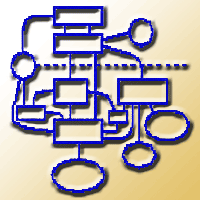
Modeling Italian forests: state of the art and future challenges
iForest - Biogeosciences and Forestry, Volume 5, Issue 3, Pages 113-120 (2012)
doi: https://doi.org/10.3832/ifor0614-005
Published: Jun 05, 2012 - Copyright © 2012 SISEF
Review Papers
Abstract
This review is a follow-up to the first meeting of the Forest modeling working group (FMWG) of the Italian Society of Silviculture and Forest Ecology (SISEF), held in December 2009. 18 talks were delivered to an audience of 40 researchers. We review the state of the art of forest ecosystem modeling in Italy, highlight findings from Italian research groups, and summarize relevant issues. Developing on the discussion session of the meeting, we indicate current research gaps and future challenges for modelers, forest ecologists and foresters alike, with a special emphasis on model validation, data availability, and communication between researchers and managers.
Keywords
Forest Models, Forest Inventory, SISEF, Ecological Modeling, Carbon Balance
Introduction
Simulation models of forest ecosystems answer two needs: clarifying the relationship between key ecosystem components, for a deeper understanding of their functioning ([63]), and predicting how the state variables of a dynamic system change due to processes in a forest stand or landscape ([18]). The comparison with desired targets will then result in improved ecosystem management. Modeling tools are increasingly used by both forest ecologists, who face the challenge of transferring knowledge to stakeholders and the general community, and managers, who benefit from the development of scenario-based supports for decision-making.
Several definitions of models exist. From a general point of view, modeling means trying to capture the essence of a system, deconstructing complex interactions between system components until only the most essential structures and processes remain ([54]). This refers to a descriptive level of ecological science. However, when management of natural resources is at stake, the value of ecological models lies in principle in their predictive power. Process-based and empirical models make it possible to predict the present value of a variable of interest (biomass, C sequestration, biodiversity, stem growth, etc.) from simultaneous values of other driving variables (climate, soil, stand density, etc.). By assuming that processes hold across time ([89]), ecologists use models developed and validated for current conditions to make predictions of future system trajectories. In this perspective, we define models as quantitative tools that predict the future probability distribution of an ecological variable, conditional upon initial conditions, parameter distributions, distributions of extrinsic drivers, and the choice of mathematical or statistical methods used to make the calculations ([26]). Simulators, on the other hand, refer to computer programs resulting from the conversion of such models into a piece of software for scenario calculation, and often visualization ([94]).
The state-of-the-art in forest ecosystem modeling has been presented in several conferences (e.g., [50], [45], [23], [40], [127], [3], [99], [68], [58]), and much of the accumulated knowledge is summarized by textbooks ([43], [40], [111], [118], [78], [124], [114], [2], [53], [58], [57], [94], [123], [93]). The diversity in ecosystem processes has resulted in the development of an extraordinary array of different models in forest ecology and management. Several and sometimes conflicting classification schemes have been proposed for models, based on their descriptive or explanatory purpose (represented by empirical and process models, respectively), ecosystem component addressed, spatial resolution and context, temporal extent, deterministic or stochastic nature ([81], [109], [15], [118], [92], [48], [88], [90], [79], [95], [113]). Pretzsch et al. ([95]) gave an overview of modeling approaches in Europe. The University of Kasselm, Germany maintains an internet-based Register of Ecological Models ([9] - ⇒ http://ecobas.org/www-server/index.html) with references to 681 models (as of March 2011); the Forest Model Archive is another such repository of forest models, maintained by the University of Greenwich, UK ([98]). Other databases include ForMIS, a database of European yield tables and empirical tree-scale models that includes model equation and parameters ([110]), and the more recent FORMODEL hosted by the Institute Européen de la Foret Cultivée ([85]). The latter is able to receive user input on newly developed models. The increasing interest in forest ecosystem modeling in Europe is reflected by the recent activation of two EU-COST[1] projects: FP0603 - “Forest models for research and decision support in sustainable forest management” (⇒ http://www.isa.utl.pt/def/fp0603forestmodels), aiming to enhance the quality and consistency of forest growth models to simulate the responses of forests to alternative management and climate scenarios ([22]); and FP0804 - “Forest Management Decision Support Systems (FORSYS)” (⇒ http://fp0804.emu.ee), that will define a European-wide framework and requirements for forest decision support systems (DSS) in a sustainable multifunctional forest management environment. FP0603 called for the identification and description of forest growth models available in Europe. Fifteen out of 23 nations have provided a country report ([87]), Italy not being among them.
The first meeting of the Working group for Forest ecosystem modeling of the Italian Society for Silviculture and Forest Ecology (SISEF), held in Bologna on 18th December, 2009, provided a comprehensive overview of the current efforts in simulating and forecasting forest ecosystem processes in Italy. Some 18 talks[2] were delivered to an audience of about 40 researchers. In this paper we review the state of the art of forest ecosystem modeling in Italy, highlighting findings from Italian research groups. Following up on the concluding remarks from the meeting, we summarize relevant issues, research gaps and future challenges, with an emphasis on data availability, calibration and validation methods, model choice and communication between researchers and managers.
Modeling forest ecosystems: country report
To set the scene, we find it useful to summarize key figures of Italian forests (Tab. 1), as assessed by the National Forest and Carbon Inventory ([60]) unless otherwise stated, in order to provide reference values for readers unfamiliar with the country and to harmonize this account to country reports produced by other members of COST FP0603.
Tab. 1 - Facts and figures of the Italian forest system.
Fact | Figure | Notes | |
---|---|---|---|
Forest cover (share of total landcover) |
8 759 200 ha (29.1%) | Canopy cover >10%, potential height > 5m. Includes plantations and temporarily unforested. | |
Growing stock and annual increment |
1 269 416 500 m3 (145 m3 ha-1) 35 872 293 m3 year-1 (4.1 m3 ha-1) |
25.9 Mt year-1 of fuelwood potentially available from coppices ([42]) | |
Annual cuts | 6 500 000 m3 ([62]) Felling rate: 18% |
1 700 000 m3 from plantation forests ([61]) Natural forests: 14% |
|
Main forest types | Other oaks European beech Turkey oak Hornbeams Chestnut Holm oak Norway spruce European larch Black locust Hybrid poplars |
1 084 000 ha 1 035 000 ha 1 011 000 ha 852 000 ha 788 000 ha 620 000 ha 586 000 ha 382 000 ha 234 000 ha 66 000 ha |
Quercus petraea, robur, pubescens Fagus sylvatica Quercus cerris Carpinus betulus, Ostrya carpinifolia Castanea sativa Quercus ilex Picea abies Larix decidua Robinia pseudoacacia Populus x euroamericana |
Forest ownership and management |
66.2% private 42% coppice, 58% high forest 15.7% with detail management plan 14.4% with roads within 25m |
Mean size of property: 7.5 ha ([61]) 89% of coppices are at or beyond rotation age |
|
Non-wood products and services |
Protection from natural hazards Recreation and cultural use Water quality Habitat and biodiversity Carbon sequestration |
87.1% with management restrictions unquantified unquantified 28.5% in protected areas 55 Mg/ha (aboveground) |
|
Main risks | Wildfire - Storm damage Biotic agents Domestic and wild ungulates Gravity-related land degradation |
16 000 to 116 000 forest ha year-1 ([35]) 5.6% of forest area is currently damaged 9% (insects, fungi) 3.2% (grazing damage) 14 % (6% rockfall, 4% erosion, 0.5% avalanche) |
The figures reported in Tab. 1 reflect the average or summary state of Italian forests, but variability is extremely high within the country. Differences in elevation (0 to 4810 m a.s.l.), climate regime (mediterranean to oceanic or continental), past and current land use, social and economic factors between regions or even adjacent valleys, give origin to a fine-grained mosaic of sites, stand structures and forest cover types. Such a diverse pattern might be one of the reasons for the lack (until recently) of a forest modeling tradition. We will report the state of the art of forest modeling in Italy following the classification scheme proposed by Pretzsch et al. ([95]).
Empirical models
Statistical stand models such as yield tables have been developed over the past fifty years for the most productive forests of the country (e.g., [10], [11], [27], [4], [25], [29]) but, like all empirical models, are hardly applicable in sites other than those they were calibrated for and they do not take into account climate changes. Moreover, some of such tables are now outdated, because they do not reflect the changes occurred since theiy were developed in site conditions or management operations. Empirical stand-scale models may still be useful as decision support systems (DSS) aimed at simulating the average development of stand structure and the provision of related forest services over well-defined areas and short to medium timespans. For example, Vacchiano et al. ([115]) developed a stand density management diagram for Scots pine (Pinus sylvestris L.) forests with a direct protective role against rockfall.
Size distribution models, on the other hand, have never obtained much practical relevance in Italy. As a notable exception, Markovian models of the transition probability between diameter classes ([20]) have been suggested for mixed, uneven-aged forests of the eastern Alps ([122], [51]).
Individual tree models explicitly simulate the development of single trees considering their interactions within a spatial-temporal system, and account for feedback loops between stand structure and individual growth. This enables them to simulate pure and mixed stands of all age structures and intermingling patterns equally well. Stand-level data for forestry management are finally provided by aggregation of the single tree results ([95]). Individual-tree empirical models have previously been designed for alpine Beech (Fagus sylvatica L.) forests ([28]), Douglas fir and hybrid poplar plantations ([105], [34], [32]) and are currently being developed to forecast yield in plantations for quality timber such as common walnut (Juglans regia L. - D. Cimini, personal communication). Morani ([80]) showed the potential of UFORE, an individual-tree growth model for predicting the dynamics and air-quality benefits of planted trees in an urban context.
Depending on the modeling purpose, several individual growth and yield simulators might be available from the international literature, e.g., CAPSIS ([41]), MOSES ([56]), SILVA ([96]) and the Forest Vegetation Simulator (FVS - [39], based on early work by [112]). Issues of accuracy and scale have been associated to the use of empirical growth and yield models in Europe ([33]). Those who intend to adopt them face two major challenges: (1) the availability of repeated forest inventories for the focus landscape that provide the input and output variables needed for calibrating empirical growth equations; (2) the inclusion of the impact of climate and site changes on future productivity ([47]). To accomplish the latter, model developers must link their input to external, process-based or bioclimatic envelope models, as discussed in his invited talk at the FMWG meeting by Nicholas Crookston from the US Forest Service ([36]).
Gap, hybrid and landscape models
Gap models ([21]) and, on a larger scale, landscape dynamics models ([59]), explicitly include site and climate drivers for predicting forest composition, structure and biomass.
Small-area or gap models reproduce the growth of single trees in forest patches (e.g., 100 m2) in relation to the prevailing growth conditions at the site ([17], [108], [67]). However, physiological processes are not explicitly accounted for, requiring statistical fitting procedures between each environmental factor and observed growth. The combination of knowledge on specific eco-physiological process with stand or single tree management models and with long-term growth measurements results in the so-called hybrid growth models ([64]). In Italy, no developments of either gap or hybrid models have been proposed to date; SORTIE-ND ([86]) might represent a suitable simulator for future adaptations.
Landscape models comprise a broad class of spatially explicit models that incorporate heterogeneity in site conditions, neighborhood interactions and feedbacks between different spatial processes ([95]). The role of these models is to develop scenarios for the sustainability of forest or landscape functions (natural resources, habitat, hydrology, socioeconomic), to forecast their response to disturbances and potential environmental change (climate, N deposition, land use), to analyze the relationship between landscape structure and regionally distributed risks, and to assess regional-scale matter fluxes, e.g., water, carbon and nutrients. One example is the mesoscale SILVA Land Surface Model ([1]) that represents the momentum, heat and water flux at the interface between land-surface and atmosphere, and has been coupled to a general circulation model (GCM) to estimate the rate of forcing by existing vegetation on precipitation patterns. At a different scale, other examples of spatially explicit landscape modeling presented at the FMWG meeting are calibrated of fire spread and behavior simulators to a Mediterranean ecosystem by Arca et al. ([5]) and eco-hydrological models currently used to forecast water (runoff, snowmelt, evapotranspiration, uptake) and energy (heat, radiation) budgets at the plot and catchment scale ([71], [100], [12]).
Landscape models should be distinguished from models based on spatial data layers at the landscape or regional scale, but without the explicit representation of neighborhood interactions. These should be rather viewed as local models embedded into geographic information systems (GIS). Output variables are predicted based on their relationship with topographic, climatic, biometric or ecophysiological information, either ground-based or remotely-sensed. The link between input and output variables is often based on empirical relationships or multivariate and multi-criteria analysis. Examples were given in the fields of fire risk prediction (e.g., [120], [66], [24]), habitat suitability ([13], [46], [19]), and plant species distribution in response to climate change scenarios ([7]).
Alternatively, GIS-based models can incorporate detailed information on ecophysiological processes, as for the development of the 3PG-s model presented by Nolé at the FMWG meeting ([31], [83]).
Matter-balance models
Most of the simulators presented at the FMWG meeting were focused on process based models (PBMs) and widely described in literature. Simulated processes involve primary productivity (3-PG - [65]; FOREST-BGC - [125]), nitrogen (3-PGN - [129]), and carbon fluxes (C-FIX - [121]), including estimation of C sequestration (NASA-CASA - [91]), heterotrophic ([83]) and autotrophic respiration ([77]). Models in the process-based family that are closest to the operational application stage can simulate growth and yield of a single stand ([70]). However, there are also models being developed to address larger, regional and successional scale problems, e.g., GIS-based models of stand development in the tropics ([38]). Process-based modeling can be defined as a procedure by which the behavior of a system is derived from a set of functional components and their interactions with each other and the system environment, through physical and mechanistic processes occurring over time ([52], [16]). Matter balance, or process-based, models focus on the description of water and nutrient (C and N) balance, based on biogeochemical processes. They consider vegetation development primarily as a change of matter in different compartments based on uptake (e.g., photosynthesis) and loss (e.g., mortality and biomass turnover) processes that in turn depend on environmental conditions ([95]). Many of these models use the well-known light use efficiency approach (or radiation use efficiency), which estimates the conversion efficiency of absorbed photosynthetically active radiation (APAR) into gross primary production (GPP). The tenet is that GPP is a linear function of APAR reduced by environmental constraints on Light Use Efficiency ([74], [65], [102]) and integrating the ecophysiological processes related to the carbon and water balance. A large validation effort has been made in recent years for these type of models, often using satellite data to develop spatially-explicit model versions, with a high simplification in the Leaf Area Index or fraction of absorbed PAR computation (i.e., 3-PGs - [82]; C-FIX - [121]).
Many process based models were developed for even-aged, monospecific stands (plantations), a fact that may reduce their usability in forests with highly complex structure. Efforts are underway by the authors to adapt simple models developed for tropical rainforests into more complex models, able to consider the presence of more than one cohort or species or integrating light competition within layered models ([30]).
Emerging themes and challenges
The FMWG meeting showed that the currently prevailing interest in the Italian forest modeling community is for the process-based modeling of forest ecosystem productivity, based on knowledge of physiological and ecosystem processes. This may be due to several reasons: the complexity of forest landscapes, the shift from a timber-oriented to an ecosystem- or even carbon-oriented management paradigm, the lack of homogenized inventory data for calibration of empirical models, or else the recently established body of research and data on carbon cycling and sequestration in European forest ecosystems, e.g., measurements from the Fluxnet and CarboEurope networks ([8], [116]).
Process models have clear advantages over empirical models, allowing researchers to answer some of the most pressing questions in current plant ecological science, such as the amount of carbon fixed by a given community or landscape, or the response of tree performance to climatic fluctuations or atmospheric N depositions (e.g., [44]). Provided that appropriate data be available as input variables (see below), we suggest directing research towards understanding ecosystem response to exogenous disturbances. Applications of process models have been run so far in controlled environments, i.e., forests or landscapes driven only by endogenous dynamics, with different climate input parameters. Since climate change is likely to affect disturbance regimes ([37]), which in turn have a strong impact on forest productivity, the study of tree and stand response to disturbances of different kinds and intensities is a desirable integration to the current modeling framework. Natural disturbance patches and events, from both abiotic and biotic agents, can serve as case studies to retrospectively test existing individual tree and stand-scale models. Moreover, specific simulators have been developed to model disturbance-related risk and impact in forest ecosystems, and could be useful for both management and ecological research ([55], [106]).
A further example of an underdeveloped research area is represented by forest-wildlife interactions, with specific reference to ungulate browsing on regeneration. Satisfactory models (empirical or GIS-based) already exist to define habitats suitable to animal species of interest. However, both process- based and growth and yield models usually lack a reliable regeneration algorithm ([97]); including the selective impact of mammal (or even insect) herbivory is a necessary step towards the simulation of actual ecosystem dynamics, especially for mountain forests ([126]).
In order to be both usable and useful for managers, models must be kept as simple as possible; adding sub-components without increasing prediction error and/or decrease usability is a difficult task. Nevertheless, two features emerged at the FMWG meeting that should be considered for general integration within existing simulators: (1) soil-related processes and (2) spatial referencing of model output. The role of soil organic matter in forest carbon budget appears at the same time very influential ([69]) and poorly understood, principally because of lacking field data. Models addressing soil-related dynamics or incorporating soil sub-components have been developed (BIOME-BGC, G-DAY, Century; see e.g. [103], [130]). More data and research are needed to improve their predictions, linking soil carbon budget to deadwood dynamics and root assimilation, and finally estimating belowground C pools for integration into aboveground simulations.
The second feature, i.e., making model outputs attributable to spatial units in the landscape, was briefly explored during the meeting, but only at the scale of nation- or region-wide simulations of carbon stocks ([73]) or statistical climate-species response profiles ([7]). Following the example of hydrological models, which by definition work on landscape cells in catchments, simulators of stand composition, biomass and productivity should predict the spatial variability of such attributes across a forested landscape. Not only managers would benefit from a landscape-explicit approach, but research about landscape connectivity, fragmentation, energy flows and functional relationships between ecosystem components would be made possible. An example of such an approach are the simulators LANDIS-II ([104]), that links process-based estimates of forest composition and growth to landscape pixels of variable size (typically 10 to 500 meters), and iLand ([107]), that adopts a hierarchical multi-scale modeling framework scaling up from individual trees to the landscape.
At any rate, models should be tailored towards the final users, be they researchers or land managers. Empirical models, where adequately calibrated, may be the best option to pursue when forest composition, yield and structure need to be described at the stand scale and for limited temporal spans. Empirical relationships or functions are often used when modeling emergent properties such as self-thinning and mortality dynamics, that have not been predicted to date by physiological processes at the individual tree level. Research on hybrid models, capable of transferring specific eco-physiological process knowledge to stand or single tree management models that are evaluated against long-term growth measurements, has been indicated as a priority for forest ecology throughout ([64], [63]).
Further challenges for modelers
A number of the issues emerged from the panel discussion of the FMWG meeting directly concerning the modelers’ community, rather than the ecological or silvicultural applications of the models. Here we briefly summarize the major points of concern:
- Data availability. Data for calibration and validation of models at all scales are not sufficiently available, due to a lack of long-term ecological studies, poor harmonization of available datasets, and poor dissemination of existing data sources (including the National Forest Inventory - INFC, see [14]). We recommend that bottlenecks in data collection and analysis be avoided as strongly as possible, and advocate for open release of raw data from the INFC following the example of some European countries (such as France or Spain) and the United States. We also think that a stronger interaction between modelers and other research communities, including field surveyors, would improve and homogenize data collection standards across studies and sites.
- Model documentation and availability. While open-sourcing a simulator remains a developer’s choice, each piece of software or equation should be accompanied by adequate metadata and documentation, in order to respect science’s transparency and repeatability canons. While metadata would improve adaptation of existing models to new species or sites, newly developed models should be registered together with their relevant documentation on existing online archives (see Introduction). Metadata templates could also be introduced in data collection routines ([76]).
- Calibration and validation. Validation of model output against independent data is a too-often missing component when reporting results of model-related research. We advise recurring to multiple data sources for evaluation of model output, e.g., by integrating field-based and remotely-sensed data, or by assessing the ecological realism of ecosystem behavior as predicted by a simulator (model “evaluation” sensu [119]). Recently developed tools for model calibration, validation and performance comparison could be of more general use, including Bayesian calibration ([117]), resampling methods ([72]), and the use of information theory ([6]).
- Issues of scale. Modelers should have an understanding of all the process represented in their simulators. They ought to recommend the most appropriate spatio-temporal scale of simulation and application of the results, in order to avoid undesired propagation of model error due to larger-than-appropriate temporal or spatial extents. Upscaling or downscaling model predictions is often carried out too lightly, as it would require an understanding of functional relationships between scales (both grain and extent, sensu [128]) and a hierarchical approach to ecosystem modeling ([84], [101]). A successful attempt to scale up from stand to continental scale was recently developed by Franklin et al. ([49]).
Conclusion
As a conclusive remark, we would like to make a case for communication. Scientists involved in ecological research should make all efforts for an effective communication of their results, especially when they can be applied for a better management of natural resources. Even when not solicited by explicit demand, model developers should make all efforts to transfer information enclosed by model output to all stakeholders and to the general public (or even the press). Contributors to COST project FP0603 are developing guidelines for forest sustainability indicators to be included as model outputs following Pan-European criteria and indicators for ecological, economic and social sustainability ([75]). However, the choice of model output variables should be tailored on prospective users, a process that could be made very effective by preliminary interaction between the two categories of subjects, in order to avoid “ivory tower” behaviors. Participation of stakeholders to forest planning is a promising field and has already been successfully implemented in some cases. Communicating with end-users, spreading “user friendly” releases of simulator software, equipping models with realistic visualization tools, and providing continuing education and training, will deserve great attention and effort if forest simulators are desired to serve as real decision support tools, and not mere computational exercises.
References
Gscholar
Gscholar
CrossRef | Gscholar
Gscholar
Gscholar
Gscholar
Gscholar
Gscholar
CrossRef | Gscholar
Gscholar
Gscholar
Gscholar
Gscholar
CrossRef | Gscholar
Gscholar
Gscholar
Gscholar
Gscholar
Gscholar
Gscholar
Gscholar
Online | Gscholar
Gscholar
Gscholar
Gscholar
Gscholar
Gscholar
Gscholar
Gscholar
Gscholar
Gscholar
Gscholar
Gscholar
Gscholar
Gscholar
Gscholar
Gscholar
Gscholar
Gscholar
Gscholar
Gscholar
Gscholar
Gscholar
Gscholar
CrossRef | Gscholar
Gscholar
Gscholar
CrossRef | Gscholar
Gscholar
Gscholar
Gscholar
CrossRef | Gscholar
Gscholar
Gscholar
Gscholar
Gscholar
Gscholar
Gscholar
Gscholar
Gscholar
Gscholar
Gscholar
Gscholar
Gscholar
Footnotes
Authors’ Info
Authors’ Affiliation
Department of Agriculture, Silviculture and Land Management, Università di Torino, v. L. da Vinci 44, I-10095 Grugliasco (TO - Italy)
Department of Fruit Tree and Woody Plant Science, Università di Bologna, v.le Fanin 46, I-40127 Bologna (Italy)
Impacts on Agriculture, Forest and Natural Ecosystem division (IAFENT), Centro Euro-Mediterraneo per i Cambiamenti Climatici, v. Augusto Imperatore 16, I-73100 Lecce (Italy)
Corresponding author
Paper Info
Citation
Vacchiano G, Magnani F, Collalti A (2012). Modeling Italian forests: state of the art and future challenges. iForest 5: 113-120. - doi: 10.3832/ifor0614-005
Academic Editor
Marco Borghetti
Paper history
Received: Mar 14, 2012
Accepted: Apr 10, 2012
First online: Jun 05, 2012
Publication Date: Jun 29, 2012
Publication Time: 1.87 months
Copyright Information
© SISEF - The Italian Society of Silviculture and Forest Ecology 2012
Open Access
This article is distributed under the terms of the Creative Commons Attribution-Non Commercial 4.0 International (https://creativecommons.org/licenses/by-nc/4.0/), which permits unrestricted use, distribution, and reproduction in any medium, provided you give appropriate credit to the original author(s) and the source, provide a link to the Creative Commons license, and indicate if changes were made.
Web Metrics
Breakdown by View Type
Article Usage
Total Article Views: 53070
(from publication date up to now)
Breakdown by View Type
HTML Page Views: 44217
Abstract Page Views: 2751
PDF Downloads: 4749
Citation/Reference Downloads: 29
XML Downloads: 1324
Web Metrics
Days since publication: 4435
Overall contacts: 53070
Avg. contacts per week: 83.76
Article Citations
Article citations are based on data periodically collected from the Clarivate Web of Science web site
(last update: Feb 2023)
Total number of cites (since 2012): 19
Average cites per year: 1.58
Publication Metrics
by Dimensions ©
Articles citing this article
List of the papers citing this article based on CrossRef Cited-by.
Related Contents
iForest Similar Articles
Research Articles
Local ecological niche modelling to provide suitability maps for 27 forest tree species in edge conditions
vol. 13, pp. 230-237 (online: 19 June 2020)
Research Articles
Distribution factors of the epiphytic lichen Lobaria pulmonaria (L.) Hoffm. at local and regional spatial scales in the Caucasus: combining species distribution modelling and ecological niche theory
vol. 17, pp. 120-131 (online: 30 April 2024)
Research Articles
Tree volume modeling for forest types in the Atlantic Forest: generic and specific models
vol. 13, pp. 417-425 (online: 16 September 2020)
Research Articles
Total tree height predictions via parametric and artificial neural network modeling approaches
vol. 15, pp. 95-105 (online: 21 March 2022)
Research Articles
Allometric models for estimating biomass, carbon and nutrient stock in the Sal zone of Bangladesh
vol. 12, pp. 69-75 (online: 24 January 2019)
Research Articles
A comparison of models for quantifying growth and standing carbon in UK Scots pine forests
vol. 8, pp. 596-605 (online: 02 February 2015)
Research Articles
Exploring machine learning modeling approaches for biomass and carbon dioxide weight estimation in Lebanon cedar trees
vol. 17, pp. 19-28 (online: 12 February 2024)
Research Articles
Modelling the carbon budget of intensive forest monitoring sites in Germany using the simulation model BIOME-BGC
vol. 2, pp. 7-10 (online: 21 January 2009)
Research Articles
Incorporating management history into forest growth modelling
vol. 4, pp. 212-217 (online: 03 November 2011)
Research Articles
Aboveground tree biomass of Araucaria araucana in southern Chile: measurements and multi-objective optimization of biomass models
vol. 14, pp. 61-70 (online: 09 February 2021)
iForest Database Search
Search By Author
Search By Keyword
Google Scholar Search
Citing Articles
Search By Author
Search By Keywords
PubMed Search
Search By Author
Search By Keyword