 36’ 16.61” E). The catchment area is about 400 ha and ranges between 1262 m and 653 m a.s.l., that represents the inlet of the Lago Grande effluent. The Monticchio lakes system within the whole area of Vulture mountain range belong to the Natura 2000 network of protected areas (European Birds Directive 79/409/CEE and European Habitats Directive 92/43/ CEE).
The two lake basins, with different and relatively considerable depths (Fig. 1) originated from two different volcanic explosions, and are divided by a narrow isthmus and communicate through an interlake canal. The two lakes are fed by both telluric and meteoric waters; Lago Piccolo gets aquifer water directly from the bottom, while Lago Grande gets the excess water from Lago Piccolo through the interlake canal. The Lago Grande outflow leads to the Ofanto River. The morphometric characteristics of the two lakes ([15]) are reported in Tab. 1.
Fig. 1 - Study area (40° 55’ 48.29” N; 15° 36’ 16.61” E) with sampling points of Secchi Disk (triangles) and chlorophyll a concentration (circles). Redrawn from [15].
Tab. 1 - Morphometric characteristics of the Monticchio lakes.
Parameters | Lago Grande | Lago Piccolo |
---|---|---|
Catchment basin size (ha) | 240 | 107 |
Volume (m 3 x 1000) | 3 270 | 2 460 |
Height (m) | 653.7 | 656 |
Surface (ha) | 41.3 | 13.9 |
Maximum depth (m) | 36 | 38 |
Mean depth (m) | 8.9 | 17.9 |
Interlake channel flow (l/s) | 10 |
Limnological data
The limnological data on water transparency and chlorophyll a content used in this study were obtained from a wider study ([15]), aimed at identifying the morphometrical, limnological and chemical-microbiological characteristics of the Monticchio lakes.
Referring to Dumontet et al. ([15]) sampling was carried out on May 15th 2001 for Lago Piccolo, and May 16th for Lago Grande. The following physico-chemical properties were measured: depth; temperature; transparency (Secchi Disk depth, at the resolution of 25 cm); water oxygen; nutrients; pH and chlorophyll a. Transparency was measured at 38 stations in Lago Grande and 22 in Lago Piccolo while chlorophyll a was measured at 22 and 23 stations respectively (Fig. 1).
Among these sampling points, the closest measurements to the coastline were excluded to avoid comparison with Landsat pixels with an ambiguous spectral response due to contamination by any vegetation around the lakes occurring in the pixel footprint. Moreover, given the close proximities of some stations, some sampling points fell into the same pixel. In such cases an arithmetic mean of the measurements was calculated. Finally, as regards the chlorophyll concentration measurements, samples collected near the bottom of the lake were excluded because of the influence of the depth on the spectral response. The statistical properties of the two limnological parameters are shown in Tab. 2.
Tab. 2 - Descriptive statistics of chlorophyll and transparency in the two lakes.
Indices | Transparency (Secchi Disk) (m) | Chlorophyll a (mg m -3) | ||
---|---|---|---|---|
Lago Grande | Lago Piccolo | Lago Grande | Lago Piccolo | |
Minimum | 0.25 | 3.00 | 4.63 | 1.11 |
Maximum | 1.00 | 3.75 | 11.35 | 4.57 |
Mean | 0.83 | 3.25 | 6.93 | 2.45 |
Range | 0.75 | 0.77 | 6.72 | 3.46 |
St. Dev. | 0.186 | 0.199 | 1.656 | 1.051 |
It is clear from the data that the conditions of the two lakes are significantly different: Lago Grande has a higher chlorophyll a concentrations and lower transparency than Lago Piccolo. Here, the transparency is measured by averaging the disappearance and reappearance values of the Secchi Disk. In terms of the parameters considered by the OECD (Organization for Economic Co-operation and Development) Lago Piccolo is meso-oligotrophic, while Lago Grande is meso-eutrophic.
Satellite data
The remotely sensed data were acquired by the TM sensor onboard Landsat 5. The scene (path 188, row 32) dates back to the 13/05/2001 (field data collection) and covers the whole Basilicata region. Weather conditions were stable between field data collection and satellite data, with no rain from 6 to 23 May, as reported from the Venosa meteorological station. The sensor’s spectral characteristics are such that TM data is amongst the most used to monitor water quality.
Pre-processing of the Landsat data consisted of radiometric calibration and atmospheric correction: necessary for quantitative studies ([24], [50]). This is especially the case for surfaces such as lake waters where the reflected light is small ([39]), since the radiance of these surfaces is less than 10% on average, and often lower than 1%, of the sensor measured total radiance ([6]).
Radiometric calibration consists of a series of equations used to convert the stored quantized energy signal (digital number: DN) of the TM data into radiance values at the sensor. Landsat images were converted into radiance values at the satellite by using the following equation (eqn. 1):
where Lsens is the radiance at satellite level of a specific band (W m-2 sr-1 μ-1); DN is the value of the digital number; G is the gain and B is the bias. The line intercept, described by the bias, takes into account the fact that even with a null input signal (Lsens = 0) the acquisition system can still give an electric output signal fundamental to the acquisition system. The gain and offset values for the single bands were obtained from the CCT header file (Tab. 3).
Tab. 3 - Gain and offset for Landsat TM5 bands.
Bands | 1 | 2 | 3 | 4 | 5 | 7 |
---|---|---|---|---|---|---|
Offset | -1.5 | -2.8 | -1.2 | -1.5 | -0.37 | -0.15 |
Gain | 0.6024 | 1.1749 | 0.8059 | 0.8145 | 0.1081 | 0.0570 |
Atmospheric correction is needed because electromagnetic radiation travels through the atmosphere along its two paths from the sun to the earth surface and from there to the sensor, undergoing alterations to the radiometric signal. For water, in particular, the sensor recorded radiometric signal is very weak compared to the atmosphere contribution ([4]). Thus, the aim is to change the sensor recorded radiance into reflectivity of the surface at the ground. The simplified model of the effects of the atmosphere is ([42] - eqn. 2):
where ρsup (λ) is the surface reflectivity; Latm↑(λ) is the atmosphere spectral radiance (path radiance) diffused upward as W m-2 sr-1 μm-1; Lsens(λ) is the sensor-measured spectral radiance; E0(λ) is the solar spectral irradiance as W m-2 μm-1; Eatm↓(λ) is the downward spectral irradiance incident on the surface due to the diffusion of solar radiation through the atmosphere as W m-2 μm-1; τ↑(λ) is the atmospheric transmittance along the sun-surface route; θz is the zenith angle of the sun.
The at-satellite radiance (Lsens) results from the radiometric calibration; E0(λ) is the extra-atmospheric solar constant [Esol = E0(λ) cosθz], where the zenith angle of the sun (θz) was calculated from the date, position and time of satellite overpass while θv (view angle) was assigned a value of 0°, which is considered an acceptable approximation for narrow FOV systems such as Landsat. The solution of the unknown terms is carried out by using several methods, which can be grouped in two categories: image based methods (used in this study) and radiative transfer models. The latter solve the equation on the base of the theory of the radiation transfer of electromagnetic energy interacting with the atmosphere but need in situ measurements of atmosphere optical properties at the time of the satellite overpass. The image-based method used in this study belongs to the Dark Object Subtraction (DOS) category where information on atmosphere properties is obtained directly from image analysis. Briefly, the unknown terms of the general equation of the atmosphere correction are: Latm↑(λ): path radiance; Eatm↓(λ): downward atmosphere irradiance; τ↑(λ): upward atmospheric transmittance; τ↓(λ): downward atmospheric transmittance.
In the method we use, spectral irradiance due to solar radiation in the atmosphere is considered null [Eatm↓(λ) = 0], while the path radiance [Latm↑(λ)] is obtained from the radiance measured by satellite of a pixel having no or very little reflectivity (dark object). In this study the dark object used was a pixel related to deep water off the Tyrrhenian coast ([11], [51]). This value, extracted for each Landsat band, is considered to be an additive contribution to total atmospheric radiance and thus subtracted from each band.
Downward atmospheric transmittance [τ↓(λ)] is approximated from the zenith angle of the sun ([12] - eqn. 3):
an approximation valid for wavelengths up to 1.1 μm, while for greater wavelengths (i.e., TM5 and TM7) it is considered to be 1.
The upward transmittance is calculated from the following equation (eqn. 4):
assuming there is only Rayleigh and not aerosol scattering. The Rayleigh optical thickness [δR(λ)] was calculated according to the equation (eqn. 5):
where λ is the wavelength in μm.
The corrected Landsat bands were subset to the area of interest and georeferred to the UTM-WGS84 system using 20 GCP before extracting the radiometric values of the limnological data at the sampling points. Average radiometric values were calculated for a 3x3 window centred on the sampling point ([16], [5]).
Results and discussion
Common statistical techniques were used in order to determine the relationship between electromagnetic energy and water quality parameters ([32], [40], [30], [29]). The functional models used to study the relation between reflectance values and limnological parameters, such as chlorophyll concentration and water transparency, include linear, exponential and logarithmic transformation models.
First, an analysis using Pearson’s correlation was carried out in order to detect the existence of significant relationships between Landsat bands and both transparency and chlorophyll concentration. Previous studies ([31], [33], [13], [20], [30]) found a very significant correlation between water transparency and the relationships among the visible bands, especially TM3/TM1. As regards to chlorophyll, some wavelengths are particularly important: 445 and 665 nm are the chlorophyll absorption bands, while 520 and 550 nm are points of minimum absorption ([1], [5]); thus, the Landsat visible bands are appropriate for detecting chlorophyll a concentration in lake water ([20], [5], [21]). The analyses carried out with Pearson’s correlation (Tab. 4) are in agreement with results from the literature: the visible bands (particularly TM1 and TM2) and especially some of their ratios (TM1/TM3, TM3/TM1, TM3/TM2) are significantly correlated with transparency and chlorophyll concentration.
Tab. 4 - Pearson’s R correlation between limnological data and Landsat bands. (**): significant correlation for p<0.05.
Bands | Transparency (SD) m | Chlorophyll a mg m -3 |
---|---|---|
TM1 | 0.56** | -0.59** |
TM2 | 0.15 | -0.32** |
TM3 | -0.28 | 0.09 |
TM4 | 0.11 | -0.21 |
TM5 | 0.21 | -0.28 |
TM7 | 0.21 | -0.29 |
TM1/TM2 | 0.20 | -0.06 |
TM2/TM1 | -0.14 | 0.04 |
TM1/TM3 | 0.71** | -0.54** |
TM3/TM1 | -0.67** | 0.47** |
TM3/TM2 | -0.81** | 0.68** |
As for the independent variables, all the visible bands and their ratios were included in the multiple regression. The procedure to select significant variables is the stepwise one, with p>0.10 as a limit for factor removal.
As for transparency, the variables selected by the statistical analysis were TM1 and the TM3/TM2, TM1/TM2 e TM2/TM1 ratios. Therefore, the functional model is (eqn. 6):
where SD is the Secchi Disk measure in m and TMi are the Landsat bands, with radiometric and atmospheric correction, as W m-2 sr-1 μm-1. In Tab. 5, the results of the regression analysis are shown.
Tab. 5 - Regression analysis between limnological parameters and Landsat bands: (A) Secchi Disk depth; (B) chlorophyll a.
(A) Dependent Variable |
SD (SD [m]; TMi [W m-2 sr-1 μm-1]) |
|
---|---|---|
Previsional Model | SD = a + bTM3/TM2 + cTM1/TM2 + dTM1 + eTM2/TM1 | |
Coefficients | a | 10.752 |
b | -5.467 | |
c | -3.783 | |
d | 401.21 | |
e | -5.581 | |
R2 | 0.82 | |
R2 change | TM3/TM2 | 0.66 |
TM1/TM2 | 0.72 | |
TM1 | 0.80 | |
TM2/TM1 | 0.82 | |
RMSE | 0.540 | |
NSE | 0.943 | |
IA | 0.985 | |
(B) Dependent Variable |
Chl-a (Chl-a [mg m-3]; TMi [W m-2 sr-1 μm-1]) |
|
Previsional Model | Chl-a = a + bTM3/TM2 + cTM1/TM2 + dTM2 + eTM2/TM1 | |
Coefficients | a | -47.515 |
b | 9.516 | |
c | 20.952 | |
d | - 873.0 | |
e | 34.889 | |
R2 | 0.72 | |
R2 change | TM3/TM2 | 0.46 |
TM1/TM2 | 0.49 | |
TM2 | 0.63 | |
TM2/TM1 | 0.72 | |
RMSE | 1.300 | |
NSE | 0.754 | |
IA | 0.925 |
Data analysis confirms the ability of radiometric values, as derived from the Landsat bands, to predict lake water transparency. Very high R2 values were found and were in agreement with similar studies ([20], [30], K[29]) and RMSE was within moderate values.
In order to assess model performance we used two indices: the Index of Agreement (IA - [55]) and the Nash and Sutcliffe Efficiency coefficient (NSE - [43]). The IA and NSE indices high values detected (Tab. 5) confirm the reliability of the fitting model. The ratios among visible bands can be used to predict SD very well, as already shown in previous studies ([31], [33], [13], [30], [21]): Among the single bands the TM1 showed the best relationship with SD, as suggested by the correlation analysis. On the other hand, even if the TM1/TM3 ratio showed a good correlation, it has been excluded from the functional model, as it was not statistically significant in the regression analysis. This behaviour is due to the high collinearity with the other independent variables included in the model.
On the other hand, the ratio TM3/TM2 was very significant accounting for 66% of SD variation; adding further terms to the functional model improves the prediction significantly reducing RMSE. The model, however, tends to slightly overestimate SD when values are low. The residuals values, the difference between predicted and observed values, show higher variability when the limnological values are low, e.g., in Lago Grande (Fig. 2), supporting the argument that it is difficult to derive low limnological parameter values from radiometric ones ([20]).
Fig. 2 - Observed and predicted Secchi Disk values with the 95% confidence and prediction limits (dashed and dash dotted lines, respectively) of the regression model.
The variability of chlorophyll a concentration was investigated using the same procedure, incorporating visible bands and their ratios as independent variables in regression analysis. From the stepwise variable selection procedure the following functional model was selected (eqn. 7):
where Chl-a is the chlorophyll a concentration in mg m-3, TMi are the radiometric and atmospheric corrected Landsat bands in W m-2 sr-1 μm-1. Regression analysis (Tab. 5) shows that the ratios among visibile bands of Landsat are very accurate predistors of chlorophyll a concentration: the ratio TM3/TM2 accounts for 50% of the variation of the studied parameter, the TM2/TM1 ratio and the TM2 band have significant contributions as expected.
The determination coefficient R2 has quite a high value, in line with similar studies ([20], [5], [21]). Moreover the IA and NSE indices, 0.925 and 0.754 respectively (Tab. 5, Fig. 3), confirm the reliability of model estimates.
Fig. 3 - Observed and predicted values of chlorophyll a concentration with the 95% confidence and prediction limits (dashed and dash dotted lines, respectively) of the regression model.
Having identified the regression functions for transparency and chlorophyll a concentration the two limnological parameters were thus mapped for the whole lake surface of the two basins (Fig. 4). Both transparency and chlorophyll a concentration show very different conditions in the two water bodies: transparency values are considerably higher for Lago Piccolo than for Lago Grande (which rarely exceeds 1 m depth). This is mostly due to the different chlorophyll a concentrations in the two lakes: in Lago Piccolo the chlorophyll values are 2.5 mg m-3 on average, with values up to a maximum of 4.5 mg m-3, while in Lago Grande the mean value is 6.8 mg m-3, with values up to a maximum of 10 mg m-3.
The correlation between transparency and chlorophyll a concentration ([29]) was also determined by correlating the two limnological parameters for all the pixels of the lake surface (Fig. 5). The linear relation between the two parameters (chl-a = 8.864 - 2.112·SD) is strong and significant with R2 = 0.93 and SEE (Standard Error of Estimate) = 0.6.
Finally, the maps created of chlorophyll a concentration and transparency were reclassified taking into account the ranges considered by OECD in order to define the trophic conditions of the two water bodies (Tab. 6).
Tab. 6 - The area based chlorophyll concentration and transparency according to the OECD classification ranges.
Range OECD |
Transparency (SD) m | Range OECD |
Chlorophyll a mg m-3 | ||||||
---|---|---|---|---|---|---|---|---|---|
Lago Grande | Lago Piccolo | Lago Grande | Lago Piccolo | ||||||
Area (ha) |
% | Area (ha) |
% | Area (ha) |
% | Area (ha) |
% | ||
<1.5 | 39.7 | 96.2 | 0.25 | 1.8 | ≤ 1 | - | - | 0.67 | 4.8 |
1.5 - 3 | 1.6 | 3.8 | 4.91 | 35.3 | ≤ 2.5 | - | - | 7.66 | 55.1 |
3 - 6 | - | - | 8.74 | 62.9 | 2.5 - 8 | 34.6 | 83.2 | 5.57 | 40.1 |
6 - 12 | - | - | - | - | 8 - 25 | 6.9 | 16.8 | - | - |
>12 | - | - | - | - | > 25 | - | - | - | - |
Most of Lago Grande has transparency values lower than 1.5 m, which is typical of a hypertrophic lake and 83.2% of the chlorophyll concentration values have characteristics of mesotrophic lakes; only 17% (mainly concentrated in lake western part), has a concentration typical of eutrophic lakes.
Most of Lago Piccolo has transparency values exceeding 3 m, which is typical of mesotrophic lakes, although an important part (around 35%) has eutrophic lake characteristics. Chlorophyll a concentration values are such that they characterize the lake as meso-oligotrophic: 95% of the values range from 2.5 to 8 mg m-3.
From the results, it can be stated that satellite remotely sensed data, especially data from Landsat, can be used effectively to assess the limnological parameters of lake waters. Radiometric data from Landsat can be used to map the areal distribution of some water biophysical parameters even for small basins such as the Monticchio lakes.
Data from the new sensors, such as Modis, can have a high temporal frequency ([36], [35]) and facilitate atmospheric correction ([29]), whilst being able to provide direct information on absorption and atmospheric scattering, unfortunately, they have the disadvantage of low spatial resolution which precludes their use on small water basins. On the other hand, data from high definition sensors, such as Ikonos, although used in different ways to study limnological parameters of inland waters, have the disadvantage of high costs making water resource management of large areas prohibitively expensive. Landsat data, on the other hand, has the advantages of reasonable spatial resolution, so that it can be used even for very small lake surfaces, and cover wide areas, allowing the management of inland waters at the regional level ([3], [29]). Finally, the fact that historical archives of Landsat data stretch back to the seventies allows the evolution of the biophysical characteristics of lake water to be studies.
Conclusions
A strong, significant, relationship was found between the spectral data in the TM bands with both chlorophyll concentration (R2 = 0.72) and with transparency (R2 = 0.82) measured as Secchi Disk depth. Multiple regression analysis on remote sensing data allowed to efficiently define the models and identify statistically significant variables. The ratios among visible bands and, most significantly, between TM3 and TM2 were good predictors both of transparency and chlorophyll concentration. The TM3 and TM2 bands have opposing trends in reflectance as a function of chlorophyll concentration: an increase of chlorophyll a concentration markedly increases reflectance in band TM2 whereas it results in a stronger absorbance in band TM3.
The two parameters can be mapped for the whole lake surface by identifying the best set of band combinations to describe the relationship linking spectral response to limnological data. The same method can be applied to the trophic conditions. In our case the synoptic view showed a different states of two small lakes providing a basis for a targeted intervention strategy.
Acknowledgments
This work was supported by EC - Interreg III B WETMUST project (code A.1.042).
References
Gscholar
Gscholar
Gscholar
Gscholar
Gscholar
Gscholar
Gscholar
Gscholar
Gscholar
Gscholar
Online | Gscholar
Gscholar
Gscholar
Gscholar
Gscholar
Gscholar
Gscholar
Gscholar
Gscholar
Gscholar
Authors’ Info
Authors’ Affiliation
International PhD in “Crop system, Forest and Environmental Sciences”, University of Basilicata, Potenza (Italy)
Corresponding author
Paper Info
Citation
Mancino G, Nolè A, Urbano V, Amato M, Ferrara A (2009). Assessing water quality by remote sensing in small lakes: the case study of Monticchio lakes in southern Italy. iForest 2: 154-161. - doi: 10.3832/ifor0507-002
Academic Editor
Marco Borghetti
Paper history
Received: Apr 29, 2009
Accepted: May 23, 2009
First online: Jul 30, 2009
Publication Date: Jul 30, 2009
Publication Time: 2.27 months
Copyright Information
© SISEF - The Italian Society of Silviculture and Forest Ecology 2009
Open Access
This article is distributed under the terms of the Creative Commons Attribution-Non Commercial 4.0 International (https://creativecommons.org/licenses/by-nc/4.0/), which permits unrestricted use, distribution, and reproduction in any medium, provided you give appropriate credit to the original author(s) and the source, provide a link to the Creative Commons license, and indicate if changes were made.
Web Metrics
Breakdown by View Type
Article Usage
Total Article Views: 53248
(from publication date up to now)
Breakdown by View Type
HTML Page Views: 43849
Abstract Page Views: 3247
PDF Downloads: 5141
Citation/Reference Downloads: 81
XML Downloads: 930
Web Metrics
Days since publication: 5476
Overall contacts: 53248
Avg. contacts per week: 68.07
Article Citations
Article citations are based on data periodically collected from the Clarivate Web of Science web site
(last update: Feb 2023)
Total number of cites (since 2009): 34
Average cites per year: 2.27
Publication Metrics
by Dimensions ©
Articles citing this article
List of the papers citing this article based on CrossRef Cited-by.
Related Contents
iForest Similar Articles
Review Papers
Remote sensing-supported vegetation parameters for regional climate models: a brief review
vol. 3, pp. 98-101 (online: 15 July 2010)
Review Papers
Accuracy of determining specific parameters of the urban forest using remote sensing
vol. 12, pp. 498-510 (online: 02 December 2019)
Technical Reports
Detecting tree water deficit by very low altitude remote sensing
vol. 10, pp. 215-219 (online: 11 February 2017)
Review Papers
Remote sensing of selective logging in tropical forests: current state and future directions
vol. 13, pp. 286-300 (online: 10 July 2020)
Research Articles
Afforestation monitoring through automatic analysis of 36-years Landsat Best Available Composites
vol. 15, pp. 220-228 (online: 12 July 2022)
Technical Reports
Remote sensing of american maple in alluvial forests: a case study in an island complex of the Loire valley (France)
vol. 13, pp. 409-416 (online: 16 September 2020)
Review Papers
Remote sensing support for post fire forest management
vol. 1, pp. 6-12 (online: 28 February 2008)
Research Articles
Modelling dasometric attributes of mixed and uneven-aged forests using Landsat-8 OLI spectral data in the Sierra Madre Occidental, Mexico
vol. 10, pp. 288-295 (online: 11 February 2017)
Research Articles
Estimation of aboveground forest biomass in Galicia (NW Spain) by the combined use of LiDAR, LANDSAT ETM+ and National Forest Inventory data
vol. 10, pp. 590-596 (online: 15 May 2017)
Research Articles
Evaluation of hydrological and erosive effects at the basin scale in relation to the severity of forest fires
vol. 12, pp. 427-434 (online: 01 September 2019)
iForest Database Search
Search By Author
Search By Keyword
Google Scholar Search
Citing Articles
Search By Author
Search By Keywords
PubMed Search
Search By Author
Search By Keyword