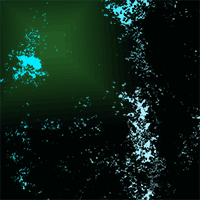
Estimation of canopy attributes of wild cacao trees using digital cover photography and machine learning algorithms
Julio Martin Duarte-Carvajalino (1) , Mónica Paramo-Alvarez (2), Pablo Fernando Ramos-Calderón (3), Carlos Eduardo González-Orozco (4)
iForest - Biogeosciences and Forestry, Volume 14, Issue 6, Pages 517-521 (2021)
doi: https://doi.org/10.3832/ifor3936-014
Published: Nov 17, 2021 - Copyright © 2021 SISEF
Short Communications
Abstract
Surveying canopy attributes while conducting fieldwork in the rain forest is time-consuming. Low-cost imagery such as digital cover photography is a potential source of information to speed up the process of vegetation assessments and reduce costs during expeditions. This study presents an image-based non-destructive method to estimate canopy attributes of wild cacao trees in two regions of the rain forest in Colombia, using digital cover photography and machine learning algorithms. Upward-looking photography at the base of each cacao tree and machine learning algorithms were used to estimate gap fraction (GF), foliage cover (FC), crown cover (CC), crown porosity (CP), clumping index (Ω), and leaf area index (LAI) of the canopy cover. Here we used the cacao wild trees found on forestry plots as a case study to test the application of low-cost imagery on the extraction and analysis of canopy attributes. Canopy attributes were successfully extracted from the canopy cover imagery and provided 92% of classification accuracy for the structural attributes of the canopy. Canopy cover attributes allowed us to differentiate between canopy structures of the Amazon and Pacific rainforests sites suggesting that wild cacao trees are associated with different vegetation types. We also compare classification results for the computer extraction of canopy attributes with a digital canopy cover benchmark. We conclude that our approach was effective to quickly survey canopy features of vegetation associated with and of crop wild relatives of cacao. This study allows highly reproducible estimates of canopy attributes using cover photography and state-of-the-art machine learning algorithms such as deep learning Convolutional Neural Networks.
Keywords
Canopy Attributes, Cover Photography, Colombia, Machine Learning, Deep Learning
Authors’ Info
Authors’ address
Corporación Colombiana de Investigación Agropecuaria - AGROSAVIA, Centro de Investigación Tibaitatá, Km 14 vía Mosquera, Bogotá, Cundinamarca (Colombia)
Corporación Colombiana de Investigación Agropecuaria - AGROSAVIA, Sede Central, Km 14 vía Mosquera, Bogotá (Colombia)
Corporación Colombiana de Investigación Agropecuaria - AGROSAVIA, Centro de Investigación Nataima, Km 9 vía Espinal-Chicoral, Tolima, Sede Florencia, Caquetá (Colombia)
Corporación Colombiana de Investigación Agropecuaria - AGROSAVIA, Centro de Investigación La Libertad, Km 14 vía Villavicencio, Puerto López, Meta (Colombia)
Corresponding author
Paper Info
Citation
Duarte-Carvajalino JM, Paramo-Alvarez M, Ramos-Calderón PF, González-Orozco CE (2021). Estimation of canopy attributes of wild cacao trees using digital cover photography and machine learning algorithms. iForest 14: 517-521. - doi: 10.3832/ifor3936-014
Academic Editor
Nicola Puletti
Paper history
Received: Jul 23, 2021
Accepted: Sep 08, 2021
First online: Nov 17, 2021
Publication Date: Dec 31, 2021
Publication Time: 2.33 months
Copyright Information
© SISEF - The Italian Society of Silviculture and Forest Ecology 2021
Open Access
This article is distributed under the terms of the Creative Commons Attribution-Non Commercial 4.0 International (https://creativecommons.org/licenses/by-nc/4.0/), which permits unrestricted use, distribution, and reproduction in any medium, provided you give appropriate credit to the original author(s) and the source, provide a link to the Creative Commons license, and indicate if changes were made.
Web Metrics
Breakdown by View Type
Article Usage
Total Article Views: 28714
(from publication date up to now)
Breakdown by View Type
HTML Page Views: 25620
Abstract Page Views: 1378
PDF Downloads: 1369
Citation/Reference Downloads: 4
XML Downloads: 343
Web Metrics
Days since publication: 1234
Overall contacts: 28714
Avg. contacts per week: 162.88
Citation Metrics
Article Citations
Article citations are based on data periodically collected from the Clarivate Web of Science web site
(last update: Mar 2025)
Total number of cites (since 2021): 2
Average cites per year: 0.40
Publication Metrics
by Dimensions ©
Articles citing this article
List of the papers citing this article based on CrossRef Cited-by.
References
Estimation of canopy attributes in beech forests using true colour digital images from a small fixed-wing UAV. International Journal of Applied Earth Observation and Geoinformation 47: 60-68.
CrossRef | Gscholar
An intensity, image-based method to estimate gap fraction, canopy openness and effective leaf area index from phase-shift terrestrial laser scanning. Agricultural and Forest Meteorology. 280: 107766.
CrossRef | Gscholar
Learning internal representations by error propagation. In: “Parallel Distributed Processing: Explorations in the Microstructure of Cognition” (Rumelhart DE, McClelland JL, Williams RJ eds). MIT Press, Cambridge, MA, USA, vol. 1, pp. 318-362.
Gscholar