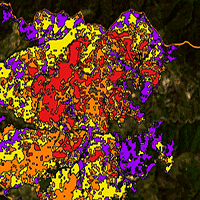
Evaluation of hydrological and erosive effects at the basin scale in relation to the severity of forest fires
iForest - Biogeosciences and Forestry, Volume 12, Issue 5, Pages 427-434 (2019)
doi: https://doi.org/10.3832/ifor2878-012
Published: Sep 01, 2019 - Copyright © 2019 SISEF
Research Articles
Abstract
This study aimed to assess the hydrological and erosive effects of different levels of the fire severity in the drainage basin of the Trionto River (Calabria, southern Italy), which was partially burned by intense fires during the summer 2017. The analysis focused on the identification of wildfire areas using a supervised classification of remote sensing images with the minimum distance algorithm. The level of severity of each fire was then discriminated based on a procedure proposed by the U.S. Department of Agriculture and adapted to the study area. To evaluate how wildfire occurrence affects the hydrological behaviour at the basin scale, the SCS-Curve Number model was used to document pre- and post-fire conditions in relation with the level of fire severity. Finally, the influence on erosion was analysed for analogous conditions at the basin scale using the RUSLE equation. The effects on hydrological balance and soil loss were evaluated by comparing the pre-fire value with three different post-fire scenarios: (a) different levels of severity on the surface covered by the fire (real case); (b) maximum level of severity on the surface covered by the fire; (c) total loss of the canopy and formation of a hydrophobic layer on the surface soil. The results confirmed that the level of severity of the forest fires, combined with climatic factors, morphological conditions, and the pedological characteristics of the basin, significantly influence changes to the hydrology and rates of erosion. Moreover, these impacts proved to be mainly dependent on the consequent, often notable, heterogeneity in the spatial distribution of burned areas with different severity.
Keywords
Forest Fire Severity, Hydrological Impacts, Soil Loss Estimation, Remote Sensing
Introduction
Wildfires are a growing threat to forests worldwide. In the Mediterranean region, the incidence and the extent of surfaces burned by fires constitute a large, complex problem with environmental and socio-economic consequences. The European Union (EU) countries most affected by this phenomenon are France, Greece, Italy, Portugal, and Spain, which altogether represent 46% of the whole European forest cover. In these countries, over 16 million hectares were burned by about 1.8 million wildfires between 1980 and 2016, with an average of 48,000 wildfires per year. Out of these five countries, Portugal has the greatest problem. Data for the period 1980-2016 ([46]) show that, on average, about 450,000 hectares of land were subjected to wildfires each year, of which 61% were concentrated between Portugal and Spain, where 68% of all fires have been recorded. Out of the remaining 39%, over half of the burnt area was in Italy (105,000 ha).
In the summer of 2017, according to provisional data, the European Commission estimated that the surface covered by fires before August was 72,039 ha in Italy, 115,323 ha in Portugal, 19,666 ha in Spain, and 9,585 ha in France ([37]). High temperatures and droughts had a considerable influence in creating conditions favourable for the development and propagation of forest fires, especially during the summer season and in certain areas. In recent years, the increase in extreme weather events has led to a greater concentration of large fires in the European Union compared to the past, increasing the areas at risk of fires northwards into Northern Europe. In the forthcoming decades, however, emergencies related to forest fires in the Mediterranean area are expected to increase, due to the likely increase in the intensity and frequency of drought and extreme temperatures, with current prevention strategies not being effective enough ([53]).
Fires have direct and indirect effects on the environment over the short or long term. In particular, the effects of fire are highly variable due to differences in the intensity and frequency of events, mixture of composition and structure of forest populations, and soil characteristics. For many wildfires documented in Europe, there has been a marked change in the hydrological response to precipitation, with surface runoff increasing, particularly with respect to peak flow rates rather than water volumes ([18], [29], [31], [3]). Moreover, soil resistance to erosion also declines ([9], [21], [45], [49]), with the rate of erosion reaching several orders of magnitude due to certain site-specific factors ([36]). From a physical perspective, the effects of forest fires on the hydrological balance are attributed to changes in forest cover and soil properties. In particular, variations in the hydrological behaviour of the basin are associated to changes in transpiration processes, interception capacity, the onset of repellency phenomena and changes to the infiltration capacity. These phenomena are particularly noticeable in areas where high fire severity causes the volatilisation of certain organic components of the surface soil layers ([10], [21], [11], [42], [47], [38], [28], [14], [51], [32]).
The hydrological balance and extent of soil erosion are influenced by the behaviour and severity of the fire, type of soil, morphology, and intensity of rainfall. The behaviour of fires is analysed using different features, such as variation in the linear intensity of the flame front and residence time. The severity of fires is also measured based on the degree of ecological change resulting from their actions on the ecosystem ([35], [25], [20]). Burn severity reflects how fire affects vegetation and soil characteristics, which subsequently determine runoff responses. Usually, burn severity is classified into discrete descriptive classes (such as high, moderate, and low), which reflect the degree of removal of both the canopy layer and the ground cover ([22]). At small scales (up to 100 m2), burn severity is assessed using physical tests, such as soil measurements of water infiltration ([43]) and the water drop penetration time test ([9]). At larger scales (hillslope to basin scales), a reliable metric of soil burn severity is derived from Landsat imagery, as proposed by the U.S. Department of Agriculture (USDA) Forest Service ([41]). Specifically, soil burn severity is assessed based on changes to land surface reflectance between two Landsat satellite images for near and short-wave infrared bands (difference between post- and pre-fire Normalized Burn Ratio, ΔNBR). From a practical perspective, ΔNBR values may be clustered into qualitative values of high, moderate, and low soil burn severity classes; however, different number of classes and thresholds have been used across studies ([7], [5]). Furthermore, some studies using remote sensing have had difficulty in discriminating fire severity classes for certain vegetation communities ([44]).
This study investigates how wildfires influenced a drainage basin located in Calabria (southern Italy) during July-August 2017. Specifically, the study examined major fire events lasting several days over a large portion of wooded territory on the Sila Plateau. The aim is to explore the potential of remote sensing via satellites to evaluate how the hydrology and erosion rates of the basin were impacted by different levels of fire severity.
Materials and methods
Study area
The drainage basin of the Trionto River is part of the Sila Greca (Calabria, Italy), which is located in the northeastern sector of the Sila Plateau. The river flows into the Ionian Sea, and the total area of the basin is 288 km2. From a geomorphological perspective, the basin is subdivided into two parts with distinct characteristics. Higher elevations and steep slopes characterise the mountainous part, while the valley part has a gentler morphology, with a riverbed in the terminal section containing large quantities of debris, that configure the typical braided course of the Calabrian rivers, called “fiumare”.
This study focused on a sub-basin of the Trionto River that was strongly impacted by wildfires from 15 July to 31 August, 2017. The drainage area of the sub-basin is 8497 ha, of which 0.3% are urbanised. The maximum and mean elevations of the sub-basin are 1644 and 1089 m a.s.l., respectively, with mean slope of 40%. The study site is located in the southwestern, mountainous part of the Trionto drainage basin (Fig. 1). This location is very difficult to access, due to its morphological complexity, resulting in it being characterised by high density of forest populations. The fire also made access to the site difficult, preventing on-site ground surveys for post-fire condition inspections; thus, it was only possible to perform photographic examination of the soils.
During the mid-1950s, large areas of this basin were reforested to address the geological instability of the region. The main tree species used was Calabrian pine (Pinus nigra J.F. Arn. ssp. laricio [Poiret] Maire), which is a hardy endemic species of the region. Because the area is composed of heavily eroded granites and shales soils characterised by steep slopes, land uses, other than forests, were not possible. Specifically, the sub-basin is composed of soils belonging to the V and the VI classes of the soil capability, according to the Land Capability Classification ([13]).
The different land use classes of the sub-basin were delineated from IV level of the Corine Land Cover (see Fig. S1 in Supplementary Material for the original data used in this analysis). The main land use of the sub-basin is forests, which cover 48.8% of the whole area (Tab. 1).
Tab. 1 - Percentage of land use in the sub-basin.
Land use | Area (%) |
---|---|
1 - Discontinuous urban territory | 0.3 |
2 - Intensive crops | 20.2 |
3 - Crop system and complex particles | 0.1 |
4 - Crops with presence of important natural areas | 2.7 |
5 - Woods with prevalence of holm oaks | 2.5 |
6 - Mixed and pure woods with prevalence of deciduous oak | 3.5 |
7 - Woods with prevalence of chestnut | 3.4 |
8 - Pine plantation and natural pine forest | 25.2 |
9 - Beech forest with sparse Calabrian pine area spatiality | 11.4 |
10 - Mixed Calabrian pine - beech forest | 2.8 |
11 - Grassland and grazing | 5.1 |
12 - High Mediterranean scrub | 4.2 |
13 - Low Mediterranean scrub and garrigue | 3.0 |
14 - Sparse and evolving areas | 14.8 |
15 - Alluvial areas | 0.8 |
The soils exhibit the specific associations listed in Tab. 2, which are linked to the corresponding drainage classes ([13]).
Tab. 2 - Classes of soil drainage.
Associations of soils | Drainage class |
---|---|
Typic Xerofluvents; Fluventic and Dystric Fluventic Xerochrepts | Excessively drained |
Lithic and Dystric Xerorthens, Dystric Xerochrepts, Lithic Xerumbrepts | Excessively drained |
Dystric Fluventic Xerochrepts, Dystric Xerochrepts, Typic Xerumbrepts; Lithic Xerorthens | Excessively drained |
Typic Xerumbrepts; Ultic Haploxeralfs, Entic and Lithic Xerumbrepts, Dystric and Dystric Lithic Xerumbrepts | Somewhat excessively drained |
Typic Haplumbrepts; Lithic Haplumbrepts and Ultic Hapludales | Somewhat excessively drained |
Lithic Ruptic-Xerorthentic Xerochrepts, Typic and Dystric Xerochrepts | Well drainage |
Lithic Xerorthens And Lithic Ruptic-Xerorthentic Xerochrepts; Typic and Ruptic-Lithic Xerochrepts | Moderately well drained |
Ultic Haploxeralf; Dystric Xerochrepts and Dystric Xerorthens | Moderately well drained |
Dystric, Aquic Distric and Typic Xerochrepts; Lithic Xerorthens, Ultic and Aquultic Haploxeralfs | Somewhat poorly drained |
Classification of burned areas related to different degrees of severity
During the summer of 2017 (July-August), a series of forest fires affected a large percentage of the study area. The most relevant events lasted for four consecutive weeks (from July 31 to August 26), and impacted a large, partially forested, area between Acri and Longobucco, which are two large municipalities of the Presila and Sila Greca in southern Italy.
All fire events that occurred in the study area during the observed period were detected using a Sentinel-2, Level 1-C image. An image acquired on September 2, 2017 with a 10 m spatial resolution was used. The potential of the Near Infra-Red was exploited, which shows strong absorption for the burnt vegetated areas. Thus, the false colour combination in the 8-4-3 bands depicted burned areas as dark grey and living vegetation as red (see Fig. S2 in Supplementary Material for the original data used in this analysis).
The area exposed to the fires was bounded through the supervised classification procedure of the satellite image using the Minimum Distance algorithm, trained by means of the ROI (Region Of Interest) approach. This algorithm classifies each pixel in an image by calculating the Euclidean distance d(x,y) between the spectral signature of the pixel and a training spectral signature that is defined for each specific macro-class as follows (eqn. 1):
where xi is the vector of the pixel’s spectral signature, yi is the vector of the training spectral signature, and n is the number of spectral bands.
The accuracy of classifying unburned vs. burned areas was assessed using test sites for which satellite images were subject to photo interpretation. Specifically, a confusion matrix was created, which obtained an overall accuracy of almost 90%. The kappa index of agreement was about 0.8, showing acceptable accordance between the classification map and reference data ([8]).
To determine the level of fire severity, the FireMon procedure ([27]) was used. This procedure uses the NBR (Normalized Burn Ratio) index, which is equivalent to the normalised difference between near infrared and short-wave infrared (eqn. 2):
This index quantifies the difference between two optical-satellite acquisitions that are spatially homogeneous but have a temporal shift (pre-fire and post-fire conditions), in which (eqn. 3):
For this purpose, two Landsat-8 images from May 14, 2017 and September 19, 2017 with a 30 m spatial resolution were used.
The ΔNBR index is based on satellite reflectance, which is associated with water content. Fig. 2 shows the deviation between normally distributed cumulative probability functions (CDFs) assumed by the ΔNBR index for data from two prevailing land use types in the burned area (forested areas and sparse vegetation).
Fig. 2 - Variation in the cumulative distribution functions (CDF) of the ΔNBR for two prevailing land uses in the basin: (a) forested areas; (b) sparse vegetation.
This high deviation led to the different land uses being considered separately when classifying the severity of wildfires to avoid the impacts being underestimated. Thus, according to the difference between the average values assumed by the ΔNBR index for the two land uses (delta), the severity rating was adapted to the forested area by using intervals suggested by FireMon. The extreme values of the intervals were reduced by a fraction of the delta using a trial and error approach, with the aim to achieve a good match between the resulting severity areas of FireMon and the accurate burned areas of Sentinel-2. It was not possible to ground-truth the results because of the difficulty in accessing the study site. Some consequences were observed only at limited sites that were accessible, and which were surveyed using pictures (Fig. 3). Thus, the choice of comparing results from different products and techniques appears cautionary. Tab. 3 reports the severity levels that were identified and the corresponding intervals of the ΔNBR index.
Fig. 3 - Effect of forest fires on the Trionto basin. (a) Heterogeneity of fire severity in a burned area; (b) reforestation of Calabrian pine (Pinus nigra) affected by low fire severity; (c) reforestation of Calabrian pine affected by mean fire severity; (d) reforestation of Calabrian pine affected by high fire severity.
Tab. 3 - Level of severity of forest fires.
Level of severity | ΔNBR interval |
---|---|
Very low | -100 → 98 |
Low | 99 → 268 |
Moderate | 269 → 438 |
High | 439 → 659 |
Very high | 660 → 1188 |
Application of the SCS-CN model to estimate hydrological effects
How forest fires impacted the hydrology at the basin scale was estimated using the SCS-CN model ([54]). This model is frequently used to evaluate surface runoff at the river basin scale. The model takes pre-fire and post-fire conditions into account, and discriminates the different levels of severity based on the procedure reported in the BAER Hydrology Special Report ([19]). In the SCS-CN model, infiltration losses are combined with surface accumulation through eqn. 4:
where Q (mm) is the excess rain, P (mm) is the height of rainfall and Ia (mm) is the initial abstraction, commonly assumed as (eqn. 5):
Parameter S (sorptivity) is the maximum retention potential of the soil, given by (eqn. 6):
where the scale factor S0 is equal to 254 mm (10 inches), while the parameter CN (dimensionless, ranging from 0 to 100) depends on land use, hydrological soil type and antecedent soil moisture (AMC, index of soil wetness - [54]). CN under pre-fire conditions (CNpre) is evaluated as the weighted average of the catchment area. It is calculated by combining information provided by land use and hydrological soil type (A, B, C or D), which is selected based on the runoff potential of the corresponding soil unit. The same parameter was obtained under post-fire conditions (CNpost) through procedures documented in the BAER Report, which considers a constant numerical increase of CNpost in relation to the severity level of the fire (Tab. 4).
Tab. 4 - Variation in the parameters CN, C and K corresponding to the different levels of severity of forest fires.
Level of severity | Variation from pre-fire condition |
||
---|---|---|---|
CN | C (%) | K(%) | |
Low | +5 | + 40 | + 40 |
Moderate | +10 | + 60 | + 60 |
High | +15 | + 80 | + 80 |
Very high | +20 | + 100 | + 100 |
Differences in sorptivity were calculated as a function of CN under pre-fire and post-fire conditions, by quantifying hydrological effects due to fires at the basin scale. Specifically, the effects were evaluated by comparing pre-fire conditions with three different post-fire scenarios: (a) different levels of severity to the burned area (realistic scenario); (b) maximum level of severity to the burned area; (c) complete loss of the canopy and waterproofing of the burned area (worst case scenario). These three scenarios were used to establish a reasonable impact framework where uncertainty prevents precise estimates.
Application of the RUSLE model to estimate soil loss
The erosive effects of forest fires on the basin were assessed by applying at the basin scale the equation which has been adopted and revised by the U.S. Department of Agriculture as RUSLE (Revised Universal Soil Loss Equation - [55]). The RUSLE equation introduces new parameters allowing the model to be better described, even on morphologically complex terrains. This equation is based on an empirical parametric model that estimates average annual soil loss rates caused by surface water erosion. The USLE-RUSLE model is based on the following relationship (eqn. 7):
where A is the mean annual value of soil loss (Mg ha-1 y-1), R is the rainfall erosivity factor (MJ mm h-1 ha-1 y-1), K is the soil erodibility factor (Mg ha-1 MJ-1 mm-1 ha h), LS is the topographic factor or slope length factor (dimensionless), C is the soil coverage (dimensionless), and P is a factor that depends on erosion control practices (dimensionless). The product among the factors, except for rainfall erosivity, provides a measure of resistance of the environment to erosive phenomena. The R factor is influenced by the intensity and the duration of precipitation, namely, the kinetic energy of the rain is transformed to mechanical energy on impact with the surface. Therefore, this phenomenon depends on the geographic-climatic location of the different parts of the basin. The R factor for the study area was obtained from a raster map constructed for a previous application of RUSLE in Calabria at regional scale ([52]).
The soil-erodibility K factor is a measure of soil susceptibility to erosion. This parameter depends on certain physical properties of the soil that influence the infiltration capacity and the movement of water within the soil. It also depends on properties that affect the dispersion, abrasion and mobility of soil constituent particles. The K factor was assigned based on values from the published literature, with the zoning of the pedological units of the area being taken into account ([13], [52]).
The topographic factor LS takes into account the length (L) and the slope (S) of hills, because the extent of erosion processes is influenced by the association of these two factors. Several methods have been proposed to improve the calculation of LS; however, sufficient accuracy has only recently been achieved due to advances in GIS and digital elevation models (DEM) for the RUSLE calculation. To quantify the convergence or divergence of superficial runoff, the L factor was replaced by the concept of “unit contributing area” ([33], [30], [12]). Thus, it is possible quantify the areas contributing to runoff and the direction of surface runoff on the raster cell grid (flow accumulation grid), which is necessary to apply the finite difference equations to calculate LS in a two-dimensional raster environment. A simple form of the equation used to compute LS is ([17], [34], [30] - eqn. 8):
where AS (m) is the upslope contributing area per unit contour width (for this study, it was assessed using ArcGIS® functions “flowacc” and “resolution”); b is the slope, m = 0.4 (value range: 0.2-0.6) and n = 1.3 (value range: 1.0-1.3 - [30]). The results were in good accordance with previous researches in the study area ([1], [39]).
The cover-management C factor quantifies the effect of land cover, and is influenced by both land use and management. Low C values (equal or close to 0) are attributed to areas with dense tree vegetation that limit the potential rate of soil erosion. In comparison, values close to 1 are assigned to poorly protective crops, such as grassland, grazing and open vegetation, or to soil subjected to mechanical agricultural processes that favour erosion. The support-practise P factor ranges from 0 to 1, and represents possible practices for erosion control, aimed essentially at reducing surface runoff (such as terracing and intercropping).
The USLE-RUSLE model was applied by creating raster layers (40 m spatial resolution) related to the study area for each of the considered factors. The erodibility rate under pre-fire conditions was obtained as a mean value at the basin scale. This procedure was replicated to estimate variation in erodibility rates under post-fire conditions. Thus, the consequent effects were considered, especially changes to forest cover, the consumption of organic substances in the soil and the reduction of surface roughness, which induce changes to C and K. It is assumed that the variation in the original values of these factors progressively increases with the level of severity (Tab. 4) ([52]). The three aforementioned scenarios were used to evaluate how pre-fire and post-fire conditions affect soil loss.
Results
The section of the Trionto sub-basin analysed in this study is mainly characterised by soils with good hydrological efficiency, based on their high retention capacity, limiting detrimental effects caused by excess surface runoff. The varying magnitude of the wildfire events of the summer of 2017 caused spatial variation in forest cover. The areas covered by fires were grouped according to levels of severity (Fig. 4a). For each level, the percentages of non-forest and forested areas were evaluated (Fig. 4b).
Fig. 4 - Percentages of: (a) levels of severity of burned areas; (b) non-forest and forested areas burned by fires.
The sub-basin was primarily covered by two types of land use: forested areas (about 66%) and sparse and evolving areas (about 27% - see Fig. S3a in Supplementary Material for the original data used in this analysis). The sparse and evolving areas were mainly re-afforestation areas, and were the same zones in which repeated fires in previous years destroyed the forest cover. The forested areas exposed to fires were almost completely (90%) pine plantations and natural pine forests (see Fig. S3b in Supplementary Material for the original data used in this analysis).
Fig. 5 shows the zoning of the burned area for the different levels of severity. The fire covered a total area of 2357 ha, which was equal to 28% of the whole sub-basin area. About 55% of the burned area was exposed to fires of moderate and low severity (Fig. 4a).
Fig. 5 - (a) Subdivision of the burned areas according to different levels of severity; (b) burned areas.
Tab. 5 shows the values of hydrological balance and soil erosion of the study area obtained using the CN method (parameters CN and S) and RUSLE method (mean annual value of soil erosion) under pre-fire and post-fire conditions based on the three scenarios. The averaged CN estimates for the entire basin show that the impact increased with increasing severity of fires under the three scenarios. Anyway, the actual proportion of the high-severity burned areas in the basin is crucial for their quantification, as shown by the CN values, which increased from 5% to 9% between the actual status (scenario a) and the modelled maximum level of severity (scenario b). CN only reached 21% in the scenario where the canopy was completely lost and the burned area was waterproofed (scenario c); however, this situation is rare. A corresponding decrease in sorptivity was detected (evaluated as the change to maximum retention potential ΔS, in comparison with the pre-fire condition), demonstrating that the basin was subject to higher runoff after wildfires. The loss of hydrological efficiency between pre-fire and post-fire conditions was quantified using the S parameter. S declined by 13% for scenario a and 21% for scenario b. Under scenario c, ΔS clearly declined (47%).
Tab. 5 - Estimates of curve number (CN) and sorptivity (S), and variation in S (ΔS) between pre-fire and post-fire conditions. Mean annual value of soil erosion (A) and its variation from the reference value (ΔA).
Scenario | CN | S (mm) |
ΔS (%) |
A (Mg ha-1 y-1) |
ΔA (%) |
---|---|---|---|---|---|
Pre-fire | 63 | 149 | - | 25 | - |
Post-fire - scenario a | 66.2 | 130 | -13 | 31 | +24 |
Post-fire - scenario b | 68.4 | 117 | -21 | 36 | +44 |
Post-fire - scenario c | 76.1 | 80 | -47 | >100 | >100 |
Based on RUSLE, variation in C and K under the post-fire conditions was attributed to canopy loss due to the wildfires; consequently, rainfall impacted the soil with greater energy. As the ability of the soil to absorb water declined, greater runoff occurred, particularly on very steep slopes characterised by burnt areas. This phenomenon contributed towards increasing the amount of sediment transport. The pre-fire value for soil erosion was comparable to the tolerable value for maximum annual soil loss in highly erodible areas (up to 25 Mg ha-1 y-1) delineated by Kirkby & Morgan ([23]), because the Trionto basin is characterised by highly eroded soils and large precipitation. Under scenario a, the mean annual value of soil erosion increased by 24%, and rose to 44% under scenario b (maximum level of severity), as shown in Tab. 5. Under scenario c, the mean annual value of soil erosion exceeded 100% that of the pre-fire value.
Discussion
Damaging wildfires impacted a large area of forested land on the Sila Plateau in southern Italy over several days in 2017. However, accessing these remote areas was difficult to establish appropriate on-site ground surveys of post-fire conditions, requiring the adoption of alternative approaches. Thus, this study compared the effectiveness of different satellite products and different scenarios to quantify how wildfires impacted the hydrology and erosion rates of the study area. The supervised classification of the burned areas was performed using a Sentinel-2 image, with the kappa index being used to establish accuracy. The results of this approach showed good agreement with ground truthing ([24]). The overall accuracy was similar to that reported by Chirici & Corona ([6]) for a forest fire in central Italy. Landsat images were used to assess the level of severity of fires based on previous studies, in which NBR represented the most optimal index derived from Landsat TM and ETM+ sensors when mapping burn severity ([15], [4], [16]). This approach allowed ΔNBR to be evaluated effectively by exploiting improved signal-to-noise ratios and the enhanced radiometric resolution of Landsat-8 under highly heterogeneous scenarios. This procedure involved the comparison of burned areas using two different satellite products, which helped to avoid errors in zoning the level of fire severity.
The hydrological status of the sub-basin before and after the fires was assessed using CN, with pre-fire values being closely related to the good condition of forest coverage in the area. In comparison, post-fire CN indicated the partial loss of hydrological efficiency. This phenomenon was also highlighted by changes to sorptivity (ΔS), which became less significant over time ([40], [50]). In particular, the comparison between the three post-fire scenarios showed how the increase in CN values and the loss in sorptivity (ΔS) assumes relevant values only with the complete destruction of the canopy and the waterproofing of the land (ΔS decreased to about half that of the initial average retention capacity). Under scenario a (the real situation, with different levels of severity), there was a moderate loss of hydrological efficiency, which increased when the whole basin was affected by the maximum level of fire severity (scenario b). The CN under the three scenarios were very similar to that obtained by Leopardi & Scorzini ([26]) for the San Giuliano basin in Abruzzo (Central Italy). Differences between pre-fire and post-fire values of CN detected in our study were comparable (percentage-wise) to those obtained by Candela et al. ([2]) in a Sicilian basin (southern Italy), based on eqn. 6 for sorptivity S and using data for two rainfall-runoff events post-fire. At the basin scale, the average retention potential was closely related to the increase in surface runoff, and strongly impacted hydraulic efficiency in the valley part of the Trionto basin, which is historically vulnerable to flooding.
Under the scenario with no discrimination of fire severity, there was a noticeable increase in soil loss (erosion), particularly when compared to scenario a (actual situation). The mean rate of soil loss estimated by RUSLE is generally higher than that of point measured values in Mediterranean fire-affected areas ([48]). However, the three scenarios explored in this study provided results that were in good agreement with the soil erosion risk scenarios evaluated by Terranova et al. ([52]) for Calabria at a regional scale. These results show that hydrological and erosive effects are progressively significant if areas affected by high fire severity are spatially large within the drainage basin. Thus, a proper evaluation of the effects at the basin scale is strictly related not only to the correct identification of the entire burned areas but also to the accurate zoning of severity levels. If neglected, this would lead to a heavy biased estimation of the fire impacts, which makes hard to prioritise recovery interventions at a large scale.
Conclusions
This study quantified how wildfires impacted a sub-basin of the Trionto River (southern Italy) under three different post-fire scenarios, by evaluating changes to the hydrology (water balance) and soil erosion rates in burned areas subjected to different levels of fire severity. The hydrological (SCS-CN) and the erosive (RUSLE) models allowed some of the main impacts of forest fires on the sub-basin to be characterised. Consequently, the basin proved to be strongly influenced by variation in fire severity, due to the importance of heterogeneity in the spatial distribution of burned areas. The level of severity of burned areas to the total surface area represents a fundamental element for the correct evaluation of hydrological and erosive parameters of the basin after wildfires. By overlooking these parameters, the effects might be overestimated, hindering the accurate interpretation of the impact of fires. This phenomenon was demonstrated by the substantial differences recorded between realistic and worst case scenarios. The methodology developed here provided a reasonable framework for establishing the priorities for post-fire recovery actions. Specifically, this study allowed the hydrological responses of the basin to be quantified, which could be used in flood risk assessments.
Acknowledgements
GC and FC carried out the GIS elaborations; AN performed the statistical analysis; FI and GC conceived the study; FI, EF and GC wrote the paper. All authors contributed to the discussion.
References
Gscholar
Gscholar
Gscholar
Gscholar
Gscholar
Gscholar
Gscholar
Gscholar
Gscholar
Online | Gscholar
Gscholar
Gscholar
Gscholar
Authors’ Info
Authors’ Affiliation
Antonino Nicolaci
Ennio Ferrari 0000-0002-4035-073X
Francesco Cruscomagno
Francesco Iovino 0000-0002-7000-255X
Department of Computer Engineering, Modeling, Electronics, and Systems Science - DIMES, University of Calabria, via P. Bucci 41C, I-87036 Rende, CS (Italy)
Corresponding author
Paper Info
Citation
Coschignano G, Nicolaci A, Ferrari E, Cruscomagno F, Iovino F (2019). Evaluation of hydrological and erosive effects at the basin scale in relation to the severity of forest fires. iForest 12: 427-434. - doi: 10.3832/ifor2878-012
Academic Editor
Angelo Nolè
Paper history
Received: Jun 02, 2018
Accepted: Jun 12, 2019
First online: Sep 01, 2019
Publication Date: Oct 31, 2019
Publication Time: 2.70 months
Copyright Information
© SISEF - The Italian Society of Silviculture and Forest Ecology 2019
Open Access
This article is distributed under the terms of the Creative Commons Attribution-Non Commercial 4.0 International (https://creativecommons.org/licenses/by-nc/4.0/), which permits unrestricted use, distribution, and reproduction in any medium, provided you give appropriate credit to the original author(s) and the source, provide a link to the Creative Commons license, and indicate if changes were made.
Web Metrics
Breakdown by View Type
Article Usage
Total Article Views: 42521
(from publication date up to now)
Breakdown by View Type
HTML Page Views: 36246
Abstract Page Views: 2936
PDF Downloads: 2618
Citation/Reference Downloads: 5
XML Downloads: 716
Web Metrics
Days since publication: 2138
Overall contacts: 42521
Avg. contacts per week: 139.22
Article Citations
Article citations are based on data periodically collected from the Clarivate Web of Science web site
(last update: Mar 2025)
Total number of cites (since 2019): 5
Average cites per year: 0.71
Publication Metrics
by Dimensions ©
Articles citing this article
List of the papers citing this article based on CrossRef Cited-by.
Related Contents
iForest Similar Articles
Review Papers
Remote sensing-supported vegetation parameters for regional climate models: a brief review
vol. 3, pp. 98-101 (online: 15 July 2010)
Review Papers
Accuracy of determining specific parameters of the urban forest using remote sensing
vol. 12, pp. 498-510 (online: 02 December 2019)
Review Papers
Remote sensing support for post fire forest management
vol. 1, pp. 6-12 (online: 28 February 2008)
Review Papers
Remote sensing of selective logging in tropical forests: current state and future directions
vol. 13, pp. 286-300 (online: 10 July 2020)
Technical Reports
Detecting tree water deficit by very low altitude remote sensing
vol. 10, pp. 215-219 (online: 11 February 2017)
Research Articles
Assessing water quality by remote sensing in small lakes: the case study of Monticchio lakes in southern Italy
vol. 2, pp. 154-161 (online: 30 July 2009)
Research Articles
Assessing the performance of MODIS and VIIRS active fire products in the monitoring of wildfires: a case study in Turkey
vol. 15, pp. 85-94 (online: 19 March 2022)
Research Articles
Afforestation monitoring through automatic analysis of 36-years Landsat Best Available Composites
vol. 15, pp. 220-228 (online: 12 July 2022)
Technical Reports
Remote sensing of american maple in alluvial forests: a case study in an island complex of the Loire valley (France)
vol. 13, pp. 409-416 (online: 16 September 2020)
Research Articles
Identification and characterization of gaps and roads in the Amazon rainforest with LiDAR data
vol. 17, pp. 229-235 (online: 03 August 2024)
iForest Database Search
Search By Author
Search By Keyword
Google Scholar Search
Citing Articles
Search By Author
Search By Keywords
PubMed Search
Search By Author
Search By Keyword