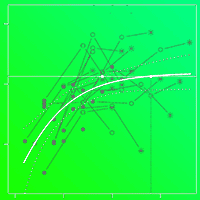
Recovery of above-ground tree biomass after moderate selective logging in a central Amazonian forest
iForest - Biogeosciences and Forestry, Volume 11, Issue 3, Pages 352-359 (2018)
doi: https://doi.org/10.3832/ifor2534-011
Published: May 04, 2018 - Copyright © 2018 SISEF
Research Articles
Abstract
We examined the recovery and dynamics of living tree above-ground biomass (AGB) after selective logging in an Amazonian terra firme forest managed by a private company. The forest consisted of 24 blocks (including one set aside for conservation) selectively logged in different years on a managed schedule. Trees ≥10 cm in diameter at breast height (dbh) were surveyed in 2006 in 192 0.25-ha plots, in 2010 in 119 plots, and in 2012-2013 in 54 plots. A logistic growth model factoring in logging dynamics and mean AGB of a block in these years was established. Referencing the mean AGB of the unlogged forest, the model indicated that the logged forest would take on average 14 years to regain its preharvest AGB after selective logging at 1.9 trees ha-1 (dbh > 50 cm). In 2010 and 2012-2013, the AGB increased significantly for small and large trees (10-20 cm and >60 cm dbh, respectively) in the logged forest. In contrast, it decreased significantly for medium-sized trees (30-50 cm dbh) in the unlogged forest. Comparisons with the previous studies mainly conducted in the other regions of Amazon suggested that the estimated AGB recovery period with moderate logging intensity was almost appropriate and likely acceptable to forest managers.
Keywords
Annual Increment, Dynamics, Logistic Growth, Recovery Period, Terra Firme Forest
Introduction
Forests in the Amazon basin are estimated to hold 86 petagrams (Pg) of carbon (C - [37]), which accounts for roughly 10% of the total carbon stored in forests worldwide (861 ± 66 Pg C - [26]). However, carbon stocks in the Amazon basin have dramatically decreased because of deforestation and forest degradation. Conversion of forest to farmland, selective logging in natural forests, and forest fragmentation ([20], [1], [4]) have all contributed to this decline in global carbon stocks. Selective logging is still widely used in the Amazon region because of high tree species diversity in natural forests and limited markets for timber from less desirable species. Carbon stock, species diversity, and timber yield from subsequent harvests have declined after selective logging ([33]). The carbon emission from logging in Brazil was assessed at 24.9 Tg C y-1, which was >15 times that of other Latin American countries that are part of Amazonia (Bolivia, Guyana and Suriname - [27]). Another study based on remote sensing data estimated the emission of almost double, 40-50 Tg C y-1, from selective logging in the Brazilian Amazon, over a period expected to last two to three decades ([18]). Therefore, selective logging and subsequent biomass loss in the Brazilian Amazon could substantially accelerate climate change.
Modern mechanized selective logging can cause serious injury to residual trees ([44]). Injured trees experience a threefold mortality rate 5 to 10 years after logging ([29]). Carbon emission from logging activities can arise from the damage to surrounding vegetation and infrastructure rather than from the timber itself ([27]). One option for reducing net carbon emissions is to reduce the damage to residual forests during selective logging operations, thereby retaining carbon in the biomass ([31]). Since the early 1950s, sustainable forest management (SFM) for timber production has been a potential tool for the conservation of large areas of tropical forest ([40]). Reduced impact logging (RIL) techniques have proven effective in decreasing damage and enhancing the recovery of logged forests. For example, collateral damage in forests after selective logging can be reduced by up to 50% with RIL techniques ([30]). In addition to this approach, strict regulation of logging intensity and expanded protection of large and commercial trees have been proposed to achieve SFM ([39], [28], [41]).
Despite low-intensity harvesting, forests lose carbon stock from damaged trees that die several years after logging. Conversely, selectively logged forests regain biomass and act as a carbon sink through the recovery process. Thus, net biomass accumulation results from the balance between the growth of residual trees, recruitment of new trees, and tree mortality. For example, living tree biomass constantly increased for 14 years after selective logging in the eastern Amazon ([48]). In contrast, biomass constantly decreased for 3 years in the eastern Amazon, as mortality outpaced growth ([15]). In addition, annual biomass increments fluctuated over time after logging ([24], [40]). Even under SFM, biomass recovery after harvesting can be complicated, with wide variation in the recovery period. The recovery period is directly relevant to management policies that regulate allowable harvest volume and cutting cycles ([36]). More examples of biomass regrowth after selective logging are needed to accurately measure carbon emissions in the Amazon basin and to achieve true SFM including carbon stock. In particular, available information on RIL-operated forests is essentially lacking in the central Amazon.
Monitoring of forest recovery after a disturbance is time intensive; therefore researchers use chronosequencing to analyze the relationships between recovery time and biomass or carbon stock in forests ([16], [19]). However, chronosequence data only permits inferences of successional change and do not facilitate the direct analysis of underlying processes that mediate the change, such as growth, mortality and recruitment ([7]). The combination of chronosequencing and dynamics analyses can provide an effective tool to explain the changes in biomass ([25]). In the present study, we examined the recovery and dynamics of above-ground biomass (AGB) after selective logging under SFM in a typical terra firme forest managed by a private company in the central Amazon. We used a chronosequence data set of logged forest blocks partly combined with repeated measurement data in some of the plots. First, we estimated the AGB recovery period by applying a logistic growth model to determine whether the current management scheme could sustain tree biomass. Second, we focused our analysis of the data on how the AGB recovery process varied by tree size classes after selective logging, that is, the contribution of trees of various sizes to AGB recovery after selective logging. Finally, we compared the relationship between logging intensity and the AGB recovery period with data from previous studies, and discussed appropriate methods of RIL in the Amazon basin for achieving SFM.
Methods
Study site
The study site was located in a managed forest owned by Precious Woods Amazon (PWA) Ltd. that was 40 km west of Itacoatiara, Amazonas, Brazil. PWA manages approximately 75.000 ha (02° 43′ - 03° 04′ S, 58° 31′ - 58° 57′ W - [14]) of dense ombrophilous terra firme forest that is dominated by species belonging to the Burseraceae, Lauraceae, Lecythidaceae, Leguminosae, and Sapotaceae families. The region has a mean annual temperature of 26 °C and a mean annual rain fall of 2.200 mm ([14]). Excluding conservation areas, catchments, and buffer zones, the forest is divided into 25 annual operating blocks of approximately 2.100 ha each, which has been selectively logged since 1995 ([47]). PWA’s selective logging scheme, targeting SFM, is based on the CELOS Management System (CMS - [11]), and has been adapted for the Amazon area. The RIL techniques used by PWA include the mapping target trees, well-designed forest roads and skidder trails, preharvest liana cutting and directional felling. PWA was certified by the Forest Stewardship Council in 1997, and under PWA’s management plan, trees with a trunk diameter at breast height (dbh) of >50 cm (preferably >60 cm), which include about 40 species, are subjected to selective logging at a maximum wood volume of 30 m3 ha-1 in a harvest cycle of 25 years ([13], [14] - personal communications in PWA). Assuming that the average log volume per tree is 6.3 m3 in the Brazilian Amazon ([44], [13]), 30 m3 ha-1 is equal to a harvesting intensity of 4.8 logs ha-1. Although a detailed record of harvested timbers and volume was unavailable, the field survey described stumps and skidder trails with a mean density of 1.9 stumps ha-1 with a mean diameter of 61 ± 19 cm (±SD), and a mean of 0.9 ± 0.8 skidder trails per plot.
Tree inventory and biomass estimation
In 2006, 192 study plots measuring 125 × 20 m (0.25 ha) were established in randomly selected locations in 24 forest blocks, including a conservation block (Tab. 1). Except for the protected area, these blocks have been selectively logged since 1995; therefore, by 2006, the period after logging in each block ranged from 1 to 11 years. The dbh of all trees was ≥10 cm as recorded for the plots. Plot locations were recorded by global positioning system (GPS) receivers because metal tags and survey poles could not be used.
Tab. 1 - Three different tree inventory studies in a managed terra firme forest in Itacoatiara, central Amazon. (a): Plot size was 125 × 20 m (0.25 ha) for all inventories.
Statistic | Inventory year | ||
---|---|---|---|
2006 | 2010 | 2012-2013 | |
Number of plots (a) (Unlogged plots) | 192 (6) | 119 (20) | 54 (5) |
Number of forest blocks | 24 | 19 | 16 |
Mean number of plots per block ± SD | 8.0 ± 2.9 | 6.3 ± 3.5 | 3.4 ± 0.9 |
Range of time since logging (yrs) | 1-11 | 5-15 | 8-18 |
In 2010, according to the GPS coordinates, 119 plots of the same size as those in 2006 were arranged again at a location that either overlapped or was adjacent to the plots established in 2006, and the trees were marked with spray paint on trunk. Of these 119 plots, 54 were revisited in 2012 or 2013, and trees were inventoried again.
The dry above-ground biomass (AGB, kg) of each tree (excluding palms and lianas) was estimated with the allometric equation ([38]) using dbh (cm - eqn. 1):
This allometric equation was developed from data of 494 harvested trees in the ZF-2 experimental forest at the Instituto Nacional de Pesquisas da Amazônia, a primary forest in the central Amazon ([38]).
Statistical analysis
AGB per plot was defined as the sum of individual AGB values. By comparing the two sequential inventory data from 2010 and 2012-2013, fates of individual trees were surveyed and AGB changes due to tree growth, recruitment, and mortality were examined in each plot.
The mean AGB in each block was obtained by the bootstrap method, in which observed values from four randomly selected plots in a block during the same inventory year were added up with 5.000 replicates to calculate the mean AGB in each block. This method proved effective in controlling for the wide variation in AGB ha-1 that was caused by the huge trees present in a plot.
Because we found a significant positive correlation between time after logging and mean block-level AGB in the 2006 data but not in the 2010 or 2012-2013 data, we used a logistic growth model to represent the recovery process of AGB after selective logging (eqn. 2):
where AGB (Mg ha-1) is the mean AGB of a forest block in an inventory year; Year is the number of years after selective logging; αi is a random effect for the i-th block, following a log-normal distribution with mean 1.0, and variance was assumed to follow a uniform distribution ranging from 0 to 1.000; K, a, and b are estimated parameters where we assumed a gamma distribution with value of 0.001 for both the shape and scale parameters for K, and a normal distribution with mean 0 and variance 1.000 for a and b, respectively. Parameters were estimated by Bayesian analysis with a Markov Chain Monte Carlo (MCMC) algorithm. The MCMC procedure was run for 10.000 iterations after a burn-in of 2.000 iterations. Convergence of MCMC was validated visually using Convergence Diagnostic and Output Analysis (CODA - [32]) and Gelman & Rubin’s convergence diagnostic (<1.05) for all parameters, including the random effect ([17]). Because we had no data for the preharvest AGB of the logged blocks, we defined the AGB recovery period as the time needed for the AGB derived from the logistic growth model to reach the mean AGB of the unlogged block. Computations were performed in the JAGS 3.2.0, R 3.0.3, R2WinBUGS and rjags software.
Results
AGB increase after logging
The mean AGB per logged block ranged from 226.4 to 314.3 Mg ha-1 in 2006, 259.7-341.0 Mg ha-1 in 2010, and 244.6-342.8 Mg ha-1 in 2012-2013 (Fig. 1a). The 2006 data showed a positive significant linear correlation between time after selective logging and mean AGB in each block (r = 0.63, P = 0.0011, df = 21), but the 2010 and 2012-2013 data showed no significant relationship (P > 0.05).
Fig. 1 - Relationship between time after logging and above-ground biomass (AGB) in a managed terra firme forest in Itacoatiara, central Amazon. (a) Mean AGB of each forest block measured after selective logging. Black circle: 2006; white circle: 2010; and asterisk: 2012-2013. Solid lines join data from the same block. (b) Logistic growth curve of AGB after selective logging. Bold line, estimated logistic growth; dashed lines, the 95% credible interval; horizontal solid line, mean AGB of an unlogged block (306.4 Mg ha-1); dashed vertical line, expected AGB recovery period (14 years). See Tab. 2 for details of the logistic curve.
The mean AGB in the unlogged block was estimated to be 304.4 Mg ha-1 (240.9-368.8 Mg ha-1, 95% confidence interval [CI] by bootstrapping) in 2006, 308.2 Mg ha-1 (255.4-356.0 Mg ha-1) in 2010, and 298.9 Mg ha-1 (271.5-319.7 Mg ha-1) in 2012-2013. These results suggest that there was no significant difference (95% CI) among the mean AGBs during any survey year in the unlogged block. The overall average of AGB was 306.4 Mg ha-1 in the unlogged block.
The parameters in the logistic growth model had estimated median values of a = -0.902, b = 0.277, K = 309.2 (Tab. 2), and αi = 0.950-1.036. The estimated value of K, the upper limit of AGB growth, was very close to the mean value of the unlogged forest block (306.4 Mg ha-1), which was within the 95% credible interval of K (295.7-339.2 Mg ha-1 - Tab. 2). As indicated by the regression line, the logged forest would regain its AGB at a rate of 15.4 Mg ha-1 y-1 one year after logging, 13.1 Mg ha-1 y-1 after 2 years, 7.2 Mg ha-1 y-1 after 5 years, and 2.1 Mg ha-1 y-1 after 10 years of logging. The logistic regression curve showed that the AGB of the logged blocks would reach the mean AGB of the unlogged block (306.4 Mg ha-1) 14 years after logging; however, the 95% credible interval places the range between 9 years to infinity (Fig. 1b).
Tab. 2 - Posterior mean ± standard deviation (SD), median, 95% credibility of parameters and Gelman & Rubin’s convergence diagnostic (R) in a logistic growth model of time after logging and AGB in a managed terra firme forest in Itacoatiara, central Amazon.
Parameter | Mean ± SD | Median | 95% credibility |
R |
---|---|---|---|---|
a | -0.910 ± 0.303 | -0.902 | -1.557 to -0.345 | 1.00 |
b | 0.287 ± 0.111 | 0.277 | 0.106 to 0.539 | 1.00 |
K | 311.0 ± 11.40 | 309.2 | 295.7 to 339.2 | 1.01 |
AGB dynamics of unlogged and logged blocks
Data collected in 2010 and 2012-2013 showed that the annual increment of AGB was 3.8 ± 3.3 Mg ha-1 y-1 (mean ± SD, n = 30) in the logged plots where 5-9 years had passed since selective logging in 2010, and 2.5 ± 5.2 Mg ha-1 y-1 (n = 19) in plots after 10-15 years (Tab. 3). Although these values were not significantly different based on the 95% CI, the plots measured 5-9 years after logging showed a marginally larger AGB annual increment than plots measured 10-15 years after logging. Under both plot conditions, AGB annual increment was significantly positive. In the earlier- (5-9 years) and later-logged (10-15 years) plots, tree growth and new tree recruits both contributed to the overall AGB increment; however, the earlier-logged plots had significantly greater AGB loss due to tree mortality than the later-logged plots (Tab. 3). The unlogged block had a significant annual decrease in AGB (-4.4 ± 6.5 Mg ha-1 y-1, n = 5) due to greater AGB loss from tree mortality (-9.2 ± 7.3 Mg ha-1 y-1), when compared to the logged blocks (-3.8 ± 3.6 Mg ha-1 y-1 for all logged plots). In addition, the increased annual AGB due to tree growth was significantly smaller in the unlogged block (3.4 ± 1.3 Mg ha-1 y-1) than in the logged blocks (5.6 ± 2.3 Mg ha-1 y-1, 95% CI). However, new tree recruitment caused similar increases in AGB in both unlogged and logged blocks (1.4 ± 1.1 and 1.3 ± 1.1 Mg ha-1 y-1, respectively).
Tab. 3 - Annual AGB increment due to tree growth, recruitment, and mortality in unlogged and selectively logged Amazonian forest plots in Itacoatiara, central Amazon. Mean ± standard deviation (Mg ha-1 y-1). Values in parentheses show the 95% confidence intervals generated by the bootstrap method (5.000 replicates, BCa). Annual AGB increments were derived from inventory data from 2010 to 2012-2013 evaluations. Logged plots were classified by time after selective logging: 5-9 years and 10-15 years by 2010.
Logging | Time after logging |
Overall | Growth | Recruit | Mortality |
---|---|---|---|---|---|
Unlogged (n = 5) |
- | -4.4 ± 6.5 | 3.4 ± 1.3 | 1.4 ± 1.1 | -9.2 ± 7.3 |
(-10.5 to -0.4) | (2.2-4.2) | (0.7-2.5) | (-16.3 to -4.4) | ||
Logged | All (n = 49) |
3.0 ± 4.6 | 5.6 ± 2.3 | 1.3 ± 1.1 | -3.8 ± 3.6 |
(1.6-4.2) | (5.0-6.3) | (1.0-1.6) | (-5.0 to -2.9) | ||
5-9 years (n = 30) |
3.8 ± 3.3 | 5.3 ± 2.1 | 1.2 ± 1.0 | -2.8 ± 2.2 | |
(2.2-5.1) | (4.5-6.4) | (0.8-1.8) | (-3.8 to -1.9) | ||
10-15 years (n = 19) |
2.5 ± 5.2 | 5.7 ± 2.4 | 1.3 ± 1.1 | -4.4 ± 4.1 | |
(0.5-4.2) | (5.0-6.7) | (0.9-1.7) | (-6.2 to -3.2) |
Small to medium-sized trees (10-40 cm dbh) contributed significantly more AGB in the unlogged block (68% of the total) than in either logged block groups (53%-55%, 95% CI - Fig. 2a). In contrast, large trees (40-60 cm dbh) had significantly less AGB in the unlogged block than in the later-logged blocks (Fig. 2a). The largest tree size class (>60 cm dbh) in all block types had wider variations of AGB than smaller tree classes. The unlogged block showed a decreased AGB in all size classes up to 60 cm dbh, which was also significant in medium-sized trees with 30-50 cm dbh (95% CI - Fig. 2b). In contrast, the logged blocks showed positive AGB increments in all tree size classes, with the exception of the 20-30 cm and 50-60 cm dbh classes in the later-logged blocks. These increments were significantly positive in the smallest (10-20 cm dbh) and largest (>60 cm dbh) size classes in the later-logged blocks and in the 20-30 cm dbh class of the earlier-logged blocks (Fig. 2b).
Fig. 2 - Above-ground biomass (AGB) dynamics by diameter at breast height (dbh) size class in the unlogged and logged forest plots in Itacoatiara, central Amazon: (a) AGB (Mg ha-1) in 2010; (b) annual AGB increment (Mg ha-1 y-1) from 2010 to 2012-2013. The logged plots were classified by time after selective logging. White bars, unlogged plots (n = 5); light gray bars, logged plots 10-15 y after logging by 2010 (n = 19); dark gray bars, logged plots 5-9 y since logging (n = 30). Vertical lines represent the 95% confidence intervals generated by the bootstrap method (5.000 replicates, BCa).
Discussion
AGB stock in unlogged forest block
The estimated mean AGB of the unlogged forest block was 306.4 Mg ha-1. The logistic growth model showed that the AGB of the logged blocks would plateau at 309.2 Mg ha-1 (Tab. 2, Fig. 1b). These estimated AGB represented potential value for typical terra firme forests in Itacoatiara, but are somewhat lower than those reported for other terra firme forests in the central Amazon. For example, AGB of natural stands in some sites near Manaus reached 320-360 Mg ha-1, as indicated by ZF-2 ([46]), the Biomass and Nutrient Experiment (BIONTE - [5]), and the Biological Dynamics of Forest Fragments Project (BDFFP - [21]; summarized in Tab. 4). In addition, trees in the 10-40 cm dbh size classes in the unlogged block had a greater proportion of AGB (68% - Fig. 2a) than trees in the same classes examined in other sites, such as the natural forests in the Reserva Florestal Adolpho Ducke (42% - [10]) and ZF-2 (50% - [46]). Soil fertility and topography account for approximately 20%-30% of the variation in AGB in Amazonian terra firme forests ([21], [10]). At the landscape level in moist terra firme forests, AGB on plateaus with clayey soil tended to be higher than that in valleys with sandy soil ([10], [42]). Because our study plots were placed randomly in each block to avoid under- or overestimating AGB by favoring particular sites, the apparent AGB bias toward small and medium-sized trees might explain the low AGB measured in this study compared to reports on other forests (Tab. 4).
Tab. 4 - Above-ground biomass (AGB) and annual AGB increment in natural and selectively logged Amazonian forests. (a): Mean ± standard deviation before harvest in logged forests; (b): mean (range in parentheses due to a temporal fluctuation in the same plot or spatial variation in multiple plots); (RIL): reduced impact logging; (CL): conventional logging.
Region | Site | Natural forest | Selectively logged forest | Condition | Reference | ||
---|---|---|---|---|---|---|---|
AGB (a) (Mg ha-1) |
Annual increment of AGB (b) (Mg ha-1 y-1) |
AGB (a) (Mg ha-1) |
Annual increment of AGB (b) (Mg ha-1 y-1) |
||||
Central | ZF-2 | 360 | -0.15 | - | - | - | Vieira et al. ([46]) |
317 | - | - | - | - | Suwa et al. ([42]) | ||
BIONTE | 328 ± 18 | +1.6 (+0.7 - +2.2) |
340 ± 26 | +3.7 (+1.8 - +5.7) |
- | Chambers et al. ([5]) | |
BDFFP | 298 ± 12 | +0.6 | - | - | Partly fragmented |
Pyle et al. ([34]) | |
356 ± 47 | - | - | - | Partly fragmented |
Laurance et al. ([21]) | ||
Ducke | 306 | - | - | - | - | De Castilho et al. ([10]) | |
Itacoatiara | 306 | -4.4 | - | +3.0 | RIL | This study | |
East | Paragominas | 337 | +1.9 | 410 ± 65 | +2.6 (-3.5 - +10.6) |
RIL | Mazzei et al. ([24]) |
462 | +0.8 | ||||||
238 | -0.6 | 260 | +2.8 | RIL | West et al. ([48]) | ||
- | - | 264 | +0.5 | CL | |||
Paracou | ≈420 | +1.0 | ≈420 | (+0.8 - +3.4) | CL | Sist et al. ([40]) | |
≈440 | +1.2 | ≈440 | (-7.8 - +6.0) | CL & treatment |
|||
Nouragues | 365 | +0.4 (-1.9 - +2.3) |
- | - | - | Chave et al. ([6]) | |
380 | +2.3 (+0.9 - +3.6) |
- | - | - | |||
Tapajos | 334 ± 15 | +2.0 | - | - | Disturbed | Pyle et al. ([34]) | |
244 | (+1.0 - +1.5) | - | - | - | Vieira et al. ([46]) | ||
335 | - | - | - | - | Figueira et al. ([15]) | ||
West | Rio Branco | 281 | (+1.0 - +1.5) | - | - | - | Vieira et al. ([46]) |
Antimary | - | - | 199 | (+0.2 - +6.9) | RIL, bamboo dominated | De Oliveira et al. ([12]) |
AGB dynamics in Itacoatiara
AGB showed a relatively large annual decrease (-4.4 Mg ha-1 y-1) in the unlogged block of Itacoatiara (Tab. 4), due mainly to the mortality of medium-sized trees (30-50 cm dbh - Fig. 2b). Suwa et al. ([43]) reported that the mortality of medium trees that are 40-50 cm dbh was higher than trees in the other size classes in the unlogged forest of ZF-2, in the central Amazon. The AGB increment due to the growth of surviving trees in the unlogged block (3.4 Mg ha-1 y-1) was similar to those reported for the natural terra firme forests in ZF-2 (3.8 Mg ha-1 y-1 - [46]) and Paragominas in the eastern Amazon (3.9 and 4.3 Mg ha-1 y-1 - [24]). The AGB increment due to tree growth and recruitment in the unlogged block (3.4 + 1.4 = 4.8 Mg ha-1 y-1) was comparable to values obtained from other sites of natural forests, including BIONTE (central Amazon, 3.4 Mg ha-1 y-1 - [5]), Paragominas (5.7 and 5.3 Mg ha-1 y-1 - [24]), and Nouragues (French Guiana, eastern Amazon, 4.2 Mg ha-1 y-1 - [6]). If the annual AGB loss due to tree mortality in the unlogged block was the same as in the logged blocks (-3.8 Mg ha-1 y-1), the net annual increment in AGB would be +1.0 Mg ha-1 y-1, which falls within the range of values from natural forests in the Amazon region (Tab. 4). Therefore, excluding tree mortality, the time-frame for AGB dynamics in the unlogged forest block in Itacoatiara is consistent with other natural forests in the Amazon. Since no evidence of destructive disturbances (e.g., storm or fire) was found in the unlogged plots during fieldwork in 2013, the reason for the large decrease in AGB, or great mortality, in the unlogged block remains unclear. The overall change of AGB (-4.4 Mg ha-1 y-1) was slightly out of the range of the annual AGB increase (-4.1 to +4.6 Mg ha-1 y-1) observed in old-growth terra firme forests used for long-term monitoring in the central and eastern Brazilian Amazon ([2]).
AGB recovery in logged forest
By 5-15 years after selective logging, the net annual AGB increment in the smallest trees (10-20 cm dbh) from the later-logged blocks and the smaller trees (20-30 cm dbh) from the earlier-logged block was significantly positive (Fig. 2b). Moreover, the logged blocks had less AGB in small and medium-sized trees (10-40 cm dbh) than the unlogged block (Fig. 2a). These results indicate that the AGB of small and medium-sized trees still tend to increase in the logged forest blocks. According to our projections, the AGB of the logged forest would be almost fully restored within 14 years after logging (Fig. 1b), which is a much shorter period than the cutting cycle (i.e., 25 years) detailed in the PWA’s management plan.
Post-logging growth patterns of remaining trees differed greatly by tree size in terra firme forests in the Amazon. For example, Figueira et al. ([15]) reported that small and medium-sized trees (10-55 cm dbh) within 30 m of canopy gaps began to increase in diameter growth within several years after selective logging. On the other hand, Mazzei et al. ([24]) indicated that large trees (>60 cm dbh) had greater diameter growth than smaller trees, and substantially contributed to AGB growth during the early recovery stage. The significant increase in AGB in the smallest and largest trees in the later-logged blocks of our study site (Itacoatiara) did not correspond with the patterns of these two previous studies (Fig. 2b). Conversely, West et al. ([48]) reported that smaller and larger trees had decreased AGB 16 years after selective logging, whereas medium-sized trees (20-60 cm dbh) contributed to AGB restoration. More information of the relationship between individual-level growth response and the overall recovery process of AGB is required to understand the factors causing such variation in post-logging biomass recovery in the Amazon Basin.
Our logistic growth model anticipated an average AGB recovery period of 14 years after selective logging at a harvest intensity of 1.9 trees ha-1. The expected annual increment in AGB 8-10 years after logging (2.1-3.5 Mg ha-1 y-1) was very close to the observed values 10-15 years (2.5 Mg ha-1 y-1) and 5-9 years (3.8 Mg ha-1 y-1) after logging (Tab. 3). These expected and observed annual increments in AGB were also equivalent to the mean value (2.7 Mg ha-1 y-1) that was estimated from the data of 79 permanent sample plots across the Amazon Basin ([36]). Tab. 5 summarizes available data of the relationship between logging intensity and estimated AGB recovery period from previously studies that examined in terra firme forests in the Amazon. Here, both conventional logging and RIL were included for reviewing effect of logging intensity on AGB recovery period. A selectively logged terra firme forest in Paragominas, east Amazon, took 16 years to regain its original AGB after harvesting at an intensity of 4.5 trees ha-1 using the RIL technique ([48] - Tab. 5). Likewise, a simple simulation calculated an AGB recovery period of 15 years for terra firme forests in Paragominas that selectively logged at 3 trees ha-1 ([24]). Our projection of the AGB recovery period (14 years) agrees with these results from the forests of eastern Amazon, suggesting that Amazonian terra firme forests could regain their original AGB stock within a dozen years after selective logging at an intensity of several trees ha-1 and proper logging techniques. However, there are some exceptions for the AGB recovery period. A bamboo-dominated rain forest in Antimary, west Amazon, recovered its AGB within only 4 years after selective logging at 6.9 m3 ha-1 ([12]), equivalent to 1.1 trees ha-1 logging (Tab. 5). This quick recovery appeared to be caused by the disappearance of the dominant bamboo because of the specific logging operation and rapid growth of residual trees around the gaps ([12]). The recovery period would be drastically prolonged if further intensified harvesting or conventional logging techniques were applied; for example, a simple simulation predicted that a logging intensity of 6 or 9 trees ha-1 would require 51 or 88 years of AGB recovery, respectively ([24]). Blanc et al. ([3]) similarly reported that the recovery period in a terra firme forest would extend to 45 years if a strong harvest (10.4 trees ha-1) was conducted using conventional logging techniques. These comparisons of AGB recovery period suggest that logging intensity should be limited to ≤5 trees ha-1 to ensure sustainable forest management while minimizing carbon emissions. According to the formula provided by Rutishauser et al. ([36]), harvesting 5 trees ha-1 (approximately 31.5 m3 ha-1) would result in a 16.7 % loss of AGB under RIL management and 22.5 years for recovery to the initial AGB level. A logging intensity of ≤5 trees ha-1 and AGB recovery period of 22.5 years would be acceptable to forest managers.
Tab. 5 - Selective logging intensity and AGB recovery period in Amazonian terra firme forests. (a): Estimated from timber volume based on an average log volume of 6.3 m3 log-1 in the Brazilian Amazon region ([44], [13]). (b): A model for total wood volume.
Region | Site | Logging intensity (n ha-1) |
Recovery period (yrs) |
Condition | Reference |
---|---|---|---|---|---|
Central | Itacoatiara | 1.9 | 14 | RIL, model | This study |
East | Paragominas | 3 | 15 | RIL, model | Mazzei et al. ([24]) |
6 | 51 | ||||
9 | 88 | ||||
4.5 | 16 | RIL, observed | West et al. ([48]) | ||
5.6 | >16 | Conventional, observed | |||
10.3 (a) | 10 | RIL, model (b) | Valle et al. ([45]) | ||
- | 35-40 | Conventional, model | |||
Paracou | 10.4 | 45 | Conventional, model | Blanc et al. ([3]) | |
20.6 | >100 | ||||
West | Antimary | 1.1 (a) | 4 | RIL, observed, bamboo dominated | De Oliveira et al. ([12]) |
Conclusions
Our study was a first trial to quantitatively evaluate RIL operated in tropical forests of the central Amazon. Comparisons with previous studies mainly conducted in other regions of the Amazon suggested that logging intensity and the estimated AGB recovery period were appropriate and likely acceptable parameters for forest management. However, maintaining tree biomass and healthy biodiversity are prerequisites for ecologically sound forest management. This study examined the process of biomass recovery in only living trees. Even after clear-cutting, AGB of trees in an Atlantic forest in Brazil recovered to a level equivalent to that of nearby preserved forest within 60 years ([22]). Carbon pools recovered more quickly than tree and epiphyte species richness in tropical secondary forests ([23]). Organic carbon stocks in soil also remained at a lower level than that measured in unlogged forest 45-50 years after selective logging ([8]). Furthermore, the post-logging timber species composition and total economic value of forest stands did not recover beyond the first cut in Para state, Brazil ([35]). Abiotic alterations from selective logging, that is, changes in light and soil conditions, raised mortality rates of major tree species in the central Amazon ([9]). Therefore, recovery not only of carbon pools but also of species composition for various taxa, and the regeneration of timber species should be further examined in order to propose a method of RIL that could achieve truly sustainable selective logging in the forests of the central Amazon.
Acknowledgments
The authors are indebted to Precious Woods Amazon Ltd. in Itacoatiara for their kind understanding and cooperation during our study, and to the Japan International Cooperation Agency (JICA) office in Manaus for its expert management of the research project. We thank the members of the international cooperative research project “Carbon Dynamics of Amazonian Forests” for their energetic support. We also thank Antonio Raimundo Gomes de Souza, Wanderley de Lima Reis, and Manoel Tiago Martins da Silva for their powerful support in our field work. Financial support was provided by the Instituto Nacional de Pesquisas da Amazônia (INPA) and the Science and Technology Research Partnership for Sustainable Development (SATREPS) program funded by the Japan Science and Technology Agency (JST) and JICA. All field activity in 2006 was performed by the INPA project, and the surveys in 2010 and 2012-2013 were conducted by the JST-JICA project.
References
CrossRef | Gscholar
Gscholar
Gscholar
Gscholar
CrossRef | Gscholar
Gscholar
CrossRef | Gscholar
CrossRef | Gscholar
Gscholar
Authors’ Info
Authors’ Affiliation
Shikoku Research Center, Forestry and Forest Products Research Institute (FFPRI), Kochi (Japan)
Marcio RM Amaral
Joaquim Dos Santos
Niro Higuchi
National Institute of Amazonian Research, Manaus, Amazonas (Brazil)
Kansai Research Center, FFPRI, Kyoto (Japan)
Federal University of Amazonas, Manaus, Amazonas (Brazil)
Corresponding author
Paper Info
Citation
Otani T, Lima AJN, Suwa R, Amaral MRM, Ohashi S, Pinto ACM, Dos Santos J, Kajimoto T, Higuchi N, Ishizuka M (2018). Recovery of above-ground tree biomass after moderate selective logging in a central Amazonian forest. iForest 11: 352-359. - doi: 10.3832/ifor2534-011
Academic Editor
Rodolfo Picchio
Paper history
Received: Jun 25, 2017
Accepted: Feb 08, 2018
First online: May 04, 2018
Publication Date: Jun 30, 2018
Publication Time: 2.83 months
Copyright Information
© SISEF - The Italian Society of Silviculture and Forest Ecology 2018
Open Access
This article is distributed under the terms of the Creative Commons Attribution-Non Commercial 4.0 International (https://creativecommons.org/licenses/by-nc/4.0/), which permits unrestricted use, distribution, and reproduction in any medium, provided you give appropriate credit to the original author(s) and the source, provide a link to the Creative Commons license, and indicate if changes were made.
Web Metrics
Breakdown by View Type
Article Usage
Total Article Views: 40584
(from publication date up to now)
Breakdown by View Type
HTML Page Views: 35111
Abstract Page Views: 2118
PDF Downloads: 2669
Citation/Reference Downloads: 12
XML Downloads: 674
Web Metrics
Days since publication: 2276
Overall contacts: 40584
Avg. contacts per week: 124.82
Article Citations
Article citations are based on data periodically collected from the Clarivate Web of Science web site
(last update: Feb 2023)
Total number of cites (since 2018): 6
Average cites per year: 1.00
Publication Metrics
by Dimensions ©
Articles citing this article
List of the papers citing this article based on CrossRef Cited-by.
Related Contents
iForest Similar Articles
Research Articles
Simplified methods to inventory the current annual increment of forest standing volume
vol. 5, pp. 276-282 (online: 17 December 2012)
Research Articles
Genetic control of intra-annual height growth in 6-year-old Norway spruce progenies in Latvia
vol. 12, pp. 214-219 (online: 25 April 2019)
Research Articles
Effects of mixture and management on growth dynamics and responses to climate of Quercus robur L. in a restored opencast lignite mine
vol. 15, pp. 391-400 (online: 05 October 2022)
Research Articles
A fast screening approach for genetic tolerance to air pollution in Scots pine field tests
vol. 6, pp. 262-267 (online: 01 July 2013)
Research Articles
Scots pine’s capacity to adapt to climate change in hemi-boreal forests in relation to dominating tree increment and site condition
vol. 14, pp. 473-482 (online: 18 October 2021)
Research Articles
Short-term effects in canopy gap area on the recovery of compacted soil caused by forest harvesting in old-growth Oriental beech (Fagus orientalis Lipsky) stands
vol. 14, pp. 370-377 (online: 10 August 2021)
Research Articles
Trends and driving forces of spring phenology of oak and beech stands in the Western Carpathians from MODIS times series 2000-2021
vol. 16, pp. 334-344 (online: 19 November 2023)
Research Articles
Post-fire recovery of Abies cephalonica forest communities: the case of Mt Parnitha National Park, Attica, Greece
vol. 11, pp. 757-764 (online: 15 November 2018)
Research Articles
Indicators for the assessment and certification of cork oak management sustainability in Italy
vol. 11, pp. 668-674 (online: 04 October 2018)
Research Articles
Forest and tourism: economic evaluation and management features under sustainable multifunctionality
vol. 2, pp. 192-197 (online: 15 October 2009)
iForest Database Search
Search By Author
Search By Keyword
Google Scholar Search
Citing Articles
Search By Author
Search By Keywords
PubMed Search
Search By Author
Search By Keyword