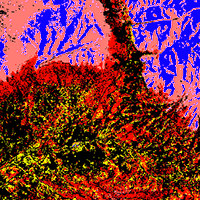
Assessing the influence of different Synthetic Aperture Radar parameters and Digital Elevation Model layers combined with optical data on the identification of argan forest in Essaouira region, Morocco
iForest - Biogeosciences and Forestry, Volume 17, Issue 2, Pages 100-108 (2024)
doi: https://doi.org/10.3832/ifor4183-016
Published: Apr 24, 2024 - Copyright © 2024 SISEF
Research Articles
Abstract
Forest resource conservation necessitates a deeper understanding of forest ecosystem processes and how future management decisions and climate change may affect these processes. Argania spinosa (L.) Skeels is one of the most popular species in Morocco. Despite its ability to survive under harsh drought, it is endangered due to soil land removal and a lack of natural regeneration. Remote sensing offers a powerful resource for mapping, assessing, and monitoring the forest tree species at high spatio-temporal resolution. Multi-spectral Sentinel-2 and Synthetic Aperture Radar (SAR) time series combined with Digital Elevation Model (DEM) over the Argan forest in Essaouira province, Morocco, were subjected to pixel-based machine learning classification and analysis. We investigated the influence of different SAR data parameters and DEM layers on the performance of machine learning algorithms. In addition, we evaluated the synergistic effects of integrating remote sensing data, including optical, SAR, and DEM data, for identifying argan trees in the Smimou area. We collected data from Sentinel-2, Sentinel-1, SRTM DEM, and ground truth sources to achieve our goal. Testing different SAR parameters and integrating DEM layers of different resolutions with other remote sensing data showed that the Lee Sigma filter with a size of 11×11 and a DEM layer of 30 m resolution gave the best results using the Support Vector Machine algorithm. Significant improvements in overall accuracy (OA) and kappa index (K) were observed in the following phase. After applying a smoothing technique, the combined use of two Sentinel constellation products improved map accuracy and quality. For the best scenario (VV+NDVI), the OA was 88.32% (K = 0.85), while for scenarios NDVI+DEM and VH+NDVI+DEM, the OAs were 93.25% (K = 0.91) and 93.01% (K = 0.91), respectively. Integrating a DEM layer with SAR and optical data has significantly improved the accuracy in the classification of vegetation types, especially in our study area which is characterized by high environmental heterogeneity.
Keywords
Argan Forest, Sentinel-2, GLCM Texture, SAR Parameters, DEM, Satellite Image Classification
Introduction
Forests represent biodiversity hotspots and play a crucial role in maintaining the ecological balance and the overall well-being of the planet ([4]). Forest cover is essential for global biodiversity, land use dynamics, and various socio-economic aspects in arid and semi-arid environments. They provide resources such as food and fibre, regulate the hydrological cycle, and protect watersheds and their vegetation, water distribution, and other ecological and human services vital to local populations. Forests also contribute to the conservation of many species of plants and animals. However, due to natural and human factors, the inherent balance of ecosystem services provided by our forests has experienced a significant decline ([25]). The arid and semi-arid region stretching from southwest to southeast Morocco is home to the forest argan tree (Argania spinosa [L.] Skeels), which belongs to the Sapotaceae family. This vegetation type, also known as arganeraie, covered about 952.200 ha and has been declared by UNESCO MAB (Man and the Biosphere Reserve) as a biosphere reserve in 1998. It constitutes the third most popular wood species in Morocco, following the sandarac gum (Tetraclinis articulata) and evergreen oak (Quercus ilex - [21]), which have a taproot reaching soil horizons faster ([2]). The argan tree is characterized by slow growth and a spiny, shrubby structure and has a remarkable lifespan of over 200 years. Due to its ecological and physiological properties, A. spinosa plays an essential role in the fight against desertification and drought ([5]). Moreover, it has enormous interest at different levels (economic, medicinal, biological, phylogenetic, ecological, biodiversity) and it is utilized in cosmetics as a revitalizing agent for the skin and the hair ([14]). Indeed, it is a multipurpose species, each part or production of the tree is popular (wood, leaves, fruits, oils) and represents a source of income for land owners. Despite the different roles played by this species, more than half of the argan forest of Morocco has disappeared (mainly on the plains), and the threat posed by its depletion is currently a significant concern among the population and scientists. The cumulative effects of overgrazing, arid climate, poor natural regeneration, and anthropomorphic impacts have exacerbated the decline in tree cover and density. In addition, the overexploitation of the argan tree is irrational, especially given the current high demand for argan oil on the international market ([36]), and climate change, combined with the rarity of natural regeneration, has led to a regression of its range area ([33]).
Creating land cover maps through satellite image data and employing machine learning techniques represent two prominent and contrasting applications within remote sensing ([22]). Remote sensing enables land dynamics to be observed, identified, mapped, assessed, and monitored at various spatial and temporal resolutions. The increasing availability of Earth observation data and technological improvements in processing capability are driving the advancement of sensing as a robust and consistent methodology. Remote sensing offers the flexibility to monitor agricultural areas in the transition from bare ground at the beginning of the season to densely vegetated areas during their maximum growth ([35]). Through freely available satellite imagery (MODIS, Landsat, Sentinel), remote sensing has become one of the most powerful and valuable instruments for investigating global phenomena such as global warming ([24]). Optical (passive sensors) and Synthetic Aperture Radar (active sensors) provide valuable geospatial information to identify tree species by using classification methods on remotely sensed data ([26]).
The use of optical remote sensing for forest type mapping is well established in the literature for such data. The classification of forest types is based on reflected spectral data recorded by optical sensors ([42]). However, there are some factors, such as the existence of many features or complex land cover within the same pixel or the presence of comparable classes (tree species) in the study region, that can produce spectral confusion, leading to a poor separation between different cover types ([15]). On the other hand, SAR remote sensing without a combination of optical sensors is not suited to this context. Nevertheless, SAR data are helpful in combination with other remote sensing products. Numerous studies have shown that, combined with optical remote sensing, it can improve classification quality as the information extracted from SAR images can easily distinguish between forest and non-forest types ([27]). For example, Qin et al. ([30]) proved the feasibility of combining PALSAR and MODIS photos to map forests across broad areas. In addition, Su et al. ([38]) showed promising findings in the ideal mix of predictors and algorithms for forest above-ground biomass mapping. The Shuttle Radar Topography Mission (SRTM) digital elevation model (DEM) was globally consistent and freely available to provide topographic indices useful for forest biomass estimation. Numerous studies proved that integrating Sentinel-2, Sentinel-1, and SRTM datasets (S2, S1, and DEM) give the best overall accuracy ([3]).
In this context, we accurately mapped the geographical distribution of argan trees and established a distinction between this indigenous species and other tree varieties within the Smimou region. Our efforts encompass a range of methodologies involving machine learning algorithms, all aimed at identifying the optimal approach to achieve the highest classification accuracy. One such study authored by Sebbar et al. ([34]) focused on the accurate mapping and precise localization of argan trees in the Smimou region. To achieve this, optical data from Sentinel-2 imagery was used and a dual classification process was performed to overcome the separability challenges. The Support Vector Machine (SVM) classifier and the Decision Tree algorithm were employed to accurately identify and distinguish argan trees from other vegetative elements in the area. Further, Moumni et al. ([26]) assessed the impact of fusing two types of remote sensing data, optical imagery (Sentinel-2) and Synthetic Aperture Radar (SAR) imagery (Sentinel-1), in mapping argan trees in the region, to improve and compare the classification results with those obtained from single source data using the SVM algorithm. A subsequent study by El Moussaoui et al. ([13]) focused on assessing the potential of integrating the Digital Elevation Model (DEM) layer with multi-sensor data (Sentinel-1 and Sentinel-2) to detect, map and identify argan trees from other forest species using various machine learning algorithms such as Support Vector Machine (SVM), Maximum Likelihood (ML) and Artificial Neural Networks (ANN). Interestingly, these studies used a standard parameterization for classification, including the type of filters in the SAR images, the resolution of the DEM layer, and the texture parameters.
The main objective of this study is to evaluate the influence of different SAR parameters, including filter type and texture, on the classification results. In addition, we aim to evaluate the effect of the DEM resolution in combination with remote sensing data in identifying argan forests in the Smimou area, western Morocco. We employed classifications derived from optical NDVI time series and SAR time series integrated with the DEM layer to distinguish argan trees from other tree species, like olive (Olea europaea L.) and sandarac gum. Among the various algorithms tested, the SVM classifier was most successful in distinguishing argan trees from other vegetation types. To achieve the above goals the following steps were conducted: (i) determining the best filter type using the combined SAR products in terms of overall accuracy; (ii) assessing how the texture features based on the GLCM affect the classification results; (iii) examining how the varying resolutions of DEM layers affect the classification accuracy.
Materials and methods
Study area and reference data
The study site is an area of approximately 20 km2 around a small town known as Smimou, located in the Essaouira province, south-eastern part of the Marrakech-Safi region in central Morocco ([13], [26] - Fig. 1). The mountain range covers much of this area, where the Jbel Amsittene is the highest peak of the province (912 m a.s.l.). The Smimou area is characterized by an arid to semiarid climate, where summers are short and hot, and winters cold and rainy. Over the year, the temperature generally ranges from 8 to 26 °C and is rarely below 5 or above 31 °C (⇒ https://fr.weatherspark.com/). Forests cover about 38% of the total Commune area, and the land cover is dominated by two main tree species: the Argan (Argania spinosa) and Sandarac gum (Tetraclinis articulata) in the upper parts ([18]). The main activity of the inhabitants is agriculture, which is slowly developing due to the difficult environmental conditions (lack of rainfall and groundwater). The production system is based on forestry (argan and Sandarac gum), beekeeping, goat, cattle, and cereal farming (barley, wheat, and corn).
Field data
During 2019, detailed information on land cover and land use were collected by visiting 574 sampling sites in collaboration with the local Forestry Research Center of Marrakesh (Centre de Recherche Forestière). The reference data were the same used in El Moussaoui et al. ([13]) and Moumni et al. ([26]). Fig. 2shows the spatial distribution of the surveyed parcels divided into two groups (training and validation).
Methodology
The methodological approach followed consists of four main steps (Fig. 3): (i) downloading Sentinel-1, Sentinel-2 imagery and DEM layers and acquiring the field data; (ii) data preprocessing; (iii) SVM classification; (iv) accuracy assessment, comparison, and analysis; (v) downloading the imagery SAR, S-2, DEM layers and acquiring the field data.
Fig. 3 - Preprocessing and process workflows conducted in the present study. (DEM 12.5 m), (DEM 30 m), (DEM 90 m): digital elevation model with a resolution of 12.5, 30, and 90 meters, respectively.
Remote sensing data
The optical and radar data used come from the two satellites, Sentinel-2 and Sentinel-1, respectively. The choice of these two sensors is mainly influenced by the free availability of their products, as well as their optimal spatial- and temporal resolutions.
Optical imagery
We used 36 Sentinel-2 images downloaded from the Theia-CNES website (⇒ https://peps.cnes.fr/), covering the period from December 2018 to December 2019. These images were distributed across the four seasons and processed to derive the NDVI vegetation index. Further details of the optical imagery can be found in Moumni et al. ([26]).
Synthetic Aperture Radar Imagery
Sentinel-1 images are ortho-rectified on the Sentinel-2 grid to facilitate the joint use of the two missions. This product named “S1Tiling” has been developed within the CNES SAR service, in collaboration with CESBIO ([29]). Sentinel-1 offers four modes of data acquisition: Strip Map (SM), Interferometric Wide Swath (IW), Extra Wide Swath (EW), and Wave (WV). In the present study, 26 SAR images covering the year 2019 were collected from Peps CNES website (⇒ https://peps.cnes.fr/) using the IW swath mode with double polarization (VV and VH).
Digital Elevation Model (DEM) layers
The Shuttle Radar Topography Mission (SRTM) is a combined mission of the National Imagery and Mapping Agency (NIMA) and National Aeronautics and Space Administration (NASA) to acquire global elevation datasets. The SRTM provided digital elevation data (DEMs) for more than 80% of the world. The Digital Elevation Model (DEM) is a numerical representation of the Earth surface that provides fundamental information about terrain relief. SRTM data, with a resolution of 10 m, is accessible for the contiguous USA ([16]). Additionally, SRTM data with resolutions of 12.5, 30, and 90 m are freely available for the entire globe, and can be downloaded from sources such as the Alaska Satellite Facility (ASF) Data Search and the USGS Earth Explorer website. In this study, we are specifically interested in investigating the impact of different DEM data on the overall accuracy and the Kappa index, which serve as key indicators of classification performance. For this reason, three available imagery Digital Elevation Model (DEM), at 12.5, 30, and 90 m spatial resolution covering our study area were acquired from the ASF (⇒ https://search.asf.alaska.edu/), and the USGS Earth Explorer (⇒ http://earthexplorer.usgs.gov/).
Data preprocessing
The Sentinel-1 data were pre-processed using the Sentinel-1 toolbox in the SNAP software and ENVI v. 5.1. The preprocessing steps included thermal noise removal, radiometric calibration; georeferencing was performed by Range Doppler Terrain Correction using a 30 m Digital Elevation Model (SRTM DEM - [19]).
To improve image readability, speckle filtering techniques were applied to reduce noise in the radar image. Several filters available in SNAP were considered, including Lee, Enhanced Lee (3 × 3 and 5 × 5 sizes), Frost, Gamma Map, Boxcar, IDAN4, Median, and Lee Sigma, which was tested with different window sizes, including 5 × 5, 7 × 7, 9 × 9, 11 × 11, 13 × 13, 15 × 15, and 17 × 17, with different parameterisations to determine the most effective configuration. All these filters were examined in order to select the best one for the next step. The filtered images were converted to a logarithmic scale with the unit of Decibel (dB) ([13], [26]).
Texture analysis was conducted to capture spatial relationships between the two pixels separated by a certain distance in the image and neighbouring pixel information ([7], [45]) The gray level co-occurrence matrix (GLCM) suggested by Rajadell et al. ([31]) is one of the most popular methods for calculating second-order texture measures. The GLCM is defined as follows: Each element (i, j) in the GLCM represents the frequency of two pixels in a particular window, with grayscale values i and j and a neighbouring distance of d in the θ direction. Typically, d assumes values of 1 or 2, and θ takes the four directions of (0°, 45°, 90°, and 135°). The GLCM texture measures were computed for double polar (VV, VH) using a 9 × 9 moving window, encompassing all directions and a co-occurrence shift of one pixel (interdigital distance) using SNAP. Generally, eight parameters derived from the GLCM were considered for quantitative texture description: (i) Angular Second Moment (ASM); (ii) Homogeneity (HOM); (iii) Contrast (CON); (iv) Dissimilarity (DIS); (v) Correlation (COR); (vi) Entropy (ENT); (vii) Variance (VAR); (viii) Mean.
Each parameter captures specific textural characteristics within the image and was calculated as follows (eqn. 1 - eqn. 8):
where P(i, j) is the normalized value of the gray-scale at positions i and j of the kernel with a sum equal to 1, while N is the number of gray levels in the quantized image. The μ is the mean for the variance texture measurement, where the terms (μx, μy), and (σx, σy) are the means and standard deviations of Pi and Pj respectively, for the correlation texture measure ([39]).
SVM classification and accuracy assessment
After preprocessing, the data was ready for classification. Support Vector Machine (SVM) classification was used in this step to categorize different land cover or vegetation types based on the available data layers ([13], [26], [34]).
The accuracy evaluation was conducted by analyzing a generated confusion matrix, revealing the correlation between the classification outcomes and the validation data derived from the ground truth. Within this matrix, the diagonal cells represent the count of accurately identified pixels. Calculating the sum of these pixels and dividing it by the total pixel count provides the percentage accuracy or overall classification accuracy (OA).
The Kappa coefficient (K) is another way to quantify the classification outcome. It measures the difference between the actual agreement (indicated by the diagonal elements of the matrix) and the Hypothesis Random Agreement (forecast by the product of the row and column margins). In contrast to overall accuracy, the user and producer accuracies provide an understanding of the quality of the classification of each class, whereas the user accuracy (UA) is defined as the percentage of classified pixels that correctly correspond to the ground truth ([32]), i.e., it is the ratio of correctly predicted observations in each column, while the producer accuracy (PA) indicates for a given class the proportion of the reference data that are classified correctly ([6]). It is calculated as the number of pixels in a given class split by the number of pixels in the reference data in that class. User and producer accuracies can be computed as follows (eqn. 9, eqn. 10):
The F1 score is a meaningful evaluation matrix and a measure of the accuracy of a test. It is calculated from the precision (P, mean producer accuracy), and recall (R, mean user accuracy) of the test, where the precision is the number of true positive results divided by the number of all positive results, including those not correctly identified, and the recall is the number of true positive results divided by the number of all samples that should have been identified as positive ([17]). The F1 score is mathematically expressed as follow (eqn. 11):
Results
Assessing the effect of speckle filter type on the classification accuracy
We present the outcomes obtained by employing diverse filters on the combined SAR product. Tab. 1 reports a comprehensive summary of the performance of different filter types, focusing on OA and K. These metrics play a pivotal role in determining the optimal filter choice for our classification objectives.
Tab. 1 - Overall accuracy (OA) and Kappa index (K) of filter types using the different SVM algorithms.
Scenarios Filter types | VV,VH/VV | VH,VH/VV | VH,VV,VH/VV | VH,VV | ||||
---|---|---|---|---|---|---|---|---|
OA (%) | K | OA (%) | K | OA (%) | K | OA (%) | K | |
Lee | 38.45 | 0.28 | 36.16 | 0.26 | 36.65 | 0.27 | 35.73 | 0.25 |
Lee Sigma (5size) | 38.01 | 0.28 | 36.02 | 0.26 | 36.56 | 0.27 | 34.98 | 0.25 |
Lee Sigma (7size) | 40.19 | 0.31 | 37.35 | 0.27 | 38.41 | 0.29 | 36.81 | 0.25 |
Lee Sigma (9size) | 43.57 | 0.33 | 38.61 | 0.29 | 40.34 | 0.31 | 37.58 | 0.27 |
Lee Sigma (11size) | 44.43 | 0.34 | 41.45 | 0.31 | 43.34 | 0.33 | 40.94 | 0.31 |
Lee Sigma (13size) | 43.57 | 0.33 | 40.83 | 0.30 | 42.01 | 0.32 | 39.97 | 0.30 |
Lee Sigma (15size) | 42.96 | 0.32 | 41.37 | 0.31 | 41.09 | 0.31 | 38.21 | 0.29 |
Lee Sigma (17size) | 42.73 | 0.32 | 41.01 | 0.31 | 42.33 | 0.32 | 39.88 | 0.30 |
Enhancedlee (3size) | 35.96 | 0.26 | 35.62 | 0.25 | 36.51 | 0.26 | 36.85 | 0.26 |
Enhancedlee (5size) | 39.60 | 0.30 | 39.57 | 0.30 | 40.96 | 0.31 | 41.65 | 0.31 |
Frost | 37.40 | 0.27 | 35.76 | 0.25 | 36.31 | 0.26 | 35.30 | 0.25 |
Gamma Map | 39.47 | 0.29 | 37.66 | 0.27 | 38.06 | 0.28 | 36.82 | 0.26 |
Boxcar | 39.58 | 0.30 | 37.23 | 0.27 | 38.82 | 0.29 | 37.01 | 0.26 |
IDAN4 | 39.77 | 0.30 | 38.74 | 0.28 | 38.18 | 0.28 | 36.42 | 0.25 |
Median | 37.43 | 0.27 | 36.51 | 0.26 | 35.60 | 0.25 | 35.53 | 0.25 |
Refinedlee | 37.75 | 0.27 | 35.85 | 0.25 | 36.21 | 0.26 | 33.92 | 0.23 |
The classification results confirm the superiority of Lee sigma with different window size filters in terms of land cover classification and performance. The OA resulting from the classification of the VV, VH/VV using Lee sigma size 11×11 and size 13×13 filters are 44.43% (K = 0.34), and 43.57% (K = 0.33), respectively. Based on the results reported in Tab. 1, it is evident that the Lee sigma 11×11 filter consistently produces superior results by effectively combining multiple SAR products. Therefore, it has been determined as the optimal filter type to be used in the subsequent classification. While numerous studies have extensively investigated the application of SAR imagery for land cover/land use (LC/LU) classification, it is interesting to note that several of these studies have opted for the Lee sigma filter with varying window sizes ([12], [23]). The current study shows notable progress in filter type compared to previous research ([26], [13]), due to the use of Lee Sigma filter with a window size of 11×11, which represents a significant improvement over previous methods and significantly increases the overall effectiveness of the classification process.
Assessing the effect of GLCM texture features on the classification accuracy
Tab. 2 reports the outcomes of the GLCM texture analysis in terms of accuracy assessment (OA) and kappa (K) values in both VV and VH polarizations. The results show that the highest OA (36.35%) and K (0.27) were achieved by employing a stack of eight GLCM textures. This stack was derived from the temporal series of the S1 SAR images, comprising 26 scenes and yielding 8 texture products for each polarization, thus forming a 416-band stack. Notably, differences were observed in the Overall Accuracy of GLCM texture. In the existing literature, the selection of GLCM texture depends on the surface type and land cover. For example, Caballero et al. ([7]) selected four GLCM textures (contrast, correlation, entropy, and variance) were selected in the analysis of an irrigated cultivated plain in the southern province of Buenos Aires, Argentina. In another study, Tavares et al. ([39]) selected three GLCM features (mean, variance and correlation) for analyzing an area in the coastal Amazon, characterised by a complex humid tropical environment with an interconnected relationship between flowing rivers and the ocean ([39]).
Tab. 2 - Summary of the results achieved with the classification based on GLCM texture. (OA): Overall Accuracy, (k): Kappa index.
GLCM texture | OA% | K |
---|---|---|
(1) Contrast | 20.24 | 0.12 |
(2) Angule Second Moment | 30.91 | 0.21 |
(3) Variance | 20.34 | 0.12 |
(4) Correlation | 25.16 | 0.15 |
(4) Mean | 28.79 | 0.20 |
(6) Entropy | 33.70 | 0.22 |
(7) Homogeneity | 32.27 | 0.21 |
(8) Dissimilarity | 27.81 | 0.16 |
(6)+(7) | 33.73 | 0.23 |
(2)+(5)+(6)+(7) | 33.13 | 0.22 |
(1)+(2)+(4)+(6) | 34.01 | 0.24 |
All statistics | 36.35 | 0.27 |
Assessing the effect of DEM spatial resolution on the classification accuracy
To assess the influence of integrating each DEM layer into the SAR, optical and combined products, several tests were carried out. The results in terms of overall accuracy (OA) and K statistic are shown in Tab. 3. Combining the DEM layer with 30 m resolution with the non-combined optical product (NDVI) and the SAR product (VV; VH/VV) gave the highest values. These reached 93.25% with K = 0.91 for the NDVI product and did not exceed 47.55% with K = 0.38 for the (VV; VH/VV) product. On the other hand, the use of the 12.5 m DEM layer gave the best result in the fusion between optical and SAR products (NDVI; VH) with an OA of 91.71% and K = 0.89.
Tab. 3 - Overall accuracy (OA) and Kappa index (K) of the classification using different spatial resolution of the DEM layer.
DEM resolution scenarios |
Without DEM | DEM (12.5 m) | DEM (30 m) | DEM (90 m) | ||||
---|---|---|---|---|---|---|---|---|
OA (%) | K | OA (%) | K | OA (%) | K | OA (%) | K | |
NDVI | 86.87 | 0.84 | 90.50 | 0.88 | 93.25 | 0.91 | Overestimation | |
NDVI; VV | 86.03 | 0.83 | 90.25 | 0.88 | 90.72 | 0.88 | 90.58 | 0.88 |
NDVI+VH | 84.82 | 0.82 | 91.71 | 0.89 | 90.25 | 0.88 | Overestimation | |
VV+VH+NDVI | 83.88 | 0.81 | 87.53 | 0.85 | 88.84 | 0.86 | 88.79 | 0.86 |
VH | 27.23 | 0.14 | 42.12 | 0.33 | 41.56 | 0.31 | 42.38 | 0.32 |
VV | 29.82 | 0 .18 | 43.31 | 0.34 | 42.56 | 0.33 | 41.23 | 0.31 |
VH/VV | 37.78 | 0.28 | 39.78 | 0.29 | 40.38 | 0.30 | 39.56 | 0.29 |
VV+VH/VV | 44.43 | 0.34 | 46.99 | 0.38 | 47.55 | 0.38 | 46.47 | 0.37 |
VH/VV+ Texture | 37.42 | 0.29 | 40.83 | 0.31 | 41.47 | 0.32 | 41.42 | 0.30 |
VV+VH/VV+ Texture | 39.03 | 0.31 | 41.43 | 0.32 | 42.21 | 0.32 | 40.69 | 0.31 |
VV+VH | 40.94 | 0.31 | 44.78 | 0.35 | 44.77 | 0.34 | 41.92 | 0.31 |
VH+VH/VV | 41.45 | 0.31 | 45.91 | 0.36 | 46.67 | 0.37 | 44.98 | 0.35 |
VH+VV+VH/VV+ NDVI | 85.64 | 0.82 | 89.68 | 0.86 | 90.84 | 0.88 | Overestimation | |
VV+VH+ VH/VV | 43.34 | 0.33 | 44.69 | 0.34 | 46.90 | 0.37 | 46.16 | 0.36 |
It should be noted that the inclusion of the 90 m DEM layer in the optical and SAR products did not result in any improvement compared to other DEM layers (12.5 m and 30 m of spatial resolution).
Comparison and analysis
The quality of the classification obtained was further assessed by visually examining the classified images. Fig. 4 illustrates the images obtained for the scenarios showing high overall accuracy (OA). Noticeable differences between the classifications of Sentinel-1 and Sentinel-2 products can be observed. The quality of the classified SAR images is comparatively lower in terms of sharpness, despite the reduction in speckle impact after applying the filter. The low quality of the image is due to the great confusion of the classes “Argan” and “No vegetation” with the olive tree stands, which are dominant on SAR-derived maps. Integrating SAR and optical data in the classification process led to a decrease in the quality of NDVI classification, which can be attributed to scattered pixels in homogeneous areas across the entire region, resulting in a visually noisy appearance of the categorized SAR products.
Fig. 4 - Mapping results using the best classifications obtained from optical (NDVI) and SAR (VV; VH; VH/VV) products.
Including a DEM layer with a resolution of 30 m on the SAR and optical products enhanced the classification results, improved the image quality, and decreased the confusion among the above classes, especially in the mountain areas. This result exceeds previous research findings ([13]).
During the classification of scenarios containing radar products, we observed a lack of sharpness in the definition of the labelled paths on the images, which appeared “bruised”. Therefore, a smoothing process was applied using the ENVI command “Majority/Minority Analysis” which filters the image by changing the value of the central pixel of a window of dimension n×n using the most frequent value in the window ([43]). To avoid an “over-smoothing”, we have chosen a window of dimension 3×3. Radar images before and after smoothing are shown in Fig. 5. Visually, the quality has improved, and these products, derived exclusively from the Sentinel-1 data, achieved the same quality obtained in our previous studies ([13], [27]). Thus, the above smoothing technique was applied once for all scenarios (optical and SAR classified images), giving satisfactory results both in terms of OA and K (Tab. 4).
Tab. 4 - Overall accuracy (OA) and Kappa index (K) before and after the application of the smoothing technique. For more details, see text.
Scenarios | Before Smoothing | After Smoothing | ||
---|---|---|---|---|
OA (%) | K | OA (%) | K | |
VV ; VH/VV | 44.43 | 0.34 | 45.90 | 0.36 |
NDVI ; VV | 86.03 | 0.83 | 88.32 | 0.85 |
NDVI ; VH; VV ; DEM | 88.84 | 0.86 | 91.66 | 0.897 |
DEM ; VV ; VH/VV | 47.55 | 0.38 | 47.99 | 0.39 |
NDVI ; VH ; DEM | 90.25 | 0.88 | 93.01 | 0.914 |
Argan forest map production
Fig. 6 presents the best-produced argan tree maps derived from the use of optical data (NDVI), the fusion of NDVI with DEM layer (NDVI, DEM) and the combination of tree data (NDVI, VH, DEM). Tab. 5 shows the results of the most accurate classification, including the producer accuracy (PA), user accuracy (UA) and F1-score of each class (tree class). These results provide invaluable insight into the performance and efficiency of our classification methodology.
Fig. 6 - Obtained maps of the spatial distribution of the Argan tree using (NDVI; DEM) and (NDVI; VH; DEM).
Tab. 5 - UA and PA of the best results of the optical product (NDVI), SAR product (VV; VH/VV), optical and SAR combined product (NDVI; VV), and optical, SAR, and DEM combined product (NDVI; DEM, VH). (UA): User accuracy, (PA): Producer accuracy.
Class | NDVI | VV; VH/VV | NDVI; VV | NDVI;DEM;VH | ||||||||
---|---|---|---|---|---|---|---|---|---|---|---|---|
PA (%) | UA (%) | F1score | PA (%) | UA (%) | F1score | PA (%) | UA (%) | F1score | PA (%) | UA (%) | F1score | |
Argan | 88.63 | 69.33 | 77.8 | 58.46 | 62.94 | 60.61 | 86.92 | 70.69 | 77.97 | 90.99 | 83.02 | 86.82 |
Olive | 54.23 | 43.25 | 48.12 | 72.52 | 31.99 | 44.39 | 76.49 | 67.84 | 71.91 | 90.04 | 91.87 | 90.94 |
Sandarac gum | 97.38 | 95.7 | 96.53 | 55.19 | 46.78 | 50.64 | 97.95 | 98.96 | 98.45 | 97.69 | 98.96 | 98.32 |
Sandarac gum+ Argan | 75.08 | 90.04 | 81.88 | 61.21 | 68.84 | 64.8 | 81.59 | 93.56 | 87.16 | 96.48 | 98.01 | 97.23 |
Fallow | 86.19 | 97.63 | 91.55 | 44.37 | 29.58 | 35.49 | 67.8 | 94.79 | 79.05 | 76.27 | 90.36 | 82.72 |
Agricultural | 98.53 | 88.16 | 93.05 | 45.06 | 29.49 | 35.65 | 99.68 | 82.59 | 90.33 | 98.41 | 80.26 | 88.41 |
No vegetation | 95.51 | 98.06 | 96.76 | 21.55 | 48.85 | 29.91 | 95.32 | 94.42 | 94.86 | 93.23 | 96.54 | 94.85 |
Discussion
In this study we tested different filters available in the SNAP software to identify the most appropriate one in terms of overall accuracy (OA) and producer accuracy (K). The results showed that the Lee sigma filter with a size of 11×11 gave the best result in term of OA and the quality of image for both polarisations (VV, VH) and other scenarios. Interestingly, this finding contrasts with the approach adopted by Moumni et al. ([26]) where the enhanced Lee filter was used as default, despite the similarity of data and study region.
In this study, most classifications showed comparable accuracy in VH and VV polarizations when only Sentinel-1 products were considered. However, the classification based on the VH/VV ratio resulted in higher accuracy levels than individual polarizations, which contrasts with the findings obtained by Chakhar et al. ([8]). The VH/VV ratio could be successfully used for biophysical parameter retrieval and direct biomass assimilation in crop models. Similar results have been reported in previous studies ([41]). Indeed, the combination of eight GLCM parameters used in our study yielded better results than the standard combination of three parameters (variance, correlation and mean) used by Moumni et al. ([26]). Moreover, the inclusion of texture statistics derived from the SAR images using the GLCM did not improve classification accuracy compared to SAR products alone. This finding contrasts with our initial expectations and differs from previous studies ([1], [44]).
Our results reveals that the optical data (NDVI) achieved the highest classification accuracy among the non-combined products, with an OA of 86.87% and K value of 0.84. In contrast, the classification of the best-case scenario using SAR data (VV, VH/VV) resulted in an OA of 45.90% and K value of 0.36 after applying a smoothing technique. The decrease in classification accuracy observed in SAR products can be attributed to the impact of various physical variables associated with crop biomass, structure, and ground conditions on SAR backscatter signals. Factors such as soil moisture, surface roughness, and terrain topography affect the backscatter from the ground, while vegetation backscatter is affected by factors like vegetation 3D structure and water content ([20], [40]). The F1 scores of the [VV, VH/VV] scenario (Tab. 5) show that the most misclassified cover types are no vegetation (29.91%), agricultural (35.65%), fallow (35.49%), and olive (44.39%). The confusion among these classes is explained by the similarity of the SAR backscatter (Fig. 7).
As for the optical classification, the olive tree is the most confused vegetation type (F1 score=48.12%). In our study area, olive trees are generally cultivated around small towns for local use, with different densities and with or without annual cultural cares. Despite there are a few zones in the study area where the argan is mixed with olive trees, the correct classification of this vegetation type is hampered by the similarity of the optical profiles (NDVI) of the olive and argan+sandarac gum ([26]). These results are consistent with those obtained by Chakhar et al. ([8]) and Clauss et al. ([9]).
In this study the fusion of multisensory data (NDVI, VV) provided the optimal combination of optical and SAR data and resulted in higher classification accuracy (OA = 88.32%, K = 0.85) compared to the classification based solely on optical data (OA = 86.87%, K = 0.84). The F1 score for the olive class significantly improved from 48.12% to 71.91% with the data combination (Tab. 5). This improvement can be attributed to reduced confusion between olive tree and other classes. These results highlight the strong correlation between the optical and SAR sensors. The combination of different data sources, including optical imagery and SRTM (DEM) led to significant improvements in classification results (OA = 93.25%, K = 0.91). The integration of data sources enhances the accuracy and reliability of the classification process, underscoring the effectiveness of using multiple data types in remote sensing studies. Tab. 5 reveals notable improvements in the PA and UA, particularly for the olive class. Prior to the addition of the DEM layer, the PA was 54.23% and the UA was 43.25% for the olive class. However, after combining the data, including the DEM layer, the PA increased significantly to 90.44%, and the UA rose up to 89.72% for the same class. This improvement can be attributed to the integration of the DEM layer, thereby achieving a better distinction between terrain relief models of varying complexity and digital surface representations.
In contrast to our findings, Sirro et al. ([37]) reported that the classification accuracy using optical data was superior to that obtained using optical+SAR data in forest and land cover classification. On the other hand, our results are fully consistent with those of Mustak et al. ([28]) who evaluated the performance of combined optical and SAR imagery for crop discrimination. These findings emphasize the importance of considering specific study contexts and objectives when interpreting classification results.
Optical, SAR, and SRTM (DEM) data have been combined in several studies focused on crop type classification ([10], [11]), confirming that the combinations of these data types yields more robust results compared to classification based solely on images. The innovative approach adopted in the current study, which involves testing SAR parameters and integrating optical, SAR and DEM data, has significantly improved the accuracy in the classification results of endemic argan tree areas in Central Morocco, making the detection of its spatial extension more effective and accurate.
Conclusion
The main goal of this study was to assess the influence of various SAR parameters and DEM layer resolutions on the identification accuracy of argan trees in the rural municipality of Smimou (Central Morocco) using remote sensing data. Additionally, we combinined optical satellite imagery, SAR data, and DEM layers aimed to improve argan tree identification.
To classify the Sentinel-1 images, we applied different filters and employed the SVM algorithm. Our results showed that the Lee sigma 11 × 11 size filter yielded the best performance in the classification of tree types. To improve accuracy, we applied a smoothing technique to the post-classified items. The classification results demonstrated that the integration of DEM layer with a resolution of 30 m produced the best OA and K values. Moreover, the application of smoothing technique to both Sentinel-1 and Sentinel-2 data led to a notable enhancement in the accuracy and quality of the resulting map. Further, the inclusion of the DEM layer in conjunction with SAR and optical products increased the accuracy by approximately 6% to 7%.
Based on our findings, future research should explore different SAR parameters to further improve the results of Sentinel-1 imagery. Additionally, combining optical and SAR time series may lead to more accurate results. We also recommend extending this strategy to cover a larger area within the Essaouira province. This study provides a more comprehensive and insightful understanding of the complex land cover patterns and dynamics in the study area. The results of this study have the potential to support decision making and more efficient management of the unique argan tree ecosystem and its associated agricultural landscape.
Conflicts of interest
The authors declare no conflicts of interest.
Acknowledgments
The authors would like to thank the Center of Forestry Research CRF (Centre de Recherches Forestières) for their technical support and accompaniment during field campaigns.
All authors express their gratitude to the reviewers for their helpful comments and suggestions.
References
Gscholar
CrossRef | Gscholar
CrossRef | Gscholar
CrossRef | Gscholar
CrossRef | Gscholar
CrossRef | Gscholar
Authors’ Info
Authors’ Affiliation
Aicha Moumni 0000-0002-0203-8462
Abderrahman Lahrouni 0000-0002-2118-8570
Department of Physics, Faculty of Sciences Semlalia, Cadi Ayyad University, Marrakech (Morocco)
Corresponding author
Paper Info
Citation
El Moussaoui EH, Moumni A, Lahrouni A (2024). Assessing the influence of different Synthetic Aperture Radar parameters and Digital Elevation Model layers combined with optical data on the identification of argan forest in Essaouira region, Morocco. iForest 17: 100-108. - doi: 10.3832/ifor4183-016
Academic Editor
Agostino Ferrara
Paper history
Received: Jul 15, 2022
Accepted: Apr 17, 2024
First online: Apr 24, 2024
Publication Date: Apr 30, 2024
Publication Time: 0.23 months
Copyright Information
© SISEF - The Italian Society of Silviculture and Forest Ecology 2024
Open Access
This article is distributed under the terms of the Creative Commons Attribution-Non Commercial 4.0 International (https://creativecommons.org/licenses/by-nc/4.0/), which permits unrestricted use, distribution, and reproduction in any medium, provided you give appropriate credit to the original author(s) and the source, provide a link to the Creative Commons license, and indicate if changes were made.
Web Metrics
Breakdown by View Type
Article Usage
Total Article Views: 239
(from publication date up to now)
Breakdown by View Type
HTML Page Views: 48
Abstract Page Views: 86
PDF Downloads: 95
Citation/Reference Downloads: 0
XML Downloads: 10
Web Metrics
Days since publication: 9
Overall contacts: 239
Avg. contacts per week: 185.89
Article Citations
Article citations are based on data periodically collected from the Clarivate Web of Science web site
(last update: Feb 2023)
(No citations were found up to date. Please come back later)
Publication Metrics
by Dimensions ©
Articles citing this article
List of the papers citing this article based on CrossRef Cited-by.
Related Contents
iForest Similar Articles
Review Papers
Accuracy of determining specific parameters of the urban forest using remote sensing
vol. 12, pp. 498-510 (online: 02 December 2019)
Review Papers
Remote sensing-supported vegetation parameters for regional climate models: a brief review
vol. 3, pp. 98-101 (online: 15 July 2010)
Research Articles
Estimation of forest cover change using Sentinel-2 multi-spectral imagery in Georgia (the Caucasus)
vol. 13, pp. 329-335 (online: 07 August 2020)
Research Articles
Estimation of forest leaf area index using satellite multispectral and synthetic aperture radar data in Iran
vol. 14, pp. 278-284 (online: 29 May 2021)
Research Articles
Mapping Leaf Area Index in subtropical upland ecosystems using RapidEye imagery and the randomForest algorithm
vol. 7, pp. 1-11 (online: 07 October 2013)
Research Articles
Estimating biomass of mixed and uneven-aged forests using spectral data and a hybrid model combining regression trees and linear models
vol. 9, pp. 226-234 (online: 21 September 2015)
Review Papers
Remote sensing support for post fire forest management
vol. 1, pp. 6-12 (online: 28 February 2008)
Review Papers
Remote sensing of selective logging in tropical forests: current state and future directions
vol. 13, pp. 286-300 (online: 10 July 2020)
Technical Reports
Detecting tree water deficit by very low altitude remote sensing
vol. 10, pp. 215-219 (online: 11 February 2017)
Research Articles
Assessing water quality by remote sensing in small lakes: the case study of Monticchio lakes in southern Italy
vol. 2, pp. 154-161 (online: 30 July 2009)
iForest Database Search
Search By Author
Search By Keyword
Google Scholar Search
Citing Articles
Search By Author
Search By Keywords
PubMed Search
Search By Author
Search By Keyword