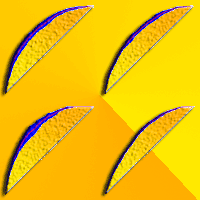
Forest fire occurrence modeling in Southwest Turkey using MaxEnt machine learning technique
iForest - Biogeosciences and Forestry, Volume 17, Issue 1, Pages 10-18 (2024)
doi: https://doi.org/10.3832/ifor4321-016
Published: Feb 02, 2024 - Copyright © 2024 SISEF
Research Articles
Abstract
Climate anomalies and potential increased human pressure will likely cause the increase in frequency and damage of forest fires in the near future. Therefore, accurately and temporally estimating and mapping forest fire probability is necessary for preventing from destructive effects of forest fires. In this study, the forest fire occurrence in Southwestern Turkey was modeled and mapped with the maximum entropy (MaxEnt) approach. We used past fire locations (from 2008 to 2018) with environmental variables such as fuel type, topography, meteorological parameters, and human activity for modeling and mapping, using data that could be obtained quickly and easily. The performances of fire occurrence models was quite satisfactory (AUC: range from 0.71 to 0.87) in terms of the model reliability. When the fire occurrence models were analyzed in detail, it was seen that the environmental variables with the highest gain when used alone were the maximum temperature, tree species composition, and distance to agricultural lands. To evaluate the models, we compared the fire locations between 2019 and 2020 with those on reclassified fire probability maps. Fire location from 2019-2020 fit substantially within the model fire occurrence predictions since many fire points in high or extreme fire probability categories has been observed. The results of this study can be a guideline for the Mediterranean forestry that has consistently struggled the forest fires and attempted to manage effectively forest lands at fire risk.
Keywords
Turkey, Fire Ignition, Fire Risk, Maximum Entropy, Machine Learning
Introduction
Forest fire has increased globally and regionally in recent years ([9], [11]). Global annual area burned is about 420 million hectares ([28], [30]). In Turkey, the average of the annual burned area (from 1988 to 2020) was 10,806 ha, while it was 139,503 ha in 2021, i.e., 13 times the annual average ([27]). Due to climate change and increased human pressures, forest fires are estimated to increase in the future ([9], [11]). Understanding the driving forces of ignitions and estimating where fires are likely to occur are critical factors in devising more effective plans to mitigate forest fire initiation and determining areas at risk ([37]).
Forest fires are ignited by either human or natural origin. The fires do not occur randomly, whether they are caused by humans or natural processes, but depend on fixed and variable environmental factors (i.e., fuel composition, topography, and weather conditions and human activities) ([43], [44], [37], [47]). Estimation of the fire occurrence requires complex temporal and spatial analyses ([31], [44], [8], [46], [32]).
Forest fire occurrence models have been developed in various regions around the world charaterized by different fuel and climatic conditions ([43], [44], [37], [47], [7], [5]). Fire occurrence models are developed based on current and past fire records to provide information that will help in preparing and planning for future fire seasons. Hence, the significance of modeling and mapping fire occurrences is evident.
Various mathematical models, such as regression models ([12], [40]), and artificial neural network models ([45], [34], [33]), are used to determine the effects of environmental factors in modeling forest fires. However, these models have their limitations for application. The regression models need to fulfill statistical assumptions such as normality, homogeneity, and independence. The violation of these assumptions may lead to serious problems in estimating the regression parameters, and therefore the predictions of statistical and biological behavior may show inconsistencies in fire studies ([53]). In this type of statistical problems, mixed-effects, autoregressive models can be used, though these models require strong theoretical knowledge ([50]). On the other hand, in case of small number of observations, the regression models may tend to produce large estimates and negative values for biological relations ([24], [48]). Neural network models may be overfitted to the data, and this may lead to the highest level of misleading success for training ([35], [36]). Indeed, incorrect inferences may be obtained when model fitting to a validation dataset is overlooked. While classical modeling approaches can be useful for small datasets, they are not sufficient for large datasets. In these cases, novel and new modeling techniques, such as machine learning, are needed.
Maximum entropy (MaxEnt) is a machine learning algorithm for modeling the probability distribution of species based on known points of occurrence by comparing independent variables associated with input coordinate points ([19]). The principle of MaxEnt is based on the theory that the probability distribution that best represents the current situation of information about a system is the one with the most entropy ([42]). MaxEnt can regulate highly sophisticated response functions by merging various function forms (linear, product, quadratic, threshold, and hinge) and make accurated predictions from limited datasets ([39]). MaxEnt may adapt jagged and sharply discontinuous responses that cannot be modeled in even the most flexible regression models ([13]).
MaxEnt model is frequently used to predict the geographic distributions from observations of the presence of species through explanatory environmental variables. Modeling species distributions is conceptually and methodologically similar to modeling fire occurrence ([37], [13]). The MaxEnt model can use fire occurrence data ([6]), in addition to environmental parameters such as temperature and precipitation, to predict potential distribution of the species or fire. Few studies have been done to model the fire occurrence according to fire occurrence data and environmental variables ([41], [13], [10], [16], [1], [6]). Dickey ([16]) examined the spatial relationship between human activities and human-induced wildfire occurrences in the Willamette National Forest in Oregon, USA and whether these activities were responsible for many wildfire ignitions. Akyüz ([1]) developed a model estimating the fire probability using only bioclimatic data and forest fire locations from 2013 to 2017 in a study conducted in Bursa, northwest Turkey. Bekar et al. ([6]) used MaxEnt approach to model fire occurrence in the Mediterranean Basin (in Turkey and Spain) and the Alps using bioclimatic variables, anthropogenic factors, road density and forest type at two spatial resolutions.
The importance of fire occurrence modeling and mapping is evident; it can help in preventing fires, planning firefighting efforts, making quick decisions, and implementing emergency measures. The fire occurrence is commonly estimated by evaluating certain data without investigating past fires and weightings these data according to expert opinion ([49]). In this study, we aim to fill this gap in the literature and try to estimate the fire probability by using past fire datasets as well as environmental variables (fuel properties, meteorological parameters, topographic factors), unlike other studies. Indeed, there is a need for novel approaches that take into account uncertainty and establish connections between the diverse long-term impacts of factors.
Machine learning techniques have proved useful in fulfilling the above goals. In this study, we used machine learning, which is one of the artificial intelligence techniques which has become increasingly popular in the recent years and started to be used in different disciplines. The MaxEnt machine learning technique has been chosen in this study for three key motivations. First, we used spatial and temporal (i.e., monthly) evaluations because the fire probability changes as meteorological factors change. Second, some variables are difficult to be included directly in the models, such as the timing and habits of vacations (e.g., annually recurrent behaviors like using fires for barbecuing during national or religious holidays) and traditional agricultural practices (e.g., burning stubble in the autumn), which are known to increase at certain times of the year. Third, it is obvious that the variables affecting fire probability may differ along the year due to the abovementioned reasons. Therefore, we preferred to assess fire occurrence estimates on a monthly basis.
The objectives of this study are: (i) to identify the drivers of the distribution of forest fires; (ii) to model and map the monthly fire occurrence with the MaxEnt machine learning technique; and (iii) to compare monthly fire probability in southwest of Turkey. Results of this study can guide for the fire potential modeling, planning and implementation of measures to prevent forest fires. Also, the model could be easily applied to different regions in the world after adaptation to local environmental conditions.
Materials and methods
Study area
The study was carried out within the Mugla Regional Directorate of Forestry (RDF), which covers an area of 2,051,212 ha in south-west Turkey (Fig. 1). Fifty-six percent (1,158,925 ha) of the Mugla RDF is covered with forests. The vegetation is composed of Calabrian pine (Pinus brutia Ten.), Anatolian black pine (Pinus nigra Arnold), Cedar (Cedrus libani A. Rich.), Stone pine (Pinus pinea L.), Oaks (Quercus spp.), Turkish sweetgum (Liquidambar orientalis Mill.) and Eucalyptus (Eucalyptus camaldulensis Dehn.) trees, and several shrubs of the maquis which form a dense dead and living cover which increases the fire probability and danger potential ([26]). Sixty-seven percent of the forests in the study area is located below 500 m a.s.l. in elevation. Human activities are high in and near these forests, as the region includes several most popular tourist attractions in Turkey, numerous mining sites, and wind turbines, which are more and more increasing. The increase in human activities has enhanced the risk of anthropogenic forest fires. Turkey faced the biggest forest fires in its history in the years 2019, 2020, and 2021. In 2021, 369 fires broke out in the study area, and a forest area of 52,219 ha was damaged by fire ([27]).
The study area is located in the Mediterranean climate zone (Fig. 1) and has high air temperatures and severe droughts in the summer. This situation impacts the occurrence of mega forest fires, which affect large areas in the summer (i.e., fire season) and the amount of damage to forest areas. Mugla RDF has not only the highest fire occurrence but also the second most damaged region from the fire in Turkey.
Maximum entropy (MaxEnt) model
In this study, MaxEnt v. 3.4.1 was used to model the spatial distribution of fire occurrence in South-West Turkey. Two kinds of data are needed as software input: (i) the coordinates of fire occurrence points; and (ii) the environmental variable maps. Also, some model calibrations are needed, such as the random test percentage, regularization multiplier, number of replicates, replicated run type, and output format (see below in the section “Modeling and performance of the model”).
The modeling process consists of 4 parts (Fig. 2): data collection, data preparation, modeling and validation, output and evaluation, which are described below.
Variables and data collection
Forest fire data
Data were obtained for 2.743 forest fire locations during fire seasons from 2008 to 2018 in the Mugla RDF. Forest fire locations were rearranged on a monthly basis and used as occurrence data (dependent variable) in the modeling. We also used fire location data from 2019 to 2020, which has been determined by field observations, to validate the developed model. Model predictions were also compared to these fire locations.
Tree species composition
Different tree species have different degrees of susceptibility to fire. It is known that especially Calabrian pine, Anatolian black pine and some maquis species have a high fire probability and potential to burn in the forests of Turkey ([2]). Therefore, the tree species composition (fuel type) map from the forest management database of Mugla RDF was complemented with field observations in the study area. Species composition maps were then converted from vector to raster format in ArcGIS® v. 10.5 ([20]).
Aspect
Aspect is one of the fixed factors affecting ignition. In the northern hemisphere, southern aspects are more exposed to higher temperatures, stronger winds, and lower air humidity than northern aspects. For these reasons, the fuel moisture content decreases in the southern aspects, making the fuel easier to ignite. Aspect maps were generated from a digital elevation model (DEM) in ArcGIS® and directly verified with field observations in the study area.
Maximum temperature
High temperatures cause the fuel to dry out. In addition, as the temperature increases, the fuel needs less heat to reach its ignition temperature. The highest temperatures and the lowest relative humidity levels occur at noon, so the fire risk is highest at this time of day. Therefore, we used the maximum temperature instead of the average temperature as the independent variable. We obtained the monthly maximum temperatures at 1-km resolution for the studied period from the open-access dataset CRU-TS 4.06 ([29]) downscaled with WorldClim 2.1 ([23]), which is freely available at ⇒ https://www.worldclim.org/data/monthlywth.html, and used to make maps of monthly maximum temperatures in the study area.
Precipitation
Precipitation increases the moisture content of fuel and thereby reduces the fire probability. We obtained monthly rainfall data from the CRU-TS 4.06 dataset ([29]). Rainfall data were extracted for the forest fire locations, and maps of monthly precipitation in the study area were prepared using ArcGIS®.
Solar radiation
As solar radiation increases, the fire probability also increases. Monthly solar radiation data for the study area were obtained from the CRU-TS 4.06 dataset ([29]), and imported in ArcGIS®.
Human activity
Early attempts to model anthropogenic fire occurrence were based on indirect assessments of human activities using demographic indicators or data on the accessibility to forests ([17]). Later attempts investigated spatially net factors such as distance from roads, distance from agricultural areas or distance from settlement areas in their assessments ([25], [46], [47]).
According to the forest fire statistics from 1988 to 2021, 90% of the fires in Turkey were caused by human-induced factors ([27]). To represent the effects of human activity in causing ignition, we created a buffer zone based on the distances of forest areas from roads, agricultural lands, and settlements. Buffer zones were classified in 5 groups according to the distance from the forest: extreme (<100m), high (101-200m), medium (201-300m), low (301-400m), very low (>400m).
Data preparation
We prepared a CSV file of the monthly coordinate values from past fire records for 2008 to 2018 during fire season. The environmental variables base maps to be used as independent variables were prepared in the ASCII format with the same resolution (1 × 1 km) and in the same coordinate system (WGS 84).
Modeling and performance of the model
We chosed bootstrap as the sampling method for our modeling, which has the advantage to automatically allow the empirical estimation of a distribution function by resampling the observed data, thereby the best selected model is not affected by autocorrelation ([3]). For the model output format, we used the logistics format, which estimates the probability of presence found in all areas with characteristic conditions for the fire ([19]).
The MaxEnt model was fitted to our dataset using 75% of the fire points for training and 25% for validation. The model run was repeated 20 times, and the regularization multiplier was taken as “1”. The regularization multiplier determines how realistic the predicted distribution is.
To assess the accuracy of model predictions, we used the area under the receiving operating characteristic (ROC) curve or area under the curve (AUC - [19]). The AUC indicates the true-positive rate as compared with the false-positive rate, thus providing a measure of model prediction accuracy and a tool for the comparison of models. Model prediction accuracy may be indicated based on the AUC as ([51]): excellent (0.9-1.0), very good (0.8-0.9), good (0.7-0.8), average (0.6-0.7) or poor (<0.6).
We used native metric and Jackknife test to determine variable importance in the fire occurrence models. First, variable importance was determined using the native metric which is regularized training gain ([37]). In addition, the Jackknife test was used to measure the variable importance based on the change in AUC using the testing data ([19]). The difference in AUC obtained with the full model (including all the variables) and the partial model (without the variable) indicates the contribution of each variable to the model ([37]), thereby assessing the relative impact of each input variable on the overall model performance ([19]).
Output and evaluation
We generated fire probability prediction maps for each model (monthly) in an ASCII format using MaxEnt software. These maps were then converted to a raster format and reclassified in 5 categories (FPC) based on the probability of fire occurrence as follows: (i) very low: P(fire) < 0.2; (ii) low: P(fire) = 0.2 to < 0.3; (iii) moderate: P(fire) = 0.3 to < 0.5; (iv) high: P(fire) = 0.5 to < 0.7; and (v) extremely high: P(fire) ≥ 0.7 ([14]). For model evaluation, we compared the fire locations from 2019 to 2020 with those on the reclassified fire probability maps and then compared the actual number of fires in each category with the fire probability values.
Results
Modeling and mapping of forest fire
In this study, we modeled the forest fire monthly occurrence during the fire season by using past fire locations from 2008 to 2018 as dependent variables and the environmental variables (i.e., climate, topography, fuel type, human activities) as independent input variables. Also, we mapped the monthly fire probability using MaxEnt software.
As expected, areas with high monthly average maximum temperature are more prone to fire. In addition, locations close to the road and agricultural land in terms of human activities and areas covered with Pinus brutia, maquis and pine stands in terms of species composition were more prone to fire.
On the monthly forest fire probability map, warm colours (red) tones indicate high fire probability, while cool colours (blue) tones indicate low fire probability (Fig. 3). The fire probability predictions on the maps were based on the probability of occurrence (P(fire)-value) as previously described. Fire probability categories (FPC) in the study area are shown in Tab. 1. The model results showed that the probability of fire changed monthly. The fire probability was high or extreme in May, June, July, and August and somewhat lower in September and October (Tab. 1).
Tab. 1 - Fire probability categories (FPC) of forest areas. P(fire): probability of fire; (very low): P(fire) < 0.2; (Low): 0.2 ≤ P(fire) < 0.3; (Medium): 0.3 ≤ P(fire) < 0.5; (High): 0.5 ≤ P(fire) < 0.7; (Extreme): P(fire) ≥ 0.7
Month | FPC | Total forest area | Month | FPC | Total forest area | ||
---|---|---|---|---|---|---|---|
ha | % | ha | % | ||||
May | Very low | 108447.1 | 9.4 | August | Very low | 125580.6 | 10.8 |
Low | 141812.4 | 12.2 | Low | 158828.3 | 13.7 | ||
Medium | 216306 | 18.7 | Medium | 215874.7 | 18.6 | ||
High | 508517.1 | 43.9 | High | 320989.2 | 27.7 | ||
Extreme | 183803.3 | 15.9 | Extreme | 337613 | 29.1 | ||
June | Very low | 145262.6 | 12.5 | September | Very low | 436964 | 37.7 |
Low | 126992.1 | 11 | Low | 163925.2 | 14.1 | ||
Medium | 217913.5 | 18.8 | Medium | 197290.5 | 17 | ||
High | 540706.2 | 46.7 | High | 242182.7 | 20.9 | ||
Extreme | 128011.4 | 11 | Extreme | 118523.3 | 10.2 | ||
July | Very low | 23524.3 | 2 | October | Very low | 660405.7 | 57 |
Low | 29483.8 | 2.5 | Low | 108133.4 | 9.3 | ||
Medium | 308482.1 | 26.6 | Medium | 97116.18 | 8.4 | ||
High | 532119.8 | 45.9 | High | 186390.9 | 16.1 | ||
Extreme | 265275.8 | 22.9 | Extreme | 106839.6 | 9.2 |
Contributions of the variables to the MaxEnt model
Tab. 2shows the estimates of the relative contributions of the environmental variables to the monthly MaxEnt model. According to the results, the contribution of precipitation to the model was limited, while maximum temperature and the distance from agricultural lands were the variables that mostly contributed to the model (Tab. 2). However, precipitation was the variable that contributed the most to the model in October (Tab. 2).
Tab. 2 - Variables contribution (VC, %) to the MaxEnt model, and their permutation importance (PI) in each month (M). (SC): species composition; (MaxTemp): maximum temperature; (Srad): Solar radiation; (Prec): precipitation; (DFR): distance from road; (DFA): distance from agriculture land; (DFS): distance from settlement.
M | Variable | VC | PI |
---|---|---|---|
May (05) | SC | 34.4 | 17.2 |
MaxTemp_05 | 15.1 | 14.7 | |
DFR | 14.1 | 14.8 | |
Prec_05 | 8.1 | 23.4 | |
Aspect | 7.9 | 6.3 | |
DFS | 7.6 | 7.8 | |
DFA | 6.8 | 4.3 | |
Srad_05 | 6.1 | 11.4 | |
June (06) | SC | 21.9 | 8.5 |
MaxTemp_06 | 19.7 | 26.5 | |
DFA | 18.3 | 8.0 | |
DFR | 10.3 | 15.4 | |
Prec_06 | 8.7 | 17.8 | |
Srad_06 | 7.8 | 6.6 | |
DFS | 7.4 | 9.4 | |
Aspect | 5.9 | 7.8 | |
July (07) | MaxTemp _07 | 22.4 | 15.3 |
Srad_07 | 20.9 | 22.4 | |
SC | 19.5 | 8.7 | |
Aspect | 11.3 | 12.9 | |
DFA | 8.8 | 11.1 | |
DFR | 6.7 | 10.4 | |
DFS | 5.7 | 9.4 | |
Prec_07 | 4.7 | 9.9 | |
August (08) | DFA | 31.8 | 19.2 |
MaxTemp _08 | 23.4 | 21.6 | |
SC | 11.3 | 9.8 | |
DFR | 10.7 | 13.8 | |
Prec_08 | 7.0 | 17.1 | |
Srad_08 | 6.5 | 5.7 | |
Aspect | 5.2 | 5.9 | |
DFS | 4.1 | 7.0 | |
September (09) | MaxTemp _09 | 38.3 | 47.3 |
Srad_09 | 32.0 | 39.5 | |
DFS | 10.0 | 1.2 | |
Aspect | 5.8 | 3.6 | |
DFS | 3.8 | 1.1 | |
DFR | 3.6 | 2.2 | |
SC | 3.5 | 0.7 | |
Prec_09 | 3.1 | 4.4 | |
October (10) | Prec_10 | 65.2 | 46.9 |
MaxTemp _10 | 11.0 | 18.8 | |
Srad_10 | 9.8 | 25.4 | |
DFR | 4.0 | 4.2 | |
SC | 3.0 | 0.5 | |
Aspect | 2.7 | 1.2 | |
DFA | 2.3 | 1.3 | |
DFS | 2.0 | 1.8 |
Performance of the MaxEnt model
We used the AUC to assess the model prediction accuracy. The results of the fire occurrence models showed that the AUC values, varied between 0.71 and 0.87 depending on the months during the fire season, and that the average AUC value was 0.79 (Fig. 3). In other words, although the model performances were different across the fire season, they were high or very high. These results were quite satisfactory in terms of the reliability of the model.
We also used the Jackknife test to determine the importance of the variables and evaluate the models. While MaxEnt provides the percent contribution of each variable to the overall model, the Jackknife test provides information on the performance of each variable in the model in terms of importance of each variable in both explaining the species distribution and providing unique information ([4]). The results of the Jackknife test indicate that the environmental variables with the highest gain when used alone were the species composition for May and June, the maximum temperature for July and September, the distance from agricultural land for August, and the precipitation for October. In addition, the environmental variable mostly decreasing the gain when omitted were precipitation for May and October, distance from the road for June and August, the maximum temperature for July and September (Fig. 4).
Fig. 4 - - Results of Jackknife test for evaluating the relative importance of environmental variables.
In addition, we compared the estimated monthly fire probability maps with fire data from the 2019 and 2020 fire seasons to check the accuracy of the model (Tab. 3). Seventy-five percent of May fires, 80% of June fires, 78% of July fires, 84% of August fires, 57% of September fires, and 55% of October fires occurred in areas with high or extreme fire probability (Tab. 3). The fire probability classes predicted by the model and the past fire locations observed were significantly overlapping.
Tab. 3 - Comparison of fire locations in 2019 and 2020 with fire locations on maps of the estimated monthly forest fire probability.
Forest fire probability | Number of forest fire (%) | ||||||
---|---|---|---|---|---|---|---|
Level | Range | May | June | Jul | Aug | Sep | Oct |
Very low | P(fire) < 0.2 | - | - | 5 | 1 | 9 | 10 |
Low | 0.2 ≤ P(fire) < 0.3 | - | 3 | 7 | 2 | 15 | 16 |
Medium | 0.3 ≤ P(fire) < 0.5 | 25 | 17 | 10 | 12 | 20 | 20 |
High | 0.5 ≤ P(fire) < 0.7 | 43 | 60 | 48 | 26 | 32 | 26 |
Extreme | P(fire) ≥ 0.7 | 32 | 20 | 30 | 58 | 25 | 29 |
Discussion
The MaxEnt approach has been widely used in spatial modeling in recent years, and its powerful ability to predict unknown distributions based on known information has been proven by studies on a variety of topics ([42], [21], [6], [52]). The MaxEnt approach satisfies all the constraints of spatial factors and increases the efficiency of finding the approximate distribution with maximum entropy thanks to machine learning algorithms ([52]). MaxEnt models are simple and precise, thus they are easy to use and understand, and can be applied to a wide range of data ([19]).
We used the MaxEnt approach for modeling and predict the probability of forest fires in different months by utilizing the relationship between fires from 2008 to 2018 with their locations and environmental factors (i.e., topography, fuel type, climatic parameters, and human activities). We decided to estimate the fire occurrence on a monthly basis rather than on annual basis. Similar to some previous studies ([38], [22]), our results demonstrate that the method applied is a useful tool for predicting fires based on the relationship between past fire occurrence and monthly climate parameters.
AUC values of our models indicate high or very high prediction accuracies (Fig. 5). Compared with previous studies ([13], [16]) the prediction accuracy was very similar. However, the prediction accuracy reported by Akyüz ([1])were lower than ours. The reason for this may be that while Akyüz ([1]) used only climatic variables, we also considered additional factors in the model like topography, fuel, and human activity.
Both native and Jackknife results demonstrated that while the contribution of precipitation in explaining forest fires is limited (except October), the contributions of maximum temperature, tree species composition, and distance from human activity (i.e., distance from road, settlement and agriculture areas) were clearly high, especially in dry months (monthly precipitation < 25 mm) from May to September (Tab. 2, Fig. 4). According to the Jackknife test, variables reduced the gain more when excluded were the distance from roads, maximum temperature, and precipitation. In other words, these variables provide to the model unique information that is not provided by any other variable (Fig. 4). Similar to previous studies ([37], [15]), our results confirmed that forest fires are caused by human activities and are facilitated by fuel and climatic conditions.
For field validation of the models, we used historical fire locations from the 2019-2020 fire seasons and compared them with the fire probability map. The rationale behind choosing the anomalous 2019-2020 fire season for model validation is to test the model in the worse scenario. As predicted by our model, the fires occurred mostly in forest areas where fire probability is high and extreme.
We analyzed the results of this study in detail in a monthly basis, finding that the most important predicting variables can be different for each month. Variables of tree species composition, maximum temperature and distance from road explain 63.6% of forest fires in May. With the start of tourism in the region in May, the density on the roads causes human-induced fires and are facilitated by fuel and climatic conditions. Meanwhile, tree species composition, maximum temperature, distance from agricultural land and roads explain 70.2% of forest fires in June. Human activity is higher in agricultural areas in June because of crop harvesting (wheat, barley), therefore distance from the agricultural area was one of the important variables in addition to the May variables. Since August is the time of stubble burning in preparation for planting, the most important determinant in our model was the distance from the agricultural area. Because precipitation drives the moisture content of the fuel and consequently the ignition of fire, the precipitation variable alone explained 65.2% of the fires in October, at the beginning of the rainy season, when the average precipitation is 75 mm ([7]). Precipitation was also found to be the most important variable in similar studies ([13], [10]). Interestingly, the determinant variable is the maximum temperature in the dry months and the precipitation in the rainy month. In a similar study by Bekar et al. ([6]), the most important variable in all regional models except for Turkey was the annual mean temperature, whereas precipitation was the most important variable in the Turkey model. This difference may result from ignoring seasonal differences at the study, which was conducted on an annual basis.
Forest managers can mitigate the damage caused by forest fires by allocating their fire suppression resources to forest areas more prone to fire. Monthly and spatially precautions should be taken against irresponsible activities such as negligent smoking and campfires, stubble burning for farming and traffic management using a forest fire probability map. Moreover, people living in areas with a high probability of fire can be informed about the impacts of their activities on the occurrence of forest fire.
Conclusion
The results of this study showed that the MaxEnt modeling method using environmental variables (i.e., topography, fuel type, climate, and human activity) can be a useful tool for predicting fire occurrence. In addition, this study demonstrated that fire occurrence changed monthly and that the most important predicting variables may be different for each month. The outputs of fire occurrence models can be considered robust and are good indicators for spatial assessment of fire occurrence, thereby helping prevent fire, predict fire probability, and organize firefighting efforts. They can also facilitate the assessment of the post-fire situation. The results of this study can be the basis for future investigations on other aspects of fire protection, such as fire danger rating systems, fire protection plans, and fire management studies.
Acknowledgements
This study is part of the Ph.D. thesis titled “Modeling and mapping of the forest fire potential based on MaxEnt approach in Southwest of Turkey” by Merih Goltas. We are grateful to the Mugla Regional Directorate of Forestry.
References
Gscholar
Gscholar
Gscholar
Gscholar
Gscholar
Gscholar
CrossRef | Gscholar
Gscholar
Authors’ Info
Authors’ Affiliation
Hamit Ayberk 0000-0002-6896-264X
Istanbul University-Cerrahpasa, Faculty of Forestry, Department of Forest Engineering, 34473, Istanbul (Turkey)
Kastamonu University, Faculty of Forestry, Department of Forest Engineering, 37100, Kastamonu (Turkey)
Corresponding author
Paper Info
Citation
Göltas M, Ayberk H, Kücük O (2024). Forest fire occurrence modeling in Southwest Turkey using MaxEnt machine learning technique. iForest 17: 10-18. - doi: 10.3832/ifor4321-016
Academic Editor
Davide Ascoli
Paper history
Received: Feb 02, 2023
Accepted: Nov 06, 2023
First online: Feb 02, 2024
Publication Date: Feb 29, 2024
Publication Time: 2.93 months
Copyright Information
© SISEF - The Italian Society of Silviculture and Forest Ecology 2024
Open Access
This article is distributed under the terms of the Creative Commons Attribution-Non Commercial 4.0 International (https://creativecommons.org/licenses/by-nc/4.0/), which permits unrestricted use, distribution, and reproduction in any medium, provided you give appropriate credit to the original author(s) and the source, provide a link to the Creative Commons license, and indicate if changes were made.
Web Metrics
Breakdown by View Type
Article Usage
Total Article Views: 14029
(from publication date up to now)
Breakdown by View Type
HTML Page Views: 9833
Abstract Page Views: 1717
PDF Downloads: 2351
Citation/Reference Downloads: 0
XML Downloads: 128
Web Metrics
Days since publication: 468
Overall contacts: 14029
Avg. contacts per week: 209.84
Article Citations
Article citations are based on data periodically collected from the Clarivate Web of Science web site
(last update: Mar 2025)
Total number of cites (since 2024): 3
Average cites per year: 1.50
Publication Metrics
by Dimensions ©
Articles citing this article
List of the papers citing this article based on CrossRef Cited-by.
Related Contents
iForest Similar Articles
Research Articles
Modeling human-caused forest fire ignition for assessing forest fire danger in Austria
vol. 6, pp. 315-325 (online: 16 July 2013)
Research Articles
Assessing the performance of fire danger indexes in a Mediterranean area
vol. 11, pp. 563-571 (online: 01 September 2018)
Short Communications
Upscaling the estimation of surface-fire rate of spread in maritime pine (Pinus pinaster Ait.) forest
vol. 7, pp. 123-125 (online: 13 January 2014)
Research Articles
Fire occurrence zoning from local to global scale in the European Mediterranean basin: implications for multi-scale fire management and policy
vol. 9, pp. 195-204 (online: 12 November 2015)
Short Communications
Estimation of canopy attributes of wild cacao trees using digital cover photography and machine learning algorithms
vol. 14, pp. 517-521 (online: 17 November 2021)
Research Articles
Wildfire risk and its perception in Kabylia (Algeria)
vol. 11, pp. 367-373 (online: 04 May 2018)
Research Articles
Probabilistic prediction of daily fire occurrence in the Mediterranean with readily available spatio-temporal data
vol. 10, pp. 32-40 (online: 06 October 2016)
Research Articles
The economic value of fire damages in Tuscan agroforestry areas
vol. 14, pp. 41-47 (online: 14 January 2021)
Research Articles
Assessing the performance of MODIS and VIIRS active fire products in the monitoring of wildfires: a case study in Turkey
vol. 15, pp. 85-94 (online: 19 March 2022)
Research Articles
Wildland fire typologies and extreme temperatures in NE Spain
vol. 10, pp. 9-14 (online: 01 November 2016)
iForest Database Search
Search By Author
Search By Keyword
Google Scholar Search
Citing Articles
Search By Author
Search By Keywords
PubMed Search
Search By Author
Search By Keyword