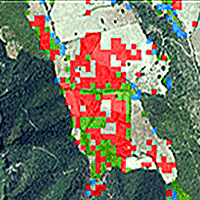
Change Detection methods for forest expansion assessment in the last twenty years in the Mediterranean Basin
iForest - Biogeosciences and Forestry, Volume 18, Issue 2, Pages 69-78 (2025)
doi: https://doi.org/10.3832/ifor4634-018
Published: Apr 16, 2025 - Copyright © 2025 SISEF
Research Articles
Abstract
The evaluation of natural forest expansion is a crucial issue for understanding fundamental processes related to climate change and carbon uptake. The identification of forest expansion/reduction dynamics at a regional scale has been provided through multitemporal analyses of high-resolution satellite images. This study aims to compare different Change Detection (CD) techniques to assess forest expansion in the Basilicata region (Southern Italy). Landsat 5 TM image from 2003 and Landsat 8 OLI image from 2019 were used for this purpose. The CD methods implemented were NDVI Differencing and Post-Classification Comparison (PCC). Using a confusion matrix, PCC showed better performance than NDVI Differencing (Overall Accuracy of 0.85 and 0.62, respectively). The comparison of the evaluated forest expansion areas with inventory data shows the good performance of CD procedures in assessing forest land use changes. The use of satellite images and Change Detection techniques can be applied where a high temporality and a wide geographical extension are required, conditions in which traditional procedures involving field surveys are too expensive in terms of time and money. Furthermore, the use of satellite images allows for the detection of changes in the Earth’s surface in particularly inaccessible areas of the globe.
Keywords
Change Detection, Forest Expansion, NDVI-Differencing, Post-Classification Comparison, PCC, Land Use Change, Climate Change, Land Abandonment, Mediterranean Land System Dynamics
Introduction
Land use/land cover (LULC) can be defined as the set of land surface features that include both natural and anthropogenic elements covering the Earth’s surface and is one of the most important environmental factors ([18]). It is evident that, in relation to both anthropogenic and naturally occurring factors, as well as climate change, land-use/land-cover (LULC) varies at varying rates over time and space ([64]).
The identification of LULC change is essential for managing resources, protecting ecosystems and their biodiversity, biogeochemical cycles, soil fertility, hydrological cycles, energy balance, soil productivity and ecosystem services ([36]). Moreover, LULC change is a key decision support for sustainable development, representing the most critical aspect of global environmental change ([64]).
The more rapidly land use changes, the more drivers (climatic, socio-economic, and political) are found to be acting simultaneously in a given area. These factors and their mechanisms operate at different spatio-temporal scales ([30]). Indeed, the factors that cause significant changes in land use involve a complex system of dependencies and interactions, affecting a wide range of sectors at different spatial and temporal levels. In recent decades, land use changes have become a major concern in studies focused on global warming ([30]), the varying capacities of natural resources to sequester carbon, and the ability of different ecosystems to adapt to climate change ([69]). The analysis of LULC change consists of identifying how and where changes are occurring, the rates at which they are occurring, and the socio-economic factors driving these changes ([47]).
A key element of spatio-temporal variation of LULC is that forest dynamics can involve contrasting processes of deforestation and natural forest expansion ([16]). Forests contribute to the economic development of many countries by providing livelihood for millions of people. They also provide a wide range of ecosystem services and serve as important carbon sinks, contributing to the reduction of carbon dioxide in the atmosphere ([21]). Forests resulting from the natural processes of expansion can contribute to improved hydrological conditions, increased biodiversity and productivity of agricultural systems, climate change mitigation and adaptation, and increased ecosystem services, in general ([44]). However, uncontrolled forest expansion can also have adverse effects by causing a range of social, environmental, and economic impacts, including loss of cultural landscapes and habitat diversity, reduced levels of biodiversity and eco-diversity ([63]), waste of economic and natural resources, loss of productive land, negative impacts on agricultural productivity and human health, depletion of some environmental services, and an increase in natural hazards such as floods, landslides, and fires ([27]). Socio-economic dynamics related to forest expansion in many Mediterranean areas, especially mountainous regions, are also significant. This included issues like population aging, depopulation, marginalization, and the erosion of traditional rural knowledge ([52]).
Bastin et al. ([4]) estimated that, globally, 9 million km2 of land area have suitable conditions for forest expansion, either through replanting new forest stands or by natural forest expansion. However, the phenomenon can be observed at small scales and local levels worldwide, primarily due to social factors related to land abandonment ([32]). To comprehend these land use changes, it is essential to place them within the framework of environmental policies that promote the development of multifunctional landscapes and enhance ecosystem services, especially those connected to regulatory and cultural aspects. Forests represent important carbon sinks: from 2001 to 2010, the carbon absorbed by forest recolonization areas accounted for more than 60% of the total carbon sink of forests (1.3 out of 2.15 Pg yr-1), and the carbon stored by forests accounted for approximately 45% of the total carbon pool of terrestrial ecosystems. Temperate forests represent an important carbon sink in this context ([54]). Carbon sinks from natural forest expansion are primarily represented by deciduous broadleaves and evergreen conifers in temperate zones, while the majority of the world’s forests are tropical and boreal. In temperate habitats, the processes of forest recolonization can be easily traced back to the abandonment of silvicultural activities ([16]). In Europe, the largest increase in forest area (approximately 35%) has been recorded in eastern Europe and can be attributed to natural processes of forest recolonization of agricultural lands that have been abandoned following the collapse of the former Soviet Union ([62]). Mediterranean Europe has also experienced a relatively sustained increase in forest area in recent years, primarily due to natural forest expansion. In the Mediterranean regions, this is fundamentally linked to the abandonment of agricultural practices and to the reduction in livestock pressure, which have been the primary drivers of change in the Italian rural landscape over the last fifty years ([52]). The causes can be traced back to rural migration, crop abandonment, particularly in mountainous areas, and the intensification of agricultural production in lowland areas ([13]).
Assessing the expansion of natural forests can enhance understanding of fundamental processes, particularly those related to climate change and carbon uptake. Conventional methods of forest mapping to identify expansion/reduction dynamics by field surveys are very costly in terms of financial and human resources, and extremely challenging in rugged morpho-topographic areas. The growing variety and availability of remote sensing data, which is often freely accessible, along with advanced remote data processing technologies, allows to obtain information on forest expansion at different scales and at a low cost ([22]). In this context, data obtained from various Landsat missions has proven invaluable for conducting multitemporal analyses on forest expansion dynamics due to its good spectral, geometric, and temporal resolution ([11], [39], [32]) and its extensive coverage of nearly 50 years. Additionally, remotely sensed data has yielded positive outcomes in producing forest maps ([22]) and studying the stages of forest stand growth ([10]), taking into account the scale and radiometric and geometric resolution of the changing areas. Short satellite revisiting times, the integration of remote sensing data from various missions and satellites, continuous improvements in geometric and spectral image resolution, and the increasing sophistication of change detection techniques all contribute to the ability to monitor large-scale changes on the Earth’s surface in very short time.
Change detection is the process of identifying differences in the state of an object by observing it at different times ([59]). Since the 1980s, numerous reviews on change detection techniques have been published ([59], [35], [29]), including analyses of the accuracy of maps derived from change detection analysis ([45]). These techniques have been applied in many fields, allowing not only the assessment of LULC changes but also their intensity, trajectories, and the drivers involved ([31]). In the forestry sector, change detection techniques have been applied to identify the extent, type, and dynamics of forest expansion ([34], [65]), forest biomass estimation ([19]), the detection of biotic and abiotic damage ([25]) or forest fires ([70]), the assessment of fire-prone areas ([6]), and forest responses to climate change ([57]). However, the improvement of change detection algorithms and procedures in forestry is an ongoing challenge ([60], [39]) because of several potential source of errors in image analysis, such as the effect of atmospheric scattering, the presence of clouds and shadows, or different sun angle ([26]), but also to the variability of the radiometric response of vegetation as a function of topography, forest type, and phenology ([14]).
Several change detection algorithms, techniques, and procedures have been reported in the scientific literature, though none of them is deemed suitable to every scenario ([35]). Desclée et al. ([15]) identified three main groups of change detection techniques: visual interpretation, pixel-based and object-based methods. The first is time-consuming as changes are identified by analyzing the shape, tone, texture, pattern, size, and association of various objects on the Earth’s surface; it also requires substantial operator experience ([35]) and is necessarily applied to limited areas. Pixel-based methods are the most widely used procedures in change detection analysis and provide satisfactory results in short times ([7]), though they are considered scene-dependent ([55]). More recently, object-based change detection methods have been proposed that combine visual interpretation and quantitative aspects, taking into account the similarity of contiguous pixels ([49]).
Pixel-based methods represent the most widely used and the better-performing technique of change detection ([66]). Within this category two different approaches are included: (i) an approach based on simultaneous multitemporal analysis of multispectral data using the single spectral bands or, more commonly, an index (e.g., NDVI) derived from the combination of the individual spectral bands; (ii) a post-classification approach, involving the independent classification of two different dates to identify the occurred changes. The above methods have often demonstrated better performance than other algorithms and procedures, though each algorithm has several advantages and disadvantages in detecting changes ([5]).
The study aims to identify and quantify the natural forest recolonization during the last two decades in an area of the Mediterranean basin (Basilicata, southern Italy) by comparing two different change detection techniques: NDVI Differencing ([41]) and post-classification comparison ([37]). We highlighted the strengths and weaknesses of each procedure and discussed the importance of accuracy analysis for performance evaluation.
Materials and methods
Study area
The study area is the Basilicata region (southern Italy) in the middle of the Mediterranean Basin (Fig. 1). The landscape in the Mediterranean area is characterized by highly variable morpho-topographic habitats, corresponding to very different climatic conditions at the local level. The diverse landscapes, shaped by a long history of human interaction and recent changes driven by climate change ([56]) and land management policies, have resulted in the development of highly varied ecosystems. These landscapes comprise agricultural areas interspersed with small-scale tree crops, horticultural crops, hayfields, and patches of natural vegetation, including different forest types with varied physiognomy and structure. The Apennine mountain range dominates the western part of the region, which has a typical oromediterranean climate. In contrast, eastern territories are characterized by low hills and large valleys that gradually flatten towards the southern coast of the Ionian Sea, where the climate is typically Mediterranean, with hot, dry summers and cold autumn and winter months, when most rainfall occurs.
Fig. 1 - Location of the study area. (A) Basilicata Region in southern Italy; (B) Landsat 5 TM of July 27, 2003 (false colors); (C) Landsat 8 OLI of July 7, 2019 (false colors).
Forests cover approximately 36% (355.367 ha) of the total area of the region. Deciduous oak forests are prevalent, accounting for 51.8% of the forest cover. Beech forests can be found at higher altitudes (8.4%), while as elevation decreases, more thermophilous forest types are encountered, such as Mediterranean macchia (7.9%), thermophilous shrublands (6.9%), and garrigue. Reforested areas are also present, with Pinus nigra predominant at higher altitudes and Pinus halepensis in the hills and lowlands.
Notably, the Mediterranean Basin is considered one of the regions most vulnerable to climate change, with future projections predicting an increase of approximately twice the average global warming temperature ([48]). This has implications for vegetation dynamics and changes in land use between agricultural and forested areas, which can significantly impact the carbon balance and sequestration in different ecosystems ([57]).
Remote sensing data and image pre-processing
Forest dynamics can only be accurately described using medium- to high-resolution imagery, such as Landsat, ASTER, SPOT, and Sentinel ([15]). The Landsat Program is one of the most important sources of remote-sensed data for Change Detection analyses ([61]), with appropriate preprocessing of images derived from different sensors (TM, ETM+, OLI), although they are very similar ([58]).
To identify areas of forest recolonization, we used images from NASA’s Landsat mission, which provided global and moderate-resolution data since 1972. Landsat images are deemed suitable for change detection at regional and local scales ([33]). Additional imagery before 1972 has a broad revisiting time and a low resolution for local-scale applications, while missions providing data at medium to high resolution (e.g., Copernicus) are still too recent and do not span the whole study period (2003-2019).
A careful analysis of the images available on the USGS (United States Geological Survey) Earth Explorer data portal (⇒ https://earthexplorer.usgs.gov/) was preliminarily conducted. Following the recommendations by Häme et al. ([23]) and Coppin et al. ([12]), two summer images with very low cloud cover were selected, both falling in tile 188/32: (i) a Landsat 5 TM image from July 27, 2003; and (ii) a Landsat 8 OLI image from July 7, 2019. The acquisition dates of the two images differ by 20 days, but they were the only ones from the summer period that belong to the same month and show low cloud cover. Indeed, atmospheric conditions, soil and vegetation moisture conditions, and plant phenology at the time of acquisition are important factors to be considered to avoid artifacts in the radiometric response between different images ([67], [15]).
To remove possible interferences that may lead to the detection of unreal differences in land use over time, radiometric or atmospheric correction, normalization, and relative calibration of images should be applied ([71], [61]). For example, variations in solar illumination and atmospheric conditions during image acquisition, and differences due to sensor calibration, must be normalized. Calibration procedures involve the use of regression functions, and appear to yield better results than absolute radiometric correction ([11]). Song et al. ([61]) demonstrated that all the above-mentioned procedures can be effective to enhance the detection of land surface changes by comparing different radiometric and atmospheric correction algorithms. Image pre-processing initially involved the co-registration of images, which is particularly important in image differentiation techniques ([68]).
The Landsat 8 OLI product specifications indicate that the geometric accuracy is within 12 meters of that of previous Landsat sensors ([28]). Therefore, the two Landsat images underwent a pre-processing that included (i) co-registration of the images, (ii) removal of clouds and shadows using the Fmask algorithm, (iii) atmospheric correction ([61]) using the SV6 algorithm; (iv) radiometric normalization of the images using the Pseudo-Invariant Procedure (PIF); and (v) cropping of the images based on the Basilicata regional boundary. Post-classification comparison minimizes the effects of atmospheric and sensor differences between shooting dates ([17]). Still, Image Differencing technique is easier to apply, though it may lead to unsatisfactory results due to differences between the two images considered ([73]).
NDVI differencing method
Image Differencing ([8]) is a straightforward and interpretable technique that categorizes pixels into two groups: those that have changed and those that have not. The process involves subtracting the pixel value of the most recent image (taken at time t2) from that of the previous image (t1).
NDVI differencing represents the NDVI difference image of the Landsat images under consideration and is calculated as (eqn. 1):
Selecting a suitable threshold for change detection is a challenging and time-consuming process that requires considerable skill. Changes are detected using a probability density function of the difference image values in the tails of the distribution. Due to ease of implementation, this technique has been widely used for change detection ([1]), either using a single radiometric band or a color composite of three bands.
The Normalized Difference Vegetation Index (NDVI) is more commonly used to detect changes in vegetation ([38]). It is calculated using the normalized difference between the red and near-infrared bands. NDVI has a strong correlation with biomass, making it a valuable index for monitoring forest expansion ([39], [2]). The NDVI for the two selected images was calculated, and then the ΔNDVI was expressed in terms of standard deviation (σ) to identify the threshold for change detection ([12], [15], [53]). The use of standard deviation is one of the most widely used approaches for identifying the optimal threshold for change detection in remotely sensed imagery ([12], [35]).
Considering that the study is focused on the increase in NDVI between the two dates, the right tail of the distribution was considered. The best threshold for ΔNDVI was calculated as μ ± n·σ, where μ is the mean value of the distribution, σ the standard deviation, and n represents the dispersion around the mean. In a normal distribution μ is equal to zero, and in the central region of the distribution (μ - n·σ < ΔNDVI < μ + n·σ), it can be assumed that there were no significant changes in LULC, while in the right tail (ΔNDVI > μ + n·σ) and left tail (ΔNDVI < μ - n·σ) actual increase and decrease in plant biomass, respectively, are likely to be detected.
To identify the best threshold, a trial-and-test procedure was used to determine the optimal value of n·σ ([42], [51], [3]). The ROC curve ([43]) was calculated, which is a compromise between the true positive rate (TPR) and the false positive rate (FPR). Basically, we started with threshold values from ΔNDVI = μ + n·σ, where n increments by 0.01. A graph was constructed by plotting 1-specificity on the x-axis and sensitivity on the y-axis. Specificity refers to the frequency of true-negative results, while sensitivity refers to the frequency of true-positive results. The best threshold value was iteratively identified as the value that maximizes TPR while minimizing FPR, i.e., the point along the curve closest to the highest value on the y-axis.
Post-classification comparison (PCC) method
The post-classification comparison (PCC) procedure consists of extracting LULC information from the remotely sensed images independently for each considered date. More specifically, the method compares pixel by pixel two images acquired at different times and independently classified using a matrix for change evaluation ([29]). By operating independently on the two dates, the technique minimizes the disturbances caused by atmospheric and sensor differences ([35]) and reduces possible misregistration between images taken at two different times ([59]). PCC is one of the most frequently used procedures in change detection ([2], [46]). Mas ([42]) reports that PCC procedure is the most accurate one, as it provides indications of the nature of changes. However, PCC requires longer lead times, higher operator experience and greater skill to properly realize LULC maps properly. The effectiveness of this technique relies on the classification accuracy of the individual images ([72]) and the final accuracy is very similar to that obtained by multiplying the accuracies of the two individual classifications ([12]). In general, automatic, unsupervised classifiers provide unsatisfactory results, while semi-supervised or supervised techniques require ground-truth data for training the classification algorithm, leading to significantly better results.
The Maximum Likelihood Classifier (MLC) algorithm was the parametric and supervised classifier ([40]) that was employed to generate the land use maps for years 2003 and 2019. In the parametric approach, the data are assumed to be distributed according to a predefined probability model, and the parameters of this distribution depend on the input data (training sites). In MLC, unknown pixels are assigned to a specific class using the probability of the contours surrounding the training area, following the maximum likelihood approach. In our study, the ground truth dataset comprises 1000 control points, divided into two subsamples of 500 points each (Fig. 2). One of the point subsets was used for training the classifier and the other for validation. For the 2003 image classification, the forest/non-forest attribute of the control points was inferred from the Forest Map of Basilicata, whose field surveys are nearly coeval with the 2003 Landsat image. For the 2019 classification, the control points that fell within a 500 m buffer from the limits of forest cover were visually checked on screen, overlaying these points on the 2020 AGEA (Agenzia per le Erogazioni in Agricoltura) orthophoto to account for possible forest expansion during the period 2003-2019.
Statistical indicators for comparing the two change detection methods
To evaluate the accuracy of the two classifications, used in the PCC method, a confusion matrix ([45]) was used to define the Overall Accuracy (OA), the User’s Accuracy (UA) and the Producer’s Accuracy (PA), the Errors of Omission (OE) and the Commission Errors (CE), with the possible aim of refining the classification ([35]).
The Kappa index of agreement (KIA) was calculated to assess the accuracy and reliability of the classification ([9] - eqn. 2):
where p0 is the proportion of agreeing units (accuracy of satellite imagery) and pc is the proportion of units for expected chance agreement (accuracy of ground truth data).
The performance of the two change-detection methods used (NDVI differencing and post-classification comparison) for detecting forest expansion was assessed using a confusion matrix. To this end, we selected 90 new, randomly extracted ground truths from an area totaling 86,285.2 ha, which corresponds to the combined areas of forest expansion identified by both change detection methods. The ground truths were examined by overlaying the points on the AGEA 2020 orthophoto available on the Basilicata Region geo-portal. Additionally, we calculated the land use dynamic degree index (K1) proposed by Peng et al. ([50]) as a measure of the change rate of the target land use type (eqn. 3):
where Ua and Ub are the areas of the target land use type at the beginning and end of the study period, respectively, and T is the study period (in years).
The percentage rate of change (Δinl , %) of a given land use class was calculated using the following equation (eqn. 4):
where a1 and a2 are the land use areas considered at the beginning and end of the survey period, respectively, and A equals a1+a2. Finally, the annual rate of land use change (ACR) was calculated as follows ([24] - eqn. 5):
where ΔA is the change in land use (a2-a1), as in the previous eqn. 4, and N is the number of years of the study period.
Results
NDVI Differencing method
The pixels in the two tails of the ΔNDVI distribution represent significant changes in vegetation cover, while those around the mean μ indicate statistically insignificant changes. In the present study, to analyze forest expansion and the increase in terms of NDVI, we focused on the right tail of the distribution to find the value of n·σ that represents the optimal threshold. This is the cut-off point which is the best trade-off between the rate of true positives and false positives. The NDVI Differencing image distribution had μ = 0.0036 and σ = 1.016. Using the trial and test procedure described above and KIA as accuracy parameter, n value was estimated to be 1.4602 (Fig. 3), a value very similar to that obtained in a previous study carried out in the same area for different years ([39]).
The total area of forest expansion was calculated to be 54,110 ha in the 16-year period between the two images analyzed (2003-2019).
Post-classification comparison method
The method of identifying forest expansion based on PCC involved the creation of two binary maps (coded as 1 = non-forests and 2 = forests) for the two comparison years (2003 and 2019). The Maximum Likelihood Classification (MLC) algorithm was used to classify the images from the two years. MLC assumes that the statistics for each class in each band are normally distributed and calculates the probability that a given pixel belongs to a specific class. The default parameters of the classifier were used, without applying a probability threshold to classify all pixels. The accuracy results (Tab. 1) derived from the confusion matrix showed good levels of accuracy, as evidenced by the high OA value (about 90% for both dates). However, based on OA and KIA better performance was found for the classification of the Landsat 5 TM image of 2003, likely due to the perfect simultaneity of the surveys for the preparation of the Basilicata Forest Map, from which the training sites were extracted.
Tab. 1 - Accuracy of the two MLC classifications. 1: non-forest, 2: forest; Ec: Commission Error; Eo: Omission Error; PA: Producers Accuracy; UA: Users Accuracy; OA: Overall Accuracy; KIA: Kappa Index of Agreement.
MLC 2003 | - | 1 | 2 | Total | Ec (%) | Eo (%) | PA (%) | UA (%) | OA (%) | KIA |
---|---|---|---|---|---|---|---|---|---|---|
1 | 243 | 37 | 280 | 13.21 | 2.8 | 97.2 | 86.79 | - | - | |
2 | 7 | 213 | 220 | 3.18 | 14.8 | 85.2 | 96.82 | - | - | |
Total | 250 | 250 | 500 | - | - | - | - | 91.2 | 0.824 | |
MLC 2019 | - | 1 | 2 | Total | Ec (%) | Eo (%) | PA (%) | UA (%) | OA (%) | KIA |
1 | 221 | 25 | 246 | 10.16 | 11.6 | 88.4 | 89.84 | - | - | |
2 | 29 | 225 | 254 | 11.42 | 10 | 90.0 | 88.58 | - | - | |
Total | 250 | 250 | 500 | - | - | - | - | 89.2 | 0.784 |
The PCC classification for the year 2003 shows a forest area of approximately 368,000 ha, i.e., 3.5% more than the area indicated in the Basilicata Forest Map. This can be attributed to the classification criteria of the Basilicata Forest Map, which limits the area mapped as forest to those ≥ 1 ha and ≥ 20 m wide. In contrast, the pixel resolution of the satellite is such that areas of 900 m2 are considered as forests, thus explaining the larger area obtained from the satellite classification. However, the two classifications provide fairly similar estimates of the forest expansion area, which amounts to approximately 54,000 ha between 2003 and 2019.
Comparison of the two change detection methods
The comparison of the two methods applied for detecting forest expansion (NDVI Differencing and PCC) provided very similar values of all statistical indicators (Tab. 2). The areas of forest expansion between 2003 and 2019 was estimated to be approximately 54,000 ha using both methods, with a difference about 100 ha over the total area investigated (i.e., more than one million ha). Consequently, the annual increase in forest area estimated over the period 2003-2019 using the two methods applied is also substantially similar, amounting to approximately 3200 ha yr-1, with a percentage rate of increase of approximately 7% and a higher rate for NDVI Differencing of about 0.25%. However, forest expansion maps derived from NDVI Differencing and PCC showed substantially different spatial distributions of forest expansion areas. Cross-tabulation analysis (Tab. 3) revealed that the areas identified as forest expansion in both methods accounted for approximately 25%, while more than 37% were exclusively identified by only one method or the other.
Tab. 2 - Statistical indicators of land use change for the two CD methods.
Parameter | Δ CLS (ha) | K1 (%) | Δ INL (%) | ACR (ha) |
---|---|---|---|---|
NDVI Differencing | 54,110.5 | 0.8956 | 7.0739 | 3,183.0 |
PCC | 53,996.0 | 0.8623 | 6.8291 | 3,176.2 |
Tab. 3 - Absolute and percentage areas of land use change for the two CD methods.
Parameter | n. pixel | ha | % |
---|---|---|---|
Forest in PCC | 357,054 | 32,134.86 | 37.3 |
Forest in NDVI Differencing | 358,326 | 32,249.34 | 37.4 |
Forest in both CD methods | 242,902 | 21,861.18 | 25.3 |
The visual analysis, obtained by overlapping the PCC and NDVI Differencing expansion areas with the AGEA 2020 orthophoto of the Basilicata Region (Fig. 4), highlights how the PCC method detected forest expansion more efficiently.
For a parametric verification of the accuracy of the two methods, 90 control points were randomly and proportionally selected from the area given by the sum of the expansion areas detected using the two methods. Each of these ground truths was examined by visual analysis on the AGEA 2020 orthophotos to verify the presence/absence of forest recolonization (Fig. 5). The results of the confusion matrix (Tab. 4) highlight the significantly better performance of the PCC method compared with NDVI Differencing.
Tab. 4 - Confusion matrix for the two CD methods.
NDVI Differencing | Post-Classification Comparison | ||||||||
---|---|---|---|---|---|---|---|---|---|
- | true | false | Total | Ec | - | true | false | Total | Ec |
true | 14 | 16 | 30 | 0.5333 | true | 24 | 6 | 30 | 0.2000 |
false | 18 | 42 | 60 | 0.3000 | false | 7 | 53 | 60 | 0.1167 |
Total | 32 | 58 | 90 | - | Total | 31 | 59 | 90 | - |
Eo | 0.5625 | 0.2759 | - | - | Eo | 0.2258 | 0.1167 | - | - |
OA | 0.6222 | OA | 0.8556 | ||||||
KIA | 0.1639 | KIA | 0.6782 |
Discussion
The analysis of the data using two different change detection methods showed that PCC had the best performance in detecting the forest natural expansion over the Basilicata region. The two methods produced fairly similar estimates, in absolute terms, as reflected in the K1, ΔINL and ACR indices. However, substantial differences were found when examining the spatial distribution of forest expansion areas in Basilicata in the period 2003-2019. Indeed, the results obtained by the NDVI Differencing method revealed an increase in the forest area of approximately 54,110 ha (annual growth rate of 7.07 %), while PCC estimates a total forest expansion of 53.996 ha (annual growth rate of 6.83 %).
Cross-classification of the results revealed that the sum of forest expansion areas identified by PCC (and not by NDVI Differencing) and those identified by NDVI Differencing (and not by PCC) is approximately 75%, while only 25% of the pixels identified as forest expansion are shared between the two methods. Overall, PCC resulted in significantly more accuracy than the NDVI difference method. Indeed, the latter is an easy-to-apply method but does not always provide satisfactory results, especially when the temporal distance of the images is large and the sensors are different, as is the case in this study. Despite the possible image corrections that can be used, identifying the best threshold is also complex due to the non-normal distribution of NDVI difference images between the two dates ([39]). On the other hand, the PCC method enables the extraction of LULC information independently for each of the two dates under consideration, i.e., it operates by comparing pixel by pixel two images acquired at different times and classified independently of each other. In this way, PCC minimizes noise due to atmospheric differences ([42]) and sensor differences ([35]).
The use of more complex CD techniques, such as PCC, is recommended whenever viable, in particular in the case of long-time operator’s skills and in-depth knowledge of the area under investigation. Indeed, much simpler techniques, such as NDVI Differencing, are significantly more sensitive to differences in atmospheric conditions related to two different acquisition times and between sensors.
The overall accuracy obtained using the PCC method (OA = 0.85) is substantially close to the product of the OAs of the two classifications (OA = 0.91 in 2003 and OA = 0.89 in 2019), confirming previous findings reported in the literature ([12]). Data from the Italian National Forest Inventories, especially IFNC (National Forest and Carbon Inventory) 2005 and IFNC of 2015, were considered to verify the accuracy of the PCC procedure. It should be noted that the INFC data, due to the structure of the sampling method ([20]), represent the most reliable dataset of forest resources on the Italian territory. For the Basilicata region, the IFNC data identified a forest area of 395.017 ha in 2015, with an increase of approximately 41.200 ha compared to 2005. In this study, the annual change rate (ACR) results in 3745 ha and about 3176 ha using NDVI Differencing and PCC, respectively. The inventory data for the total forest area considered in the comparison refers to the year 2015. If the same rate of increase in annual forest area for the period 2005-2015 is applied, the total forest area in the Basilicata region in the year 2019 should amount to 409,997 ha. In this study, using the PCC method, we estimated a total forest area of 422,336 ha, which differs by about 3% from the inventory data. These slight differences may be likely attributable to the minimum mappable units, as the classification conducted by Landsat image analysis considers its pixel size a unit area (900 m2), thus classifying as forested areas even areas below the minimum threshold of 5000 m2 provided in the INFC.
Change-detection methods based on satellite imagery, when properly selected, carefully analyzed, and correctly applied, can produce satisfactory results with high repeatability at a low cost.
Conclusions
This study aimed to assess the dynamics of forest expansion in the Mediterranean Basin area over the past 16 years using change detection techniques derived from remote sensing satellite data. The analysis focused on the period from 2003 to 2019, utilizing images from Landsat missions, specifically Landsat 5 TM (2003) and Landsat 8 OLI (2019). The PCC method, based on the comparison of classifications elaborated for the two years under consideration, provided better performance than the NDVI Differencing technique. While the estimates of the forest expansion areas and annual rates of increase in forest area between the two methods appear to be similar, the forest expansion areas differed substantially in spatial terms. Despite the small differences detected in absolute terms, only 1/4 of the forest expansion areas were identified by both methods. Based on the comparison with appropriately selected ground truth samples, PCC method showed significantly smaller errors than NDVI Differencing (OA of 0.85 and 0.62, respectively). This is likely because NDVI Differencing is more sensitive than PCC to differences between satellite image acquisitions. In fact, despite possible corrections, images taken over such a broad time interval can generally have differences that affect the accuracy of the results, in particular when data from different sensors are used. However, when properly conducted, change detection obtained through satellite image processing shows good results when compared with traditional methods. Although they are still less reliable than traditional inventory methods based on field surveys, change detection techniques based on satellite data are significantly less expensive in terms of human and financial resources, and are suitable for any situation that requires a high level of repeatability and/or extensive coverage of the study area.
Author Contributions
Conceptualization, G.M.; methodology, G.M.; software, G.M. and A.F.; validation, G.M., A.F. and M.L.T.; formal analysis, G.M.; investigation, G.M. and A.F.; resources, G.M. and A.F.; data curation, G.M.; writing - original draft preparation, G.M.; writing - review and editing, G.M, A.F. and M.L.T.; visualization, G.M. and A.F.; supervision, G.M.; project administration and funding acquisition, M.L.T.
All authors have read and agreed to the published version of the manuscript.
Funding
This work is part of the project “SPRINt” (2018-AMB-00120), funded by “Fondazione Con il Sud”. This research received no external funding.
Conflicts of Interest
The authors declare no conflict of interest.
References
CrossRef | Gscholar
CrossRef | Gscholar
Gscholar
Gscholar
Gscholar
CrossRef | Gscholar
Gscholar
Gscholar
Gscholar
Gscholar
Authors’ Info
Authors’ Affiliation
Antonio Falciano 0000-0003-2354-1101
Maria Lucia Trivigno
Centro di Geomorfologia Integrata per l’Area del Mediterraneo, v. Domenico Di Giura 54, 85100 Potenza (Italy)
Corresponding author
Paper Info
Citation
Mancino G, Falciano A, Trivigno ML (2025). Change Detection methods for forest expansion assessment in the last twenty years in the Mediterranean Basin. iForest 18: 69-78. - doi: 10.3832/ifor4634-018
Academic Editor
Carlotta Ferrara
Paper history
Received: May 03, 2024
Accepted: Feb 03, 2025
First online: Apr 16, 2025
Publication Date: Apr 30, 2025
Publication Time: 2.40 months
Copyright Information
© SISEF - The Italian Society of Silviculture and Forest Ecology 2025
Open Access
This article is distributed under the terms of the Creative Commons Attribution-Non Commercial 4.0 International (https://creativecommons.org/licenses/by-nc/4.0/), which permits unrestricted use, distribution, and reproduction in any medium, provided you give appropriate credit to the original author(s) and the source, provide a link to the Creative Commons license, and indicate if changes were made.
Web Metrics
Breakdown by View Type
Article Usage
Total Article Views: 1081
(from publication date up to now)
Breakdown by View Type
HTML Page Views: 321
Abstract Page Views: 338
PDF Downloads: 372
Citation/Reference Downloads: 1
XML Downloads: 49
Web Metrics
Days since publication: 51
Overall contacts: 1081
Avg. contacts per week: 148.37
Article Citations
Article citations are based on data periodically collected from the Clarivate Web of Science web site
(last update: Mar 2025)
(No citations were found up to date. Please come back later)
Publication Metrics
by Dimensions ©
Articles citing this article
List of the papers citing this article based on CrossRef Cited-by.
Related Contents
iForest Similar Articles
Research Articles
Landsat TM imagery and NDVI differencing to detect vegetation change: assessing natural forest expansion in Basilicata, southern Italy
vol. 7, pp. 75-84 (online: 18 December 2013)
Research Articles
Land use inventory as framework for environmental accounting: an application in Italy
vol. 5, pp. 204-209 (online: 12 August 2012)
Review Papers
Remote sensing-supported vegetation parameters for regional climate models: a brief review
vol. 3, pp. 98-101 (online: 15 July 2010)
Technical Reports
The treatment of land use, land use change and forestry in the post-2012 climate agreement: a perspective from non-Annex I Parties
vol. 3, pp. 56-58 (online: 17 May 2010)
Research Articles
Afforestation monitoring through automatic analysis of 36-years Landsat Best Available Composites
vol. 15, pp. 220-228 (online: 12 July 2022)
Research Articles
An assessment of climate change impacts on the tropical forests of Central America using the Holdridge Life Zone (HLZ) land classification system
vol. 6, pp. 183-189 (online: 08 May 2013)
Research Articles
Role of forest cover, land use change and climate change on water resources in Marmara basin of Turkey
vol. 8, pp. 480-486 (online: 31 October 2014)
Research Articles
Assessing the performance of MODIS and VIIRS active fire products in the monitoring of wildfires: a case study in Turkey
vol. 15, pp. 85-94 (online: 19 March 2022)
Research Articles
Mapping the vegetation and spatial dynamics of Sinharaja tropical rain forest incorporating NASA’s GEDI spaceborne LiDAR data and multispectral satellite images
vol. 18, pp. 45-53 (online: 01 April 2025)
Research Articles
Future land use and food security scenarios for the Guyuan district of remote western China
vol. 7, pp. 372-384 (online: 19 May 2014)
iForest Database Search
Google Scholar Search
Citing Articles
Search By Author
Search By Keywords