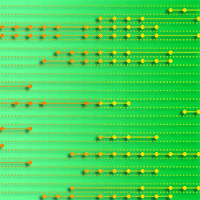
Universal reaction norms for the sustainable cultivation of hybrid poplar clones under climate change in Italy
iForest - Biogeosciences and Forestry, Volume 15, Issue 1, Pages 47-55 (2022)
doi: https://doi.org/10.3832/ifor3989-015
Published: Feb 08, 2022 - Copyright © 2022 SISEF
Research Articles
Abstract
The cultivation of hybrid poplar clones is increasing worldwide. Hundreds of hectares of plantations now occur across Europe and other continents such as North America, using tested clones and novel genotypes. Research effort aims are to develop fast growing disease- and pest-resistant clones to improve production quality and quantity. In this study the phenotypic plasticity of poplar clones was tested across environmental and temporal gradients. The growth performance of 49 hybrid poplar clones recorded between 1980 and 2021 was analysed using a mixed-effects model with climatic data as a predictor variable. Clones were aggregated into two groups according to their breeding protocol (i.e., standard clone, and improved material) and their growth modelled for future climate scenarios of RCPs 2.6 and 8.5 using a downscaled version of the variants 01 and 21 of UKCP18 climate projections dataset for three 30-year normal period time-slices: 2030s, 2040s, 2050s. The fitted growth models showed highly significant results, explaining more than 85% of the variance, with a mean relative absolute error of approximately 2%. Improved material showed more resistance to warmer and drier climates and less sensitivity to the changing climate. While no unique pattern was found when comparing growth performances, new improved clones were more productive than older clones (e.g., “I-214”) with an additional benefit of resistance to rust and pests. Spatial predictions confirmed the Po valley as the most important geographic area for poplar cultivation in Italy, but zones in Central and Southern Italy show potential. However, the Po Valley is also where poplars are predicted to be suitable in the next decades with large uncertainties. The analysis identified the need for more research on the topic of poplar breeding. For example, models using the most extreme (warm and dry) climate projection, variant 01 of RCP8.5 of the UKCP18, exceeded the historic climate threshold, and predictions used model extrapolation, with associated statistical uncertainty. Therefore, predictions should be considered with care and more research effort is required to test clones over wider environmental conditions.
Keywords
Forest Tree Breeding, ClimateDT, Universal Response Function, B4EST
Introduction
Poplar (Populus spp.) clones form one of the main resources for productive plantation forestry worldwide, with thousands of hectares planted for plywood, roundwood or biomass ([41], [3]). Specialized poplar cultivation has for decades contributed to the development of important economic and productive sectors such as paper, wood-based plywood and furniture, by providing high-quality raw materials. This production has been supported by the identification and deployment of new genotype clonal selection ([25]). Besides the ability to remove CO2 from the atmosphere the use of poplar timber for long-lasting wood-based products is an important carbon store. Long-lived products for furnishing and construction are obtained from specialized poplar plantations grown at a spacing layout of 6×6 meters and with a rotation period of 10-12 years ([47]). This planting arrangement additionally contributes ecosystem services benefits to society, such as climate change mitigation and environmental and landscape improvement in rural areas. Poplar trees are cultivated globally with approximately 31.4 million hectares. The largest plantations occur in Canada and China, followed by Europe with France and Italy providing the main areas of plantation. In Italy, about 43,400 hectares are located in Northern Italy in the Po valley ([11]).
Historically, specialized poplar plantations in Northern Italy have continued to be grown due to the cultural dependence of expert knowledge and tradition. The production of poplar wood has a particular importance in the internal wood-furniture and paper sector. However, it does not cover the demand from industry, which is increasingly interested in optimizing supplies through an in-depth knowledge of the areas of growth and regional cultivation. In Italy an inventory of poplar crops has now been completed ([11]) but the likely future impact of climate change on cultivation and production is unknown. To date there are no results from ecological studies to evaluate the influence of environmental drivers on poplar clones in Italy.
Spatial modelling of forest tree species niche suitability provides a useful technique to support forest management strategies and conservation planning ([42], [6]). Among the range of tools and algorithms currently available, several conceptual approaches have been developed. The first and oldest group is represented by Species Distribution Modelling techniques (SDM), where the spatial distribution of a target species is considered as a proxy of its realised niche ([14], [33]). While SDM techniques have been used widely across time and space ([24], [43]), their shortcomings have been recognised, such as the need to account for phenotypic plasticity and genetic adaptation through phenotyping traits in genetic trials ([44]). Actually the huge genetic variability and phenotypic plasticity of tree species is an important resource for species performance across ecological gradients ([30]), observed using common garden experiments and reciprocal transplanting activities. Tree breeders and forest ecologists have developed models to account for genetic components such as: response functions ([32], [46], [8]), transfer functions ([4], [19]), reaction norms ([15]) and ΔTrait-SDM ([2]). The latter models (ΔTrait-SDM) has demonstrated an improved approach to disaggregate the genetic components influencing traits of forest tree species measured in common garden genetic trials and is able to evaluate the genetic adaptation (or variation) and the phenotypic plasticity of a phenotype in a single model.
The modelling framework for a sustainable and cost-efficient cultivation of polar clones in this study is inspired by the approach forest geneticists now adopt to analyse and summarize the performance of different provenances across ecological gradients ([21], [37]). In this paper we propose such a modelling framework for hybrid poplar clones in Italy. Data between 1980 and 2021 for 49 poplar clones widely tested in Italy were here clustered in two groups and single-group reaction norms were calculated to predict the potential productivity across time and space.
Materials and methods
Species Distribution Model (SDM) terminology and techniques have a clear conceptual scheme and usage. However, there is no clear boundary between Response Functions (RF), Transfer Functions (TF) and Reaction Norms (RN) since all of them deal with the variability of a measured trait across an ecological gradient. In addition, RF and TF have been often pooled across planting sites using more sophisticated techniques (e.g., mixed-effects models), and the term “Universal” added to the name of the approach. To be clear, in this study, we use the following semantic structure:
- Response Function (RF): the genetic expression of a single genotype across the range of ecological distance from the climate of origin;
- Transfer Function (TF): the genetic expression of a single genotype across the range of ecological distance from the climate of origin using a spatial coordinate system predictor;
- Reaction Norm (RN): the pattern of phenotypic plasticity of a single genotype across a range of environments;
- Universal Response Function (URF), Universal Transfer Function (UTF) and Universal Reaction Norm (URN): models where the response/reaction of different genotypes or provenances is pooled in a unique model using various mathematical techniques.
According to this hierarchical structure, the URN concept will be used for the models developed in this study, since no climate of origin can be derived for clones, no spatial predictors were used, and genotypes (i.e., poplar clones) were pooled into groups.
Clonal materials and experimental network
The dataset used consists of 49 poplar clones from various poplar species tested across Italy, though cultivation and production is more abundant in Northern Italy. Clones in our study cover almost all the historical breeding effort including old and experimental clones, and we grouped these into two main clusters based on characteristics that include: years of selection (starting from 1929 to present), expected production ability, pest and disease resistance. The names of these two groups were First GeNeration clones (FGN) and IMProved clones (IMP), the latter include the newest Italian MSA (Maggiore Sostenibilità Ambientale or Higher Environmental Sustainability) clones. All the tested clones and their groups are reported in the online supplementary material (Tab. S1).
The experimental network is geographically focused on Northern Italy, mainly across the Piedmont, Lombardy, Veneto and Emilia-Romagna regions, but also including some trials in Central Italy and the Mediterranean region (Tab. 1).
Tab. 1 - List of trials included in this study and main average climatic parameters occurred over the growing period of clones (10 years). The variables bio1 and bio12 represent the mean annual temperature (°C) and the mean total annual precipitation (mm), respectively, while AHM (annual heat moisture index, °C m-1), bio4 (temperature seasonality, sd °C·100) and MSP (mean summer precipitation, mm) are the variables we used for modelling.
Site name | Establ. (year) |
Long E |
Lat N |
Elev (m) |
bio1 | bio12 | AHM | bio4 | MSP |
---|---|---|---|---|---|---|---|---|---|
Azienda Cesurni | 1990 | 12.72377 | 41.94512 | 53 | 16.4 | 681.8 | 38.8 | 649.7 | 207.6 |
Azienda Ovile | 1990 | 12.3556 | 41.90792 | 43 | 16.5 | 708.8 | 37.4 | 635.7 | 188.7 |
Azienda Scottine | 1990 | 9.48700 | 45.06267 | 55 | 14.3 | 814.0 | 29.8 | 752.8 | 326.0 |
Azienda Volpares | 1985 | 13.09427 | 45.79843 | 7 | 13.9 | 1176.2 | 20.3 | 737.6 | 511.7 |
Brisighella | 1982 | 11.76317 | 44.21714 | 106 | 14.1 | 730.1 | 33.0 | 745.5 | 280.8 |
Carpaneta | 2007 | 10.88056 | 45.17917 | 24 | 14.9 | 732.8 | 34.0 | 761.9 | 317.9 |
Castiglion d Pescaia | 1986 | 10.94816 | 42.82812 | 52 | 15.9 | 574.3 | 45.1 | 622.5 | 177.5 |
Cavagnolo | 2012 | 8.08833 | 45.16861 | 153 | 14.7 | 730.7 | 33.8 | 757.5 | 298.9 |
Cavarzere | 2007 | 11.97333 | 45.14 | 4 | 15.2 | 688.8 | 36.6 | 753.8 | 298.5 |
Ceva | 2000 | 8.0002 | 44.38667 | 445 | 13.0 | 602.3 | 38.2 | 691.2 | 229.6 |
Dronero | 2000 | 7.38583 | 44.45472 | 605 | 11.9 | 645.0 | 33.9 | 708.2 | 289.0 |
Feltre | 1982 | 11.93074 | 46.03544 | 338 | 11.5 | 930.5 | 23.1 | 742.3 | 464.4 |
Frassinello | 2004 | 8.40611 | 45.04611 | 142 | 14.3 | 634.8 | 38.2 | 758.3 | 264.0 |
Gabiano | 2003 | 8.20361 | 45.17389 | 143 | 14.2 | 661.6 | 36.5 | 779.0 | 284.9 |
Grassaga | 1987 | 12.59043 | 45.67981 | 1 | 14.1 | 1059.0 | 22.8 | 719.4 | 471.7 |
Istrana | 1984 | 12.08753 | 45.68105 | 37 | 13.8 | 935.6 | 25.4 | 744.5 | 430.9 |
Lorenzana | 1991 | 10.52623 | 43.53259 | 41 | 15.6 | 722.7 | 35.4 | 648.8 | 235.5 |
Marsicovetere | 1983 | 15.84118 | 40.34179 | 612 | 13.0 | 536.4 | 43.0 | 635.7 | 143.7 |
Mezzi | 1997 | 8.5125 | 45.13722 | 105 | 14.4 | 700.4 | 34.8 | 756.8 | 302.9 |
Mezzi-Baldo | 2013 | 8.50639 | 45.14056 | 106 | 15.1 | 701.3 | 35.8 | 761.1 | 261.4 |
Migliarino | 1991 | 10.30831 | 43.75409 | 1 | 15.8 | 778.1 | 33.1 | 660.6 | 241.1 |
MolinellaMarmorta | 1993 | 11.72237 | 44.61177 | 4 | 15.1 | 639.9 | 39.2 | 744.1 | 267.8 |
Mombello | 2006 | 8.28972 | 45.115 | 146 | 14.3 | 643.6 | 37.8 | 752.9 | 274.3 |
Monticello d’Alba | 1992 | 7.93583 | 44.71778 | 267 | 13.6 | 693.1 | 34.1 | 700.3 | 343.9 |
Piopp.Pisano | 2004 | 8.49944 | 45.09167 | 121 | 14.4 | 697.1 | 35.0 | 760.1 | 279.1 |
PoggioUgolino | 1993 | 11.29399 | 43.69585 | 205 | 14.5 | 746.4 | 32.8 | 701.5 | 254.1 |
Rosignano M.mo | 2000 | 8.42111 | 45.06694 | 151 | 14.0 | 692.9 | 34.6 | 760.5 | 295.1 |
San Basilio A.P. | 1979 | 12.18473 | 44.95184 | 1 | 14.2 | 619.0 | 39.1 | 750.8 | 280.4 |
Sant’Agata | 2004 | 11.20417 | 44.64694 | 17 | 15.1 | 669.4 | 37.5 | 766.5 | 249.3 |
Trino | 2003 | 8.30833 | 45.185 | 131 | 14.2 | 637.4 | 38.0 | 781.4 | 275.6 |
A total of 30 trials with different planting years (between 1980 and 2013) were analysed and clones were irregularly distributed across a wide range of climate (Fig. 1). All the trials and data were characterised by a standardised cultivation scheme maintained across time and space.
Fig. 1 - Ecological distribution of the 30 trials considering the two main climatic parameters (AHM for aridity and bio4 for continentality) and abundance of tested clones across considered sites.
Two-year-old cuttings were always used as starting material and established with 36 m2 of space available for each tree (spacing of 6×6 meters) with a final harvest at the age of 10 or sometimes 12. The cultivation trials followed the standard model for poplar growth with small differences due to the layout of each farm, and irrigation. Irrigation is a common practice in poplar cultivation but not all the trials were irrigated due to pedo-climatic conditions, or the lack of irrigation infrastructure. For this reason, irrigation was added into the model as a dummy variable.
According to the wide range of measurements and ages across the dataset (Fig. 2) a large degree of variability between trials was observed. Given that the main product from poplar cultivation is harvested at age 10 and considering that most of the plots were presenting data for this age, a common age of 10 years after planting was used as the standard measurement for growth of poplar clones, avoiding extensive extrapolation across the dataset.
In this work a sigmoid function was used to interpolate the single-clone average diameter at breast height (DBH) at age 10 for trials where this record was missing. Single-clone average DBH was the simple arithmetic mean of the measured trees and among all the possible sigmoid functions available in literature we selected the Gompertz function ([35]) whose equation was as follows (eqn. 1):
where k, a and b are the parameters to be estimated and x is the age of the tree. Among the large family of sigmoid functions this model was selected given its ability to be asymmetric on the cartesian axes (i.e., the inflection point is not forced to centre of the range of y values).
Modelling framework: climatic characterizations and predictors
A reaction norm captures the phenotypic expression of a trait for a single genotype across a range of environments. For every genotype, phenotypic trait, and environmental variable, a different reaction norm can be calculated. In forest science this kind of information is generally handled by means of a mixed modelling approach where fixed effects are selected climatic variables and the site variation is a random effect. However, the use of clones did not allow us to calculate an ecological distance (i.e., climate of the origin vs. climate of the trial), thus the two clonal groups were modelled separately using the clone as a random effect. Therefore, the growth of poplar clones belonging to the same group was modelled across sites and then merged statistically in the mixed-effects model using DBH at age 10 as the target trait.
Tailored climatic data for all the trials in Italy were derived from ClimateDT (⇒ https://ibbr.cnr.it//climate-dt/), a web portal where scale-free climatic data are provided freely at global level using CRU-TS data ([20]) for the historical period (1901-current year) and UKCP18 for the future period until 2098 ([26]). This system is an online version of the ClimateEU ([28]) and Climate NA ([45]) software and implements a scale-free dynamic downscaling, providing more than 100 climatic variables and indices for ecological analysis, spatial prediction and research purposes in general. ClimateDT was used twice in the study: firstly the historical climate for all the 30 trials was requested and 10-year averages were calculated to describe the climate of each trial between establishment and harvesting (i.e., when the diameter at age 10 was measured). Secondly, spatial layers for 30-year climate normal periods for the current climate (average 1991-2020) and three future scenarios (2030s: 2016-2045; 2040s: 2026-2055; and 2050s: 2036-2065) were calculated using two variants (numbers 01 and 21) from the perturbed physics ensemble (PPE - [26]) of UKCP18 scenarios RCP2.6 and RCP8.5 . These scenarios represent the range of representative concentration pathway (RCPs) scenarios available from the Fifth Assessment Report (AR5). We used ClimateDT to generate climate data rasters with a spatial resolution of 250 m for spatial prediction across time and space.
In this work, we followed the model format used by several previous studies ([4], [2], [19]) specifying a three-term second-order polynomial model with an interaction term to predict the target trait using climatic variables. Climate variables were selected to provide information about the variation among the test sites. These were: (i) the Annual Heat Moisture index (AHM), calculated as (eqn. 2):
where MAT is the Mean Annual Temperature (°C) and MAP is the Mean Annual Precipitation (mm); (ii) the bio4 variable (temperature seasonality) from WorldClim ([22]), calculated as (eqn. 3):
where Tavg is the average temperature of each month; (iii) the total precipitation from May to September (MSP).
The AHM and bio4 describe the relationship between poplar growth and a changing climate in a Mediterranean country (i.e., aridity and continentality respectively), and MSP was added to incorporate the water availability across the growing season. Therefore, the resulting mixed-effects model for each group was (eqn. 4):
where (rnd) denotes the random effect among trial sites and is mathematically represented by a different intercept for each clone. To avoid bias resulting from different scaling and aligning operations, spatial predictors were not mathematically modified or standardised. The central (mean) value and the standard deviation used for standardization was known to be different between the dataset and the rasters. Additionally, DBH data were log-transformed, a common procedure in spatial modelling and forest sciences in general ([2], Hallingbäck et al. 2021). Even if known to be able to generate biased coefficients in some circumstances ([27]) this transformation does not impact on variables importance and model predictions. All computations were done in the R environment ([38]) with the “lme4” package ([1]).
Results
The quality check on the interpolation of missing data (i.e., the DBH at age 10) was controlled removing the data when available (i.e., DBH measurements at age 10) and comparing the predicted versus the observed values. On average the interpolation process performed very well, with an average error of ± 0.44 cm (maximum error was ± 1.41 cm) which corresponds to an average relative absolute error of ± 1.62% (maximum: ± 4.53%). With the complete dataset the fitted mixed-effects models were able to account for 85.8% of the variance for the FGN clones and 84.4% for the IMP clones. A small amount of the total variance explained was attributed to random effects (3.4% for FGN and 2.9% for IMP) with the fixed effects able to explain 82.3% and 81.5% for FGN and IMP, respectively. Most of the variation was explained by the supply of irrigation, and this was larger than the effect of the FGN clones. When models were adjusted by this effect, the aridity predictor (AHM) was more influential than the continentality index (bio4), with a negative coefficient associated to the second-order term and resulting in a convex curve on the x-y axes. The bio4 predictor was slightly more influential for IMP than for FGN clones and, conversely, the MSP predictor showed a greater influence in the model fitted for the FGN clones. The estimated coefficients for each model are reported in Tab. 2and the relative effect of each predictor is shown in Fig. 3. The concavity of the curves from bio4 demonstrates higher productivity at the extremes of the environmental sites tested while the relationship with MSP was linear with a very large slope for both models. However, the large confidence intervals prevented direct comparison between the two URNs calculated. We performed a cross-validation procedure to evaluate and fit the URNs (75% training - 25% testing repeated 10.000 times) and this showed very low prediction errors, with a mean absolute error of ± 2.2 cm and a mean relative absolute error of ± 8.5%.
Tab. 2 - Results of the model fitting. FGN model: R2 = 0.858, Fx = 0.823, Rn = 0.034; IMP model: R2 = 0.843, Fx = 0.815, Rn = 0.029.
Predictor | FGN model | IMP model | ||||||
---|---|---|---|---|---|---|---|---|
Estimate | Std. Error |
Pr(>|t|) | Sig. | Estimate | Std. Error |
Pr(>|t|) | Sig. | |
Intercept | 1778 | 301.4 | 6.81E-07 | *** | 1859 | 320.9 | 1.33E-07 | *** |
AHM | -11.170 | 7.655 | 0.152876 | - | -8.061 | 8.299 | 0.334252 | - |
I(AHM2) | -0.162 | 0.042 | 0.000463 | *** | -0.147 | 0.048 | 0.00318 | ** |
bio4 | -4.451 | 0.572 | 1.71E-09 | *** | -4.996 | 0.549 | 5.39E-14 | *** |
I(bio42) | 0.002 | 0.000 | <0.000001 | *** | 0.003 | 0.000 | 6.03E-15 | *** |
MSP | -2.322 | 1.299 | 0.082127 | - | -1.971 | 0.854 | 0.023575 | * |
WATER | 4.755 | 0.857 | <0.000001 | *** | 2.242 | 0.614 | 0.000477 | *** |
AHM:bio4 | 0.035 | 0.010 | 0.001235 | ** | 0.028 | 0.008 | 0.000401 | *** |
AHM:MSP | 0.063 | 0.035 | 0.075775 | . | 0.065 | 0.027 | 0.018987 | * |
bio4:MSP | 0.004 | 0.002 | 0.045837 | * | 0.003 | 0.001 | 0.006201 | ** |
AHM:bio4:MSP | -9.896E-05 | 0.000048 | 0.046503 | * | -9.795E-05 | 0.00004 | 0.007153 | ** |
Fig. 3 - Statistical relationship between the measured diameter at breast height (DBH) at age 10 (y-axis, cm) and AHM, bio4 and MSP in the fitted models. The curves for each parameter were generated maintaining the two others on median values.
To show the ecological potential of poplar clones across time and space, the spatial predictions across the whole of Italy were generated removing the irrigation effect. For the current climate (1991-2020 normal period - Fig. 4) the analysis accurately located the “productive core” of poplar cultivation in Italy in the North and across the Po valley for both groups of clones. This region is where most poplar plantations are located and where timber is harvested. However, additional areas were highlighted in the North-West and parts of Central and Southern Italy. The area predicted by the FGN and IMP models were in agreement showing the same spatial pattern, and predicted that IMP clones were (on average) more productive than FGN clones. The comparison in spatial predictions showed that FGN clones were in places more productive than the most recent improved clones (Fig. 5).
Fig. 4 - Expected diameter at breast height (in cm) of poplar clones at age 10 without irrigation under the current 30-years normal climate (1991-2020). The experimental sites used for modelling the group are shown as black dots and the statistical extrapolation outside the investigated ecological domain is shown as shaded area.
Fig. 5 - Predicted difference in diameter at breast height (in cm) at age 10 between poplar FGN and IMP clones across Italy. Positive values indicate that IMP clones are more productive than FGN clones and vice-versa. Values expresses the delta of DBH in centimetres.
When URNs were used to predict the growth and suitable area into the future an uncertain scenario was observed. While projections across RCPs and time-slices were almost in agreement with the expectations of a decrease in land suitability which was delayed for the RCP2.6 scenario compared to the RCP8.5 scenario, a regular trend across time (i.e., 2030s-2040s-2050s) in both RCPs due to a warmer and drier climate was forecast, with a very large difference between variants. Currently this difference was much larger than that observed between RCPs or between time slices. An example is reported in Fig. 6where the difference between the two variants in the 2040s for RCP8.5 is proposed.
Fig. 6 - Predicted DBH (cm) at age 10 for the two groups of poplar clones in 2040s under RCP8.5 using the variant01 (up) and variant21 (down). The experimental sites used for modelling the group are shown as black dots and the statistical extrapolation outside the investigated ecological domain is shown as shaded area.
Discussion
The URNs we developed showed the possible effects of a changing climate on poplar clone cultivation in Italy. Overall, the improved clonal material, which include MSA material (Higher Environmental Sustainability clones), showed less sensitivity to climatic aridity and a lower effect on growth from irrigation. These results may suggest a possible reduction of cultivation inputs as well as a more stable production of wood assortments in the future than with the current standard clonal types. In addition, the resistance to biotic stress that MSA clones achieve marks them as an important candidate source of reproductive material for poplar cultivation in the future. Adding to the traditional and widely cultivated clones, such as the well-known “I-214” and here included into the FGN group, new clones selected in recent years show a predicted growth rate comparable with “I-214” and better in places. However, several issues remain unclear and must be investigated in greater depth.
Growth rates, carbon sequestration and water supply in a changing climate
In recent decades, due to projected climate change scenarios, the research on hybrid poplar clones has received a strong impulse on fast growing genotypes, drought-resistant and resistant to pathogens ([40], [34]). In this scenario the breeding activity and clonal selection of new genotypes is aimed mainly at clones with high production capacity associated with a high resistance to the main diseases of poplar (e.g., rust - Melampsoralarici-populina; leaf spot - Marssonina brunnea). Our results showed that IMP clones may be a valuable FRM resource opportunity for the future. In addition to showing some resistance to all the main diseases of poplar trees, improved (IMP) clones show a growth improvement of up to 15% in volume, compared with the traditional (FGN) clones, and allowing for anticipated harvesting by 1 or 2 years earlier.
As fast-growing tree species, poplars are able to absorb high amounts of CO2 and to sequester carbon in wood used industrially for durable products with a long life-time (plywood, furniture, etc.). It has been calculated that a traditional poplar stand (as considered in this study) of 278 plants per hectare can sequester about 25 t ha-1 y-1 of CO2 ([9]) and that there is additionally a positive carbon storage in the soil, comparing agricultural annual crops and poplar stands ([48]). In addition, a future increase in atmospheric CO2, linked to the greenhouse effect, has been predicted to positively affect the growth and carbon storage of poplar ([18]). Within this framework our results suggest that IMP clones may be an excellent candidate material to store CO2 in timber and soil in a changing climate with less water availability and warmer temperatures, represented by the AHM term in the URN mixed effects model, due to greater water use efficiency compared with traditional clones. In addition to the research and selection of more adaptable clones, innovative and sustainable cultivation techniques would allow optimization of waste water use derived from urban or agricultural activities ([13]), or the innovative cultural models of agroforestry that allows irrigation application simultaneously for forest and food-feed crops.
Soil effect on spatial predictions: the need of expert-knowledge during trials establishment
Undoubtedly, soil physical and chemical properties such as depth and fertility are important components to consider when modelling growth. However, currently there are no high-quality, high-resolution data layers that can be used for such model improvements, thus preventing its inclusion for range-wide modelling purposes. The growth of Populus spp. is particularly linked to soil properties and the lack of soil predictors in this study may leave the door open to questions concerning the real suitability of poplar clones across the spatial extent projected by the URN models. Poplar clones are demanding of soil physical and chemical properties, needing a well-structured, fertile silty-loam soil for successful and profitable cultivation ([47], [23]). Soil physical properties (i.e., micro and macropores in the soil) may influence the water availability across time and space and suggest the need for irrigation, despite rainfall occurring during the growing season. Further research should focus on including soil properties for the models, particularly for available water capacity and cation exchange capacity. While high-quality trial-level data were available for this study, only low resolution spatial layers were available and found unsuitable for modelling. This shortcoming relies on the fact that while climate can be interpolated to obtain spatial layers from meteo-stations by means of different methods ([12], [16], [20]), such techniques are much less reliable for soil properties, given the lower sampling and the lack of spatial autocorrelation or external drift. Despite the omission of soil in the model, the climatic suitability of clonal material can provide an initial spatial focus for poplar trials in the future, with the detail of soil properties assessed as part of the potential site assessment. This study therefore provides an important initial filter to locate potential areas in regions where clonal material can be tested. In addition, soil properties that are essential for poplar cultivation can be written into a decision support tool that delivers the climatic suitability of specific clones in a region ([39]).
Modelling uncertainties in spatial and temporal extrapolation: the need for a larger network of trials
Our analysis investigated the whole life-span of poplar cultivation and not just the early establishment stage of tree growth, which is less common in the forestry sector ([31]). To evaluate the quality and productivity of forest material, provenances, clones and other reproductive material, it is best to test the material in common garden trials and compare traits with older material, used as a reference threshold. There is much literature concerning tree breeding activities, models and the climatic shift of tree species niches across time and space ([5], [2]) and local material is not always the best choice under climate change projections ([7], [29]). Beyond any modelling activities, however, large ecological domains must be investigated to derive reliable data on growth performance of forest tree species. The dataset we used in this work can be considered a good sample of a wide spectrum of climates across Italy. With an observed ecological range of 4.97 °C in mean annual temperature (between 11.5 °C at Feltre site and 16.5 °C at Az. Ovile site) and a range in total annual precipitation of 639.8 mm (max: 1176 mm - Az. Volpares site; min: 536 mm - Marsicovetere site), our dataset can investigate a large ecological domain, resulting in a good predictive potential of URNs able to project the growth of poplars into the future. However, the comparison between the two clonal groups and the proposed models must be considered with respect to the large confidence intervals for each model parameter. This was mainly due to a large degree of variation within each clonal group we proposed, and by modelling single-clone RNs may allow us to reduce the variation, as would modelling larger datasets across wider environmental gradients. Both the range, and the distribution of data are important issues when assessing the results from statistical models. In this study a shortcoming of our database was the uneven distribution of clones across the sites (partially solved by the grouping) as well as the asymmetric distribution of the trials, both geographically and environmentally. Most of the sites are clustered in the Po valley and very few sites are at the leading and trailing edge of the ecological range. Low AHM and low bio4 values were not investigated at all (e.g., AHM<30 and bio4<700) and neither were drier conditions (e.g., AHM>40) which play a key role for future ecological range of the data (i.e., the climate of maximum or minimum growth) which also affects the use of the models for future scenarios with a rapid change of growth with climate variables. This requires an enlargement of the network that includes old and new clones to add to historical data, and predicting the growth of the same clones in the same sites but under past climatic conditions ([36]).
The need for high-quality data must not shade the requirement of reliable future projections. Climatologists and atmospheric scientists are improving future scenarios with new GCM and RCM models, and climate projections based on international COP agreements. Indeed, new frameworks and new knowledge and scenarios will soon be delivered under the CMIP6 ([17], [10]). Unfortunately, cross validation on future projections cannot be performed: therefore ecological modellers must rely on conceptual schemes and use multiple outputs to manage uncertainties ([19]). In this work, more focused on reaction norms on historical and current climate than on future scenarios, we have shown a partial example of how difficult future predictions can be. The use of the two different UKCP18 variants (i.e., the standard UKCP18 - variant01, and the least extreme projection according to ClimateDT - variant21) produced completely diverging scenarios after the 2030s. In addition, the projection made with variant01 was mostly from extrapolation, with high uncertainty. For these reasons, much more effort is needed to support decision makers with additional data. Further, researchers must test genotypes outside the “expected” ecological niche. In this framework we provide spatial projections for the current time (i.e., 1991-2020 climatic normal period) and the 2030s to represent a valuable guideline to plan the future establishment of additional trials using the same materials to be tested outside the environment we have observed in this work. Furthermore, it is important to point out that future scenarios are highly uncertain, not only due to the wide range of possible climate conditions but also because the future dynamics of pests and diseases are not easily predictable. However, in this scenario the use of MSA clones may fix this problem, given their ability to resist to the two main biotic threats (rusts and aphids).
Conclusions
High quality timber assortments and wood products in general are important to society and provide long-term methods of sequestering carbon. Durable products and fast-growing trees are efficient at reducing the amount of CO2 in the air and mitigate the emissions. This is much more evident when compared to bioenergy and the use of biomass in general, where the total amount of carbon components is maintained at stable level and not sequestered for long periods. Poplars are among the most important forest tree species used in agroforestry systems and we have provided URNs to show the improvement that can be made to poplar cultivation under climate change scenarios. However, the need for future assessments is demanding, and additional efforts should be made to improve the dataset (and predictions) we have developed. Different reaction norms could be expected in the future from more extensive experimental trials, showing a more stable production of clones across the ecological gradients.
Acknowledgements
This research work was developed in the framework of WP1 and WP5 of the Horizon 2020 B4EST project “Adaptive BREEDING for productive, sustainable and resilient FORESTs under climate change”, UE Grant Agreement 773383.
References
Gscholar
CrossRef | Gscholar
CrossRef | Gscholar
CrossRef | Gscholar
Gscholar
Gscholar
Supplementary Material
Authors’ Info
Authors’ Affiliation
CNR, Institute of Biosciences and BioResources - IBBR, Division of Florence, v. Madonna del Piano 10, I-50019 Sesto Fiorentino, FI (Italy)
Roberto Barbetti 0000-0003-0885-3158
Gianni Facciotto 0000-0001-9550-0806
Pier Mario Chiarabaglio 0000-0002-3610-5395
Giuseppe Nervo 0000-0002-8282-7110
CREA - Research Centre for Forestry and Wood, s.da Frassineto 35, I-15033 Casale Monferrato, AL (Italy)
Centre for Ecosystems Society and Biosecurity, Forest Research, Roslin, EH25 9SY Scotland (UK)
Natural Resources Institute Finland - LUKE, Latokartanonkaari 9, FI-00790 Helsinki (Finland)
Corresponding author
Paper Info
Citation
Marchi M, Bergante S, Ray D, Barbetti R, Facciotto G, Chiarabaglio PM, Hynynen J, Nervo G (2022). Universal reaction norms for the sustainable cultivation of hybrid poplar clones under climate change in Italy. iForest 15: 47-55. - doi: 10.3832/ifor3989-015
Academic Editor
Gianfranco Minotta
Paper history
Received: Oct 04, 2021
Accepted: Jan 12, 2022
First online: Feb 08, 2022
Publication Date: Feb 28, 2022
Publication Time: 0.90 months
Copyright Information
© SISEF - The Italian Society of Silviculture and Forest Ecology 2022
Open Access
This article is distributed under the terms of the Creative Commons Attribution-Non Commercial 4.0 International (https://creativecommons.org/licenses/by-nc/4.0/), which permits unrestricted use, distribution, and reproduction in any medium, provided you give appropriate credit to the original author(s) and the source, provide a link to the Creative Commons license, and indicate if changes were made.
Web Metrics
Breakdown by View Type
Article Usage
Total Article Views: 26698
(from publication date up to now)
Breakdown by View Type
HTML Page Views: 23610
Abstract Page Views: 1661
PDF Downloads: 1154
Citation/Reference Downloads: 5
XML Downloads: 268
Web Metrics
Days since publication: 1017
Overall contacts: 26698
Avg. contacts per week: 183.76
Article Citations
Article citations are based on data periodically collected from the Clarivate Web of Science web site
(last update: Feb 2023)
Total number of cites (since 2022): 3
Average cites per year: 1.50
Publication Metrics
by Dimensions ©
Articles citing this article
List of the papers citing this article based on CrossRef Cited-by.
Related Contents
iForest Similar Articles
Review Papers
Prospects for evolution in European tree breeding
vol. 17, pp. 45-58 (online: 06 March 2024)
Research Articles
Properties and prediction accuracy of a sigmoid function of time-determinate growth
vol. 8, pp. 631-637 (online: 13 January 2015)
Research Articles
Local ecological niche modelling to provide suitability maps for 27 forest tree species in edge conditions
vol. 13, pp. 230-237 (online: 19 June 2020)
Research Articles
Spatio-temporal modelling of forest monitoring data: modelling German tree defoliation data collected between 1989 and 2015 for trend estimation and survey grid examination using GAMMs
vol. 12, pp. 338-348 (online: 05 July 2019)
Research Articles
Distribution factors of the epiphytic lichen Lobaria pulmonaria (L.) Hoffm. at local and regional spatial scales in the Caucasus: combining species distribution modelling and ecological niche theory
vol. 17, pp. 120-131 (online: 30 April 2024)
Research Articles
Adaptive response of Pinus monticola driven by positive selection upon resistance gene analogs (RGAs) of the TIR-NBS-LRR subfamily
vol. 10, pp. 237-241 (online: 02 February 2017)
Review Papers
Breeding and improvement of black locust (Robinia pseudoacacia L.) with a special focus on Hungary: a review
vol. 16, pp. 290-298 (online: 28 October 2023)
Research Articles
Modeling the fine root biomass dispersion using a special influence function
vol. 1, pp. 141-144 (online: 25 November 2008)
Short Communications
Dynamic modelling of target loads of acidifying deposition for forest ecosystems in Flanders (Belgium)
vol. 2, pp. 30-33 (online: 21 January 2009)
Research Articles
Modelling the carbon budget of intensive forest monitoring sites in Germany using the simulation model BIOME-BGC
vol. 2, pp. 7-10 (online: 21 January 2009)
iForest Database Search
Search By Author
Search By Keyword
Google Scholar Search
Citing Articles
Search By Author
Search By Keywords
PubMed Search
Search By Author
Search By Keyword