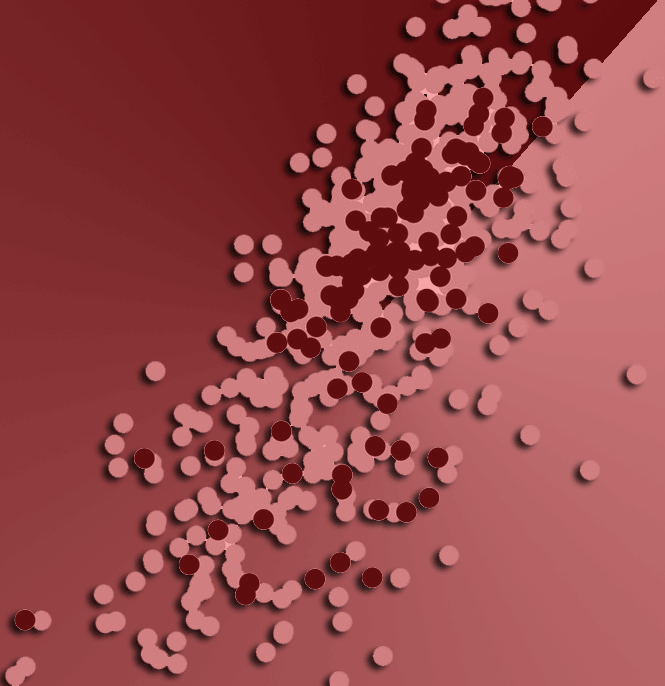
Validation of visual and machine strength grading for Italian beech with additional sampling
iForest - Biogeosciences and Forestry, Volume 14, Issue 3, Pages 260-267 (2021)
doi: https://doi.org/10.3832/ifor3649-014
Published: May 29, 2021 - Copyright © 2021 SISEF
Research Articles
Collection/Special Issue: Research Project PRIN-MIUR 2015
The forest-wood value chain: biomass supply, traceability, C-footprint. Innovation for bioarchitecture and energy efficiency
Guest Editors: Scarascia Mugnozza G, Maesano M, Romagnoli M
Abstract
The typical variability of wood properties, higher than for other construction materials, requires a thorough knowledge of its mechanical performance. To make the use of wooden structural products safe and at the same time efficient, a proven methodology for the selection of the raw material is also crucial. As a general rule, the initial sampling is of vital importance to develop effective strength grading processes, but it is rarely verified with additional samples, besides those already included during the development of the grading procedure itself. Here, a new source of sawn timber was collected to verify the original sampling and to validate the visual and machine strength grading early developed for Italian beech. The new pieces were graded, destructively tested and the characteristic values of the graded material were calculated. The dispersion of the new data was found to be entirely included in that of the original data, proving an effective sampling of the resource variability. The correlations between properties were very similar comparing the original and the new sample, with the exception of the correlations between the dynamic modulus of elasticity and both bending strength and stiffness, which were lower in the new sample. The characteristic values of the graded new material were generally higher than the characteristics values of the corresponding strength classes as tabled in the technical standard. Only for one grade in the machine grading the strength reached 98.9% the class value, anyhow above the 90% required by the procedure of the checking of existing settings in a particular location, as provided by the related standard. In conclusion, the earlier development of the strength grading of Italian beech confirmed to be correct and safe.
Keywords
Hardwood, Visual Grading, Machine Grading, Structural Timber
Introduction
Valuable efforts are made to create local timber supply chains. The reason for that is found in the target of sustainable forest management and in the intent to make the best use of raw materials favouring a multi-purpose use. Several studies for the utilization of hardwoods in the timber structural sector move in this direction, also aided by the excellent mechanical properties of the material and the increasing presence of species such as beech, chestnut, ash and oak in the European forests ([1]). In particular, beech (Fagus sylvatica L.) proved a great potential both in the use as solid timber and especially in the production of glued structural products ([28], [25], [2], [24], [12], [13], [14], [31]).
To make the use of wooden structural products safe but at the same time efficient two key aspects should be investigated: (i) the knowledge of the raw material; (ii) the bonding of the sawn timber for production of engineered wood products (both process parameters and quality control).
As regards the second point, past studies analysed several facets of the gluing of beech wood for the production of laminated beams and structural panels ([3], [37], [41], [2], [32], [30], [6]), but further steps are needed, especially from the point of view of the technical standardization. On the other hand, the typical variability of wood certainly requires a thorough knowledge of its mechanical properties, but also a proven methodology for the selection of the material ([40]). This is because, if the use of beech in the structural field aims at products of high mechanical performance, the raw material needs to achieve the quality requirements of the market ([43], [45]). In this sense, the implementation of efficient visual rules and/or machine settings for strength grading the material is extremely important both for the economic sustainability of the nascent market and to guarantee the safety of the structures.
In the recent past, steps forward have been done in the description of the mechanical properties of beech and their prediction by non-destructive methods ([7], [9], [10], [11], [23], [39], [44], [45], [4]). The next step is the development of visual or machine strength grading. At time, only visual grading rules have been approved by the European standardization system for beech: the German national standard ([8]) allows the assignment of the best grade (named LS13) to the strength class D40 (the strength classes for hardwoods, named D, are described in the European standard EN 338 2016) and the second grade (LS10 & better) to D35 ([19]); in France a new visual grading rule has been published [33]) providing two grading combinations, each with two visual grades assigned to the strength classes D40-D24 (combination 1) or D35-D18 (combination 2 - [31]).
In Italy, the properties of beech timber were investigated to develop visual grading rules and machine grading settings ([5]). Four visual rules were established using two different knot parameters with limitations aimed at either maximize the yields in the high strength classes or minimize the rejects. The visual rules grouped the raw material in two qualitative grades, assigned to the strength class combinations D45-D24 or D45-D18. In the same work, settings for machine grading were derived using one of the most commonly measured property to grade timber by machine, already verified for the grading of sweet chestnut hardwood ([36]): the dynamic modulus of elasticity.
As a general rule, for the efficient and suitable grading of the timber resource to be exploited, the initial sampling is of vital importance: it needs to cover the variability of the raw material to the best of the knowledge and represent it correctly. But rarely sampling and grading systems are verified with additional samples, besides those already included during the development of the grading procedure itself.
In the study of Brunetti et al. ([5]), beech timber was collected from four different sources along north, centre and south of Italy, including high and low quality material. The aim of the present study is the verification of the original sampling and the rules/settings early developed for the grading of Italian beech, by applying those to a new sample from a different source not used for the development of the grading rules/settings themselves.
Materials and methods
Sampling
The material tested in the study came from the Foresta Umbra in the Gargano area (southern Italy), from land owned by the Puglia Region (regional forest property). The Gargano beech forests are the Italian beech populations that extend to the lowest altitudes in the country. They are the beech forests most directly in contact with the thermos-xeric Mediterranean vegetation and those closest to the coastline of the entire peninsula.
The sampling was carried out as part of a project aimed at enhancing the production of timber and the creation of an efficient and multipurpose forest-wood supply chain. Fig. 1 shows the location of the Gargano sample and the four sources studied by Brunetti et al. ([5]) that formed the original national sample. These four are referred to “Italy” from now on and will be compared to the Gargano sample.
Fig. 1 - Location of the four provenances of the Italy sample used in Brunetti et al. ([5]), North-West (NW), North-East (NE), Central (C) and South (S), and of the new sample coming from Gargano area. In grey the distribution map of beech (from EUFORGEN ⇒ http://www.euforgen.org/, modified).
The felled trees had a diameter at breast height ranging from 45 to 120 cm and were 30-50 m tall. Logs were cut 4.2 m long and processed in the sawmill for the production of 120 boards with three different cross sections: 20 × 100 mm2, 40 × 110 mm2, 50 × 140 mm2. No special requests were made to the sawmill, so it can be assumed a normal cutting pattern was used.
Visual and machine grading and laboratory tests
Each board was examined in relation to its visual and machine grading characteristics. Afterwards they were destructively tested to determine their physical and mechanical properties and the grading was verified by the calculation of the characteristic values of the several grades obtained.
By visual inspection, the strength-reducing characteristics, such as knots, slope of grain, ring width and the presence of pith included in the cross section were measured in the third destructively tested (see the description of the test below). The position of each knot, as well as the minimum diameter and its projection on the side of the board were registered. This allowed to calculate two parameters: the ratio of the minimum diameter of the knot to the dimension of the board side where it is measured (Dm/S) and the ratio of the sum of the knot projections on all the board sides to the double of the board width (sPr/2W). For each board, the highest value of both knot parameters considered alternatively in the visual rules developed in the previous work ([5]) was selected for further data analysis concerning knots.
The slope of grain was measured as the general inclination of the wood fibres to the longitudinal axis of the timber piece. The measurement was made on a length of one metre following the fissures if present or by means of a swivel handle scribe, and expressed as a percentage ([18]). Local fibre deviations, for example close to knots, were ignored.
The presence of the pith included in the cross section was also registered and the average ring width was measured on the transversal section of the piece along the longest straight line normal to the growth rings ([18]).
After the visual inspection, the natural frequency of vibration in the longitudinal direction was measured for each specimen by means of the ViSCAN® portable grading machine (MiCROTEC, Bressanone, Italy). The piece was placed on two supports and a percussion provided the excitation necessary to cause vibration which natural frequency was measured by a non-contact laser interferometer.
The weight and dimensions of each piece were also measured and the dynamic modulus of elasticity was calculated by the following formula (eqn. 1):
where f is the natural frequency of vibration, L the length of the timber piece and ρ is the density, calculated by the timber weight divided by its volume.
After the non-destructive measurements, four point edgewise bending tests were carried out as described in the European standard EN-408 ([17]). The total span was 18 times the nominal depth and the shear span was 6 times the nominal depth. The local deformation was measured in the neutral axis on both sides of the timber piece and the mean of the two measures was used to calculate the local modulus (Em,l - eqn. 2). In the same test setup, the total deformation was measured in the central point on the tension edge of the beam and used to calculate the global modulus of elasticity (Em,g - eqn. 3). Then the load was applied until failure and the bending strength (fm) was computed (eqn. 4):
where ΔF is the applied load increment, Fmax is the load at failure, l is the length between the two supports, b is the thickness of timber piece, h is the width, a is the distance between the load point and the nearest support, l1 is the central gauge length, Δwlocal and Δwglobal are the deformation increments.
After the destructive testing, density and moisture content were determined using the over dry method in accordance with ISO-13061-2 ([29]) and EN-13183-1 ([20]) respectively, cutting a small specimen of full cross section from each timber piece. Density and modulus of elasticity values were corrected to a moisture content of u = 12% according to the adjustment equations given in EN-384 ([16]).
Measured values of bending strength were adjusted to a reference depth (h) of 150 mm using the kh = (150/h)0.2 factor calculated in accordance with EN-384 ([16]), since the pieces had depth less than 150 mm and the characteristic density was less than 700 kg m-3 (657 kg m-3).
Data analysis
Descriptive statistics were obtained for the mechanical properties, and the parameters measured visually and by the machine were calculated. The relationships of several properties were then analysed by linear regression.
The visual strength reducing characteristics (i.e., the single knot parameters and the presence of the pith) were used to assign each piece of timber to a grade according to the rules, named visual-1 and visual-2, as previously reported ([5]). Similarly, the machine settings calculated for the strength class combinations D45/D24 and D45/D18 (the same classes achievable by the visual grading rules) were applied to the new sample.
The validation of the grading was then performed calculating the characteristic values of strength (5th percentile), modulus of elasticity (mean) and density (5th percentile) according to the standard EN-14358 ([22]) for the pieces assigned to each strength class. The results were then compared to the characteristic values of the corresponding classes reported in the standard EN-338 ([15]). For the calculation of the characteristic values the local modulus of elasticity was used.
Results and discussions
Representativeness of sampling
The results of the non-destructive and destructive tests are reported in Tab. 1, Tab. 2and in Fig. 2. Among the three cross sections of the Gargano sample, the smallest (20 × 100 mm2) had the highest bending strength, but slightly lower modulus of elasticity and density than the other two cross sections. However, the only significant difference (after comparison of means by Tukey HSD test) was in density, which was lower in the smaller pieces.
Tab. 1 - Mean values and coefficient of variation (in brackets) of physical and mechanical properties as the results of destructive tests and machine measurements; for strength and density the 5th percentile values are also reported (with 05 as subscript). (§): Data from Brunetti et al. ([5]).
Property | Symbol | Unit | Gargano | Italy § | |||
---|---|---|---|---|---|---|---|
Cross section (mm2) | All | ||||||
25×100 | 40×110 | 50×140 | |||||
No. pieces | - | - | 40 | 50 | 30 | 120 | 465 |
Bending strength | fm,mean | MPa | 73.6 (17.6) | 66.2 (28.6) | 69.8 (27.6) | 69.6 (25.0) | 69.4 (34.6) |
fm,05 | MPa | 30.7 | 28.8 | 28.8 | 30.7 | 26.6 | |
Global MoE | Em,g | GPa | 12.4 (12.1) | 13.0 (10.1) | 14.0 (8.9) | 13.1 (11.3) | 12.8 (18.7) |
Local MoE | Em,l | GPa | 13.1 (16.2) | 13.4 (14.4) | 14.7 (12.0) | 13.6 (14.9) | 13.3 (22.2) |
Dynamic MoE | Edyn | GPa | 14.1 (8.9) | 13.8 (8.1) | 14.3 (7.7) | 14.0 (8.3) | 13.9 (14.5) |
Density | ρmean | kg m-3 | 687 (3.5) | 713 (4.1) | 723 (4.1) | 707 (4.4) | 716 (5.8) |
ρ05 | kg m-3 | 644 | 667 | 665 | 657 | 660 |
Tab. 2 - Mean values of visual properties. (§): Data from Brunetti et al. ([5]).
Property | Symbol | Unit | Gargano | Italy § | |||
---|---|---|---|---|---|---|---|
Cross Section (mm2) | all | ||||||
25×100 | 40×110 | 50×140 | |||||
Knottiness (n. of knots) | Kn | - | 0.05 | 0.50 | 0.80 | 0.43 | 1.54 |
Single knot | Dm/S | - | 0.05 | 0.19 | 0.25 | 0.16 | 0.30 |
sPr/2W | - | 0.02 | 0.12 | 0.13 | 0.09 | 0.17 | |
Slope of grain | SGv | % | 3.2 | 1.3 | 2.9 | 2.4 | 2.2 |
Ring width | W | mm | 2.3 | 2.2 | 2.3 | 2.3 | 2.9 |
Pith (boards with pith) | P | % | 0.0 | 6.4 | 15.4 | 5.8 | 15.9 |
Fig. 2 - Relationship between the bending strength and the static modulus of elasticity for Gargano and Italy samples.
Overall, comparing the results of the Gargano source with the Italy sample, the physical and mechanical properties measured were found very similar on average (Tab. 1, Fig. 2); no significant difference was observed after mean comparison by Student’s t-test. Moreover, Fig. 2shows a general overlap of the strength and stiffness data. This confirms that the mechanical and physical properties of the new sample were covered by the variability included in the Italy sample. Similar physical and mechanical properties were reported for Italian beech grown in central Italy ([7]).
The variability of data was lower in the Gargano sample than in the national one (see CVs in Tab. 1). This is explained by the smaller amount of pieces tested in the verification sample and by the presence in the Italy sample of lower quality material (NE provenance in [5]), which is characterized by higher variability. Similar results are also reported in other studies ([45]).
Regarding the visual parameters (Tab. 2), in the Gargano sample the smallest boards had less knots and generally no pith included. This might be due either to the small size of the boards or to the fact that they have been cut from the outermost part of the logs. All the other characteristics were similar across sections.
Compared to the Italy sample, the Gargano sample showed on average less knottiness and a lower percentage of pieces with pith included. Possible explanations could be the relative higher number of boards with small cross section (a third of the whole), with no knots and no pith included and/or the different dimension of the original logs (smaller logs would need a larger number to be processed and an increasing number with pieces with pith included). Looking at the dimension of knots, the highest Dm/S and sPr/2W of each board were on average very small (0.16 and 0.09 - Tab. 2), but the statistic included also the boards without knots (knot parameter = 0). If only the boards with knots were considered, the mean knot dimensions were Dm/S = 0.45 and sPr/2W = 0.26, which is similar to that observed in Brunetti et al. ([5] - Dm/S = 0.43 and sPr/2W = 0.25 on average excluding boards without knots).
Slope of grain was comparable between new and previous sample (no significant differences after Student’s t-test).
Regarding the ring width, the Gargano sample had narrower rings than the Italy sample, but this did not correspond to a different density. Indeed, the ring width seems to be weakly correlated to wood density for beech ([5]).
The relationship between the non-destructive and destructive properties were analysed by linear regression and the coefficients of determination obtained are reported in Tab. 3. Bending strength correlated to the other properties (stiffness and knots parameters) similarly to what found out in the previous work for Italy ([5]). The most noticeably differences with the Italy sample were the relationships between the dynamic modulus of elasticity and the strength and static modulus: the coefficients of determination were 0.28 and 0.44 for strength and local modulus respectively, while in the Italy sample they were 0.47 and 0.71 respectively. Looking at the values of the coefficients of determination for the single sources of the Italy sample, timber coming from the south showed considerably lower values (0.30 and 0.53 with strength and static modulus, respectively) than the overall national sample, closer to the findings for Gargano. This might suggest specific characteristics of wood from southern latitudes, although there is not enough evidence to confirm it.
Tab. 3 - Coefficient of determination (R2) of the linear regression between several properties of the Gargano sample. Symbols are explained in Tab. 1and Tab. 2.
Property | f m | E m,g | E m,l | E dyn | ρ | Dm/S |
---|---|---|---|---|---|---|
E m,g | 0.42 | - | - | - | - | - |
E m,l | 0.48 | 0.82 | - | - | - | - |
E dyn | 0.28 | 0.53 | 0.44 | - | - | - |
ρ | 0.02 | 0.03 | 0.01 | 0.01 | - | - |
Dm/S | 0.54 | 0.16 | 0.33 | 0.12 | 0.07 | - |
sPr/2W | 0.51 | 0.19 | 0.35 | 0.16 | 0.12 | 0.80 |
Density did not correlate with the other properties (Tab. 3). As already stated in previous works ([34], [36], [7], [10], [44], [45]), density was confirmed to have little influence on strength and stiffness in beech wood, as well as in other hardwoods aimed to structural use.
The relationship of knot parameters with mechanical properties were comparable to what observed in the Italy sample. The coefficients of determination of the Gargano sample were included in the range of those observed for the different sources sampled in Italy ([5]) where the coefficient of determination of Dm/S with strength varied from 0.24 to 0.55, with stiffness from 0.14 to 0.41; sPr/2W with strength from 0.40 to 0.59 and with stiffness from 0.20 to 0.53. Similar values were also reported for other sources of beech wood ([27], [10]).
Finally, the slope of grain did not show any correlation neither with strength nor with modulus of elasticity. Here the general direction of the grain was considered and not the local deviation due to the presence of defects. Indeed the general direction was already reported as not correlated with the mechanical properties of beech ([7], [5]), while the local grain deviation could be an effective parameter to predict the mechanical performance, but it should be measured by specific instruments ([11], [38]).
Validation of visual grading rules and machine settings
Each board of the Gargano sample was assigned to a strength class applying both the visual rules and the machine settings previously developed ([5]). The main parameters and their limitations established for the visual rules, as well as the settings for the machine and the results of the grading simulations are reported in Tab. 4 (class combination D45-D24-R) and Tab. 5 (class combination D45-D18-R). In the visual grading rule named visual-1, the Dm/S parameter was used to measure knots and in the visual-2 sPr/2W was used. The number of pieces and the characteristic values of strength, stiffness and density were calculated and reported for each strength class. The yields in percentage are displayed in Fig. 3 for the grade combination D45-D24-R, applying both visual and machine grading.
Tab. 4 - Permissible limits of the strength reducing characteristics of the visual rules and machine setting for the grade combination D45-D24-R; number (number of rejects in round brackets), characteristic values and their ratio to the requirement (in square brackets) for the pieces assigned to each class for the Gargano sample. Symbols are explained in Tab. 1. (NP): Not Permitted; (P): Permitted.
Strength characteristics |
Visual-1 | Visual-2 | Machine | |||
---|---|---|---|---|---|---|
D45 | D24 | D45 | D24 | D45 | D24 | |
Edyn (GPa) | - | - | - | - | ≥ 14.2 | < 14.2 & ≥ 10.4 |
Knot parameter | Dm/S | Dm/S | sPr/2W | sPr/2W | - | - |
Knot limitation (-) | ≤0.2 | ≤0.7 | ≤0.2 | ≤0.4 | - | - |
Pith | NP | P | NP | P | - | - |
N (-) | 82 | 29 (9) | 90 | 23 (7) | 55 | 65 (0) |
fm,05 (MPa) | 62.1 [1.38] | 28.9 [1.20] | 59.5 [1.32] | 24.4 [1.02] | 44.5 [0.99] | 28.8 [1.20] |
Em,1 (GPa) | 14.2 [1.05] | 13.4 [1.34] | 14.2 [1.05] | 13.0 [1.30] | 14.7 [1.09] | 12.8 [1.28] |
ρ05 (kg m-3) | 644 [1.11] | 663 [1.37] | 646 [1.11] | 661 [1.36] | 672 [1.16] | 650 [1.37] |
Tab. 5 - Permissible limits of the strength reducing characteristics of the visual rules and machine setting for the grade combination D45-D18-R; number (no. of rejects in round brackets), characteristic values and their ratio to the requirement (in square brackets) for the pieces assigned to each class for the Gargano sample. Symbols are reported in Tab. 1. (NP): not permitted; (P): permitted.
Strength characteristics |
Visual-1 | Visual-2 | Machine | |||
---|---|---|---|---|---|---|
D45 | D18 | D45 | D18 | D45 | D18 | |
Edyn (GPa) | - | - | - | - | ≥ 14.2 | < 14.2 & ≥ 9.12 |
Knot parameter | Dm/S | Dm/S | sPr/2W | sPr/2W | - | - |
Knot limitation (-) | ≤0.2 | ≤0.8 | ≤0.2 | ≤0.7 | - | - |
Pith | NP | P | NP | P | - | - |
N (-) | 82 | 32 (6) | 90 | 30 (0) | 55 | 65 (0) |
fm,05 (MPa) | 62.1 [1.38] | 29.6 [1.64] | 59.5 [1.32] | 23.3 [1.29] | 44.5 [0.99] | 28.8 [1.60] |
Em,l (GPa) | 14.2 [1.05] | 13.3 [1.40] | 14.2 [1.05] | 12.1 [1.27] | 14.7 [1.09] | 12.8 [1.35] |
ρ05 (kg m-3) | 644 [1.11] | 663 [1.40] | 646 [1.11] | 661 [1.39] | 672 [1.16] | 650 [1.37] |
Fig. 3 - Yields (in percentage) for the two visual rules and the machine grading for the grade combination D45-D24-R (Gargano sample).
As first observation, the characteristic values of the several grades generally achieved the values of the strength classes tabled in the European standardization ([15]). In particular, regarding bending strength, which is often the limiting property for the assignment to a strength class ([35], [42], [26]), the visual grades showed a far higher characteristic strength for the highest class: 62.1 MPa and 59.5 MPa for visual-1 and visual-2 respectively, compared to the required value of the class of 45 Mpa, while the machine grading showed a slightly lower value (44.5 MPa). However, this was above the 90% of the class value (namely 98.9%), which is the requirement to be met in the second step of the verification during the development of the machine settings ([21]): each verification sample (part of a country in our case) should reach the 90% of the characteristic strength value of the class. This means that if the settings would have been calculated with all the five sources (the four of the previous study and the Gargano sample), the settings for the machine would have resulted the same and the characteristic values of strength for the Gargano sample would have been accepted. Moreover, the standard EN-14081-2 ([21]) provides also a procedure for the check of the existing settings in a particular location. To prove that the settings are correctly grading the timber coming from the specific location, the 90% of the strength characteristic values should be reached, and this requirement was met for the Gargano sample.
For the lower classes (D24 and D18) the characteristic strength was always higher than the requirements (much higher than the requirements for visual-1 which uses the Dm/S as knot parameter). In the same way, both density and modulus of elasticity showed characteristic values always higher than the requirements. Furthermore, the two class combinations (D45-D24-R and D45-D18-R) gave very similar results, both in terms of yields and characteristic values obtained for the several groupings (see D24 vs. D18). This could be expected because the pieces not included in D45 were graded to the lower grade or rejected (R), considering the relatively small number of pieces excluded from D45, the differences were not very large for the lower classes.
As regards the grading yields (Fig. 3), we found both some confirmations and minor discrepacies with regard to observations previously reported in the literature. First, machine grading minimized the number of rejects (none), as observed also for the national sample ([5]). Nevertheless, the visual rules also allowed for very low percentage of rejects (6-7% for the class combination D45-D24-R and 0-5% for the combination D45-D18). On the contrary, the yield in the highest class D45 was higher for the visual grading in respect to the machine. In our previous work ([5]), the yields between the two procedures were comparable and close to 50%. Indeed, the reduced knottiness of the Gargano boards allowed higher yields in the visual grading compared to the original study, and the weak correlations between the dynamic modulus of elasticity (the property used by the machine) and the mechanical properties of raw material likely led to a low efficient machine grading in terms of selecting the higher quality material.
The initial assignment of the visual grades to the strength classes D45-D24 and D45-D18 were penalized by the number of sub-samples during the development of the grading system; at least five sub-samples are required to avoid reduction in the characteristic values calculation ([16]), while originally only four sub-samples were available ([5]). Therefore further improvement of the visual rules could be possible, such as assignment of the grades to higher strength classes or more permissive limitation for the visual characteristics (higher values for the knot limitation in the highest grade).
A final comparison was done between the two knot parameters used in the visual rules (Fig. 4). The rule visual-2, based on the ratio sPr/2W, had better yields both in terms of number of pieces in the higher class and in terms of fewer rejects, and this is in agreement with our previous findings ([5]). In Fig. 4, the grey square highlights the pieces graded in an inefficient way (i.e., assigned to the second class but with a strength higher than 45 Mpa), and these were more numerous for Dm/S than for sPr/2W. On the other hand, the wrong graded pieces (i.e., knot parameter below 0.2 and therefore assigned to the best class, but with strength below 45 MPa) were more numerous for sPr/2W (3) than for Dm/S (1). Therefore, considering both the characteristic values obtained (much higher than the class requirements), and the assignments of the individual pieces (the inefficient and the unsafe ones), the visual-1 rule seems to be more efficient, though further improvement are needed by adjusting the limits of the visual parameters used.
Fig. 4 - Scatterplot of bending strength (Dm/S, panel a) and knot parameter (sPr/2W, panel b), for the Gargano sample. Horizontal line sets the characteristic strength of 45 MPa; vertical line sets the limit provided by the visual rules for the single knot parameter (0.2). The grey square highlight the pieces inefficiently graded.
Conclusions
A new sample of sawn timber was collected to validate the visual rules and the machine settings developed for the strength grading of the Italian beech in a previous work ([5]). A representative sampling is crucial for the success of the strength grading, which means accuracy, safety and efficiency of the raw material sorting.
The mechanical properties values as well as the visual and machine parameters of the new sample fell within the expected range of values derived from a previous work, confirming the effective sampling of the resource variability. The correlations between wood properties for the new sample were very similar to the previous sample, with the exception of the correlations between the dynamic modulus of elasticity and the mechanical characteristics (both strength and stiffness), suggesting peculiar characteristics of southern beech wood.
The weak correlations observed for the dynamic modulus of elasticity led to a poor efficiency of the machine settings previously developed in terms of yield and characteristic strength. Nonetheless, the latter parameter was higher (98.9%) than the standard threshold required for the correct grading of timber of a particular provenance.
As previously reported, the number of rejects was lower for machine grading than for visual rules. However, visual grading of wood sample resulted in very few rejects, high yields in the best class and characteristic strength higher than required, thus showing to be more efficient than machine grading in this regards, likely thanks to the few knots present in the verification sample.
In conclusion, the former setup of the strength grading of Italian beech was confirmed to be correct and accurate. Nonetheless, further improvements could be undertaken, e.g., by adding new samples to the national one, thereby allowing either the achievement of higher strength classes or more permissive limitations for the visual characteristics (better yields). For the machine grading, a combination of several parameters would increase its efficiency. Knots and local grain deviation demonstrated to be important parameters to take into account. Both the above characteristics could be combined with the dynamic modulus of elasticity to implement the machine grading of the beech raw material.
Acknowledgments
The national sampling and the development of the strength grading of Italian beech were realized in the framework of the PRIN2015 project (no. 2015YW8JWA) “Short chain in the biomass-wood sector: supply, traceability, certification and carbon sequestration. Innovations for bio-building and energy efficiency”, coordinated by G. Scarascia-Mugnozza. The verification sample was studied under the Program Agreement “For the implementation of a pilot project for the activation of a forest - wood - energy supply chain through the management, protection and enhancement of forest ecosystems - Gargano Area” among the Gargano Land Reclamation Authority, the Puglia Region, the Regional Agency for Irrigation and Forestry Activities (ARIF) and the Gargano National Park Authority. Finally, many thanks to the sawmill “Cooperativa Castagno” and the personnel who helped during the practical work.
References
Gscholar
CrossRef | Gscholar
Gscholar
Gscholar
Gscholar
Gscholar
CrossRef | Gscholar
Gscholar
Gscholar
Gscholar
Gscholar
Gscholar
Gscholar
Gscholar
Gscholar
Gscholar
Gscholar
Gscholar
Gscholar
Gscholar
Gscholar
Gscholar
Gscholar
CrossRef | Gscholar
Gscholar
Gscholar
Gscholar
Authors’ Info
Authors’ Affiliation
Giovanni Aminti 0000-0003-2262-4095
Michela Nocetti 0000-0003-0906-0483
CNR-IBE, Sesto Fiorentino (FI), 50019 (Italy)
Corresponding author
Paper Info
Citation
Brunetti M, Aminti G, Nocetti M, Russo G (2021). Validation of visual and machine strength grading for Italian beech with additional sampling. iForest 14: 260-267. - doi: 10.3832/ifor3649-014
Academic Editor
Rodolfo Picchio
Paper history
Received: Sep 07, 2020
Accepted: Mar 02, 2021
First online: May 29, 2021
Publication Date: Jun 30, 2021
Publication Time: 2.93 months
Copyright Information
© SISEF - The Italian Society of Silviculture and Forest Ecology 2021
Open Access
This article is distributed under the terms of the Creative Commons Attribution-Non Commercial 4.0 International (https://creativecommons.org/licenses/by-nc/4.0/), which permits unrestricted use, distribution, and reproduction in any medium, provided you give appropriate credit to the original author(s) and the source, provide a link to the Creative Commons license, and indicate if changes were made.
Web Metrics
Breakdown by View Type
Article Usage
Total Article Views: 31622
(from publication date up to now)
Breakdown by View Type
HTML Page Views: 27787
Abstract Page Views: 1604
PDF Downloads: 1751
Citation/Reference Downloads: 9
XML Downloads: 471
Web Metrics
Days since publication: 1494
Overall contacts: 31622
Avg. contacts per week: 148.16
Article Citations
Article citations are based on data periodically collected from the Clarivate Web of Science web site
(last update: Mar 2025)
Total number of cites (since 2021): 6
Average cites per year: 1.20
Publication Metrics
by Dimensions ©
Articles citing this article
List of the papers citing this article based on CrossRef Cited-by.
Related Contents
iForest Similar Articles
Research Articles
Deploying an early-stage Cyber-Physical System for the implementation of Forestry 4.0 in a New Zealand timber harvesting context
vol. 17, pp. 353-359 (online: 13 November 2024)
Research Articles
Density, extractives and decay resistance variabilities within branch wood from four agroforestry hardwood species
vol. 14, pp. 212-220 (online: 02 May 2021)
Research Articles
Modifying harvesting time as a tool to reduce nutrient export by timber extraction: a case study in planted teak (Tectona grandis L.f.) forests in Costa Rica
vol. 9, pp. 729-735 (online: 03 June 2016)
Research Articles
Shear modulus of old timber
vol. 10, pp. 446-450 (online: 24 March 2017)
Research Articles
Characterization of VOC emission profile of different wood species during moisture cycles
vol. 10, pp. 576-584 (online: 08 May 2017)
Research Articles
Comparison of timber-house technologies and initiatives supporting use of timber in Slovenia and in Sweden - the state of the art
vol. 10, pp. 930-938 (online: 07 December 2017)
Research Articles
Identification of wood from the Amazon by characteristics of Haralick and Neural Network: image segmentation and polishing of the surface
vol. 15, pp. 234-239 (online: 14 July 2022)
Research Articles
Fuel consumption comparison of two forwarders in lowland forests of pedunculate oak
vol. 12, pp. 125-131 (online: 11 February 2019)
Research Articles
Comparison of alternative harvesting systems for selective thinning in a Mediterranean pine afforestation (Pinus halepensis Mill.) for bioenergy use
vol. 14, pp. 465-472 (online: 16 October 2021)
Research Articles
NIR-based models for estimating selected physical and chemical wood properties from fast-growing plantations
vol. 15, pp. 372-380 (online: 05 October 2022)
iForest Database Search
Search By Author
Search By Keyword
Google Scholar Search
Citing Articles
Search By Author
Search By Keywords
PubMed Search
Search By Author
Search By Keyword