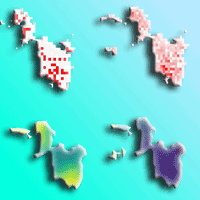
Modelling natural regeneration of Oak in Saxony, Germany: identifying factors influencing the occurrence and density of regeneration
iForest - Biogeosciences and Forestry, Volume 16, Issue 1, Pages 47-52 (2023)
doi: https://doi.org/10.3832/ifor4064-015
Published: Feb 16, 2023 - Copyright © 2023 SISEF
Research Articles
Abstract
In the course of climate change, natural regeneration of oaks (Quercus spp.) is gaining in importance for forest conversion to climate-adapted mixed forests. In order to predict areas in which natural oak regeneration could establish, variables influencing the occurrence and density of oak regeneration were identified using geostatistical zero-altered negative binomial generalized linear models (ZANB). For this purpose, large-scale inventory data from the state forest of Saxony were analysed. The dataset was derived from 6060 permanent plots. The results show that the occurrence of oak regeneration depends on a number of environmental variables. In addition to seed availability, the establishment environment, especially with regard to the light ecology of oak regeneration, was important. High basal area of pine increased the probability for oak regeneration occurrence. The most important variables for the regeneration density of oak have similarly been found to be those describing the seed availability. The highest regeneration densities are predicted within oak stands, with an optimum relationship at 25 m2 ha-1 of oak basal area. The results further show that a high regeneration density was achieved on sites with low fertility and favourable light conditions. Oak regeneration density increased with increasing browsing percent on rowan, indicating that browsing on oak can be reduced if other palatable species are available. Using the identified variables, the occurrence and density of oak regeneration can be predicted in space with high accuracy. The statistical tool developed can be used for planning forest conversion incorporating natural regeneration.
Keywords
Oak, Established Natural Regeneration, INLA, Zero-altered Negative Binomial Model, Spatial Random Effects, Bayesian Inference
Introduction
Pedunculate oak (Quercus robur L.) and Sessile oak (Quercus petraea [Matt.] Liebl.) are particularly widespread in Central Europe, and are among the ecologically and economically most important deciduous tree species ([35], [40]). In the course of climate change, both European oak species are important tree species that will probably gain in importance as components of mixed species stands due to their drought tolerance ([54], [2], [28]). Modern silvicultural planning prefers natural oak regeneration to planting in terms of cost reduction ([51], [24]), genetic diversity ([9]) and undisturbed root development ([20]). However, natural oak regeneration often fails because a number of environmental factors take effect during the regeneration cycle ([52], [49], [41], [26]).
Regeneration establishment is the result of a cascade of successive processes, which start with flowering and fruiting ([18]). Studies suggest that the availability of acorns (i.e., masting behavior - [8], [22]), as well as their dispersal potential ([4]), are not relevant in limiting the occurrence of natural regeneration ([10]). In contrast, during storage, many acorns can be damaged by fungi ([48]) or destroyed by predation ([12], [45], [44]). During germination and following establishment, abiotic factors such as radiation availability ([53], [1], [39]) and water availability, but also biotic factors such as competing vegetation ([11], [23], [33]), pathogens ([13]) and browsing by deer ([6]) are crucial for successful regeneration.
Combining large-scale inventory data and environmental information (e.g., seed dispersal) factors influencing the success of natural oak regeneration can be identified ([1], [41]). Based on these influencing factors, spatial predictions of natural oak regeneration can be made with geostatistical models and thus be integrated into forest management practice ([7], [27]). This leads to the research question of the present work: (i) which are the most relevant factors influencing the presence and absence of natural oak regeneration? And (ii) if oak regeneration is present, which factors influence the density of oak regeneration?
Material and methods
Study area and inventory design
The study was conducted in the state forest of Saxony, Germany. The data used for the study were compiled as part of a forest inventory and provided by Sachsenforst, i.e., the administration that manages the state forest of Saxony. The data was collected in the period from 2018 to 2020. As part of the forest inventory, 6060 plots were surveyed on a square grid with a side length of 200 metres.
The plots are located in the two altitudinal zones “hill terrain” and “lower mountain terrain” of the Ore mountains (Fig. 1). An altitudinal gradient of 94 to 592 m a.s.l. is covered. The hill terrain is dominated by a strong loess-loam influence. In the hill terrain, temporally waterlogged sites are particularly frequent.
The presence and density of naturally regenerated oaks were recorded within a subplot with a radius of 2 m (≈ 12.57 m2) located 5 m north of the plot centre. Within each subplot, regeneration with a height ≥ 20 cm and < 50 cm was measured. In order to examine factors influencing the dependent variable, various explanatory variables were used. These are divided into plot-specific variables, site variables and variables describing the surrounding. Plot-specific information for the overstory was obtained from two concentric plots (6 and 12 m radius). Information obtained from the plots are quadratic mean diameter (dq), total basal area, tree species-specific basal areas, stand density index (SDI) and a spatially interpolated browsing percentage on rowan. For further information on the inventory design, please refer to Axer et al. ([3]). Since seed dispersal also extends the radius of the plot ([30]), remote sensing data were incorporated to determine distances to the nearest potential seed trees. Site variables that were considered for the analysis were altitude, nutrient content, moisture level and pH, and spatially interpolated C stocks and N stocks.
Modelling oak regeneration occurrence and density
Due to a spatial dependence of the regeneration data and a huge proportion of plots without oak regeneration, zero-altered negative binomial generalized linear models (ZANB) with spatial random effects were used (eqn. 1). The occurrence {0.1} and density of oak regeneration (ODi - i =, 1, …, n) at n observations in the plots depending on covariates can be examined and thus facilitate an ecological understanding. Following the example of Zuur et al. ([55]), the model was fitted in a Bayesian framework using the integrated nested Laplace approximation of the package INLA ([34]) in R ([47]). The following formula describes the full ZANB model used to investigate the variables (eqn. 1 to eqn. 7):
where P0 = [k/(µi+k)]k, πi is the probability that oak regeneration occurs on the plot and is modelled via a Bernoulli GLM (General Linear Model). The γs represent the n coefficients of the Bernoulli model multiplied by each of the n chosen explanatory variables zi. A negative binomial distribution with zero truncation is used for the non-zero frequencies μ1. The βs represent the m coefficients of the count model multiplied by each of the m selected explanatory variables xi. The zero-truncated negative binomial model estimated a hyperparameter k termed size that accounts for overdispersion. For the parameter estimation of the fixed effects, the default prior distributions of R-INLA (i.e., normal distributions) were used.
The terms ui and vi are random intercepts, which are assumed to be spatially correlated in the occurrence and density part of the model with the mean value 0 and the covariance matrix ∑. It was assumed that u and v show Markovian behavior and follow a Gaussian Markov Random Field (GMRF). To quantify the associated covariance matrices of u and v, Matérn covariance functions were used and numerically approximated using SPDE (continuous domain stochastic partial differential equation). In this way, parameters are obtained that characterize the spatial random field.
A comparative analysis conducted previously suggests that those models containing only covariates and those models containing only a spatial random term had poorer model goodness of fit than the full model, which includes both covariates and spatial random effects. This result from the preliminary analysis suggests that the spatial relationship between plots contains valuable information that is not captured by the environmental covariates on their own. Therefore, the final ZANB model included spatial correlation in both the count and binary parts of the model. The fixed effects for regeneration occurrence and density are described separately below. Subsequently, the spatial random effects and the overall prediction by the model are presented.
Results and discussion
Occurrence of oak regeneration
For 83.4% of the plots, there was no oak regeneration in the size class investigated. However, oak regeneration occurred on 1005 plots. The most important variables for the occurrence of oak regeneration were oak quadratic mean diameter in the overstory of a plot and the distance to the nearest oak stand (Fig. 2, Tab. 1). These two variables indicate that both barochory and zoochory are decisive for the dispersal of acorns. In oak stands with dq ≥ 40 cm, oak regeneration can be expected. With increasing distance to the nearest oak, the probability of oak regeneration occurrence decreases, but oak regeneration can be expected even at distances greater than 1 km ([4]). Here, however, the establishment environment is crucial: while high total basal area and beech basal area have a negative effect on the occurrence and reflect the light requirements of oak regeneration ([1], [39]), the probability of oak regeneration increases with increasing pine basal area (Tab. 1). A preference of the Eurasian jay (Garrulus glandarius L.) for the dispersal of acorns into pine stands is clearly indicated ([19], [31]). Furthermore, the favourable conditions for the establishment of oak regeneration in pine stands are due to the comparatively high crown transparency, the low influence of abiotic and biotic damaging factors, the favourable stand climate, and a favourable flora composition ([42], [14], [38], [46], [30], [31]). An admixture with beech, on the other hand, often leads to problems with oak regeneration due to shading and interspecific competition within the regeneration ([33], [1]). With a beech basal area of 20 m2 ha-1, the probability of oak regeneration is significantly reduced (Fig. 2).
Fig. 2 - Predicted probability of the occurrence (above) and density (below) of natural regeneration of oak depending on distance to the nearest oak stand, oak basal area, oak quadratic mean diameter (dq), N content and total basal area with varying pine basal area (BA_SP), beech basal area (BA_EB), and pH for regeneration occurrence and with varying pine quadratic mean (dqSP), beech basal area, browsing percent and Cation exchange capacity (CEC) (all continuous variables are set to their mean; all factors are set to their most frequent factor level).
Tab. 1 - Fixed effects of the occurrence and density part of the ZANB models for oak natural regeneration. The optimised model with the lowest WAIC values are presented. In the table the output is shown with the posterior mean and the 95 % credible intervals (0.025 and 0.975). Interpreting the estimated values, it should be noted that the covariates have quite different ranges.
Model | Covariate | Mean | 0.025 quant |
0.975 quant |
---|---|---|---|---|
Occurrence | Intercept | -2.039 | -3.179 | -0.912 |
Distance to oak stand | -0.001 | -0.001 | -0.001 | |
Dq oak | 0.023 | 0.016 | 0.030 | |
Dq pine | 0.007 | 0.001 | 0.014 | |
N content | -0.002 | -0.003 | -0.001 | |
pH value | 0.001 | 0.001 | 0.004 | |
Basal area | -0.030 | -0.056 | -0.004 | |
Basal area beech | -0.053 | -0.086 | -0.023 | |
Basal area oak | 0.054 | 0.039 | 0.070 | |
Basal area pine | 0.036 | 0.021 | 0.051 | |
Stand density index | -0.001 | -0.002 | -0.001 | |
Canopy cover | 0.819 | 0.231 | 1.406 | |
Groundwater-affected | -0.933 | -1.653 | -0.232 | |
Well-drained | 0.407 | -0.031 | 0.865 | |
Temporarily waterlogged | 0.329 | -0.122 | 0.797 | |
Density | Intercept | 0.812 | 0.432 | 1.182 |
Distance to oak stand | -0.001 | -0.002 | -0.001 | |
Dq oak | 0.021 | 0.016 | 0.027 | |
Dq pine | -0.009 | -0.014 | -0.004 | |
Basal area beech | -0.048 | -0.083 | -0.012 | |
Basal area oak | 0.070 | 0.049 | 0.091 | |
I(Basal area oak)^2 | -0.001 | -0.001 | -0.001 | |
Browsing percent rowan | 0.009 | 0.004 | 0.014 | |
CEC | -0.006 | -0.009 | -0.003 | |
N content | -0.001 | -0.002 | -0.001 |
While beech regeneration is less frequent on temporarily waterlogged sites ([3]), no difference between well-drained sites and temporarily waterlogged sites can be demonstrated for oak regeneration (Tab. 1). Only on groundwater-affected locations oak regeneration probability is reduced ([32]). A negative influence of high nitrogen stocks was demonstrated (Fig. 2), which is in accordance with the investigations of Mirschel et al. ([38]). Furthermore, a higher probability of oak regeneration occurrence was demonstrated at higher pH values. Both, nitrogen content and pH value characterise soil quality. Similar results with regard to soil enzyme activity were reported by Dobrowolska et al. ([15]).
Humus cover and the type of ground vegetation are indirectly described by the nitrogen content of the topsoil. A negative influence of ground vegetation has also been demonstrated in previous studies ([11], [41]).
Density of oak regeneration
On average, 4.5 oak saplings (3600 ind. ha-1) were found in the 1005 colonised plots (16.5 % colonised). The distance to the nearest oak stand strongly influences the regeneration density ([4]). At high dispersal distances, only low regeneration densities around 1000 trees per hectare were predicted (Fig. 2). These densities are consistent with previous studies of jay induced natural oak regeneration ([42]). The influence of pine dq indicates that there seems to be a window of opportunity for oak regeneration. As the diameter of the pine stand increases, the predicted oak regeneration density of the investigated size class decreases (Tab. 1). This can be attributed to an increasing disruption of canopy closure due to thinning and the establishment of other competitive tree species and grasses at different successional phases ([17]). This successional process within pine stands was also demonstrated by Onaindia et al. ([46]).
Oak stands have a significant positive effect on oak regeneration density ([1]). For plots within oak stands, an optimum relationship results with regard to the oak basal area (Tab. 1). As the oak basal area increases, the proportion of other tree species in the basal area decreases, so that light transmission improves in addition to seed quantity ([36], [25]). The highest regeneration densities were predicted at 30 m2 ha-1 (Fig. 2). This is in line with Annighöfer et al. ([1]), who were able to identify an oak basal area of 25 m2 ha-1 as the optimum. The significant influence of the beech basal area also affects the oak regeneration density: with a higher beech basal area, the oak regeneration density is clearly reduced ([37]). In line with the oak basal area, the oak dq also shows a positive influence on the regeneration density (Fig. 2). With increasing dbh, the number of seeds produced increases and the light transmission improves.
The browsing percentage on rowan showed a surprising effect. When the percentage of rowan browsing is high, the density of oak regeneration is higher (Tab. 1). Similar results could also be found by Mirschel et al. ([38]) and Annighöfer et al. ([1]). Due to the high browsing preference of rowan, it may happen that oak is protected by selective browsing ([43], [29]). However, oaks are also preferentially browsed ([21]). Nevertheless, recent studies suggest that short-term browsing mainly reduces the growth of oak seedlings rather than increasing its mortality ([6], [5]). A significantly lower proportion of plots with larger oak regeneration in the study area supports this observation (> 50 cm and < 130 cm height: 5 %; height > 130 cm and dbh < 7 cm: 2.4 % colonised).
The variables describing soil conditions have a similar effect on the density as on the occurrence of oak regeneration ([38]). Vegetation cover indicating low-nitrogen sites, such as blueberry (Vaccinium myrtillus L.) and moss, has proven to be particularly favourable for high regeneration density ([50], [38], [16]). The same effect is indicated for the cation exchange capacity. On sites with lower CEC, the predicted regeneration density is higher (Fig. 2). This has also been confirmed by the work of Annighöfer et al. ([1]).
Model prediction
Maps for the presence and density of oak regeneration can be created based on the explanatory variables and random effects ([27]). Spatial predictions for a given location are possible because the model provides an approximation of the entire random field ([34]). The spatial random field was defined by the hyperparameters θ1 and θ2 which defined the spatial range and variance of the random field given in Tab. 2.
Tab. 2 - Random field hyperparameters for the ZANB model. The term u characterizes the spatial random field of the zero-truncated part of the model, while v characterizes the spatial random field for the Bernoulli part of the model.
Spatial random field |
Hyper-parameter | Mean | 0.025 quant |
0.975 quant |
---|---|---|---|---|
u | θu1 | 8.367 | 5.231 | 12.397 |
θu2 | -5.508 | -6.732 | -3.557 | |
v | θv1 | 6.845 | 5.625 | 8.232 |
θv2 | -6.900 | -7.716 | -6.176 |
Fig. 3 shows the spatial predictions for a part of the study area. It also becomes apparent that in many areas there is a very good fit between observation and prediction. It becomes clear that natural regeneration occurs in many areas. However, the spatial random fields indicate that there was an obvious spatial pattern of unexplained variability where the fixed effects alone underestimate both presence and density. At the same time, there are areas where the fixed effects overestimate the occurrence probability and the regeneration density (Fig. 3). The precision of the overall prediction provided by the model can be seen both in Fig. S1 (Supplementary material) and in the CPO values (Tab. S1). The comparison of observed densities with predicted densities, root mean square error (RMSE) and bias estimate in the form of the mean prediction error (E), as well as the CPO values of the leave-one-out cross-validation indicated a quite good prediction accuracy (Tab. S1 in Supplementary material).
Fig. 3 - Example area within the study area with observed occurrence and density of oak regeneration (left), spatial prediction (centre) and spatial random field of the ZANB model for a part of the study area. Dark blue shaded areas have a lower probability of occurrence and high density of regeneration than predicted by the fixed effects. Yellow areas have a higher probability.
Based on the spatial patterns of the random effects, unobserved processes responsible for these patterns can be deduced ([55]). Influencing factors such as hunting intensity, areas avoided by deer due to forest visitors, small-scale differences in ground vegetation, or unrecorded seed sources could, for example, be the underlying factors for these patterns.
Conclusions
Geostatistical models offer the possibility to analyse regeneration data. Factors influencing the occurrence and density of oak regeneration can be studied separately. Considering spatial random effects assists in explaining more variation in the model and thus improves model prediction. In addition, the patterns of spatial random effects provide indications of unobserved processes. These predictions can be used to incorporate natural regeneration in decisions on forest conversion activities at the forest management level.
Oak regeneration can be used in the conversion of coniferous-dominated stands into climate-adapted mixed stands. Areas where artificial regeneration is necessary can also be identified using predictive models. This is of enormous importance for forest management in terms of cost reduction. The results suggest that there is a huge natural regeneration potential. The ingrowth of natural regeneration can be promoted by targeted measures such as thinning of the overstory, fencing or intensified hunting.
Acknowledgements
This work was supported by Staatsbetrieb Sachsenforst. The authors would like to thank Sachsenforst for providing valuable data from their forest inventory. We also thank the anonymous reviewers for their useful advices. We would like to express our special thanks to Robert Larkin for proofreading the manuscript and providing valuable advice.
Author Contributions
M.A. conceived the study and methodology, processed data, performed statistical analysis and validation, wrote the original draft, and visualised the published work. S.M. participated in conceptualisation and methodology of the study, contributed to data processing and validation, reviewing, and editing of the paper. R.S. assisted with statistical analysis and validation and, contributed to reviewing the paper. D.E. participated in conceptualisation of the study, provided resources, and contributed to validation and reviewing the paper. S.W. conceived the study, administered the project, provided resources and supervision, reviewed, and edited the paper, and acquired funding.
References
CrossRef | Gscholar
Gscholar
CrossRef | Gscholar
Gscholar
CrossRef | Gscholar
CrossRef | Gscholar
Gscholar
Authors’ Info
Authors’ Affiliation
Sven Wagner 0000-0003-3796-3444
Chair of Silviculture, Institute of Silviculture and Forest Protection, TU Dresden, 01737, Tharandt (Germany)
Dirk-Roger Eisenhauer
Competence Centre Forest and Forestry, State Forest Enterprise Sachsenforst, 01796, Pirna (Germany)
Chair of Forest Biometrics and Forest Systems Analysis, Institute of Forest Growth and Forest Computer Sciences, TU Dresden, 01737, Tharandt (Germany)
Corresponding author
Paper Info
Citation
Axer M, Martens S, Schlicht R, Eisenhauer D-R, Wagner S (2023). Modelling natural regeneration of Oak in Saxony, Germany: identifying factors influencing the occurrence and density of regeneration. iForest 16: 47-52. - doi: 10.3832/ifor4064-015
Academic Editor
Marco Borghetti
Paper history
Received: Jan 11, 2022
Accepted: Dec 22, 2022
First online: Feb 16, 2023
Publication Date: Feb 28, 2023
Publication Time: 1.87 months
Copyright Information
© SISEF - The Italian Society of Silviculture and Forest Ecology 2023
Open Access
This article is distributed under the terms of the Creative Commons Attribution-Non Commercial 4.0 International (https://creativecommons.org/licenses/by-nc/4.0/), which permits unrestricted use, distribution, and reproduction in any medium, provided you give appropriate credit to the original author(s) and the source, provide a link to the Creative Commons license, and indicate if changes were made.
Web Metrics
Breakdown by View Type
Article Usage
Total Article Views: 5667
(from publication date up to now)
Breakdown by View Type
HTML Page Views: 2074
Abstract Page Views: 1991
PDF Downloads: 1256
Citation/Reference Downloads: 3
XML Downloads: 343
Web Metrics
Days since publication: 865
Overall contacts: 5667
Avg. contacts per week: 45.86
Article Citations
Article citations are based on data periodically collected from the Clarivate Web of Science web site
(last update: Mar 2025)
Total number of cites (since 2023): 4
Average cites per year: 1.33
Publication Metrics
by Dimensions ©
Articles citing this article
List of the papers citing this article based on CrossRef Cited-by.
Related Contents
iForest Similar Articles
Research Articles
Methods for predicting Sitka spruce natural regeneration presence and density in the UK
vol. 12, pp. 279-288 (online: 23 May 2019)
Research Articles
Spatial diversity of forest regeneration after catastrophic wind in northeastern Poland
vol. 9, pp. 414-421 (online: 29 January 2016)
Research Articles
Bayesian geographically weighted regression and its application for local modeling of relationships between tree variables
vol. 11, pp. 542-552 (online: 01 September 2018)
Research Articles
Distribution factors of the epiphytic lichen Lobaria pulmonaria (L.) Hoffm. at local and regional spatial scales in the Caucasus: combining species distribution modelling and ecological niche theory
vol. 17, pp. 120-131 (online: 30 April 2024)
Research Articles
Modeling compatible taper and stem volume of pure Scots pine stands in Northeastern Turkey
vol. 16, pp. 38-46 (online: 22 January 2023)
Research Articles
Nonlinear mixed model approaches to estimating merchantable bole volume for Pinus occidentalis
vol. 5, pp. 247-254 (online: 24 October 2012)
Research Articles
Modeling stand mortality using Poisson mixture models with mixed-effects
vol. 8, pp. 333-338 (online: 05 September 2014)
Review Papers
Problems and solutions to cork oak (Quercus suber L.) regeneration: a review
vol. 16, pp. 10-22 (online: 09 January 2023)
Research Articles
Short- and long-term natural regeneration after windthrow disturbances in Norway spruce forests in Bulgaria
vol. 11, pp. 675-684 (online: 23 October 2018)
Research Articles
A geographically weighted deep neural network model for research on the spatial distribution of the down dead wood volume in Liangshui National Nature Reserve (China)
vol. 14, pp. 353-361 (online: 27 July 2021)
iForest Database Search
Search By Author
Search By Keyword
Google Scholar Search
Citing Articles
Search By Author
Search By Keywords
PubMed Search
Search By Author
Search By Keyword