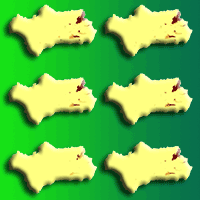
Climate change may threaten the southernmost Pinus nigra subsp. salzmannii (Dunal) Franco populations: an ensemble niche-based approach
iForest - Biogeosciences and Forestry, Volume 11, Issue 3, Pages 396-405 (2018)
doi: https://doi.org/10.3832/ifor2588-011
Published: May 15, 2018 - Copyright © 2018 SISEF
Research Articles
Abstract
We used Species Distribution Modeling to predict the probability of Iberian pine (Pinus nigra subsp. salzmannii [Dunal] Franco) occurrences in southern Spain in response to environmental variables and to forecast the effects of climate change on their predicted geographical distribution. The ensemble modeling approach “biomod2” was used, together with present Iberian pine data, to predict the current potential distribution based on bioclimatic explanatory variables (200 m resolution) and to forecast future suitability by studying three periods (2040, 2070, and 2100), considering the Global Circulation Models BCM2, CNCM3, and ECHAM5, and the regional model EGMAM, for different scenarios (SRAB1, SRA2, SRB1). Model evaluation was performed using Kappa, True Skills Statistic (TSS), and Area Under the Curve (AUC) values. The biomod2 approach highlighted the average number of days with a minimum temperature equal to or below 0°C, annual precipitation, and aridity index as the most important variables to describe the P. nigra occurrence probability. Model performances were generally satisfactory and the highest AUC values and high stability of the results were given by GAM and GLM, but MaxEnt and the SRE model were scarcely accurate according to all our statistics. The ensemble Species Distribution Modeling of P. nigra in Andalusia predicted the highest probability of species occurrence in the eastern areas, Sierra de Cazorla being the area with the highest occurrence of P. nigra in Andalusia. In the future habitat, the general probability of P. nigra occurrence in Andalusia will decrease widely; the species is expected to lose habitat suitability at moderate altitudes and its occurrence probability will have decreased by nearly 70% on average by 2100, affected by the selected scenario. Populations in Sierra de Cazorla are those most suitable for P. nigra growth, even under the most pessimistic scenarios. It is likely that the natural southern populations of P. nigra will be very sensitive to changes in climate.
Keywords
Species Distribution Modeling, Climate Change, Ensemble Modeling, Iberian Pine, Mediterranean Relict Forests
Introduction
Tree species distribution is mainly determined by climate and soil (i.e., ecological drivers), despite the effect of historical factors such as the presence of glacial refuges ([43]) and the naturalization of species beyond their former range ([36]). Projected trends in the context of global change suggest an increase in the mean annual temperatures, drought, and frequency of extreme events in the Mediterranean Basin. Understanding the effects of climate on tree distribution is particularly relevant in relict drought-prone forests; for example, those of southern Iberia ([7]), which are considered to be highly exposed to climate change, in which both warming and aridification trends have been observed ([19]). These recent trends had negative effects on the tree growth ([40]), forest productivity ([27]), and distribution of the southernmost Iberian coniferous forests ([7]). An improved understanding of the responses to climate of the distribution of relict circum-Mediterranean pine populations provides a valuable system for investigating the role played by ongoing warming at the southern distribution limit of these species, where they usually face severe drought stress.
The Iberian pine (Pinus nigra Arn. subsp. salzmannii [Dunal] Franco - hereafter abbreviated as P. nigra subsp. salzmannii) is widely distributed in the western Mediterranean area, ranging from the large populations in the eastern Pyrenees Mountains to the southernmost isolated populations in southeastern Spain and northern Morocco. At the southernmost edge of their distribution, Iberian pine populations are fragmented and located in the high mountains of the Baetic Range ([8]), which makes them especially vulnerable to climate change effects ([8], [32]). In Andalusia (in the south of the Iberian Peninsula), native high-mountain tree species like P. nigra subsp. salzmannii used to cover large areas ([13]). However, as a result of historical forest harvesting, pruning, and grazing over hundreds of years, most of the natural stands are heavily degraded, remaining as isolated relict populations ([13]).
Nowadays, forest harvesting has been reduced but the main risk faced by these populations is climate change. Previous studies have shown that black pine is sensitive to an increase in temperature and decrease in precipitation ([40], [32]). In this area, the forecasted climate trends indicate a notable reduction in annual precipitation (3-20%), an increase in mean temperature (0.3-1.5 °C per decade), and an increase in the frequency, duration, and intensity of droughts.
Species Distribution Modeling (SDM) forecasts the probability of species occurrences in response to environmental variables, and could predict the future habitat suitability of species under climate change scenarios ([14]). However, SDM faces several methodological issues that may affect the prediction of the above habitat suitability - such as the choice of the model’s algorithm ([29]), the selection of environmental variables ([30]) or background points used for the model’s calibration, and the conversion of occurrence probabilities into presence and absence estimates ([48]). However, careful implementations of SDM have demonstrated the usefulness of the models with regard to predicting the habitat suitability of a given species. Moreover, multiple studies have compared the accuracy and performance of such models for Mediterranean tree species ([4], [31], [3], [6], [28]), although no superiority of any single one has been proved ([29]). Araújo & New ([2]) underlined the benefit of ensemble models over single models. The former combine the results of several independent models, which surpass the influence of a single algorithm in model predictions, and this is expected to balance the accuracy and robustness of the models ([51]). Many studies using SDM have been carried out in the Iberian Peninsula ([18], [26]), and a few have modeled the changes in habitat suitability of P. nigra subsp. salzmannii and its future distribution in the Iberian Peninsula ([15], [26]), but the use of ensemble SDM approaches might improve the accuracy of biodiversity conservation and management policies in relict forests ([35]). For P. nigra subsp. salzmannii, it is at the edge of its distribution and in the highest mountains where we can expect the strongest response to purely climatic conditions. Likewise, the relict P. nigra subsp. salzmannii populations at the southernmost edge of the current distribution represent an important genetic reservoir for the future conservation of the species under increasing drought and aridity ([42]). The genetic characteristics of P. nigra subsp. salzmannii might help to conserve the species at higher latitudes, even in areas where it already occurs but is not genetically adapted to the forecasted change in climate conditions ([21], [26]). Moreover, in situ conservation and biodiversity management require information on plant species distribution at a local scale ([49]).
The modeling of the distribution of endangered species, like P. nigra subsp. salzmannii, is especially challenging because of the lack of data and the high level of human disturbance in some of the populations ([14]). In this paper, we have used ensemble SDM of habitat to explore current and future impacts of climate change on relict populations of Iberian pine in the southern Iberian Peninsula. We used the biomod2 R-package ([46]), which combines 10 different model algorithms and calculates a final ensemble prediction. We aimed to identify the major environmental variables explaining black pine climate suitability in the southernmost Iberian forests, and then to explore the potential responses of the species to predicted changes in climate using three intercontinental Global Circulation Models and one regional model, for three different scenarios, at a very fine scale (200 meters resolution). In addition, we discuss whether the factors driving the occurrence of this tree species in the southern Iberian Peninsula are similar to those reported previously for other Iberian relict regions.
Material and methods
Study areas
The study areas are located in Andalusia, southern Spain, a characteristic region of the Mediterranean Basin located in the south of the Iberian Peninsula (Fig. S1 in Supplementary material). The climate in this region is typically Mediterranean, with hot and dry summers and precipitation concentrated in spring and autumn. Forests of P. nigra subsp. salzmannii are located in the highest forested range of the Baetic mountains, characterized by great topographical variability and a high degree of natural disturbances, including wild fires and, often, non-sustainable forest management. Andalusia contains most of the natural stands of Iberian pine in its southernmost populations, with the exception of those located in Morocco and Algeria ([8]). The natural P. nigra subsp. salzmannii forests in eastern Andalusia are dominant in north-oriented and high-elevation sites (1500-2270 m a.s.l.) and occupy ca. 107.000 ha. There are also extensive plantations (ca. 40.000 ha) of P. nigra subsp. salzmannii, which were mostly planted in mid to high-elevation sites (1200-2150 m a.s.l.) between the 1960s and 1970s. We did not consider these plantations in this study because they may be established outside their bioclimatic range ([26]).
Location data and environmental variables
Information on species occurrences and bioclimatic variables was compiled from the Andalusian Regional Government database ([39]). We used 977 presence records (points) of natural P. nigra subsp. salzmannii forests from the third National Spanish Forest Inventory ([50]). We selected the presence records within natural populations because natural P. nigra subsp. salzmannii stands presented a more plastic response to climate change and more severe decline have been associated to planted trees ([41]), which suggest that some of P. nigra subsp. salzmannii plantations might be outside of its bioclimatic range. An equal number of pseudo-absences (Prevalence equal to 0.50) with 10 replicates ([5]) within the study area were generated using the biomod2 R package ([46]).
The climate data consisted of 29 environmental variables (Tab. S1 in Supplementary material) averaged for the period 1960-2000 and the future predictions were carried out for three periods: 2011-2040; 2041-2070, and 2071-2099, following regional scenarios ([39]). For each period, we considered three intercontinental Global Circulation Models (BCM2, CNCM3, and ECHAM5) and one regional model (EGMAM), developed in the project Climate Change Scenarios updated in the 4th IPCC report, as well as three climate change scenarios (A1B, A2, and B1 - [22]), to take into account a high variability in future projections. The complete dataset had a spatial resolution of 200-meter grids. In all our analyses, we used average period values.
Variable reduction and importance
The initial 29 environmental variables were reduced by the variable inflation factor (VIF<10 - [37]), principal component analysis (PCA), and the relative quality of the statistical models measured by Akaike’s Information Criterion (AIC) values. We took the non-collinear variables as the means of the first two principal components ([16]), and from those we selected the final variables based on their importance in predicting the climate suitability, as judged by AIC.
Variable importance was quantified using the Variable Importance function within biomod2, which is implemented by calculating the Pearson’s correlation (r) between model predictions including all remaining variables (a “full model”) and predictions excluding the variable being tested (a “reduced model”). If a variable has a low contribution to a model, the two outputs would be similar, and (1-r) would be low, while the opposite would be the case for important variables ([46]). This variable importance procedure does not allow the comparison of importance between models. Therefore, we used the ranking system described in Syphard & Franklin ([44]) to compare variable selection between model types.
Statistical models
To deal with model selection, we used ensemble models from 10 SDM algorithms ([46]), employing the biomod2 package ([38]). This package allows one to perform ensemble SDM using presence-absence data that includes: Generalized Linear Models (GLM), Generalized Additive Models (GAM), Classification and Regression Trees (CART), Flexible Discriminate Analysis (FDA), Artificial Neural Networks (ANN), Multivariate Adaptive Regression Splines (MARS), Maximum Entropy Modelling (MaxEnt), Random Forest (RF) and Boosted Regression Trees (BRT) and one similar to BIOCLIM, Surface Range Envelop (SRE).
We used ensemble models calculated using the mean, median, coefficient of variation, upper and lower confidence interval, committee averaging and probability mean weight decay of the single model predictions ([46]).
The consensus methods employed in this study make up a representative sample of the most commonly used techniques. Four methods (Median, Mean, Coefficient of Variation, and Confidence Intervals) are based on their statistical functions, whereas the Probability Mean Weight Decay (WD) and Committee Averaging (CA) methods preselect the single models based on certain predefined criteria. In WD, half of the single-model outputs are preselected on the basis of the True Skills Statistic (TSS) values. The selected single models are combined using an averaging function. In CA the probabilities of the selected models are first converted into binary, according to the maximal TSS threshold, and the CA score is calculated as the average of the binary predictions ([46]).
Model calibration and validation
We randomly divided our dataset into two subsets, which we used to train the model (70% of the data) and to evaluate it independently (the remaining 30%). This yielded 100 different fits per model, each with its own accuracy indicator, mean, and quantiles of model accuracy calculated. Models with higher mean values and smaller variations were considered as being the most accurate ones ([12]).
Model performance was evaluated by the AUC of the Receiver Operating Characteristic plot, Kappa, and TSS. The AUC represents the models’ discriminative capacity with regard to the data and is obtained by plotting the commission error (1-specificity; false positives) on the horizontal axis, versus the omission error (sensitivity; actual positives which are correctly identified as such) on the vertical axis, for numerous thresholds. The AUC ranges between 0 (= opposite prediction) and 1 (= perfect prediction) with 0.5 = random, where 1 represents a perfect discrimination between presence and absence and 0.5 represents a random fit. As an evaluation metric, AUC has the advantage of being prevalence and threshold independent ([16]).
Cohen’s Kappa (κ) was derived from the 2 × 2 confusion matrix to measure the rate of agreement between actual and predicted values in the spatial space for categorical Kappa values; however, the matrix depends on the defined threshold for presence. Values of κ near to 0.5 indicate no discrimination capacity (random agreement), whereas a value of 1 represents the perfect discrimination model ([10]).
The TSS is concerned with omission and commission errors and is also prevalence independent. It ranges from -1 to +1, where +1 indicates perfect agreement and <0 indicates a random performance. The TSS measures the difference between the actual agreement and the randomly expected agreement; it is particularly useful for the modeling of rare species with limited point locations and it can be used to compare different modeling techniques. The TSS is defined as ([1] - eqn. 1):
Finally, Kappa and TSS were calculated and evaluated, considering a threshold equal to prevalence ([48]).
Distribution maps
We generated continuous probabilistic maps with values of between 0 and 1 for each grid point, for the present and future habitat distributions of P. nigra subsp. salzmannii in Andalusia. In order to assist the visual interpretation of the model predictions, the probability values were classified into four categories (0-25%; 25-50%; 50-75%; 75-100%). To calculate the shifts in the range of the surface area from the present to the future distributions, we reclassified the probabilities as 0 (unsuitable habitat) and 1 (suitable habitat); the methods used to estimate the threshold were the same as those employed to calculate TSS and Kappa. Finally, we focused on six different relict areas (Andalusia, Sierra Cazorla, Sierra María, Sierra de Baza, Sierra Nevada, and Sierra de las Nieves - Fig. 1) to evaluate the effect of climate change on each population.
Fig. 1 - Response curve of the ensemble model Committee Averaging for the explanatory variables: average reference evapotranspiration (ETO), aridity index (IAR), average number of days with a minimum temperature equal to or below 0 °C (NDF), annual precipitation (PRE), annual sum of the positive differences between precipitation and reference evapotranspiration (SSUP), and average snow precipitation (SNOW).
Results
Variable reduction
The results of the variable selection highlighted that 17 predictors out of 29 were affected by collinearity problems (VIF<10), of which eight were selected with high correlations with the first two principal components of the PCA and six were highlighted by AIC model selection. The final set of the six variables selected included the: average reference evapotranspiration (ETO); aridity index (IAR); average number of days with a minimum temperature equal to or below 0 °C (NDF); annual precipitation (PRC); annual sum of the positive differences between precipitation and reference evapotranspiration (SSUP); and average snow precipitation (SNOW - Tab. 1). Moreover, the biomod2 ranked classification of variable importance (Tab. 2) highlighted NDF as the most important variable, followed by PRC and IAR. The single scorings of variable importance from the separate model techniques generally agreed with this classification, reinforcing our variable selection (Tab. 2).
Tab. 1 - Variable importance ranking and parametric characterization of P. nigra subsp. salzmannii for 1000 runs and 10 habitat prediction model techniques. Variable abbreviation is indicated in parentheses. (Qtl): quartile.
Rank | Variable | Min | 1st Qtl | Mean | 3rd Qtl | Max | Units |
---|---|---|---|---|---|---|---|
1 | Average number of days with a minimum temperature equal to or below 0 °C (NDF) | 35.30 | 65.40 | 76.30 | 90.00 | 158.90 | days |
2 | Annual precipitation (PRC) | 306.00 | 558.00 | 760.00 | 919.00 | 1513.00 | mm |
3 | Aridity index (IAR) | 35.00 | 94.00 | 116.00 | 160.00 | 296.00 | - |
4 | Annual sum of the positive differences between precipitation and reference evapotranspiration (SSUP) | 1.00 | 142.00 | 312.00 | 453.00 | 1046.00 | mm |
5 | Average reference evapotranspiration (ETO) | 92.00 | 840.00 | 891.00 | 934.00 | 1087.00 | mm |
6 | Average snow precipitation (SNOW) | 27.00 | 134.00 | 266.00 | 446.00 | 1170.00 | mm |
- | Aspect (ASPECT) | -453.00 | -27.00 | 8.00 | 49.00 | 333.00 | degree |
- | Sum of water balances at the end of each month (BH) | 1.00 | 685.00 | 1894.00 | 3011.00 | 7707.00 | mm |
- | Digital elevation model (DEM) | 7.00 | 1164.00 | 1423.00 | 1705.00 | 2995.00 | m |
- | Average net primary production (DF) | 182.00 | 1223.00 | 1928.00 | 2471.00 | 3371.00 | hours |
- | Average number of days with a maximum temperature equal to or above 35 °C (NDC) | 0.10 | 5.70 | 10.20 | 16.20 | 44.40 | days |
- | Annual radiation (RN) | 26.00 | 95.00 | 103.00 | 107.00 | 126.00 | Julian m-2 |
- | Annual sum of the negative differences between precipitation and reference evapotranspiration (SDEF) | 84.00 | 394.00 | 440.00 | 506.00 | 657.00 | mm |
- | Slope (SLOPE) | 1.00 | 11.00 | 17.00 | 24.00 | 100.00 | % |
- | Average maximum temperature (T_MAX) | 12.20 | 16.60 | 17.60 | 18.60 | 22.20 | °C |
- | Average mean temperature (T_MED) | 4.10 | 10.20 | 11.20 | 12.20 | 15.60 | °C |
- | Average minimum temperature (T_MIN) | -4.50 | 3.70 | 5.00 | 6.10 | 9.50 | °C |
- | Maximum of the monthly average maximum temperatures (TMAXC) | 24.40 | 28.80 | 30.00 | 31.10 | 34.70 | °C |
- | Average maximum temperature of all months (TMC) | 14.30 | 20.20 | 21.20 | 22.50 | 26.00 | °C |
- | Average minimum temperature of all months (TMF) | -1.80 | 3.10 | 4.00 | 4.90 | 8.50 | °C |
- | Minimum of the monthly average minimum temperatures (TMINF) | -8.40 | -2.10 | -1.00 | -0.10 | 3.30 | °C |
Tab. 2 - Mean variable importance values for 100 runs, for each selected variable and the habitat prediction model techniques. Generalized Linear Models (GLM), Generalized Additive Models (GAM), Classification and Regression Trees (CART), Flexible Discriminate Analysis (FDA), Artificial Neural Networks (ANN), Multivariate Adaptive Regression Splines (MARS), Maximum Entropy Modelling (MaxEnt), Random Forest (RF) and Boosted Regression Trees (BRT) and one similar to BIOCLIM, Surface Range Envelop (SRE). Variable description in Tab. 1. (*): P < 0.05; (**): P < 0.01.
Variable | ANN | BRT | CART | FDA | GAM | GLM | MARS | MaxEnt | RF | SRE |
---|---|---|---|---|---|---|---|---|---|---|
ETO | 0.19 | 0.02 | 0.03 | 0.06 | 0.30 | 0.18 | 0.28 | 0.01 | 0.03 | 0.31 |
IAR | 0.07 | 0.01 | 0.03 | 0.06 | 0.12 | 0.04 | 0.13 | 0.01 | 0.04 | 0.11 |
NDF | 0.73** | 0.83** | 0.90** | 0.96** | 0.67* | 0.82** | 0.73* | 0.75* | 0.58* | 0.57* |
PRC | 0.56* | 0.00 | 0.03 | 0.98** | 0.99** | 0.80** | 0.97** | 0.01 | 0.02 | 0.08 |
SNOW | 0.24 | 0.00 | 0.05 | 0.38 | 0.39 | 0.32 | 0.39 | 0.00 | 0.02 | 0.07 |
SSUP | 0.07 | 0.00 | 0.01 | 0.03 | 0.04 | 0.04 | 0.03 | 0.07 | 0.03 | 0.03 |
Our results show that the probability of P. nigra subsp. salzmannii occurrence increases with PRC and NDF, while it decreases with increases in ETO and SSUP. We also observed slight responses to aridity and snow cover (Fig. 1). Locations with low annual precipitation (PRC < 915 mm) or high transpiration losses (ETO > 908 mm or SSUP > 719 mm) were highly unsuitable for P. nigra subsp. salzmannii growth. Surprisingly, we observed a weak response to aridity; low aridity values maximized the occurrence probability but the response was otherwise rather flat. Similarly, snow cover did not significantly affect the occurrence probability (Fig. 1).
Model selection and validation
The single model predictions were compared by their accuracy as given by TSS, Kappa, and AUC; overall, these showed good model accuracy (Fig. 2). The model performances were generally satisfactory and the highest AUC values and lowest variances were shown by GAM and GLM, although they had lower TSS and Kappa values than ANN, BRT, and RF, which were also highly accurate. Although CART, FDA, and MARS possessed low predictive power and stability, they exhibited high (CART) or moderate (FDA and MARS) correlation with other models. However, the MaxEnt and SRE models were scarcely accurate according to all our statistics (Fig. 2). The bivariate Pearson correlation analysis highlighted the low correlation of the MaxEnt and SRE predictions with those of the rest of the model techniques used. On the other hand, it is interesting to note that the models, in general, presented higher correlations (Tab. 3). The classification (BRT, CART, and RF) and regression method (GAM and GLM) predictions were highly correlated with each other. Moreover, ANN, FDA, and MARS presented acceptable correlations with the rest of the models (Tab. 4).
Fig. 2 - Adjustment values obtained for 10 different distribution models in Andalusia, including Artificial Neural Networks (ANN), Boosted Regression Trees (BRT), Classification and Regression Tress (CART), Flexible Discriminate Analysis (FDA), Generalized Additive Models (GAM), Generalized Linear Models (GLM), Multivariate Adaptive Regression Splines (MARS), Maximum Entropy (MaxEnt), Random Forest (RF), and Surface Range Envelop (SRE).
Tab. 3 - Pearson’s correlation matrix of the concordance of the different spatial distribution models analyzed: Generalized Linear Models (GLM), Generalized Additive Models (GAM), Classification and Regression Trees (CART), Flexible Discriminate Analysis (FDA), Artificial Neural Networks (ANN), Multivariate Adaptive Regression Splines (MARS), Maximum Entropy Modelling (MaxEnt), Random Forest (RF) and Boosted Regression Trees (BRT) and one similar to BIOCLIM, Surface Range Envelop (SRE). All correlation were significant with p<0.001 (2-tailed).
Model | ANN | BRT | CART | FDA | GAM | GLM | MARS | MaxEnt | RF | SRE |
---|---|---|---|---|---|---|---|---|---|---|
ANN | 1.000 | - | - | - | - | - | - | - | - | - |
BRT | 0.885 | 1.000 | - | - | - | - | - | - | - | - |
CART | 0.843 | 0.966 | 1.000 | - | - | - | - | - | - | - |
FDA | 0.890 | 0.888 | 0.865 | 1.000 | - | - | - | - | - | - |
GAM | 0.920 | 0.832 | 0.782 | 0.856 | 1.000 | - | - | - | - | - |
GLM | 0.891 | 0.878 | 0.841 | 0.862 | 0.971 | 1.000 | - | - | - | - |
MARS | 0.831 | 0.876 | 0.828 | 0.844 | 0.873 | 0.875 | 1.000 | - | - | - |
MaxEnt | 0.821 | 0.881 | 0.856 | 0.751 | 0.762 | 0.799 | 0.872 | 1.000 | - | - |
RF | 0.921 | 0.950 | 0.912 | 0.867 | 0.851 | 0.889 | 0.889 | 0.801 | 1.000 | - |
SRE | 0.603 | 0.666 | 0.651 | 0.623 | 0.588 | 0.601 | 0.620 | 0.685 | 0.642 | 1.000 |
Tab. 4 - Adjustment values obtained with the ensemble models of Pinus nigra habitat prediction in Andalusia: Cohen’s Kappa, Area Under the Curve (AUC), True Skills Statistic (TSS), sensitivity (true positive rate), and specificity (true negative rate).
Ensemble model | Kappa | TSS | AUC | Sensitivity | Specificity | Threshold |
---|---|---|---|---|---|---|
Mean | 0.786 | 0.904 | 0.986 | 0.9725 | 0.9313 | 0.869 |
Lower Confident interval | 0.786 | 0.904 | 0.986 | 0.9714 | 0.9332 | 0.846 |
Upper Confident interval | 0.784 | 0.904 | 0.986 | 0.9735 | 0.9311 | 0.881 |
Median | 0.774 | 0.903 | 0.983 | 0.9724 | 0.9307 | 0.913 |
Committee averaging | 0.802 | 0.905 | 0.987 | 0.9756 | 0.9298 | 0.800 |
Probability mean weight decay | 0.786 | 0.904 | 0.986 | 0.9725 | 0.9319 | 0.806 |
All ensemble models presented TSS>0.77, Kappa> 0.90, and AUC> 0.98, the ensemble models committee averaging (CA) and probability mean weight decay (WD) being the best ones (Tab. 4). In this table, we have excluded the results corresponding to the ensemble models in accordance with the coefficient of variance result appearing in Fig. 3, with variability between all model predictions of below 25%, which means that, mostly, all the models agree with the predictions. Pixels with a high coefficient of variance are recognized as being the uncertain pixels predicted.
Fig. 3 - Present probability of occurrence of P. nigra subsp. salzmannii in Andalusia (Spain). Four ensemble model predictions are shown here: (A) committee averaging; (B) mean; (C) probability mean weight decay; (D) coefficient of variation.
Black pine present and future habitat suitability
The ensemble SDM of P. nigra subsp. salzmannii in Andalusia predicted the highest probability of occurrence over the eastern areas (Sierra Cazorla, Sierra María, Sierra de Baza, and Sierra Nevada - Fig. 3), with a lesser presence in the mid-south (Sierra de las Nieves), which largely agrees with the current distribution of the species. The three ensemble models selected (CA, WD, and mean) showed a high degree of agreement in their predictions (Fig. 4). Sierra de Cazorla appears as the area with the highest P. nigra subsp. salzmannii occurrence within Andalusia, with a total surface area coverage of 50% (Fig. 3, see also Tab. S2 in Supplementary material) in its current prediction.
Fig. 4 - Percentage loss of the area of habitat suitability of P. nigra subsp. salzmannii under future projections (2040, 2070, and 2099): for different scenarios (SRA2, SRA1B, and SRB1), three Global Circulation Models (GCM: BCM2, CNCM3, and ECHAM5), and one Regional Circulation Model (EGMAM). Percentage of habitat suitability remaining in relation to the total present area of P. nigra subsp. salzmannii, predicted by the Probability Mean Weight Decay (WD) and Committee Averaging (CA) ensemble models.
In the forecasted habitat suitability, the general probability of P. nigra subsp. salzmannii occurrence in Andalusia widely decreases (Fig. 4, see also Tab. S2, Fig. S2 in Supplementary material), and the species is expected to lose habitat suitability at moderate altitudes, probably extending to higher ones as climate change proceeds (Fig. 4, Fig. S2). The southern areas (Sierra de las Nieves) disappear and the probability of species occurrence decreases, compared to the present predictions, for the eastern populations. Overall, all the populations showed a strong decrease in habitat suitability across the different scenarios (Fig. 4, Tab. S2 and Fig. S2 in Supplementary material). The predictions were significantly affected by the scenario, especially for longer periods of time (Fig. S2). The probability occurrence of P. nigra subsp. salzmannii will have diminished by nearly 70% on average by 2099 (Fig. 4, Tab. S2 and Fig. S2). It is worth mentioning that, potentially, Sierra de Cazorla will remain the most suitable area for P. nigra subsp. salzmannii growth, even under the most pessimistic scenarios.
The future predictions forecast a reduction in the potential habitat of P. nigra subsp. salzmannii, of 50% in 2040 and 99% in 2099 (Tab. S2 and Fig. S2 in Supplementary material). These results varied depending on the GCM used and the scenario, while the ensemble modeling technique used had a minor effect. The more optimistic results predict a habitat suitability reduction close to 40% (BCM2, SRB1) by 2099, while others predict a loss of over 99% (EGMAN, SRA2) in the same period. In general, the regional GCM (EGMAM) presented predictions more pessimistic than those of the intercontinental ones (Tab. S2 and Fig. S2). The most optimistic results were given by CNCM3 and BCM2; the potential area remaining exceeded 20% in some cases.
Discussion
Species distribution models are a simple yet efficient statistics-based method used to map the spatial range of species and to forecast climate change impacts on species ranges ([16], [14]). They have been used for many different purposes, including: conservation management, theory testing in biogeography and ecology, species management, and climate change impact assessment ([16]). In this work, we used an integrated model approach to assess the spatiotemporal patterns of suitable habitats for P. nigra subsp. salzmanni in the southern Iberian Peninsula, under four climate models. Biomod2 has shown the simplicity involved in the modelling, which enables its use for model distribution shifts resulting from climate change. In particular, our study constitutes a new approach with respect to other models previously used for predicting the distribution of the species ([3], [26], [28]), due to its focus on a relevant regional-scale design and on the populations most sensitive to climate change. One of the remarkable characteristics of our approach is the applicability of the results in biodiversity conservation and forest management ([20]).
Current potential habitat description
The six main environmental variables used, listed in order of importance, were: NDF, PRC, IAR, SSUP, ETO, and SNOW (Tab. 1). NDF had the strongest influence on P. nigra distribution, in agreement with other studies that stressed the key role of low temperatures to explain the geographical distribution of P. nigra in Mediterranean areas ([17], [13]). P. nigra is benefited by an increase in the NDF, particularly in dry Mediterranean mountain areas, where it can compete with other co-existing coniferous species which are more sensitive to drought (P. sylvestris and P. pinaster) or to low temperatures (P. halepensis). This geographic distribution has traditionally been related to a relatively short vegetative period, high moisture, and a high degree of continentality ([13]), as well as to variables that are negatively related to the minimum average temperature ([41]). This agrees with the experimental findings of Gandullo & Palomares ([17]), who reported an optimal distribution for this species on northern slopes above 1000 m a.s.l., with mean annual temperatures ranging between 6.1 and 10.5 °C.
The dependence of P. nigra subsp. salzmannii on the annual precipitation shows its high sensitivity to annual and seasonal rainfall oscillations. The greatest potential suitability for the species has been reported in areas with annual rainfall of over 950 mm, with minimum summer precipitation of over 25-30 mm ([17], [26]). Consequently, the species requires a certain degree of soil moisture, although it is also one of the few tree species that resists the most severe winter conditions (SNOW) in southern Spain as well as very dry summers (IAR), frequently found in Mediterranean high mountains supporting a snowpack as an important compensation feature for dry conditions. Under such conditions (mountain climate at high altitudes), P. nigra subsp. salzmannii is highly competitive and is one of the main forest species in the Mediterranean region ([21]).
Model selection
The biomod2 software has been used to predict the habitat of forest species of different forms and parameterizations ([12]). In this work, we have demonstrated differences in prediction performance between modeling methods at a regional scale. In general terms, the results agree with those obtained from the application of biomod2 to the prediction of the distribution of forest species at a local and regional scale ([26]). The linear methods (GLM and GAM) surpassed in accuracy the rest of the techniques used. Simple linear relationships using the selected climate variables and occurrence point locations could explain the highly specific habitat suitability of P. nigra subsp. salzmannii. The classification methods (BRT, CART, and RF), which are based on multiple data partitioning and increase the model’s complexity, also gave accurate relationships, supporting the linear relationship between the habitat suitability of P. nigra and the environmental variables. The methods ANN, FDA, and MARS yielded accurate responses, although with a high variance; such responses might be due to chance or proper set data through cross-validation. MaxEnt showed a surprisingly low predictive power and stability for the three statistics studied, despite being one of the most widely used modeling techniques and being able to build linear relationships between response and explanatory variables ([12]). Finally, the power of SRE in the modelling of species distribution, in comparison with other methods, has been doubted. In this study, the used ensemble models differed in terms of future predictions, depending on the GCM studied. The ensemble model calculated by WD presented a higher overall total area for the current prediction than the ensemble modeling calculated by CA. Moreover, the percentage area loss was also higher for the ensemble model calculated by WD. These results could be due to an over-prediction of the current area, the difference being marked in the Sierra Nevada study area with 500 ha more in the ensemble model calculated by WD than for CA (Tab. 1, Tab. 2).
Future habitat projection
Recent studies have predicted a reduction in P. nigra subsp. salzmannii habitat suitability and tree growth in the next few decades in southern Europe ([40], [26], [6], [28]), related to an increase in temperatures. Accordingly, our results suggest that winter temperatures and seasonal precipitation shape the distribution of P. nigra subsp. salzmannii in southern Spain ([8]), and that shifts to higher altitudes rather than higher latitudes are expected in the future ([32]). Habitat models showed the sensitivity of this species to minimum temperatures and precipitation, in agreement with previous studies in the Mediterranean region indicating these as crucial factors determining, for example, Quercus ilex and Q. faginea forests ([15]).
Our results suggest a dramatic reduction in locations representing a suitable habitat for P. nigra subsp. salzmannii due to climatic transitions: by 24.8% (ECHAM5-SRA2) to 52.9% (EGMAM-SRA1B) of the extent of their current distributions (as simulated for the present climate) in 2040, and by 55.5% (BCM2-SRB1) to <99% (ECHAM5-SRA2, EGMAM-SRA2 and SRB1) in 2099 (Tab. 4). This trend, namely the loss of suitable habitats, was consistent among climate scenarios and populations.
Alternatively, the populations could extend northwards to central Spain in response to future conditions, and we assumed here that the species fully achieves its potential changes in distribution (meaning that we did not assume any dispersal limitation). That is, however, an unlikely and optimistic assumption. The fact that P. nigra subsp. salzmannii is a mountain species whose populations are highly isolated, with scant mountain ecosystems connecting the northern and central Iberian Peninsula populations with the southernmost ones, suggests that this scenario would be unlikely ([9]). Therefore, P. nigra subsp. salzmannii may only persist in the long term as residual populations derived from its present distribution. Also, there is a great threat of local extinction, especially for those populations with a more restricted distribution (Sierra de Baza, Sierra de la Almijara, and Sierra de María - [8], [32]). Regarding altitude migration, although there are well-documented examples of populations migrating to altitudes higher than those at which they occur today as an apparent response to the ongoing climate change, the speed of that migration is much slower than would be needed to track the changing climate ([24]). In the case of P. nigra subsp. salzmannii in southern Spain, an upward migration of 300-400 m would be required to compensate for the change in climate expected for the year 2040 as predicted, for instance, by the A2 scenario of the Canadian GCM ([9]). Forest mortality may be an observable response to these changes, as suggested by previous studies where dendrochronological and ecological data showed the critical and marginal situation of P. nigra subsp. salzmannii populations ([25], [34], [41]).
However, projections of potential future distributions also need to be interpreted with caution. Even if the models presented in this study are quite accurate and are commonly used to assess the impact of global change ([45]), we have not accounted here for biotic interactions that may modify habitat suitability. For example, positive interactions with mycorrhizal fungi may enable trees to withstand drier conditions without any significant growth reduction ([11]). Furthermore, trees are incredibly long-lived and resilient ([33]) and therefore we can expect individuals to persist under sub-optimal conditions for long periods of time ([40]), while waiting for infrequent benign conditions to be reproduced. Therefore, an important aspect that must be considered is forest monitoring. Currently, only time and empirical data will give us a real idea about the reliability of such models ([23]).
Our results have serious implications for species adaptation and forest management ([8], [41]). If this is coupled with the loss of a critical stopover site, the results for P. nigra subsp. salzmannii populations in southern Spain might be catastrophic. Some populations, of course, may be able to migrate (Sierra de Cazorla) to higher latitudes or altitudes in the face of climate change; but other populations would seem to face local extinction. The Sierra de Cazorla populations showed the greatest overlap between the potential and future suitable distributions, highlighting this area as a key genetic resource for the in situ conservation of P. nigra forests in the southern Mediterranean region ([47])
Conclusion
Given the accumulating evidence of recent climate changes, an evaluation of the current and future distributions of P. nigra subsp. salzmannii in Andalusia (southern Spain) is described in this paper. This information is necessary to ensure that the most threatened populations of such species will be afforded protection in response to climate change, by adjusting their protection status and forest management. Our results show an important contraction of the distribution area for all GCM models, with a minimum potential reduction of 40% (BCM2, SRB1) in 2099. The changing climate will inevitably result in impacts on biomes and community structures. Thus, its mitigation, as well as adaptation to potential future scenarios, is vital to the conservation of climate-sensitive species. Future research that combines bioclimatic niche modeling with a mechanistic disturbance, dispersal, and competition model will be likely to provide a greater insight into the potential range of P. nigra subsp. salzmannii in the face of climate change. Furthermore, it would supply information that influenced forest management options and restoration - that may include ecological restoration, selected thinning, or assisted migration. Some alternatives would ensure “fine-grained” landscapes with patches of a diverse range of semi-natural habitats - including woodlands, heathlands, and shrubs - that offered suitable habitat niches to sustain distribution responses to changing environmental conditions. Additionally, the distribution of protected areas should be revised to include extensive areas of natural or semi-natural habitats of coniferous Mediterranean forests, in particular plantations such as artificial P. nigra subsp. salzmannii forests, which offer a diverse range of physical habitats.
Acknowledgements
We acknowledge the financial support of ESPECTRAMED (CGL2017-86161-R), University of Córdoba - Campus de Excelencia CEIA3, and AEMET (Agencia Estatal de Meteorologia) for providing meteorological data. R. Sánchez-Salguero has been supported by a postdoctoral fellowship (FEDER - Programa de Fortalecimiento en I+D+i de las Universidades 2014-2015 de la Junta de Andalucía) and the “CoMo-ReAdapt” Spanish project (CGL2013-48843-C2-1-R). The authors also thank Dr. Miguel B. Araujo for his valuable comments at the beginning of this work.
Authors’ contributions
RMNC conceived the study, analyzed data, performed research, and wrote the paper; JDL analyzed data and contributed new methods; RDM conceived the study and performed research; RSS and GPR wrote the paper.
References
Online | Gscholar
Gscholar
Gscholar
Gscholar
Gscholar
Gscholar
CrossRef | Gscholar
Gscholar
CrossRef | Gscholar
Gscholar
Authors’ Info
Authors’ Affiliation
Joaquín Duque-Lazo
Guillermo Palacios-Rodriguez
Department of Forestry, School of Agriculture and Forestry, University of Córdoba, Edf. Leonardo da Vinci, Campus de Rabanales s/n, Mail Box 3048, E-14071 Córdoba (Spain)
Institute of Plant Sciences, University of Bern, Altenbergrain 21, CH-3013 Bern (Switzerland)
Area de Ecología, Dpto. Sistemas Físicos, Químicos y Naturales, Universidad Pablo de Olavide, Ctra. Utrera km. 1, E-41013 Sevilla (Spain)
Corresponding author
Paper Info
Citation
Navarro-Cerrillo RM, Duque-Lazo J, Manzanedo RD, Sánchez-Salguero R, Palacios-Rodriguez G (2018). Climate change may threaten the southernmost Pinus nigra subsp. salzmannii (Dunal) Franco populations: an ensemble niche-based approach. iForest 11: 396-405. - doi: 10.3832/ifor2588-011
Academic Editor
Francesco Ripullone
Paper history
Received: Aug 07, 2017
Accepted: Mar 10, 2018
First online: May 15, 2018
Publication Date: Jun 30, 2018
Publication Time: 2.20 months
Copyright Information
© SISEF - The Italian Society of Silviculture and Forest Ecology 2018
Open Access
This article is distributed under the terms of the Creative Commons Attribution-Non Commercial 4.0 International (https://creativecommons.org/licenses/by-nc/4.0/), which permits unrestricted use, distribution, and reproduction in any medium, provided you give appropriate credit to the original author(s) and the source, provide a link to the Creative Commons license, and indicate if changes were made.
Web Metrics
Breakdown by View Type
Article Usage
Total Article Views: 41328
(from publication date up to now)
Breakdown by View Type
HTML Page Views: 34754
Abstract Page Views: 2708
PDF Downloads: 3043
Citation/Reference Downloads: 14
XML Downloads: 809
Web Metrics
Days since publication: 2265
Overall contacts: 41328
Avg. contacts per week: 127.72
Article Citations
Article citations are based on data periodically collected from the Clarivate Web of Science web site
(last update: Feb 2023)
Total number of cites (since 2018): 8
Average cites per year: 1.33
Publication Metrics
by Dimensions ©
Articles citing this article
List of the papers citing this article based on CrossRef Cited-by.
Related Contents
iForest Similar Articles
Research Articles
Predictive capacity of nine algorithms and an ensemble model to determine the geographic distribution of tree species
vol. 15, pp. 363-371 (online: 20 September 2022)
Review Papers
Climate change impacts on spatial distribution, tree-ring growth, and water use of stone pine (Pinus pinea L.) forests in the Mediterranean region and silvicultural practices to limit those impacts
vol. 14, pp. 104-112 (online: 01 March 2021)
Review Papers
Modeling Italian forests: state of the art and future challenges
vol. 5, pp. 113-120 (online: 05 June 2012)
Review Papers
Impacts of climate change on the establishment, distribution, growth and mortality of Swiss stone pine (Pinus cembra L.)
vol. 3, pp. 82-85 (online: 15 July 2010)
Review Papers
Quantifying and modeling water availability in temperate forests: a review of drought and aridity indices
vol. 12, pp. 1-16 (online: 10 January 2019)
Research Articles
Modeling of early stage litter decomposition in Mediterranean mixed forests: functional aspects affected by local climate
vol. 8, pp. 517-525 (online: 18 November 2014)
Short Communications
Determining Pleiades satellite data capability for tree diversity modeling
vol. 10, pp. 348-352 (online: 19 November 2016)
Research Articles
Predicting impacts of climate change on forest tree species of Bangladesh: evidence from threatened Dysoxylum binectariferum (Roxb.) Hook.f. ex Bedd. (Meliaceae)
vol. 10, pp. 154-160 (online: 25 May 2016)
Research Articles
Spatial modeling of the ecological niche of Pinus greggii Engelm. (Pinaceae): a species conservation proposal in Mexico under climatic change scenarios
vol. 13, pp. 426-434 (online: 16 September 2020)
Research Articles
Predicting the effect of climate change on tree species abundance and distribution at a regional scale
vol. 1, pp. 132-139 (online: 27 August 2008)
iForest Database Search
Google Scholar Search
Citing Articles
Search By Author
Search By Keywords