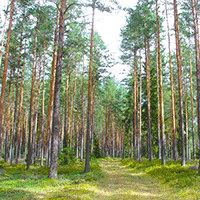
Belowground biomass models for young oligotrophic Scots pine stands in Latvia
iForest - Biogeosciences and Forestry, Volume 11, Issue 2, Pages 206-211 (2018)
doi: https://doi.org/10.3832/ifor2553-010
Published: Mar 01, 2018 - Copyright © 2018 SISEF
Research Articles
Abstract
The increasing interest in carbon budget estimation and the growing use of woody biomass in bioenergy production raises the necessity for precise estimates of belowground biomass and soil carbon pools in forest ecosystems, particularly in terms of changes in the age structure of forests. The aim of this study was to estimate the belowground biomass of young (< 40 years) stands of Scots pine (Pinus sylvestris L.) in Latvia. The biomass of small roots (diameter 2-20 mm), coarse roots (diameter > 20 mm), and stumps of 39 trees from eight stands growing on dry, nutrient-poor mineral soils was measured and compared to the aboveground variables of sampled trees. The results revealed that stumps, small roots, and coarse roots comprised 43%, 35% and 22%, respectively, of the belowground biomass of young Scots pines. The proportion of belowground biomass over the total tree biomass was age-dependent, ranging from 33% to 17% for 8-year and 40-year old trees, respectively. Aboveground tree variables were significantly correlated with the belowground biomass, being stemwood volume and basal area the best predictors (R2 = 0.86-0.98, relative errors = 26-43%) of the belowground biomass components. Accordingly, the developed models produced more accurate estimates compared to previous models for the region, thus reducing the uncertainty in determining the carbon budget for belowground biomass. Still, an analysis of a more comprehensive dataset is needed to account for the effect of the social status of trees, as well as the within- and between-stand variation.
Keywords
Hemiboreal Forests, Europe, Pinus sylvestris, Allometric Equation, Coarse Roots, Total Root Biomass
Introduction
The increasing international efforts aimed at mitigating climate change have raised interest in forest biomass for bioenergy production ([40]), and highlighted the role of forest biomass in carbon (C) cycling ([9], [41]). Many studies have recognized the importance of large-scale, as well as stand-level factors in determining the dynamics of forest biomass ([44], [28]), particularly in boreal forests, which represent one of the largest C pools in the world ([15]).
Belowground biomass accounts for 18-45% of the total tree biomass in coniferous stands ([17], [12], [25]), indicating a large variability and uncertainty in belowground C estimations ([15], [2]). The root system consists of various components which are difficult to be directly estimated, despite their vital role in the development and functioning of trees ([27], [31]). Numerous field methods and allometric models for the estimation of aboveground biomass have been previously published ([37], [30]). Contrastingly, the development of accurate methods for quantifying belowground biomass has lagged behind, primarily due to methodological challenges and the laborious and costly collection of empirical data on heterogeneous root systems ([7], [45], [26]).
Many factors, such as location (climate), species, age, forest type, and soil oxygen and moisture regime, influence the development of root system of trees ([27], [13], [16]). Accordingly, the need to develop local, site-based belowground biomass models for various tree species has been recognized throughout Europe ([47], [29]). Emphasis has been placed on the extension of models across different species, in order to predict belowground biomass using easy-to-measure aboveground variables of trees ([11], [30]).
Scots pine (Pinus sylvestris L.) is one of the main tree species in the Eurasian boreal and boreo-nemoral zone, representing about 20% of northern forests ([20]). In Europe, it is an economically important species that can withstand various weather extremes ([13]). Whilst numerous studies on Scots pine biomass have been conducted in Fennoscandia ([16], [36]), studies on belowground biomass of Scots pine in the Baltic region are scarce, though some results have been recently published ([5], [24]). Moreover, from 1990 to 2010, the proportion of young Scots pine stands have increased, due to the intensification of harvesting (58- 62% of total annual increment harvested - [23]) and of natural disturbances related to climate change ([38]). Therefore, understanding the dynamics of belowground biomass and the C sink capability of Scots pine young trees and stands is crucial ([33]).
The aim of this study was to estimate root biomass and to develop models for the assessment of belowground biomass of young Scots pine stands growing in oligotrophic conditions in Latvia.
Material and methods
Study sites and sampling
Latvia is situated in the hemiboreal forest zone, with mixed broad leaf-coniferous forests ([3]). In the study area, the mean annual temperature was +6.4 °C, ranging from -5.3 °C in January to +17.3 °C in July, with the mean annual precipitation of 640 mm ([21]). Scots pine covers 29% of the total forest area in Latvia, and approximately half of the stands are located on dry mineral soils ([4]). Turf podsoled soils cover more than half of the country ([14]).
The study material was collected from eight stands during the vegetation period (June to August 2015). The age of the sampled trees ranged from eight to 40 years. Seven of the studied stands had been regenerated using 2-year-old, bare-rooted or containerized seedlings; the soil had been prepared by disk trenching. One of the youngest stands was left to regenerate naturally, without soil preparation. The stands were located on dry, nutrient-poor sandy and silty automorphic soils, and corresponded to Cladinoso-callunosa, Vacciniosa and Myrtillosa forest types, according to the classification by Bušs ([10]), which represent the typical conditions of Scots pine forests in Latvia.
In each stand, one circular sampling plot was established (eight sampling plots in total). The plot size varied according to the stand’s age: 100 m2 (diameter = 11.28 m) in stands younger than 15 years, and 500 m2 (diameter = 25.24 m) in the others. In each plot, the diameter at breast height (DBH, ± 0.1 cm) of all trees and the height (H, ± 0.1 m) of 10 sample trees were measured (Tab. 1). To determine the forest type, soil samples were collected from all sampling plots (Tab. 1).
Tab. 1 - Characteristics of sampled Scots pine stands. (H): tree height; (DBH): mean diameter at breast height of sampled trees.
Stand age |
Stand location | No. of sampled trees |
H (m) |
DBH (cm) |
Basal area (m2 ha-1) |
Stand density (tree ha-1) |
Forest type | |
---|---|---|---|---|---|---|---|---|
Lat. N | Long. E | |||||||
8 | 56°50.54′ 56°50.60′ |
24°38.33′ 24°38.34′ |
12 | 2.6 | 2.0 | 2.1 | 6200 | Myrtillosa |
12 | 56°34.58′ | 25°01.21′ | 2 | 4.0 | 6.6 | 7.3 | 2145 | Cladinoso-callunosa |
13 | 56°24.58′ | 25°11.21′ | 5 | 4.6 | 5.2 | 4.8 | 2215 | Cladinoso-callunosa |
14 | 56°51.34′ | 24°35.01′ | 6 | 4.8 | 5.3 | 7.2 | 3200 | Myrtillosa |
24 | 56°41.00′ | 24°27.43′ | 10 | 10.0 | 11.0 | 13.2 | 1385 | Vacciniosa |
40 | 56°43.00′ 56°43.00′ |
24°34.62′ 24°34.12′ |
4 | 17.5 | 19.5 | 17.1 | 575 | Cladinoso-callunosa |
In total, 39 sample trees (2-6 trees per plot), according to the distribution of DBH classes in the stands, were selected randomly for destructive sampling of their root biomass. Trees with visible signs of disease, stem defects (e.g., broken top, double stems), or leaders were avoided. To assess total tree biomass, the fresh weight of aboveground components was determined (± 0.02 kg). Stumps with root components were carefully dug out by hand, separated from soil particles, and washed. The fresh weight of small roots (diameter 2-20 mm), coarse roots (diameter > 20 mm), and the stump ([15]) was measured (± 0.02 kg). Fine roots (diameter < 2 mm) were not collected, as they are associated with the soil microflora, and thus their accurate weight is difficult to assess ([1]). The stump components included both belowground and aboveground (5-8 cm above the root collar) parts. For the smallest trees (DBH < 5.6 cm), representing all of the 8-year-old sample trees and the six smallest of the 12- to 14-year-old sample trees, only small roots were found. Samples of fresh weight from the tree components (with bark) were collected, dried at 105 °C, and weighed separately for calculating the dry biomass, in accordance with Uri et al. ([43]).
Data analysis
Pearson’s correlation analysis was used to determine the relationships between the measured variables. To ensure a wide application to the established models of belowground biomass, they were not developed for specific forest site types. Models for stumps, small, and coarse roots, as well as total belowground biomass, were developed for individual trees, based on the aboveground variables: DBH, H, and approximations of stem basal area (S = DBH2) and stemwood volume (V = DBH2·H).
The regression model (eqn. 1) was:
where yi is the belowground biomass component (kg) of the i-th tree, xi represents the independent variables (predictor) for the tree, and a and b are the parameters to be estimated. Additionally, models based on DBH and H as predictors were evaluated (eqn. 2):
where a, b and c are the parameters to be estimated.
To select the best fitting model for the estimation of belowground biomass components, R2 value, F-value, relative error (RE), root-mean square deviation (RMSE), and the Akaike’s information criterion (AIC) were used. Modelled data residuals were graphically checked for normality, linearity, homoscedasticity, independency and outlier identification. A logarithmic transformation of data was tested, but preliminary results showed a worst fit to the empirical data; hence, simple linear models were applied. Differences between model predictions and the observed biomass were assessed using t-tests (α = 0.05).
To assess the accuracy of the developed models, their predictions were compared to predictions from the models of Repola ([36]) for Scots pine stumps and roots (diameter > 10 mm), which have been previously applied in the Baltic Sea region. Coarse root and small root biomass were summed to obtain total root biomass for comparison. The accuracy of the model recently developed for Scots pine belowground biomass in Latvia ([24]) was also tested, with an emphasis on young stands. The comparisons were made by t-test for paired observations. All statistical analyses were made using the software R version 3.3.1 ([35]).
Results
The DBH and H of the sampled trees (n = 39) ranged from 1.2 to 18.6 cm, and from 2.2 to 17.5 m, respectively. The mean total belowground biomass (± 95% confidence interval, CI) of young Scots pine was 11.32 ± 5.34 kg, comprising 12-58% of the total tree biomass. The mean ratio (± standard deviation) of the belowground and total tree biomass decreased with tree age; for 8-, 14-, 24- and 40-year-old Scots pine, it was 0.33 ± 0.07, 0.21 ± 0.06, 0.16 ± 0.03 and 0.17 ± 0.01, respectively. Since not all of the trees had coarse roots, the stump appeared to be the largest belowground component, followed by small roots, then coarse roots (Tab. 2). The estimated total belowground biomass of individual trees was highly variable, ranging from 0.7 to 67.4 kg. The mean biomass ± CI of the coarse roots, small roots, and stumps was 7.99 ± 4.29, 2.08 ± 0.65 and 4.18 ± 1.91 kg, respectively.
Tab. 2 - Mean ratios of root components biomass (± standard deviation) from the measured tree biomass. (AGB): Aboveground biomass; (BGB): belowground biomass.
Root ratio from tree biomass components |
Small roots (2-20 mm) |
Coarse roots (>20 mm) |
Stump | Total BGB |
---|---|---|---|---|
Ratio of total BGB | 0.35 ± 0.17 | 0.22 ± 0.21 | 0.43 ± 0.12 | - |
Ratio of AGB | 0.12 ± 0.10 | 0.05 ± 0.09 | 0.15 ± 0.11 | 0.33 ± 0.23 |
Ratio of total tree biomass | 0.09 ± 0.06 | 0.04 ± 0.04 | 0.10 ± 0.05 | 0.23 ± 0.10 |
Significant correlations (0.61 < r < 0.99) between the root biomass components and the tree variables were observed (Tab. 3). The belowground biomass components had a stronger correlation with DBH than with H, as the mean r was 0.91 and 0.76, respectively (Tab. 3). Trees with a larger DBH (R2 = 0.78) and H (R2 = 0.68) had greater total belowground biomass, indicating an age-dependent increase (Tab. 3); however, the age effect was not included in the calculations, as it correlated with both DBH and H (r = 0.69 and 0.93, respectively; Tab. 3). Total belowground biomass was highly correlated (r = 0.99) with coarse root and stump biomass.
Tab. 3 - Pairwise Pearson’s correlation coefficients (below diagonal) and their significance (p-values - above diagonal) among variables of young Scots pine trees. (DBH): diameter at breast height; (BGB): belowground biomass.
Variable | Tree height |
DBH | Age | Stump biomass |
Coarse root biomass |
Small root biomass |
Total BGB |
---|---|---|---|---|---|---|---|
Tree height | 1 | < 0.001 | < 0.001 | < 0.001 | < 0.001 | < 0.001 | < 0.001 |
Tree DBH | 0.86 | 1 | < 0.001 | < 0.001 | < 0.001 | < 0.001 | < 0.001 |
Stand age | 0.93 | 0.69 | 1 | < 0.001 | < 0.001 | < 0.001 | < 0.001 |
Stump biomass | 0.79 | 0.91 | 0.73 | 1 | < 0.001 | < 0.001 | < 0.001 |
Coarse root biomass | 0.80 | 0.91 | 0.68 | 0.96 | 1 | < 0.001 | < 0.001 |
Small root biomass | 0.66 | 0.89 | 0.61 | 0.93 | 0.90 | 1 | < 0.001 |
Total belowground biomass | 0.80 | 0.92 | 0.74 | 0.99 | 0.99 | 0.93 | 1 |
The allometric belowground biomass models (eqn. 1) based on stemwood volume (V) and stem basal area (S) gave the most precise estimates (R2 = 0.80-0.98) of root biomass components (Tab. 4), particularly for total belowground (R2 = 0.98) and coarse root (R2 = 0.98) biomass. The models including V as predictor of total, coarse root, and stump biomass estimation demonstrated the highest precision (R2 > 0.92), but the best model for small root biomass prediction (R2 = 0.86) included S as predictor (Tab. 4). Averaged across the sites, the modelled values gave a RE of only 3.6% in the weighed total belowground biomass. Both parameters of the biomass models were significant (p < 0.05), except for the intercept for the coarse roots model (Tab. 4). Graphical analysis showed that models based on S and V best performed in the estimation of root biomass components; however, the REs for the total belowground and coarse root biomass were 26% and 43%, respectively, indicating different predictive performances (Tab. 4). The variance explained by the models based on both H and DBH as independent variables was lower (R2 = 0.54- 0.82).
Tab. 4 - Parameter estimates and statistics of the models developed for root biomass prediction in young Scots pine trees in Latvia. (S): stem basal area; (V): stemwood volume. Standard error of parameter estimates (a, b) are given in brackets. (BGB): Belowground biomass; (RMSE): root-mean square deviation; (df): degrees of freedom; (*): p < 0.05; (**): p < 0.01; (***): p < 0.001.
Model Statistics | Small roots (2-20 mm) |
Coarse roots (>20 mm) |
Stump | Total BGB | |
---|---|---|---|---|---|
Model | S = DBH2 | V = DBH2 · H | V = DBH2 · H | V = DBH2 · H | |
Parameters | a | 0.648*** (0.156) |
-0.582 (0.431) |
0.766* (0.291) |
1.543** (0.496) |
b | 196.490*** (13.317) |
57.872*** (1.938) |
38.421*** (1.746) |
110.155*** (2.905) |
|
R2 | 0.858 | 0.977 | 0.929 | 0.975 | |
Adj-R2 | 0.854 | 0.976 | 0.927 | 0.974 | |
df | 1, 36 | 1, 21 | 1, 37 | 1, 37 | |
F-statistics | 217.7 | 891.7 | 484.0 | 1438.0 | |
Overall significance (p) | < 0.001 | < 0.001 | < 0.001 | < 0.001 | |
RMSE | 0.729 | 1.471 | 1.549 | 2.576 | |
Relative error (%) | 36.9 | 42.6 | 28.4 | 25.9 |
The models proposed by Repola ([36]) and Liepins et al. ([24]) significantly (p < 0.001) underestimated the actual belowground biomass of young Scots pine in oligotrophic stands, and their predictions were lower compared to our models (Fig. 1). Accordingly, the RE for the predictions of total belowground biomass was 76% using the model proposed by Repola ([36]) and 68% using the model proposed by Liepins et al. ([24]). Our model of total belowground biomass for young Scots pine in Latvia gave a substantially higher proportion of variance explained (RE = 26%), thus reducing the RE by 42% compared to both previously published models.
Discussion
In this study, the mean value of the total belowground biomass observed was similar to the estimates obtained for Scots pine in Central Europe ([46]), though comparable studies in the literature are still sparse and the belowground biomass estimates highly variable. Moreover, differences in biomass allocation pattern have been observed among populations of Scots pine, with a general trend of lower root biomass in Northern Europe stands ([32]). Such differences have been explained by the effects of forest type, soil structure, moisture regime, and chemical composition ([13]), which are considered key factors affecting the formation of root systems. This might also be related to age differences among the studied stands, as well as to the competition between young trees leading to their rapid development ([45]). The root system has proven to adapt to different environmental conditions, so trees growing in nutrient-poor forests (as is the case of our study area) are expected to develop a smaller amount of root biomass (Vanninen et al. 1996, [6]).
The distribution of belowground biomass components (Tab. 2) observed in this study was highly variable, according to previous reports ([17], [33], [34]); however, the belowground biomass estimates (Tab. 2) were comparable to the results of Helmisaari et al. ([19]) and Vanninen et al. ([44]) in Finnish Scots pine, likely due to environmental conditions similar to those present in Latvia. Further, the ratio of belowground on total biomass of trees decreased with tree age, due to more rapid development of aboveground biomass over the same time span ([42]). Tree dimensions (in terms of DBH and H) showed a strong correlation with belowground biomass components (Tab. 3); accordingly, both variables could be successfully used for root biomass estimation in nutrient-poor sites, as previously demonstrated by Xiao & Ceulemans ([46]) and Lehtonen et al. ([22]). Stemwood volume (V) and stem basal area (S), which were included in our models (Tab. 4), have previously been demonstrated to be the best individual variables for fine root biomass estimation ([22]). Since the best prediction of coarse root biomass was obtained using stemwood volume (Tab. 4), models for stump and total belowground biomass estimations were based on this variable. The high accuracy of V in the prediction of root biomass has previously been shown for Scots pine seedlings ([18]). The stem basal area was the best variable for small root biomass estimation (Tab. 4), indicating a link between the amount of absorbing roots and the crown size with the stem diameter, as proposed by the pipe-model theory ([39]). Overall, biomass models using V as predictor had lower errors (Tab. 4), hence both DBH and H affect tree size over time ([24]). The coarse root biomass estimates had the highest errors (RE = 43% - Tab. 4), which could be explained by the smaller sample size compared to the other analyzed components. The belowground biomass models developed in this study showed higher precision than previously published models for Scots pine, which were calibrated for a wider spectrum of stand ages ([36], [24]). Herein, the application of stump and root models proposed by Repola ([36]) led to underestimate the belowground biomass components (Fig. 1), suggesting a limitation for its wider application ([8]). The model developed by Liepins et al. ([24]) closely followed the values we obtained using the Repola’s model (Fig. 1c), indicating that stand age should be accounted for when estimating precise belowground biomass. Considering that similar belowground biomass estimates were obtained in Estonia ([45]), along with the similarity of Scots pine forest structure therein, we speculate that our models might be successfully applied to predict belowground biomass of young Scots pine trees over the eastern Baltic region. However, a more comprehensive dataset covering a larger area of comparable conditions ([2]) would be desirable to this purpose.
Conclusions
The models developed in this study reduced the overestimation of belowground biomass of young Scots pine trees, as compared with previously developed models based on a full rotation cycle in the Baltic Sea region. This leads to a reduction of uncertainty in the estimation of carbon budget of belowground biomass. The developed models also appear to be useful tools for the estimation of belowground biomass supply in young Scots pine stands in relation to the potential for bioenergy production. However, the analysis of a more comprehensive dataset is desirable, in order to account for the effect of the social status of trees, and for the withinand between-stand variation.
Acknowledgements
This study was funded by the European Regional Development Fund Project “Development of decision support tools for prognosis of storm damage in forest stands on peat soils” (No. 1.1.1.1/16/A/260).
References
Gscholar
Gscholar
Gscholar
Gscholar
Gscholar
CrossRef | Gscholar
CrossRef | Gscholar
Gscholar
Authors’ Info
Authors’ Affiliation
Andis Bardulis
Roberts Matisons
Rolands Kapostins
Aris Jansons
Latvian State Forest Research Institute Silava, Rigas Street 111, LV-2169 Salaspils (Latvia)
Corresponding author
Paper Info
Citation
Kenina L, Bardulis A, Matisons R, Kapostins R, Jansons A (2018). Belowground biomass models for young oligotrophic Scots pine stands in Latvia. iForest 11: 206-211. - doi: 10.3832/ifor2553-010
Academic Editor
Claudia Cocozza
Paper history
Received: Jul 14, 2017
Accepted: Dec 20, 2017
First online: Mar 01, 2018
Publication Date: Apr 30, 2018
Publication Time: 2.37 months
Copyright Information
© SISEF - The Italian Society of Silviculture and Forest Ecology 2018
Open Access
This article is distributed under the terms of the Creative Commons Attribution-Non Commercial 4.0 International (https://creativecommons.org/licenses/by-nc/4.0/), which permits unrestricted use, distribution, and reproduction in any medium, provided you give appropriate credit to the original author(s) and the source, provide a link to the Creative Commons license, and indicate if changes were made.
Web Metrics
Breakdown by View Type
Article Usage
Total Article Views: 39323
(from publication date up to now)
Breakdown by View Type
HTML Page Views: 33854
Abstract Page Views: 1811
PDF Downloads: 2891
Citation/Reference Downloads: 14
XML Downloads: 753
Web Metrics
Days since publication: 2316
Overall contacts: 39323
Avg. contacts per week: 118.85
Article Citations
Article citations are based on data periodically collected from the Clarivate Web of Science web site
(last update: Feb 2023)
Total number of cites (since 2018): 8
Average cites per year: 1.33
Publication Metrics
by Dimensions ©
Articles citing this article
List of the papers citing this article based on CrossRef Cited-by.
Related Contents
iForest Similar Articles
Research Articles
Fine root morphological traits and production in coniferous- and deciduous-tree forests with drained and naturally wet nutrient-rich organic soils in hemiboreal Latvia
vol. 16, pp. 165-173 (online: 08 June 2023)
Research Articles
Effects of understory removal on root production, turnover and total belowground carbon allocation in Moso bamboo forests
vol. 9, pp. 187-194 (online: 20 November 2015)
Research Articles
Allometric relationships for volume and biomass for stone pine (Pinus pinea L.) in Italian coastal stands
vol. 6, pp. 331-335 (online: 29 August 2013)
Research Articles
Fine root production and distribution in the tropical rainforests of south-western Cameroon: effects of soil type and selective logging
vol. 3, pp. 130-136 (online: 27 September 2010)
Research Articles
Predicting total and component biomass of Chinese fir using a forecast combination method
vol. 10, pp. 687-691 (online: 17 July 2017)
Research Articles
Equations for estimating belowground biomass of Silver Birch, Oak and Scots Pine in Germany
vol. 12, pp. 166-172 (online: 15 March 2019)
Research Articles
Modeling the fine root biomass dispersion using a special influence function
vol. 1, pp. 141-144 (online: 25 November 2008)
Research Articles
Allometric equations to assess biomass, carbon and nitrogen content of black pine and red pine trees in southern Korea
vol. 10, pp. 483-490 (online: 12 April 2017)
Research Articles
Forest litter as the mulch improving growth and ectomycorrhizal diversity of bare-root Scots pine (Pinus sylvestris) seedlings
vol. 8, pp. 394-400 (online: 20 August 2014)
Research Articles
Allometric equations to estimate above-ground biomass of small-diameter mixed tree species in secondary tropical forests
vol. 13, pp. 165-174 (online: 02 May 2020)
iForest Database Search
Search By Author
Search By Keyword
Google Scholar Search
Citing Articles
Search By Author
Search By Keywords
PubMed Search
Search By Author
Search By Keyword