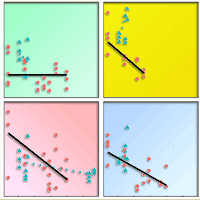
Climate-wise models of biomass productivity for hybrid poplar clones in Europe
iForest - Biogeosciences and Forestry, Volume 16, Issue 3, Pages 188-194 (2023)
doi: https://doi.org/10.3832/ifor4211-016
Published: Jun 30, 2023 - Copyright © 2023 SISEF
Research Articles
Abstract
Renewable bioenergy has the potential to contribute sustainably to the energy sector. Forestry is the main source of biomass for energy in Europe, and poplar (genus Populus) is widely used for short rotation coppice (SRC). Many studies have assessed poplar clones’ productivity but there is a lack of regional studies and links with the climate. We investigated the biomass productivity of twenty hybrid poplar clones for SRC. Clones were planted in sixteen locations across nine countries in Europe, although not all clones were replicated in all locations. In each location, clones were planted in three replicated plots. All plots were harvested after four years, and the aboveground dry biomass estimated. We fitted clone-specific linear mixed models of total aboveground dry biomass production at plot level as function of climatic variables. For many clones (eight) only annual heat moisture deficit negatively affected productivity, in few cases (3) together with a quadratic term for a smoother relationship. In some other clones (five) only the mean summer precipitation positively and linearly affected productivity. On average, the variance explained by the fixed effects in those models was 56%. For the remaining clones (seven), no climate variables resulted significant. Our study explicitly investigated the quantitative link between water availability and poplar SRC productivity, one of the most important known factors but not often studied with a modelling approach. Further, we show the most productive clones in dried conditions. We also highlight the need to larger scale regional experiments to produce models that can be used in climate change scenarios.
Keywords
Hybrid Poplar, Short Rotation Coppice, Aridity Index, Water Availability, Above Ground Biomass
Introduction
Bioenergy can play a key role in achieving the EU’s renewable energy target, provided it is produced, processed and used in a sustainable and efficient way ([7]). Forestry is the main source of biomass for energy in Europe, and short rotation coppice (SRC) using fast growing species is an important potential component ([3]). In Europe, the Salicaceae family, Populus spp. and Salix spp., presents the greatest developments on an industrial level for SRC ([20]). However, the global consensus is that growing woody SRCs for energy production is not yet economically feasible ([22]). So far, data on productivity of SRCs are rather scarce and limited to small experimental-scale plantations ([23]); therefore better information are needed to improve the decision making process on SRCs.
Poplars are among the fastest growing trees in temperate latitudes and their high productivity comes with high water demands, although a wide diversity in water requirements has been reported (see [16] and references therein). To improve and optimize the biomass production across Europe, it is important to know which clone can perform better at a given site according to its characteristics. To this purpose, a better understanding of the relationship between productivity and local climate is required.
Various studies have analysed the productivity of poplar SRCs by comparing different clones ([6], [14], [16], [23], [8], [18], [20]). However, most of the mentioned studies addressed only one country, generally with a limited number of clones established in few sites. Further, even when a large number of clones/sites were used ([17], [12]), productivity was compared to identify the best performing clones at the experimental site, but without investigating their relationships with the site characteristics. To the best of our knowledge, only few studies focused on modeling poplar SRC biomass productivity as a function of climate variables, with contrasting results ([4], [9], [19]). The latter was the only study we found involving data across different European countries. Werner et al. ([25]) employed a process-based model for simulating poplar SRC productivity, but it was validated only against one clone. Navarro et al. ([16]) identified more resistant clones to arid conditions by investigating their stomatal behavior from only one site.
The objective of this study was to overcome the gap in cross-European studies on climate-productivity relationships in poplar SRCs for bioenergy production. The final aim is to provide stakeholders with better information for decision making at a larger scale such as landscape planning ([22]). We used a dataset including several sites from various countries across Europe where the same poplar clones were planted in SRCs. We calibrated linear mixed models to identify the relationship between climate variables and clones’ productivity.
Material and methods
Study sites and clones
We retrieved data on twenty hybrid poplar clones, belonging to eight different genetic groups (or “families”), planted in 16 locations across 9 European countries (Fig. 1). On average, there were 15 clones planted in each site (from 8 to 20). Tab. 1 shows more details about the location of each site, while the genetic group for each clone is reported in Tab. 2.
Fig. 1 - Map of the study sites, with indication of their mean productivity (m3 ha-1 year-1) considering all clones and plots (both irrigated and non-irrigated sites).
Tab. 1 - List of the locations where poplar clones were employed. Coordinates are in WGS84 decimal degrees. Sites with an asterisk were irrigated and excluded from modelling. (AHM): Annual Heat Moisture.
Location | Country | Lat N | Long E | AHM (°C m-1) |
---|---|---|---|---|
1 | Belgium | 50.763 | 3.879 | 34.3 |
2 | Bulgaria | 42.217 | 24.320 | 56.5 |
3 | 43.511 | 23.367 | 49.3 | |
4 | Croatia | 45.576 | 18.686 | 42.2 |
5 | Czech Republic | 49.992 | 14.578 | 49.4 |
6 | Germany | 47.863 | 12.064 | 24 |
7 | 47.973 | 10.823 | 23.9 | |
8 | Hungary | 47.276 | 16.973 | 46.1 |
9 | 47.314 | 19.485 | 53.4 | |
10 | Italy | 41.906 | 12.355 | 42.1 |
11* | 45.142 | 8.516 | 34.6 | |
12* | 44.709 | 7.678 | 35.9 | |
13* | 44.595 | 7.617 | 30.8 | |
14 | Romania | 44.192 | 27.308 | 60 |
15* | Spain | 40.666 | -3.169 | 76.8 |
16* | 40.513 | -3.310 | 73.7 |
Tab. 2 - Details of the study clones. Codes for genetic groups: (d) Populus deltoides; (k) P. koreana; (m) P. maximowiczii; (n) P. nigra; (t) P. trichocarpa. Sites with an asterisk were irrigated and excluded from modelling. (AGBp): above ground biomass productivity (mean ± standard deviation).
Clone name |
Genetic group |
Locations | AGBp (Mg year-1 ha-1) |
|||||||||||||||
---|---|---|---|---|---|---|---|---|---|---|---|---|---|---|---|---|---|---|
1 | 2 | 3 | 4 | 5 | 6 | 7 | 8 | 9 | 10 | 11* | 12* | 13* | 14 | 15* | 16* | |||
AF18 | (d×n) | x | - | - | - | - | x | x | x | x | x | x | x | x | x | - | - | 9.536 ± 5.410 |
AF34 | (d×n) | x | x | x | - | - | x | x | - | - | x | x | x | x | x | x | x | 8.649 ± 5.468 |
AF8 | (t×d) | x | x | x | - | x | x | x | x | x | x | x | x | x | x | x | x | 7.090 ± 4.116 |
Antonije | (d×n)×d | x | - | - | x | - | x | x | x | - | x | x | - | x | x | x | x | 4.930 ± 3.298 |
Baldo | (d×d) | x | x | x | x | - | x | x | x | x | x | x | x | x | x | x | x | 7.133 ± 5.290 |
Brenta | (d×n) | x | x | x | - | x | x | x | x | x | x | x | x | x | x | x | x | 6.750 ± 4.379 |
Dellinois | (d×d) | x | - | - | - | - | x | x | - | - | x | x | - | x | - | x | x | 8.385 ± 3.300 |
Delrive | (d×d) | x | - | - | x | - | x | x | - | - | x | x | - | x | - | x | x | 7.617 ± 3.201 |
Grimminge | d×(t×d) | x | x | x | - | - | x | x | x | x | x | x | x | x | x | x | x | 5.834 ± 3.859 |
Hybride275 | (n×m) | x | x | x | x | x | x | x | x | - | - | x | x | x | x | x | x | 3.470 ± 2.805 |
Koreana | (t×k) | x | x | x | x | x | x | x | x | - | - | x | x | x | x | x | x | 5.302 ± 5.341 |
Matrix21 | (t×m) | x | x | x | x | x | x | x | x | - | - | x | x | x | - | x | x | 4.461 ± 4.034 |
Max 1-4 | (n×m) | x | x | x | x | x | x | x | x | - | x | x | x | x | x | x | x | 6.323 ± 3.411 |
Muur | (d×n) | x | - | - | - | x | x | x | x | x | x | x | x | x | x | - | - | 4.740 ± 3.359 |
Orion | (d×n) | x | - | - | - | - | x | x | x | - | x | x | x | x | x | - | - | 10.293 ± 5.348 |
Oudenberg | (d×n) | x | - | - | - | - | x | x | x | x | x | x | x | x | x | - | - | 6.783 ± 3.999 |
SV885 | (d×n) | x | - | - | x | - | x | x | x | - | x | x | - | x | x | - | - | 5.580 ± 3.024 |
SW 822 | (d×n) | x | - | - | - | - | x | x | x | x | - | x | - | x | x | - | - | 5.108 ± 1.927 |
Skado | (t×m) | x | x | x | - | x | x | - | x | x | - | x | x | x | x | x | x | 6.061 ± 4.846 |
Vesten | (d×n) | x | x | x | - | - | x | x | x | - | x | x | x | x | x | - | - | 5.709 ± 3.607 |
Experimental design
All plots were established in winter 2013-2014. There was a common minimum design across all locations. At least four rows with ten plants were planted in a plot (spacing 3 × 1 m) over a minimum area of 120 m2, with a buffer of one row each side to avoid edge effects. When a clone was planted in a location, there were three replicates. All plots were ploughed approximately to 30-40 cm, and weed were controlled manually or chemically until the end of July 2014. No fertilization was carried out. Plots were chosen as homogenous as possible in flat locations on arable land.
Irrigation was carried out in five sites in Mediterranean conditions (Spain and Italy). Irrigation strongly and positively affected the productivity of the plots, thus introducing a bias in the climate-growth relationship. Indeed, preliminary results showed that when a dummy variable reflecting watering is included in the productivity models, no significant climate-growth relationship is found. Furthermore, no clear details on the irrigation practices carried out were available at these sites, therefore they were excluded them from modelling. After removing those sites, each clone was employed in at least other seven locations.
After four years (winter 2017-2018), the 16 plants in the centre of each plot were harvested and fresh weighted. For most locations wood moisture was directly evaluated by comparing fresh and dry weight of samples collected from the harvested trees. Wood moisture ranged from to 47% to 65%, with an overall mean of 56%. For other sites (Bajiti, Hungary; Frassineto and San Isidoro, Italy; Guadalajara and La Canaleja, Spain), no data on wood moisture were available, and in these cases it was considered equal to the average level (58%), except for the Suniglia site, where the same values of nearby Casale Monferrato site were used. Consequently, for each plot, total aboveground dry biomass productivity (AGBp, Mg ha-1 year-1) was calculated as the variable of interest. While we retrieved and kept separately the values for the three plot of each clone in most of the locations, for one (Tvrdavica, Croatia) we were able to retrieve only the average of the three plots for each clone (data from [24]).
Data analysis
Climatic data for all the study sites were retrieved from ClimateDT (⇒ https://ibbr.cnr.it/climate-dt/), a web portal where scale-free climatic data are freely provided at global level using CRU-TS data ([11]) for the historical period (1901-current year - [15]). Several variables of interest can be selected on the portal, based on monthly and annual values of temperature and precipitation, along with a wide array of derivative indices. Climatic values for each site were retrieved for the years 2014-2017 and averaged. We carried out a preliminary analysis of the relationship between the climatic variables and AGBp. According to Marchi et al. ([15]), we selected the following variables as potential predictors of productivity: Mean Summer Precipitation (MSP, mm), calculated from May to September; a continentality index (CONT), defined as the standard deviation of the average monthly temperatures; and the Annual Heat Moisture (AHM, °C mm-1), defined as (eqn. 1):
where MAT is the Mean Annual Temperature (°C) and MAP the Mean Annual Precipitation (mm). High MSP corresponds to high water availability during the vegetative season, high CONT corresponds to high degree of continentality in terms of annual temperature variation, and high values of AHM are due to high temperature and/or low precipitation, resulting in low water availability for the plants.
Soil data were retrieved from SoilGrids (⇒ https://soilgrids.org/), a system for digital soil mapping based on a global compilation of quality-assessed and standardised soil profile data (WoSIS - [2]). After preliminary analyses, the soil parameters retrieved were either not significant for most of the clones, or had negligible influence, or were not biologically sound. Thus, all soil parameters were excluded as predictors from modelling. Unfortunately, no soil data collected directly in the field were available at the study sites.
We fitted clone-specific linear mixed models for AGB productivity as follows (eqn. 2):
where quadratic terms were used to account for possible non-linearity of the response; ul are random effects for each location to account for the spatial correlation; and εlm the error for each measurement. We then selected the best model for each clone according to lower Akaike Information Criteria (AIC), analysis of the residuals, and biological interpretation of the predictors’ effects. All analyses were carried out using the package “lme4” ([1]) in the R statistical environment ([21]). As previously mentioned, irrigated sites were excluded from the analysis. Once the models were selected, we carried out a sensitivity analysis of all predictors. We simulated AGBp as a function of only one predictor, which we let to vary from the minimum to the maximum value observed in the data used for calibrating the models, while the values of the other variables were kept equal to the observed mean.
Results
Significant climate-growth relationships were found in thirteen out of twenty AGBp models (Tab. 3). For eight models, AGBp was significantly and negatively correlated with AHM using a linear term, and in three of those cases, the negative trend tended to stabilize to a minimum towards high values of AHM, due to a significant positive quadratic term. For five other clones, AGBp was significantly and negatively correlated with MSP using a linear term. For the remaining seven clones, no fixed effect was found significant. CONT was significant in only three models, but it was removed from the analysis since its inclusion pushed the coefficients for AHM and MSP to extreme illogical values, indicating an overfitting with no biological meaning.
Tab. 3 - Results of clone-specific linear mixed models. (Sig.): significance of the p-values according to the standard notation. (MAE): mean absolute error; (RMSE): root mean square error. R2 is the variance explained by the model, either considering only the fixed effects (R2 marginal) or both fixed and random effects (R2 conditional).
Location | No. of samples |
Coefficient | Estimate | p-value | Sig. | MAE | RMSE | R2 marginal |
R2 conditional |
---|---|---|---|---|---|---|---|---|---|
AF18 | 21 | (Intercept) | 7.10847 | 0.00034 | *** | 2.094 | 2.772 | 0.00 | 0.26 |
AF34 | 20 | (Intercept) | 0.10698 | 0.94571 | - | 2.194 | 2.778 | 0.41 | 0.41 |
MSP | 0.01970 | 0.00176 | ** | 2.194 | 2.778 | 0.41 | 0.41 | ||
AF8 | 30 | (Intercept) | 0.81599 | 0.66112 | - | 1.354 | 1.743 | 0.34 | 0.63 |
MSP | 0.01859 | 0.02315 | * | 1.354 | 1.743 | 0.34 | 0.63 | ||
Antonije | 19 | (Intercept) | 3.91550 | 0.01111 | * | 1.006 | 1.243 | 0.00 | 0.75 |
Baldo | 28 | (Intercept) | -3.05146 | 0.20270 | - | 1.994 | 2.597 | 0.51 | 0.66 |
MSP | 0.03353 | 0.00546 | ** | 1.994 | 2.597 | 0.51 | 0.66 | ||
Brenta | 26 | (Intercept) | 9.56719 | 0.00670 | ** | 1.225 | 1.532 | 0.26 | 0.64 |
AHM | -0.12388 | 0.06367 | . | 1.225 | 1.532 | 0.26 | 0.64 | ||
Dellinois | 12 | (Intercept) | 19.59646 | 0.00026 | *** | 1.903 | 2.697 | 0.51 | 0.51 |
AHM | -0.37528 | 0.00716 | ** | 1.903 | 2.697 | 0.51 | 0.51 | ||
Delrive | 13 | (Intercept) | 7.95905 | 0.01401 | * | 0.819 | 1.057 | 0.00 | 0.91 |
25 | (Intercept) | -3.45854 | 0.01018 | * | 1.689 | 2.276 | 0.73 | 0.73 | |
25 | MSP | 0.03539 | 0.00000 | *** | 1.689 | 2.276 | 0.73 | 0.73 | |
Grimminge | 23 | (Intercept) | 25.82406 | 0.00150 | ** | 1.184 | 1.527 | 0.71 | 0.74 |
23 | AHM | -0.95448 | 0.00827 | ** | 1.184 | 1.527 | 0.71 | 0.74 | |
Hybride275 | 23 | I(AHM^2) | 0.00947 | 0.02064 | * | 1.184 | 1.527 | 0.71 | 0.74 |
24 | (Intercept) | 42.79374 | 0.00294 | ** | 1.176 | 1.712 | 0.80 | 0.89 | |
24 | AHM | -1.50734 | 0.01828 | * | 1.176 | 1.712 | 0.80 | 0.89 | |
Koreana | 24 | I(AHM^2) | 0.01369 | 0.05519 | . | 1.176 | 1.712 | 0.80 | 0.89 |
21 | (Intercept) | 36.46674 | 0.00358 | ** | 1.123 | 1.380 | 0.84 | 0.89 | |
21 | AHM | -1.31474 | 0.02028 | * | 1.123 | 1.380 | 0.84 | 0.89 | |
Matrix21 | 21 | I(AHM^2) | 0.01251 | 0.05660 | . | 1.123 | 1.380 | 0.84 | 0.89 |
25 | (Intercept) | 5.12385 | 0.00035 | *** | 1.100 | 1.497 | 0.00 | 0.63 | |
24 | (Intercept) | 3.81112 | 0.00819 | ** | 0.874 | 0.975 | 0.00 | 0.86 | |
Max 1-4 | 15 | (Intercept) | 18.19725 | 0.00005 | *** | 1.791 | 2.331 | 0.57 | 0.57 |
Muur | 15 | AHM | -0.30283 | 0.00092 | *** | 1.791 | 2.331 | 0.57 | 0.57 |
Orion | 21 | (Intercept) | 5.24868 | 0.00119 | ** | 2.116 | 2.617 | 0.00 | 0.26 |
Oudenberg | 19 | (Intercept) | -0.40648 | 0.84369 | - | 1.053 | 1.654 | 0.49 | 0.68 |
SV885 | 19 | MSP | 0.02029 | 0.03270 | * | 1.053 | 1.654 | 0.49 | 0.68 |
18 | (Intercept) | 4.89019 | 0.00035 | *** | 1.238 | 1.632 | 0.00 | 0.21 | |
SW 822 | 21 | (Intercept) | 19.27073 | 0.00566 | ** | 3.598 | 4.742 | 0.30 | 0.30 |
Skado | 21 | AHM | -0.27800 | 0.02690 | * | 3.598 | 4.742 | 0.30 | 0.30 |
23 | (Intercept) | 13.18552 | 0.00210 | ** | 1.328 | 1.701 | 0.48 | 0.71 | |
Vesten | 23 | AHM | -0.19773 | 0.01444 | * | 1.328 | 1.701 | 0.48 | 0.71 |
21 | (Intercept) | 7.10847 | 0.00034 | *** | 2.094 | 2.772 | 0.00 | 0.26 |
The clone-specific models showed a good fitting to the observed values of productivity for most clones (Fig. 2). These was confirmed by the conditional R2 values obtained, which ranged from 0.21 to 0.91 and averaged to 0.64 (Tab. 3). However, it is worth noting that only in thirteen cases the good fit of the clone-specific model was maily due to the fixed effects (AHM or MSP), while in the other cases fitting was largely explained by the random effects.
Fig. 2 - Comparison of observed AGBp (above ground biomass productivity, in Mg year-1 ha-1) vs. its predicted values for all clone-specifics models.
For the models showing significant fixed effects, the marginal R2 values (considering only the fixed terms) still showed satisfactory results, ranging from 0.26 to 0.84, on average 0.56. Also, it should be stressed that the conditional values for the intercept only models were on average 0.68, only due to random effects. Model residuals did not reveal any bias against the predictions, although in very few cases there was a slight tendency towards a larger variance for higher predicted values (Fig. 3).
Fig. 3 - Residuals of the above ground biomass productivity (Mg year-1 ha-1) vs. predicted values for all clone-specifics models.
The sensitivity analysis shows how the poplar clones reacted to either AHM (Fig. 4) or MSP (Fig. 5). Very low productivity values were found in the AHM range 40-50 °C mm-1, where the minimum productivity was reached for the models with a quadratic term. For most clones planted at irrigated sites, the productivity was generally higher and not influenced by AHM, except for seven clones (Delrive, Matrix21, Hybride275, SV885, SW822, Skado and Vesten). The above evidence indicates that over a AHM threshold value of about 40-50 °C mm-1, irrigation seems to be essential to ensure a good productivity of poplar SRC for most clones. Below that threshold, the best performing clones were Dellinois, Koreana, Matrix21, Orion, and Skado. On the contrary, the best clones performing with irrigation were AF34, AF8, Baldo and Brenta. In the model using MSP as fixed effect, AGBp increased linearly until reaching around 10 Mg ha-1 year-1 for values of mean summer precipitation higher than 400 mm.
Fig. 4 - Lines: above ground biomass productivity (AGBp, Mg year-1 ha-1) predicted as function of Annual Heat Moisture index (AHM) for all clones. Points: observed values of AGBp across different sites. Irrigated locations (green points, not used in modeling) are also shown for comparison.
Fig. 5 - Lines: above ground biomass productivity (AGBp, Mg year-1 ha-1) predicted as function of Mean Summer Precipitation (MSP) for all clones. Points: observed values of AGBp across different. Irrigated locations (green points, not used in modelling) are also shown for comparison.
Discussion
Our study highlights the need of large regional studies for modeling poplar SRC productivity, which can be used to fit climate-wise models. Currently, there is a lack of such models in the literature on this topic. Understanding the clone-specific relationships between climate and poplar SRC productivity can help in improving both the profitability and sustainability of renewable biomass production, for example by reducing the needs for irrigation or fertilization ([20], [10], [26]). This is especially important given both the current concerns in water uptake at European level ([23]) and the climate change impact ([13]). The general level of productivity in this study (from 1 to 26 Mg ha-1 year-1) was similar to that of various European countries and clones reported in Oliveira et al. ([20]), which also included some of the clones tested in this work. The selection criteria used for commercial poplar genotypes should take into account drought resistance together with biomass productivity ([16]). Our research expanded the findings of Bergante et al. ([4]) by including sites from several countries in Europe, and those of Fischer et al. ([9]) and Navarro et al. ([16]) by providing links between clone productivity and water availability estimated by climatic datasets.
Most of the clones (thirteen out twenty) were significantly affected by either AHM or MSP, similarly to the findings reported by Bergante et al. ([4]). This is in line with the species’ ecology and its high requirements of water availability ([5]). Njakou Djomo et al. ([19]) did not find a significant relationship between clone productivity and water availability, likely due to the lack of inclusion of species-specific and/or site management effects. A quadratic term was used also in Marchi et al. ([15]), but the inclusion of MSP in the model resulted in different (positive) relations for AHM. Also, continentality did not enter any model in this study, in contrast to Marchi et al. ([15]) where the same predictor did significantly affect poplar productivity.
For the thirteen clones were either AHM or MSP was significant in this study, the R2 was 0.56 on average, indicating an adequate fit. For the rest of clones, only the random effect due to the plot location influenced the model, so there was no possible biological interpretation. The high values of R2 even in many of those cases (on average 0.68) suggest the presence of environmental factors which drastically contrast among different sites. Indeed, these environmental factors could explain much of the variation, though were not included in the models.
Some clones, namely Dellinois, Koreana, Matrix21, Orion, and Skado, showed the best performances below a AHM threshold of 40-50 °C mm-1, i.e., in areas with higher water availability. Thus, they are potential candidates for poplar SRC to be established in sites where water availability is not a constraint. On the contrary, except for the clone Orion, they had amongst the lowest productivity values at high level of AHM, i.e., in the driest conditions, and a weak positive response to irrigation. Over AHM 40-50 °C mm-1, all clones performed very poorly without irrigation. Marchi et al. ([15]) in a similar study on hybrid poplar in Italy did not investigated sites with conditions drier than AHM 40 °C mm-1, so comparisons are not possible. Regarding MSP, we found a linear increase in productivity in both studies, although slightly exponential in Marchi et al. ([15]) for MSP values until 400-500 mm, the highest in both datasets. As expected, irrigation generally improved productivity. Oliveira et al. ([20]) reported that the highest levels of productivity are shown in irrigated Mediterranean sites, as in this study. In our models, when irrigation was included, we did not find any significant climate-relationship. Similarly, Marchi et al. ([15]) found out that irrigation explained most of the variance, followed by AHM. In this study, ee decided to discard the irrigated sites from the analysis to better study the climate-productivity relationships and find the clones less susceptible to drought. However, some clones (AF34, AF8, Baldo and Brenta) seemed to have the best performance when irrigation was carried out, regardless of the site aridity.
Soil properties were excluded from modelling due to their low or inconclusive contribution to explain the model variance, while they are considered crucial for productivity ([5]). All plots were established in agricultural land where the managers expected a good productivity for poplar. Either there was a low variation in the overall soil potential across sites (all equally favourable for good productivity), or we could not find reliable data at such level of details for all sites. Marchi et al. ([15]) also highlighted the lack of high-quality, high-resolution soil data layers to be used for range-wide modelling purposes. Soil texture and organic matter content may interact with plant growth due to their influence on soil structure and hydrological characteristics ([4]), and may be included in further modelling experiments.
It is worth to highlight that few replicates for each clone were analyzed in this study, especially when data from irrigated sites were discarded (from seven to eleven locations). Moreover, the plot size was relatively small (minimum 120 m2), and it has been found that using small-scale plantations tend to overestimate biomass production values ([23]). Further, some of the models included a quadratic term for AHM that was crucial in smoothing the climate-growth relationship, although it can have an unplausible positive effect at AHM values higher than those included in our dataset. For the above reasons, we recommend caution in extrapolating the results of our models outside the specific range of the study. Finally, our study stresses the need for larger network of trials, especially in the light of the uncertainty of climate change, as also reported by Marchi et al. ([15]).
Conclusions
We presented climate-wise model for hybrid poplar productivity using a dataset collected at European scale. Our results show water availability, expressed by climatic variables linked to precipitation and aridity, increased poplar productivity in short rotation coppice with a quantitative modelling approach. However, some limitations of the study, such as soil variables not included in the models, and the few replicates in the dataset, may not allow extrapolating the results of the models presented here outside the specific range of the study. Nonetheless, we demonstrated that that using a larger dataset in further study, a more comprehensive model for poplar productivity in short rotation coppice can be developed as function of climatic variables.
Acknowledgements
The data were collected within the project “Testing of poplar clones from EU member states for the use in short rotation coppice”. We wish to thank for this study: Linda Meiresonne, Marijke Steenackers (INBO, Belgium); Albena Bobeva (Executive Forest Agency, Bulgaria); Davorin Kajba (University of Zagreb, Croatia); Jan Weger (Silva Tarouca, Czech Republic); Andrea Barban, Francesco Fabbrini (Alasia Franco Vivai, Italy); Enrico Alasia, Fabiano Bertaina, Valerio Crescenzo (Biopoplar, Italy); Giuseppe Pignatti, Stefano Verani (CREA - Research Centre for Forestry and Wood, Italy); Randolf Schirmer (AWG Teisendorf, Germany); Boglárka Nemethne, Sándor Bordács (National Food Chain Safety Office, Hungary); Attila Benke (Savar, Hungary); Catherine Bastien (INRAE, France); Hortensia Sixto (CIFOR-INIA, Spain); and Georgeta Mihai (Forest Research & Management Institute, Romania).
This research was funded by the Academy of Finland under the UNITE (Forest-Human-Machine Interplay) flagship ecosystem (Grant no. 337655) and by the European Union Horizon 2020 Programme under the B4EST (“Adaptive breeding for productive, sustainable and resilient forests under climate change”) project (Grant no. 773383).
References
CrossRef | Gscholar
CrossRef | Gscholar
CrossRef | Gscholar
Authors’ Info
Authors’ Affiliation
Daesung Lee 0000-0003-1586-9385
Jari Hynynen 0000-0002-9132-8612
LUKE, Latokartanonkaari 9, F-00790, Helsinki (Finland)
Gianni Facciotto 0000-0001-9550-0806
Giuseppe Nervo 0000-0002-8282-7110
CREA, str. Frassineto 35, I-15033, Casale Monferrato, AL (Italy)
Corresponding author
Paper Info
Citation
Bianchi S, Lee D, Bergante S, Facciotto G, Hynynen J, Nervo G (2023). Climate-wise models of biomass productivity for hybrid poplar clones in Europe. iForest 16: 188-194. - doi: 10.3832/ifor4211-016
Academic Editor
Andrea Cutini
Paper history
Received: Aug 25, 2022
Accepted: Mar 30, 2023
First online: Jun 30, 2023
Publication Date: Jun 30, 2023
Publication Time: 3.07 months
Copyright Information
© SISEF - The Italian Society of Silviculture and Forest Ecology 2023
Open Access
This article is distributed under the terms of the Creative Commons Attribution-Non Commercial 4.0 International (https://creativecommons.org/licenses/by-nc/4.0/), which permits unrestricted use, distribution, and reproduction in any medium, provided you give appropriate credit to the original author(s) and the source, provide a link to the Creative Commons license, and indicate if changes were made.
Web Metrics
Breakdown by View Type
Article Usage
Total Article Views: 9626
(from publication date up to now)
Breakdown by View Type
HTML Page Views: 8239
Abstract Page Views: 717
PDF Downloads: 587
Citation/Reference Downloads: 0
XML Downloads: 83
Web Metrics
Days since publication: 308
Overall contacts: 9626
Avg. contacts per week: 218.77
Article Citations
Article citations are based on data periodically collected from the Clarivate Web of Science web site
(last update: Feb 2023)
(No citations were found up to date. Please come back later)
Publication Metrics
by Dimensions ©
Articles citing this article
List of the papers citing this article based on CrossRef Cited-by.
Related Contents
iForest Similar Articles
Research Articles
Effective woody biomass estimation in poplar short-rotation coppices - Populus nigra × P. maximowiczii
vol. 16, pp. 202-209 (online: 25 July 2023)
Research Articles
First vs. second rotation of a poplar short rotation coppice: leaf area development, light interception and radiation use efficiency
vol. 8, pp. 565-573 (online: 27 April 2015)
Research Articles
Linking biomass production in short rotation coppice with soil protection and nature conservation
vol. 7, pp. 353-362 (online: 19 May 2014)
Research Articles
Efficient measurements of basal area in short rotation forests based on terrestrial laser scanning under special consideration of shadowing
vol. 7, pp. 227-232 (online: 10 March 2014)
Research Articles
Comparison of soil CO2 emissions between short-rotation coppice poplar stands and arable lands
vol. 11, pp. 199-205 (online: 01 March 2018)
Research Articles
Field performance of poplar for bioenergy in southern Europe after two coppicing rotations: effects of clone and planting density
vol. 5, pp. 224-229 (online: 02 October 2012)
Research Articles
A comparative study of four approaches to assess phenology of Populus in a short-rotation coppice culture
vol. 9, pp. 682-689 (online: 12 May 2016)
Research Articles
Biomass production of Populus nigra L. clones grown in short rotation coppice systems in three different environments over four rotations
vol. 7, pp. 233-239 (online: 10 March 2014)
Research Articles
Variations in the performance of hybrid poplars subjected to the inoculation of a microbial consortium and water restriction
vol. 16, pp. 352-360 (online: 13 December 2023)
Review Papers
Quantifying and modeling water availability in temperate forests: a review of drought and aridity indices
vol. 12, pp. 1-16 (online: 10 January 2019)
iForest Database Search
Search By Author
Search By Keyword
Google Scholar Search
Citing Articles
Search By Author
Search By Keywords
PubMed Search
Search By Author
Search By Keyword