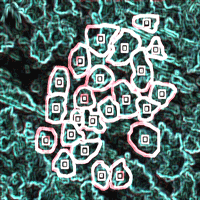
Estimating crown defoliation of Scots pine (Pinus sylvestris L.) trees using small format digital aerial images
iForest - Biogeosciences and Forestry, Volume 6, Issue 1, Pages 15-22 (2013)
doi: https://doi.org/10.3832/ifor0705-006
Published: Jan 14, 2013 - Copyright © 2013 SISEF
Research Articles
Collection/Special Issue: IUFRO 7.01.00 - COST Action FP0903, Kaunas (Lithuania - 2012)
Biological Reactions of Forest to Climate Change and Air Pollution
Guest Editors: Elena Paoletti, Andrzej Bytnerowicz, Algirdas Augustaitis
Abstract
This study focuses on the possibilities of using small format digital aerial images for the estimation of tree crown condition. The test area was located in the eastern part of Lithuania where Scots pine (Pinus sylvestris L.) trees prevail and was photographed using a Canon EOS-1DsMark II digital camera installed on-board a SkyArrow ultra-light aircraft. The camera lenses were adopted to capture images corresponding to conventional color-infrared photography. In addition, the test area was photographed using a large format digital frame aerial camera (Vexcel UltraCam D) installed on board a Rockwell Turbo Commander 690A high performance commuter aircraft. The ground sampling density of the images taken was around 9-10 cm. Crown defoliation was assessed in the field for more than 500 Scots pine trees located in 46 sample plots representing stands of trees that were either 65 years old or 170 years old. Spearman’s correlations coefficients were used to check for relationships between tree crown defoliation and image characteristics. The defoliation was also predicted using the non-parametric k-Nearest Neighbor method applied on data available from aerial images alone. The results were validated using the “Leave One Out” technique by comparing the obtained data with data from the field assessed defoliation rates. The prediction root mean square errors were calculated using data from the small format aerial images as being 11.5% for the younger trees, whereas those calculated using conventional aerial images were between 9.5 and 9.9%. The differences in predicted root mean square errors disappeared in the older stands and both methods produced errors of between 8.1 and 8.5%. Defoliation class was correctly predicted for approximately 84-88% of the older tree crowns and correctly for 75-85% of the younger tree crowns. These results showed that small format aerial images had the potential to predict defoliation in tree crowns and were comparable with results obtained using conventional aerial images. Their main advantage is that small format images are much cheaper to obtain than conventional images when the areas targeted are thousands of hectares in size.
Keywords
k-Nearest Neighbor, Small Format Aerial Images, Tree Crown Defoliation, Ultra-light Aircraft
Introduction
Tree crown defoliation or foliage transparency is the most important indicator of tree health today, especially when the potential of forests to mitigate and adapt to climate change is becoming increasingly more relevant. However, visual assessment of the crown defoliation rate is seen as being unreliable due to the subjective nature of the observation involved ([20]), as well as the crown defoliation is questioned as an adequate indicator of forest condition ([28]) and this has resulted in a large number of suggested reasons as to why there has been a general decline in the overall condition of forests. A lack of long term studies based on a fully standardised methodology has led to increased uncertainty in this field ([30]). Despite this, visual estimations of defoliation are the basis of methodological frameworks adopted by various trans-national and national forest health monitoring programs, e.g., the European International Co-operative Program on Assessment and Monitoring of Air Pollution Effects on Forests (ICP-Forests) that has been operational since 1986 ([56], [28]) or the Lithuanian National forest inventory ([32]). It has been also shown that the field collection of forest defoliation data is an expensive and time consuming procedure, resulting in spatially incomplete sampling ([39]). Therefore, the aim of our study in general is to find more objective indicators of forest health and remote sensing based techniques to facilitate forest health assessment.
Deteriorating forest health normally leads to defoliation and/or changes in foliage color ([6], [18]), reduced tree crown parameters and changes in the concentrations of chlorophyll and other pigments ([27]) and in the leaf or needle structure. Foliage reflectance in the visible region of the electromagnetic spectrum is known to be mainly related to absorption by photosynthetic pigments, such as chlorophylls a and b and other accessory pigments ([21]), while the near infrared reflectance is influenced by multiple refractions of radiation along cell wall-water-air interfaces due to differences in refractive indices associated with their foliar components ([22]). Damage, usually accompanied by defoliation, causes changes in the reflectance of tree crowns, especially in the near-infrared region ([33], [16], [5], [19], [10]). Furthermore, defoliation decreases the contrast between the tree crowns and ground surface ([23]). This means that changes in forest health conditions affect properties that can be analyzed by remote sensing techniques.
The role of remote sensing in forest health assessment has been acknowledged in numerous research and technical publications ([13], [51], [57]). Satellite image based forest health assessment is a widely-used technique for large-scale surveys ([14], [26], [58], [4], [25]). The importance of satellite remotely sensed images in the cartographic modeling of tree crown defoliation at a relatively local level has been previously reported in Lithuania ([7]). That study found that SPOT (Satellite Pour l’Observation de la Terre) multispectral images (XS), used along with other parameters derived from forest geographic databases and panchromatic orthophotos in multiple linear regression models, improved the sample plot level prediction of tree crown defoliation by 10%. However, the use of satellite remote sensing for forest health assessment at a local level has several key limitations: the spatial resolution of satellite images depends on the satellite sensor type, acquisition of timely imagery may be too expensive, if not impossible, due to natural reasons, e.g., cloud cover. Aerial photography offers higher spatial and temporal resolutions and has been widely applied for forest health inventories ([31], [57]). Methods used to extract forest health related attributes from aerial images range from visual interpretation to fully automated processing ([23]). The potential to detect crown defoliation classes corresponding to the ones used by UN-ECE/ICP-Forests for pine and spruce stands using visual analytical interpretation of color-infrared (CIR) aerial photographs at a scale 1:10000 in Lithuania has been reported by Daniulis & Mozgeris ([17]). More recently, Augustaitis et al. ([9]) reported on the potential to assess tree crown defoliation from color-infrared images using a Leica RC30 film camera and Kodak Aerochrome III Infrared 1443 aerial film at a nominal scale of 1:10000. To date, the research trends in remote sensing aided forest health assessment have been towards using modern data acquisition techniques, such as laser and hyperspectral scanning ([52], [35], [48], [11], [12]). However, conventional aerial photography, laser and hyperspectral scanning, etc., all share a common limitation: the relatively high costs associated with data acquisition and processing, especially when relatively small areas need to be inventoried.
Small Format Aerial Photography (SFAP) traditionally is referred to using 35 mm and 70 mm cameras for image acquisition ([13]). SFAP techniques have found some applications in forestry investigations and assessments over the past few decades ([3], [2]). However, their use in forest health applications remains at the level of tests and demonstrations ([13]). Nevertheless, SFAP is considered as a potentially effective and inexpensive tool to assess the health condition of trees and forests ([2], [36], [1]).
The 36x24 mm CMOS (Complementary Metal Oxide Semiconductor) sensor of the Canon EOS-1DsMark II digital camera installed on-board the ultra-light SkyArrow aircraft used in this study includes the limitations of SFAP: a relatively small land area covered by a single image and a limited number of distinguishing objects for photogrammetric processing. However, the costs associated with small format images are much less than those associated with conventional color-infrared aerial photography used in Lithuanian stand-wise forest inventory ([45]), especially if relatively small areas are to be flown. Recently, orthophotos developed using the same small format imaging system as described in this paper were found to be suitable for solving tasks connected with the conventional stand-wise forest inventory in Lithuania ([44]).
The aim of the current study was to investigate the potential for using small format digital aerial images to estimate Scots pine (Pinus sylvestris L.) crown defoliation. The research was based on a comparison of the relationships between various image characteristics and crown defoliation as well as the defoliation prediction accuracies achieved using small format digital aerial images and images captured using a large format digital frame aerial camera.
Material and methods
Ažvinčiai forest in Aukštaitija National park (26° E, 55.4° N) was chosen as the study area (Fig. 1). The forest contains multi-aged and multi-layered mature and over mature pine and spruce stands growing on haplic arenosol soils, which become albic and gleyic arenosols in lower areas. In the forest areas the soil is a histosol (eutrophic deep peat soil). The climate is characterized as being moderately cold with high humidity and abundant precipitation. Annual mean air temperature is 5.8ºC, mean annual precipitation is 682 mm and the vegetation growth period is 189 days ([9]).
The test area was photographed using a Canon EOS-1DsMark II digital camera, installed on-board a SkyArrow ultra-light aircraft on August 1, 2008. The camera lenses were adopted to capture images corresponding to conventional color-infrared photography, i.e., the resulting image bands represented near infrared, red and green spectral regions. The flights were carried out at 300 m altitude above ground surface. Side overlap was 50%, forward overlap 75%, flying speed was 150 km h-1, average image size on the ground was 469x313 m and the ground sampling density was 9 cm. An EnsoMOSAIC system was used for flight management, aerial triangulation and image rectification. “Digipreprocess” software was used for image conversions and spectral enhancement ([50]). Only 16 images forming a block around the forest compartments used in the study were ortorectified. 4 ground control points on the corners and 1 in the middle of the block, manual tie point collection during the aerial trianguliation and no digital elevation model were utilized. Hereafter, these images are referred to as “small format digital aerial images”.
The same area was photographed using a large format digital frame aerial camera (Vexcel UltraCam D) on September 14, 2008. The camera system included electronic forward movement compensation and RGB and CIR image capture took place simultaneously. A Rockwell Turbo Commander 690A high performance commuter aircraft was used to carry out the flights. Flying altitude was around 1100 m. The side and forward overlaps were 40% and 80%, respectively, resulting in an image ground sampling density of around 10 cm and average image size on the ground of 1150x750 m. The camera unit was equipped with an Applanix POS/AV 510 GPS/INS system. A ground-based GPS-receivers receiver was also used in order to achieve all six parameters of image exterior orientation. Raw image data from the camera were processed into final TIFF images using Office Processing Centre software from Vexcel Imaging by Blom Kartta Oy, Finland. Only 3 images based on near-infrared, red and green spectral bands were used for this study. The technical characteristics of this type of aerial photography are similar to the ones for standard forest inventory aimed aerial photography in Lithuania (except the ground sampling density, which is around 40-50 cm). Hereafter, these images are referred to as “conventional digital aerial images”.
Crown condition in the field was assessed in August, 2008, on 4 Permanent Observation Stands (POS), established in 1994. Each POS (see Tab. 1 for detailed characteristics) initially included 12 circular sample plots with on average 15-20 trees in each plot, i.e., about 150-200 sample trees per stand ([29], [8]). In the current study, data from 46 sample plots representing middle aged (age 65 years) and over mature (age 170 years) stands were used - Fig. 1. Tree crown defoliation for the trees observed had been visually assessed annually for the previous decade by the same two people, thus minimizing the influence of human factors on the ground reference material. Historical records on the defoliation estimates for all trees under the investigation were available and used by one of the specialists, too. The ICP Forest monitoring methodology was employed to assess tree defoliation ([56]). Additionally, data on tree and stand parameters (mean diameter and height of trees, tree density, basal area and tree volume per hectare) were available.
Tab. 1 - Characteristics of Permanent Observation Stands (POS) used in the study.
Age, years | Number of | Soil type | Volume per ha (m3) | Relative basal area | Mean height (m) | Mean diameter (cm) | Defoliation | ||
---|---|---|---|---|---|---|---|---|---|
Sample plots | Trees | Mean (%) | Standard deviation | ||||||
170 | 24 | 391 | Nbl | 380 | 0.7 | 29 | 38 | 16.9 | 8.1 |
65 | 22 | 147 | Nbl | 220 | 0.7 | 20 | 20-22 | 20.3 | 13.5 |
Only Scots pine (Pinus sylvestris L.) trees were used in the study. The field surveyed trees were identified on both the “small format” and “conventional” aerial images simultaneously with the assessment of tree crown defoliation. Crown projections of each identified tree were manually digitized on the aerial images and stored in a GIS database. Only the central parts of the image were used for tree identification and digitalization. Each of the trees selected had its corresponding characteristics measured from the ground as well as from the air.
The following image characteristics were extracted for the zones, corresponding to each crown identified, using the Zonal Statistics tool of ArcGIS software: mean, majority, maximum, median, minimum, minority, range, standard deviation, sum and variety of digital numbers for each image band. Three types of zones were used in the study to separate sun illuminated parts from the shade parts of the crown and to facilitate the manual crown identification (Fig. 2): (i): zones corresponded to the delineated crown projection polygons (ZONES_1); (ii): the interior area of tree crown polygons was divided into two parts using unsupervised Iso Cluster classification, potentially corresponding to sun illuminated parts (ZONES_2a) and shaded parts of the crown (ZONES_2b); and (iii): the crown projection centroid was located and a 15x15 cell window was generated around the centroid (ZONES_3). Image characteristics coming from sun illuminated and shaded parts of the crown were also used simultaneously to predict the crown defoliation - this type of zones is further referred to as ZONES_2ab. The following types of digital images were used: original images as 3-band grid stacks and transformed images. Two types of image transformation were used: principal component transformation (PC), where all three principal components were used; and the normalized difference vegetation index (NDVI) based on the near infrared and red original image bands.
Fig. 2 - The three types of zones used to extract image characteristics. (A): ZONES_1; (B): ZONES_2a, ZONES_2b; and (C): ZONES_3.
Several criteria were used to judge the potential for aerial images to estimate tree crown defoliation. First, Spearman’s rank order correlations were calculated to check the relationships between tree crown defoliation and the selected image characteristics (range, mean and the standard deviation of digital numbers for each image band). Next, defoliation was predicted based on data available just from aerial images and compared with the true, ground estimated parameters. Defoliation was predicted at the single crown level.
The non-parametric k-Nearest Neighbour (k-NN) method was used to predict defoliation for each crown. The k-nearest neighbour method ([53]), or the multi-dimensional version of inverse distance weighted technique, can be briefly described as follows. Some trees in a sample are inspected in-situ by estimating the defoliation. All the crowns are then divided into two groups: A-observations and B-observations. Both input (auxiliary, e.g., aerial image characteristics) and output (defoliation) data are known for the B-observations but only input (auxiliary) data are known for the A-observations. The crown defoliation can be predicted on the basis of auxiliary aerial image information for all A-observations by utilizing knowledge on the relationships between auxiliary and in-situ information developed using the B-observations. Euclidean distance di,p is then calculated between each A-observation tree crown p in n dimensional feature space of the auxiliary information and the B-observation unit i with field measured defoliation. The letter n refers to the total number of layers of auxiliary information - all proper image characteristics for all image bands or principal components and the area of the zone, corresponding to each crown. Values for k (10 was used in this study, i.e., the 2nd-11th nearest neighbours, as the 1st neighbor was always the tree crown that was being inspected) distances (di,p - d(1),p ... d(k),p, d(1),p ≤ ... ≤ d(k),p) are found and the weight is calculated as (eqn. 1):
Value of defoliation for tree crown p of A-observation equals (eqn. 2):
where m(j),p with j=1,...k are the values of tree crown defoliation of k nearest B-observation tree crowns to p.
Defoliation was also predicted as a defoliation class (1: defoliation 0-10 %; 2: 10-25 %; 3: 25-60 %; 4: 60-95 %; and 5: 95-100 %). The defoliation class for the tree being inspected was assigned as the (i) modal or (ii) median class value of the 2nd-11th nearest neighbor tree crowns.
As all trees had the “true” defoliation estimate from the field assessment, this value was compared to the predicted defoliation in order to validate the prediction accuracy, i.e., the “Leave One Out” technique was used to compute validation statistics. The following validation statistics were calculated: bias, root mean square error (RMSE) and correlation coefficient (R) between the true and the predicted defoliation for percentage defoliation and the percentage of crowns with a correctly predicted defoliation class ([34]).
The Most Similar Neighbor ([38], [15]) program was used for the k-NN prediction. Standard GIS, photogrammetric and statistical processing packages (ArcGIS®, EnsoMosaic, Statistica®, MS Excel®, etc.) were used to prepare, manipulate and analyze the input and output data.
Results and discussion
Of the trees measured in the field plots, 89 % were identified on the small format aerial images. Average defoliation value of the identified trees was less by 0.5 compared to one of all trees. Ninety seven percent of the trees were identified on the conventional aerial images, resulting in an average defoliation value less by 0.6 compared to one of all trees. As usual, smaller trees of the 5th Kraft class (suppressed) were not identified on the images.
Spearman’s correlation coefficients between tree crown defoliation and selected image characteristics are displayed in Tab. 2. Just three image characteristics out of 10 were used in this study: range, mean and the standard deviation of digital numbers for each image band. Only weak or none pair-wise correlations were observed between tree crown defoliation and various other characteristics available from the small format aerial images. Conventional aerial images produced larger correlations than the small format aerial images, especially in the younger (65 years old) stands. Relatively largest correlations were detected using image characteristics extracted for sun illuminated parts of the crowns. The mean values of the digital numbers of the image cells belonging to the delineated tree crown zones was the most correlated image characteristic, especially for conventional images. Larger correlations were detected for image bands referring to the near infrared spectral region, potentially due to the reflectance properties of differently defoliated tree crowns. However, this tendency was better seen using the conventional images, too. Image transformations into principal components and normalized difference vegetation indexes did not increase the correlations. Practically no significant correlations were observed for the image statistics when the crown shadow and 15x15 cells around the crown centroid areas were used as the analysis zones. Correlation coefficients for the small format aerial images were nearly 1.5 times less than the ones observed in a previous investigation located near the study area but using 15 x 15 cm resolution color-infrared images from a Leica RC30 frame camera ([9]). Conventional aerial images resulted in up to 20% higher correlations compared to the study mentioned above. Worth to note, that no separation of sun illuminate part of the crown was used in that study.
Tab. 2 - Spearman rank order correlations between tree crown defoliation and image characteristics. For each image band or transformation type, upper number (in plain text) refers to small format aerial images, lower number (in italic) refers to conventional aerial images. (*): p < 0.05.
Age, years | Image band or transf. type | Types of zones used to extract image characteristics | |||||||||||
---|---|---|---|---|---|---|---|---|---|---|---|---|---|
ZONES_1 | ZONES_2a | ZONES_2b | ZONES_3 | ||||||||||
Range | Mean | STD | Range | Mean | STD | Range | Mean | STD | Range | Mean | STD | ||
65 | NIR band |
-0.232* | -0.191* | -0.202* | -0.266* | -0.240* | -0.240* | -0.078 | -0.101 | -0.059 | -0.033 | -0.059 | -0.001 |
-0.287* | -0.495* | -0.277* | -0.345* | -0.572* | -0.288* | -0.031 | -0.333* | -0.037 | 0.334* | -0.504* | 0.237* | ||
Red band |
-0.200* | -0.181 | -0.131 | -0.185* | -0.225* | -0.174* | 0.015 | -0.077 | 0.004 | -0.012 | -0.055 | 0.003 | |
-0.254* | -0.405* | 0.255* | -0.368* | -0.330* | -0.320* | -0.100 | -0.193* | 0.089 | 0.236* | -0.384* | 0.183* | ||
Green band |
-0.187* | -0.192* | -0.150 | -0.247* | -0.262* | -0.216* | 0.014 | -0.111 | -0.007 | 0.016 | 0.035 | 0.020 | |
0.340* | -0.379* | -0.415* | -0.416* | -0.416* | -0.347* | -0.013 | -0.267* | 0.044 | 0.078 | -0.451* | 0.017 | ||
170 | NIR band |
-0.240* | -0.137* | -0.275* | -0.181* | -0.321* | -0.159* | -0.108* | 0.021 | -0.082 | -0.010 | 0.115* | -0.029 |
-0.276* | -0.180* | -0.347* | 0.019 | -0.466* | -0.020 | -0.203* | -0.044 | -0.190* | 0.086 | -0.216* | 0.035 | ||
Red band |
-0.160* | -0.078 | -0.215* | -0.207* | -0.207* | -0.138* | -0.019 | 0.093 | 0.017 | 0.003 | 0.114* | 0.006 | |
-0.081* | -0.032 | -0.192* | -0.094 | -0.056 | -0.069 | 0.026 | 0.185* | 0.091 | 0.090 | -0.056 | 0.045 | ||
Green band |
-0.162* | -0.081 | -0.216* | -0.192* | -0.219* | -0.116* | -0.015 | 0.084 | -0.021 | 0.077 | 0.103* | 0.036 | |
-0.065* | -0.101 | -0.186* | -0.054 | -0.160* | -0.022 | -0.087 | 0.107* | 0.054 | 0.098 | 0.098 | 0.014 | ||
65 | PC1 | -0.205* | -0.185* | -0.161 | -0.227* | -0.242* | -0.203* | -0.174 | 0.064 | -0.154 | 0.002 | 0.055 | 0.009 |
-0.335* | -0.467* | -0.316* | -0.408* | -0.485* | -0.373* | 0.110 | -0.274* | 0.038 | 0.257* | -0.455* | 0.170* | ||
PC2 | -0.310* | -0.102 | -0.167 | -0.381* | -0.196* | -0.231* | -0.003 | 0.106 | 0.085 | 0.185* | 0.096 | 0.133 | |
-0.222* | -0.139 | -0.058 | -0.240* | -0.130 | -0.029 | -0.199* | -0.191* | -0.167 | 0.095 | -0.119 | 0.166 | ||
PC3 | -0.101 | -0.248* | -0.019 | -0.179* | -0.320* | -0.001 | -0.217* | -0.112 | -0.059 | -0.188* | -0.195* | -0.195* | |
-0.373* | -0.373* | -0.314* | -0.355* | 0.176* | -0.277* | -0.103 | -0.131 | -0.051 | -0.240* | -0.285* | -0.284* | ||
170 | PC1 | -0.194* | -0.095 | -0.245* | -0.176* | -0.250* | -0.250* | 0.005 | -0.006 | 0.024 | 0.028 | 0.109* | 0.002 |
-0.156* | -0.103* | -0.276* | -0.035 | -0.311* | -0.053 | -0.118* | 0.040 | -0.063 | 0.099 | -0.138 | 0.037 | ||
PC2 | -0.044 | -0.239* | 0.034 | -0.120* | -0.120* | -0.026 | 0.089 | 0.185* | 0.292* | 0.284* | 0.155* | 0.334* | |
0.030 | -0.128* | 0.062 | 0.007 | -0.130* | 0.109* | 0.010 | -0.117 | 0.145 | 0.104* | -0.099 | 0.120* | ||
PC3 | -0.052 | -0.066 | -0.023 | -0.109* | -0.105* | -0.013 | -0.492* | -0.246* | -0.392* | -0.356* | -0.240* | -0.417* | |
-0.043 | 0.148* | -0.010 | -0.042 | 0.112* | -0.005 | -0.142* | 0.178 | 0.002 | 0.048 | 0.067 | 0.011 | ||
65 | NDVI | -0.105 | 0.121 | 0.092 | -0.001 | -0.044 | 0.238* | -0.078 | 0.081 | -0.041 | 0.045 | -0.002 | -0.038 |
-0.136 | -0.090 | 0.186* | -0.236* | -0.162 | 0.043 | -0.128 | -0.190* | 0.011 | 0.303* | -0.077 | 0.345* | ||
170 | NDVI | 0.024 | -0.116* | 0.081 | -0.093 | -0.038 | 0.191* | -0.081 | 0.184* | 0.187* | 0.055 | 0.045 | 0.077 |
-0.008 | -0.285 | 0.127* | 0.062 | -0.340* | 0.197* | -0.008 | -0.231* | 0.065 | 0.204* | -0.270* | 0.231* |
There were two levels of tree crown defoliation prediction bias, depending on the age of stands being analyzed (Tab. 3). Predicted defoliation was overestimated by approximately 1% in the 65 year old trees and underestimated by 3-4% in the 170 year old trees. Conventional aerial images resulted in a smaller bias than the small format aerial images on the older stands. Prediction root mean square errors fluctuated depending on the prediction approaches, such as image transformations or the type of zone used to extract image characteristics. Taking the best performing prediction settings, the root mean square errors achieved using small format aerial images for younger trees were around 11.5% and the ones for conventional aerial images were 9.5-9.9%. However, the differences in prediction root mean square errors practically disappeared in the older stands. The best achieved root mean square errors were 8.1-8.5%. Sometimes the small format aerial images produced better results than the conventional images.
Tab. 3 - Defoliation prediction accuracies using the non-parametric k-Nearest Neighbor estimation technique at a single crown level. For each image transformation, upper number (in plain text) refers to small format aerial images, lower number (in italic) refers to conventional aerial images.
Age, years | Image transf. | Zone types used to extract image characteristics | ||||||||||||||
---|---|---|---|---|---|---|---|---|---|---|---|---|---|---|---|---|
ZONES_1 | ZONES_2A | ZONES_2B | ZONES_2AB | ZONES_3 | ||||||||||||
Bias | RMSE | R | Bias | RMSE | R | Bias | RMSE | R | Bias | RMSE | R | Bias | RMSE | R | ||
65 | No | -1.16 | 12.11 | 0.18 | 0.16 | 12.08 | 0.24 | -1.26 | 12.73 | 0.04 | -1.06 | 12.27 | 0.14 | 0.24 | 12.99 | 0.07 |
-0.89 | 10.19 | 0.31 | -0.79 | 9.76 | 0.40 | -0.92 | 10.71 | 0.14 | -1.10 | 9.93 | 0.35 | -0.76 | 9.81 | 0.40 | ||
PC | -1.28 | 11.64 | 0.29 | -0.80 | 11.44 | 0.35 | -1.01 | 12.65 | -0.01 | -0.95 | 11.52 | 0.31 | -0.87 | 12.58 | 0.02 | |
-1.44 | 10.05 | 0.36 | -0.80 | 9.79 | 0.38 | -1.84 | 10.79 | 0.08 | -1.39 | 9.84 | 0.39 | -1.56 | 9.71 | 0.44 | ||
NDVI | -1.50 | 12.10 | 0.18 | -0.94 | 12.24 | 0.15 | -0.11 | 11.91 | 0.24 | -0.13 | 12.47 | 0.11 | 0.39 | 13.12 | -0.07 | |
-0.89 | 9.92 | 0.37 | -1.08 | 9.75 | 0.40 | -0.77 | 10.90 | 0.11 | -1.38 | 9.75 | 0.40 | -1.10 | 9.46 | 0.47 | ||
170 | No | 3.49 | 9.39 | 0.21 | 3.55 | 9.39 | 0.27 | 4.33 | 9.74 | 0.22 | 3.99 | 9.45 | 0.27 | 4.02 | 10.11 | 0.10 |
3.22 | 8.82 | 0.28 | 2.88 | 8.15 | 0.33 | 3.39 | 8.80 | 0.26 | 2.84 | 8.19 | 0.35 | 3.61 | 9.44 | 0.10 | ||
PC | 3.47 | 8.96 | 0.31 | 3.60 | 8.91 | 0.35 | 2.60 | 8.06 | 0.45 | 2.71 | 8.40 | 0.38 | 3.57 | 8.80 | 0.40 | |
3.32 | 8.44 | 0.33 | 3.25 | 8.49 | 0.30 | 3.58 | 8.82 | 0.24 | 2.83 | 8.33 | 0.28 | 3.53 | 8.53 | 0.23 | ||
NDVI | 4.05 | 9.72 | 0.23 | 3.55 | 9.39 | 0.27 | 4.34 | 9.74 | 0.22 | 3.20 | 9.07 | 0.32 | 3.68 | 9.79 | 0.26 | |
3.29 | 8.44 | 0.35 | 2.97 | 8.50 | 0.26 | 3.30 | 9.00 | 0.22 | 1.03 | 8.07 | 0.30 | 3.18 | 9.52 | 0.09 |
Separating sun illuminated parts from the shaded parts of the crown and thus reducing the factors influencing the crown representation in the image was expected to improve the defoliation prediction accuracies. However, the prediction root mean square error decreased by several percent. A somewhat larger prediction accuracy gain was achieved using conventional aerial images. In general, image transformation into principal components or normalized difference vegetation indexes resulted in slightly decreased prediction root mean square errors. Principal component transformation of small format aerial images resulted in up to 15% lower root mean square errors compared to using the digital numbers from the images. The idea behind using image statistics from the 15x15 cell windows around the crown centroid was based on the expectation that acceptable prediction accuracies could be achieved without delineating the crown projections. Just having to pin-point the tree crown on the aerial images was expected to be simpler task when using automatic and manual interpretation tools. However, the prediction root mean square errors were practically the same as using image characteristics for crown projection polygons from conventional aerial images.
The class of tree crown defoliation was better predicted using the modal class value of the 10 nearest neighbor tree crowns. The percentage of crowns with the correct defoliation class was 20-50% larger than using the median value (Tab. 4). Otherwise, rather similar results were achieved for all cases being studied. Defoliation class was correctly predicted for approximately 84-88% of older and 75-85% of younger tree crowns. Similar percentages of crowns with correctly predicted defoliation classes were achieved for younger trees using both small format and conventional aerial images. However, conventional aerial images yielded on average values that were 2% larger than values from the small format aerial images.
Tab. 4 - Percentages of crowns with correctly predicted defoliation classes. For each image transformation, upper number (in plain text) refers to small format aerial images, lower number (in italic) refers to conventional aerial images.
Age, years | Image transf. | Zone types used to extract image characteristics | |||||||||
---|---|---|---|---|---|---|---|---|---|---|---|
ZONES_1 | ZONES_2A | ZONES_2B | ZONES_2AB | ZONES_3 | |||||||
Mode | Median | Mode | Median | Mode | Median | Mode | Median | Mode | Median | ||
65 | No | 80.0 | 59.1 | 80.8 | 51.2 | 77.5 | 54.3 | 78.9 | 50.4 | 78.9 | 55.1 |
75.3 | 58.2 | 81.0 | 67.4 | 79.8 | 56.7 | 80.7 | 60.6 | 77.4 | 59.7 | ||
PC | 74.6 | 51.2 | 83.1 | 50.4 | 85.4 | 66.9 | 81.7 | 61.4 | 83.8 | 63.0 | |
82.1 | 56.7 | 81.5 | 59.1 | 84.1 | 58.2 | 79.5 | 56.8 | 81.0 | 64.2 | ||
NDVI | 76.8 | 53.5 | 81.9 | 56.7 | 79.7 | 55.1 | 80.8 | 58.3 | 80.6 | 55.1 | |
79.5 | 61.2 | 80.0 | 63.6 | 82.2 | 60.4 | 82.7 | 61.4 | 80.0 | 62.7 | ||
170 | No | 83.7 | 55.7 | 85.2 | 57.0 | 85.8 | 58.9 | 83.6 | 56.5 | 86.2 | 61.5 |
87.3 | 61.9 | 87.5 | 61.7 | 87.1 | 64.1 | 86.4 | 60.3 | 88.0 | 59.6 | ||
PC | 85.4 | 56.5 | 84.8 | 56.2 | 83.8 | 71.1 | 85.2 | 74.5 | 86.9 | 71.6 | |
86.2 | 64.3 | 86.0 | 62.4 | 87.6 | 62.9 | 86.0 | 63.5 | 87.0 | 60.7 | ||
NDVI | 85.3 | 58.4 | 85.4 | 63.4 | 86.6 | 71.6 | 86.3 | 69.2 | 84.9 | 59.4 | |
85.8 | 60.8 | 86.2 | 59.4 | 87.0 | 63.0 | 85.4 | 61.8 | 87.6 | 61.5 |
Only one tree crown defoliation prediction technique, the non-parametric k-nearest neighbour method, was used in this study. There are numerous other solutions that have been widely discussed in forestry remote sensing literature, such as regression (e.g., [24], [47], [41]), static and dynamic stratification (e.g., [49], [40]) and k-Most Similar Neighbor estimation ([38], [15]), which can also be used to predict defoliation. Some of them may even perform better in defoliation prediction for tree crowns under some circumstances than the k-NN method ([46]). However, the main advantage of non-parametric prediction is that it avoids the need for explicit models but it does presume that the total variation of the variable under focus is represented in the B-observations ([54]). Usually, the operational use of k-NN should be preceded by the optimization of the settings for the estimator and the list of auxiliary variables, using, e.g., the “Leave One Out” cross-validation technique ([54], [37]). The approach was to use the same approach for both types of images analyzed - all the image characteristics, t=2 and k=10. The conclusion from previous research has been that the quality of the auxiliary data, including the aerial images, is the most important factor affecting the prediction accuracy, while the implementation tactics and estimators (if the settings are appropriate - the ones mentioned above usually resulted in lowest prediction bias and root mean square errors in other our studies) have a secondary role ([42], [43]). This discussion has therefore focused on comparing the types of aerial images, whether small format aerial images may support tree crown level defoliation assessment and whether small format aerial imaging was compatible with the assessments achieved using conventional aerial images. Only two groups of pine trees (age 65 and 170 years) were analyzed in this study. Other tree species and conditions were disregarded due to the small number of tree crowns where there were field inventoried defoliation data available.
The results of this study showed that the small format aerial images may contain the potential to predict defoliation at the tree crown level with a similar accuracy to conventional aerial images. Similar pine tree crown defoliation prediction accuracies were achieved using both types of aerial images (small format and conventional images) on the over-mature stands (170 years old stands). On the middle aged stands (65 years old stands), the defoliation was better predicted by using conventional aerial images. However, both techniques showed a similar accuracy when predicting defoliation class. The rationale to use them at the operational level is the reduced costs related to image acquisition.
It was difficult to compare the costs involved in getting the small format and conventional aerial images used in the current study as both were obtained on non-commercial basis. However, it was possible to use the price lists of the aviation company that carried out the SFAP to the prices for carrying out conventional aerial photography for forest inventory purposes, including large scale aerial photography to investigate tree crown defoliation ([9], [45]), in Lithuania during the last decade. This enabled a rough cost estimation of both imaging techniques to be made. To take into account the effects of time and inflation, the price was adjusted to the official price index. Consequently, in order to calculate the present cost, a real discount rate of 3 % was used. Conventional aerial photography starts to be more cost effective than the SFAP when the project area is more than 20000-30000 ha (Fig. 3 - price values are omitted as they contain confidential information). However, when dealing with small area projects (1000-5000 ha), when the defoliation of individual crowns are targeted and, consequently, very high spatial resolution aerial images are needed, SFAP becomes several times more cost effective than use of conventional aerial photography.
Fig. 3 - Prices of SFAP and conventional aerial photography per area unit, depending on the size project area.
What could be the use of small format aerial photography within the frames of forest health monitoring programs? We see the niche of SFAP in local level forest inventories or forest health monitoring projects, such as International Cooperative Programme on Integrated Monitoring of Air Pollution Effects on Ecosystems ([55]), assessment of forest conditions after natural calamities such as insect attacks, wind damages, etc. Most likely such projects would be initiated on the need or could be carried-out permanently to monitor and archive the forest health status, they would target tree health statistics and would be used simultaneously with the survey of sample trees in the field.
Conclusions
The main conclusions of this study, aimed to compare the potential for using small format digital aerial images captured from an ultra-light aircraft to estimate Scots pine crown defoliation versus the one for conventional aerial images, were:
- Weak or none pair-wise correlations were observed between pine tree crown defoliation and various characteristics available from small format aerial images. Conventional aerial images resulted in larger correlations than the small format aerial images.
- Tree crown defoliation, predicted using the non-parametric k-Nearest Neighbor method, was overestimated by approximately 1% for 65 years old trees and underestimated by 3-4% for the 170 years old trees, for both small format and conventionally acquired images. The prediction root mean square errors achieved using small format aerial images for younger trees were around 11.5%, and around 9.5-9.9% when conventional aerial images were used. The differences in prediction between the root mean square errors mostly disappeared on older stands where root mean square errors were around 8.1-8.5%.
- Defoliation class was correctly predicted for approximately 84-88 % of the older tree crowns and 75-85 % of the younger tree crowns. Small format aerial images produced on average 2% lower figures than the conventional aerial images of the over-mature stands.
- In general, the small format aerial images may contain the potential to predict defoliation at tree crown level as accurately as the conventional aerial images but are several times cheaper when the areas targeted are thousands of hectares in size, thus they may be recommended for use within the frames of local level forest inventories or forest health monitoring projects.
Acknowledgments
The study was carried out within the framework of the national project No VP1-3.1-ŠMM-08-K-01-025 entitled “Specific, genetic diversity and sustainable development of Scots pine forest to mitigate the negative effects of increased human pressure and climate change” supported by the EU Social Fund.
References
Gscholar
Gscholar
Gscholar
Gscholar
Gscholar
Gscholar
Gscholar
Gscholar
Gscholar
Gscholar
Gscholar
Gscholar
Gscholar
Gscholar
Gscholar
Gscholar
Gscholar
Gscholar
Gscholar
Gscholar
Gscholar
Gscholar
Gscholar
Gscholar
Gscholar
Gscholar
Gscholar
Gscholar
Gscholar
Authors’ Info
Authors’ Affiliation
Institute of Forest Management and Wood Science, Aleksandras Stulginskis University, Studentu 11, LT-53361 Akademija, Kaunas distr. (Lithuania)
Laboratory of Forest Monitoring, Institute of Forest Management and Wood Science, Aleksandras Stulginskis University, Studentu 11, LT-53361 Akademija, Kaunas distr. (Lithuania)
Corresponding author
Paper Info
Citation
Mozgeris G, Augustaitis A (2013). Estimating crown defoliation of Scots pine (Pinus sylvestris L.) trees using small format digital aerial images. iForest 6: 15-22. - doi: 10.3832/ifor0705-006
Academic Editor
Elena Paoletti
Paper history
Received: Jul 26, 2012
Accepted: Nov 19, 2012
First online: Jan 14, 2013
Publication Date: Feb 05, 2013
Publication Time: 1.87 months
Copyright Information
© SISEF - The Italian Society of Silviculture and Forest Ecology 2013
Open Access
This article is distributed under the terms of the Creative Commons Attribution-Non Commercial 4.0 International (https://creativecommons.org/licenses/by-nc/4.0/), which permits unrestricted use, distribution, and reproduction in any medium, provided you give appropriate credit to the original author(s) and the source, provide a link to the Creative Commons license, and indicate if changes were made.
Web Metrics
Breakdown by View Type
Article Usage
Total Article Views: 46611
(from publication date up to now)
Breakdown by View Type
HTML Page Views: 39351
Abstract Page Views: 2139
PDF Downloads: 3904
Citation/Reference Downloads: 39
XML Downloads: 1178
Web Metrics
Days since publication: 4121
Overall contacts: 46611
Avg. contacts per week: 79.17
Article Citations
Article citations are based on data periodically collected from the Clarivate Web of Science web site
(last update: Feb 2023)
Total number of cites (since 2013): 7
Average cites per year: 0.64
Publication Metrics
by Dimensions ©
Articles citing this article
List of the papers citing this article based on CrossRef Cited-by.
Related Contents
iForest Similar Articles
Research Articles
Predicting tree crown defoliation using color-infrared orthophoto maps
vol. 6, pp. 23-29 (online: 14 January 2013)
Review Papers
Monitoring the effects of air pollution on forest condition in Europe: is crown defoliation an adequate indicator?
vol. 3, pp. 86-88 (online: 15 July 2010)
Research Articles
First results of a nation-wide systematic forest condition survey in Turkey
vol. 4, pp. 145-149 (online: 01 June 2011)
Research Articles
A fast screening approach for genetic tolerance to air pollution in Scots pine field tests
vol. 6, pp. 262-267 (online: 01 July 2013)
Research Articles
Long-term monitoring of air pollution effects on selected forest ecosystems in the Bucegi-Piatra Craiului and Retezat Mountains, southern Carpathians (Romania)
vol. 4, pp. 49-60 (online: 05 April 2011)
Research Articles
Rapid assessment of wind storm-caused forest damage using satellite images and stand-wise forest inventory data
vol. 6, pp. 150-155 (online: 08 April 2013)
Research Articles
Influence of climate on tree health evaluated by defoliation in the ICP level I network (Romania)
vol. 10, pp. 554-560 (online: 05 May 2017)
Research Articles
The patterns of nearest neighbor trees in a temperate forest
vol. 15, pp. 315-321 (online: 23 August 2022)
Technical Reports
Air pollution regulations in Turkey and harmonization with the EU legislation
vol. 4, pp. 181-185 (online: 11 August 2011)
Research Articles
Fuel characterization and crown fuel load prediction in non-treated Calabrian pine (Pinus brutia Ten.) plantation areas
vol. 15, pp. 458-464 (online: 03 November 2022)
iForest Database Search
Search By Author
Search By Keyword
Google Scholar Search
Citing Articles
Search By Author
Search By Keywords
PubMed Search
Search By Author
Search By Keyword