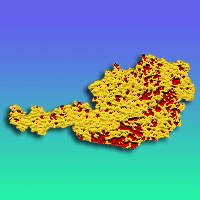
Modeling human-caused forest fire ignition for assessing forest fire danger in Austria
iForest - Biogeosciences and Forestry, Volume 6, Issue 6, Pages 315-325 (2013)
doi: https://doi.org/10.3832/ifor0936-006
Published: Jul 16, 2013 - Copyright © 2013 SISEF
Research Articles
Abstract
Forest fires have not been considered as a significant threat for mountain forests of the European Alpine Space so far. Climate change and its effects on nature, ecology, forest stand structure and composition, global changes according to demands of society and general trends in the provision of ecosystem services are potentially going to have a significant effect on fire ignition in the future. This makes the prediction of forest fire ignition essential for forest managers in order to establish an effective fire prevention system and to allocate fire fighting resources effectively, especially in alpine landscapes. This paper presents a modelling approach for predicting human-caused forest fire ignition by a range of socio-economic factors associated with an increasing forest fire danger in Austria. The relationship between touristic activities, infrastructure, agriculture and forestry and the spatial occurrence of forest fires have been studied over a 17-year period between 1993 and 2009 by means of logistic regression. 59 independent socio-economic variables have been analysed with different models and validated with heterogeneous subsets of forest fire records. The variables included in the final model indicate that railroad, forest road and hiking trail density together with agricultural and forestry developments may contribute significantly to fire danger. The final model explains 60.5% of the causes of the fire events in the validation set and allows a solid prediction. Maps showing the fire danger classification allow identifying the most vulnerable forest areas in Austria and are used to predict the fire danger classes on municipality level.
Keywords
Forest Fire, European Alpine Space, Austria, Infrastructure, Socio-economic Factors, Geographic Information System, Logistic Regression
Introduction
Fire danger is generally understood as the likelihood of a fire to occur ([14], [17]). In fire danger assessments the evaluation of the chances of fire ignition is generally done by identifying the contributing factors and their integration into an index quantifying the level of danger ([16], [54], [17], [18]). For the probability of a fire to occur, two agents are identified: natural (predominantly lightning) and anthropogenic causes, which are mainly related to human activities. In this context, the probability of human-caused fire ignition is the result of the direct or indirect presence of human activity in the landscape ([46]). International studies indicate that roughly 90% of forest fires are human-caused, whereas only a small percentage of forest fires have natural causes, i.e., lightning ([11], [30], [48], [59]). In Europe, human activities account for the majority of fire ignition ([41], [13], [46]).
Various goods and services provided by forests such as water supply, carbon sinks, recreation and protection services are most likely to be impacted by wildfires ([66], [30], [10], [25], [13], [20], [65]). Especially in the densely populated European mountain forests the danger of fire ignition is of high significance for the maintenance of its ecosystem goods and services as these ecosystems are very sensitive to environmental changes ([56], [43]). Additionally the economic value of goods and services of the alpine region experiences an increased recognition especially through sporting activities and outdoor recreation ([33]). Furthermore, the alpine region is crossed by important transit and trade routes. They aid in promoting tourism in the mountainous regions across Europe ([26], [50], [8]), which is leading to an increased development of touristic infrastructure besides the extensive use by nature-based tourism ([26], [34]). The herewith associated rise in pressure on forests through a growing number of tourists is potentially increasing danger of fire ignition. The increasing significance of transportation for the supply of living goods as well as for the provision of access to services potentially affects fire ignition as well. Several international studies have shown the significance of the distance of forest fires to roads, settlements and infrastructure, or specific land uses or even its abandonment as predisposition for fire ignition ([62], [28], [37], [13], [46]).
Although human factors are relatively important when analyzing forest fire ignition, little attention has been paid to their significance so far. The reason is the relatively high complexity of human behavior, which is often hard to measure. Especially data on human activities in forest areas are often lacking ([45], [62]). Due to the difficulties in obtaining detailed data on human activities, the human factor for wildfire ignition is difficult to model, since behavioral factors need to be identified, quantified and mapped (see [61], [46]). In the past, estimations of human activities have been based on indirect assessments. Underlying data for studies on the influence of socio-economic variables on forest fire ignition have been derived from census data or surveys ([19], [46]). However, the influence of human factors on forest fire danger is closely linked to the characteristics of the region. A number of studies analyzing the influence of human factors on forest fires have been conducted and decision support systems have been established in southern Europe ([37], [28], [54], [46]). However, a detailed analysis of the role of human factors on forest fire danger in the European Alps is rather rudimentary and has primarily been conducted together with the analysis of the influence of climate change on forest fire occurrence ([65], [64]) or the selective burning of forest vegetation ([51]).
Austria is a predominantly Alpine country with a highly diverse landscape ranging from the plains in the east to the Alps in the west and a forest cover of 47.2%. As the country is a popular tourist destination and is crossed by important transit routes, the influence of human factors on fire ignition probability cannot be disregarded. Compared to current research in other - especially southern European countries - forest fires have not been a serious concern in Austria so far. Forest fire records, which have been collected for Austria, indicate that the majority of forest fires are human-caused, whereas lightning accounts for 15% of the forest fires ([49]). Considering the vulnerability of the whole Alpine Region to climate change and land abandonment ([7], [57], [53], [27], [64]) we regard the investigation of the human-caused fire ignition as substantial for a better understanding of fire danger for Austrian forests and the goods and services they provide.
The objective of this study is to present a fire danger model to predict the spatial occurrence of human-caused fire ignitions in Austria. Records of human-caused forest fires for the period from 1993 to 2009 have been extracted from the AFFRI wildfire database and have been used together with potentially explanatory socio-economic variables in order to develop different logistic regression models and describe the influence of socio-economic factors on forest fire ignition. A model has been chosen, which represents the influence of the socio-economic factors on forest fire ignition probability the best. Based on the validation of the model’s performance, maps of potential fire ignition in Austria related to socio-economic factors have been produced.
Material and methods
Study area and fire database
Austria is a central European country covering 83 855 km2 and a population size of 8.47 million people. Austria is a largely alpine country (68%) with a temperate to alpine climate. Average temperatures range from -10 °C in winter to 20 °C in summer. Austria has a total number of 2357 municipalities with areas ranging from 32.4 km2 for the smallest municipality to 466.9 km2 for the largest municipality. Some 47.6% of Austria is covered with forests, out of which the main part is coniferous forest ([1]).
Underlying data on forest fires have been obtained from the wildfire database, which has been created within the frame of the Austrian Forest Fire Research Initiative (AFFRI) currently taking place at the University of Natural Resources and Life Sciences, Vienna. Wildfire records have been obtained from municipalities, fire brigades, the OEBB (Austrian Railway Company) as well as the Federal Ministry of Agriculture and Forestry, Environment and Water Management (BMLFUW). The database includes information for each fire record on geographical coordinates and other characteristics (e.g., municipality, cause of fire, time of day of start and end of fire, time of detection, ignition type, vegetation type and tree species affected) for the period 1993-2009 ([59], [21]). Given that this study analyzes the role of socio-economic factors for forest fire ignition, only 955 human-caused forest fires recorded in the database and matching the selected criteria (e.g., full record of information available, size of the burned area) have been used for this study, totaling to 1660 fire records. Lightning caused fires have been analyzed in a separate study and are not part of this dataset ([49]). Fires in agricultural areas have not been considered.
Parameter and model selection
Different methods ranging from logistic regression to artificial neural networks to classification and regression tree algorithms have been used to model wildfire occurrence. Logistic regression is one of the most frequently used methods ([3], [60], [16], [2], [9], [67]). It has been used to develop regional models with a large spatial extent ([15], [46]) as well as for developing models on a local scale ([62], [60]). In other studies logistic regression has been used to develop temporal models that are used to predict daily human-caused fire occurrence ([45], [44], [62]).
Model events with binary or dichotomous variables - such as the presence or absence of fire ignition - are best described by means of logistic regression. Forward stepwise logistic regression has been chosen for this study, since the interest was on identifying the significance of a group of predicting variables affecting fire occurrence as well as to model ignition probability. Moreover, logistic regression proved to be relatively flexible, since it accepts a combination of continuous, categorical and non-normally distributed variables ([6]).
In relation to the human-caused forest fires recorded in our database, five different sets of 955 points randomly distributed over Austria have been generated with ArcGIS™ and were classified as “no fire events”. These randomly generated sets of points have been joined with the 955 human-caused “fire events” from the forest fire dataset in order to be able to quantify the predictive ability of the socio-economic variables chosen. Ignition and non-ignition points were coded in a binary format (0: no fire; 1: fire) representing the dependent variable fire ignition. In order to be able to test the final model, 100 fire ignition points and 100 no-fire ignition points were selected randomly from each of the five datasets for validation, resulting in five sets with 855 fire and 855 no-fire events for calculating the model.
The analysis is based on the following function (eqn. 1):
where P
is the probability of occurrence of an event and z
is the linear function of the independent variables (eqn. 2):
The presence or absence of forest fire ignitions has been chosen as the dependent variable for the analysis.
Previous studies have identified a number of human factors to be significant for wildfire ignition (Tab. 1 - [11], [42], [16], [62], [9], [2], [63]). Based on the background of these studies, some 59 socio-economic parameters that were assumed to be characteristic for Austrian conditions were chosen as independent variables to model the potential influence on the fire danger. These socio-economic variables were clustered in five groups with similar characteristics on municipal level:
Tab. 1 - Potential human factors influencing forest fire ignition.
Modules | Abbreviation | Built-in module | Measure | Data source |
---|---|---|---|---|
- | TOTAREA | Total area of municipality | km2 | Corine land cover |
Urban module |
INHAB | Inhabitants of municipality | Numerical | Statistik Austria |
RESAREA | Residential area | km2 | Corine land cover | |
RESAREA/TOTAREA | Residential area / total municipal area | km/km2 | - | |
INHAB/TOTAREA | Inhabitants / total area | N/km2 | - | |
INHAB/RESAREA | Inhabitants / residential area | N/km2 | - | |
INHAB/AGRAREA | Inhabitants / agricultural area | N/km2 | - | |
INHAB/FORAREA | Inhabitants / forest area | N/km2 | - | |
Touristic module |
OVERN | Overnights | Numerical | Statistik Austria |
OVERN/INHAB | Overnights / inhabitants | Numerical | - | |
CABLE | Cable cars | Numerical | ⇒ http://www.seilbahnen.at | |
HUT | Huts | Numerical | ⇒ http://www.huetten.at | |
HIKETRAIL | Hiking trails | km | Corine land cover | |
CABLE/TOTAREA | Cable cars / total municipal area | N/km2 | - | |
CABLE/RESAREA | Cable cars / residential area | N/km2 | - | |
CABLE/AGRAREA | Cable cars / agricultural area | N/km2 | - | |
CABLE/FORAREA | Cable cars / forest area | N/km2 | - | |
HUT/TOTAREA | Huts / total municipal area | N/km2 | - | |
HUT/RESAREA | Huts / residential area | N/km2 | - | |
HUT/AGRAREA | Huts / agricultural area | N/km2 | - | |
HUT/FORAREA | Huts / forested area | N/km2 | - | |
HIKETRAIL/TOTAREA | Hiking trails / total municipal area | km/km2 | - | |
HIKETRAIL/RESAREA | Hiking trails / residential area | km/km2 | - | |
HIKETRAIL/AGRAREA | Hiking trails / agricultural area | km/km2 | - | |
HIKETRAIL/FORAREA | Hiking trails / forested area | km/km2 | - | |
Agricultural module | AGRAREA | Agricultural area | km | Corine land cover |
AGRAREA/TOTAREA | Agricultural area / total municipal area | km2 /km2 | - | |
AGROP | Agricultural operations | Numerical | Statistik Austria | |
AGRPAST | Agricultural holding with pasture land | Numerical | Statistik Austria | |
AGR/TOTAREA | Agricultural operations / total municipal area | N/km2 | - | |
AGR/RESAREA | Agricultural operations / residential area | N/km2 | - | |
AGR/AGRAREA | Agricultural operations / agricultural area | N/km2 | - | |
AGR/FORAREA | Agricultural operations / forest area | N/km2 | - | |
AGRPAST/TOTAREA | Agricultural holding with pasture land / total municipal area | N/km2 | - | |
AGRPAST/REAREA | Agricultural holding with pasture land / residential area | N/km2 | - | |
AGRPAST/AGRAREA | Agricultural holding with pasture land / agricultural area | N/km2 | - | |
AGRPAST/FORAREA | Agricultural holding with pasture land / forest area | N/km2 | - | |
Forest module |
FORAREA | Forest area | - | - |
FORAREA/TOTAREA | Forest area / total municipal area | km2 | Corine land cover | |
FORROAD | Forest roads | km | Corine land cover | |
FORHOL | Forestry operations | Numerical | Statistik Austria | |
FORROAD/TOTAREA | Forest roads / total municipal area | km/km2 | - | |
FORROAD/RESAREA | Forest roads / residential area | km/km2 | - | |
FORROAD/AGRAREA | Forest roads / agricultural area | km/km2 | - | |
FORROAD/FORAREA | Forest roads / forest area | km/km2 | - | |
FORHOL/TOTAREA | Forestry operations / total area of municipality | N/km2 | - | |
FORHOLRESAREA | Forestry operations / residential area | N/km2 | - | |
FORHOL/AGRAREA | Forestry operations / agricultural area | N/km2 | - | |
FORHOL/FORAREA | Forestry operations / forest area | N/km2 | - | |
Infrastructural module | ROAD | Roads | km | Corine land cover |
RAIL | Railroads | km | Corine land cover | |
ROAD/TOTAREA | Roads / total municipal area | km/km2 | - | |
ROAD/RESAREA | Roads / residential area | km/km2 | - | |
ROAD/AGRAREA | Roads / agricultural area | km/km2 | - | |
ROAD/FORAREA | Roads / forest area | km/km2 | - | |
RAIL/TOTAREA | Railroads / total municipal area | km/km2 | - | |
RAIL/RESAREA | Railroads / residential area | km/km2 | - | |
RAIL/AGRAREA | Railroads / agricultural area | km/km2 | - | |
RAIL/FORAREA | Railroads / forest area | km/km2 | - |
- Factors related to the human presence in urban, agricultural and forested areas in general (e.g., number of inhabitants, size of urban area);
- Factors related to touristic infrastructures and their activities (e.g., number of overnight stays, number of huts, cable cars and hiking trails);
- Factors related to agricultural production (e.g., agricultural area, intensity of agricultural operations);
- Factors related to forest management (e.g., density of forest area, forest roads, forestry operations, storm blow-down salvage harvesting);
- Factors related to infrastructure and their density (e.g., roads, railroads).
Tab. 1 shows all independent socio-economic variables chosen for the study.
Information on socio-economic factors in this study has been derived from the Statistik Austria census database on municipality level as well as from the Corine Landcover classification ([22]). Besides the above socio-economic data, additional variables have been generated in order to obtain socio-economic factors potentially relevant for forest fire ignition.
The logistic regression analysis was performed using SPSS® software, version 15.0.
Model construction
In order to eliminate multicollinearity between the variables selected for this study, the Pearson correlation analysis has been conducted for all five datasets independently. All variables with a correlation higher than 0.5 were not further considered for the model building procedure. Five different models have been calculated with the remaining variables using the different datasets in order to choose the best fit to Austrian conditions.
Various methods were tested examining the level of significance through which the variables were introduced and removed from the equation. Variables were introduced into the model according to a significance of p < 0.05 (Wald significance) and removed from the model with a p > 0.1 (Wald significance - see [46]). The Nagelkerke’s R2 test and the likelihood ratio -2LL value was used to estimate goodness-of -fit ([47]). 2 x 2 classification tables of observed and predicted responses were calculated in order to test the predictive capability of the models. To this purpose, municipalities were categorized with a probability threshold of 0.5. Municipalities with values lower than 0.5 were classified as being of low ignition danger and would therefore assigned as “no fire event” (0), whereas municipalities with a value higher than 0.5 would be classified as having a high danger of ignition and be assigned 1 as a predictive value for a recorded “fire event”.
In order to be able to evaluate the influence of individual variables in the model, a number of criteria were computed and analyzed:
- the Nagelkerke’s R2 and the Log-Likelihood ratio;
- the exponential of the logit coefficient B where Exp(B) < 1 corresponds to an increased value of the variable consistent with decreasing odds of a fire occurrence, whereas values of Exp(B) > 1 correspond to increasing odds of a fire occurrence;
- the step at which the variable was introduced in the model,
- the change in likelihood in case of the removal of the variable.
Even though other levels and approaches for assessing probability could be used (see [12]) the cut-off point 0.5 - the midpoint of the logistic function - was chosen for this study, since it is the one most extensively used in other comparable studies ([35], [60], [46]).
The outputs of the logistic regression model were compared to the spatial patterns of the original forest fire database. The binary classification of municipalities in high or low danger was compared with the actual values of the original dataset, assessing over- and under-estimation using 0.5 as classification criterion. Underestimations would be the municipalities with predicted low ignition danger though actually having a high forest fire danger, while overestimation would be the opposite.
Results
The best logistic model to analyze the influence of socio-economic factors on forest fire ignition has been selected out of the five models computed (Tab. 2 and Tab. 3) in order to identify the best combination of explaining variables. Railroad density (RAIL/ TOTAREA), density of forest roads (FORROAD/TOTAREA) and hiking trail density (HIKETRAIL/TOTAREA) were found to be significant in all five models. Model 2 has been chosen as the best model to represent the influence of socio-economic factors on forest fire ignition. In selecting the final model a combined evaluation of the statistical parameters for significance (Tab. 2), the socio-economic factors in the model (Tab. 3) and the percentage of predicting observed fire ignitions in the validation set was done. The selected model includes five variables (p<0.05, Tab. 4), predicting forest fire ignition with an accuracy of 54.2% (Tab. 5a) and a quite acceptable goodness-of-fit, with a Nagelkerke´s R2 of 0.153 and a -2 Log-Likelihood of 2158.925 (Tab. 2). The variables included in the selected model indicate that touristic infrastructures (i.e., hiking trail density, agricultural and forestry developments, forest road density and railroad density) are the principal socio-economic and infrastructural factors explaining forest fire danger in Austria. The 2 x 2 classification table (Tab. 5a) computed for the model building set with 1710 cases of “fire” and “no fire” events reached a total percentage of correctly predicted cases of 63.4. The validation dataset (Tab. 5b) showed a slightly lower total value of 60.5 %. Since the original database is relatively small (955 cases) and forest fires are spread over fairly large areas and long time periods, the results can be considered satisfactory. For both data sets reported in Tab. 5 better performances were obtained in identifying municipalities with lower fire danger (72.8 % for the model building dataset and 63.0 % for the validation dataset). Fig. 1 shows the probabilities distribution of the logistic model for the “fire” and “no fire” events from the model data set (Fig. 1a) and the validation data set (Fig. 1b). In the model data set, a left-sided distribution was obtained, whereas in the validation data set no clear tendency was observed.
Tab. 2 - Model outcomes for choice of best model fit. (df): degrees of freedom.
Omnibus Test of Model coefficients |
Parameters | Model 1 | Model 2 | Model 3 | Model 4 | Model 5 |
---|---|---|---|---|---|---|
Step | χ2 | 9.471 | 79.014 | 71.923 | 91.032 | 106.471 |
df | 1 | 1 | 1 | 1 | 1 | |
Prob | 0.002 | 0.000 | 0.000 | 0.000 | 0.000 | |
Block | χ2 | 69.784 | 79.014 | 71.923 | 91.032 | 106.471 |
df | 2 | 1 | 1 | 1 | 1 | |
Prob | 0.000 | 0.000 | 0.000 | 0.000 | 0.000 | |
Model | χ2 | 190.558 | 208.863 | 264.397 | 238.587 | 210.025 |
df | 5 | 6 | 5 | 4 | 4 | |
Prob | 0.000 | 0.000 | 0.000 | 0.000 | 0.000 | |
Model Summary | -2 Log-Likelihood | 2177.233 | 2158.925 | 2102.002 | 2131.976 | 2160.539 |
Cox & Snell R | ´0.106 | 0.115 | 0.143 | 0.130 | 0.116 | |
Nagelkerke’s R | 0.141 | 0.153 | 0.191 | 0.174 | 0.154 | |
Hosmer- Lemeshow-Test | χ2 | 16.365 | 21.009 | 16.541 | 8.243 | 22.069 |
df | 8 | 8 | 8 | 8 | 8 | |
Prob | 0.037 | 0.007 | 0.035 | 0.410 | 0.005 |
Tab. 3 - Variables included in the five models.
Model 1 | Model 2 | Model 3 | Model 4 | Model 5 |
---|---|---|---|---|
HIKETRAIL/TOTAREA | HIKETRAIL/TOTAREA | HIKETRAIL/TOTAREA | HIKETRAIL/TOTAREA | HIKETRAIL/TOTAREA |
- | - | HIKETRAIL/FOR | - | HIKETRAIL/FOR |
- | AGRPAST | - | - | - |
AGR/TOTAREA | - | - | - | - |
- | FORHOL | - | FORHOL | - |
- | - | FORHOL/TOTAREA | - | - |
FORROAD/TOTAREA | FORROAD/TOTAREA | FORROAD/TOTAREA | FORROAD/TOTAREA | FORROAD/TOTAREA |
ROAD/TOTAREA | - | - | - | - |
RAIL/TOTAREA | RAIL/TOTAREA | RAIL/TOTAREA | RAIL/TOTAREA | RAIL/TOTAREA |
Tab. 4 - Variables included in the final model. (SE): Standard error; (df): degrees of freedom; (CI): Confidence Intervals.
Variable | Coefficient B |
SE | Wald | df | Prob | Exp(B) | 95.0% CI EXP(B) |
Change in -2LL if removed |
Step for input into model |
|
---|---|---|---|---|---|---|---|---|---|---|
Lower | Upper | |||||||||
HIKETRAIL/ TOTAREA |
0.479 | 0.109 | 19.345 | 1 | 0.000 | 1.615 | 1.304 | 1.999 | 24.381 | 1 |
AGRPAST | 0.028 | 0.007 | 18.798 | 1 | 0.000 | 1.029 | 1.016 | 1.042 | 47.875 | 1 |
FORHOL | 0.008 | 0.003 | 10.006 | 1 | 0.002 | 1.008 | 1.003 | 1.013 | 8.514 | 2 |
FORROAD/ TOTAREA |
0.247 | 0.051 | 23.273 | 1 | 0.000 | 1.280 | 1.158 | 1.416 | 36.624 | 1 |
RAIL/ TOTAREA |
3.033 | 0.371 | 66.820 | 1 | 0.000 | 20.758 | 10.031 | 42.955 | 79.014 | 1 |
CONSTANT | -1.249 | 0.121 | 106.243 | 1 | 0.000 | 0.287 | - | - | - | - |
Tab. 5 - Classification table for model building dataset and validation dataset. Percentage of correctly classified: p=0.5.
Dataset | - | Class | Predicted | % Correct | |
---|---|---|---|---|---|
No fire | Fire | ||||
(a) Model building data | Observed | No fire | 622 | 233 | 72.7 |
Fire | 392 | 463 | 54.2 | ||
Total percentage | - | - | 63.4 | ||
(b) Validation dataset | Observed | No fire | 63 | 37 | 63 |
Fire | 42 | 57 | 58 | ||
Total percentage | - | - | 60.5 |
Fig. 1 - Distribution of probabilities of the logistic model for the “fire” and “no fire” events for the validation data set.
A sensitivity analysis has been performed in order to identify the influence of the significant variables included in the model. In Tab. 6 the six variables included in the model are ranked by a global score, obtained by adding the ranks of the five evaluation criteria: the lower is the ranking the more important is the variable in the model. The railroad, hiking trail and forest road density were found to be the most relevant factors independently from the chosen variable of significance. The ranking presented only slight variations with the different criteria, similar trends were observed for the criteria (i), (ii), (iii) and (v).
Tab. 6 - Ranking of influence for the input variables according to the sensitivity analysis.
Variables | Wald (i) |
Exp(B) (ii) |
Step (iii) |
Change in -2LL (iv) |
Regression Coefficient B (v) |
Global Score (Sum) |
---|---|---|---|---|---|---|
RAIL/TOTAREA | 1 | 1 | 1 | 5 | 1 | 8 |
HIKETRAIL/TOTAREA | 3 | 2 | 1 | 2 | 2 | 9 |
FORROAD/TOTAREA | 2 | 3 | 1 | 3 | 3 | 11 |
AGRPAST | 4 | 4 | 1 | 4 | 4 | 16 |
The variables found to be highly relevant for forest fire danger were used to compare the model prediction power in relation to the real fire observations (Tab. 7). In general, model outputs underestimated the number of forest fires with a low density of variables, whereas the number of likely fire ignitions was overestimated by the model for all variables at high densities, respectively. Fig. 2 shows the comparison between the numbers of fire ignitions based on the model prediction in relation to the real fire observations for the railroad density (RAIL/TOTAL) in the respective categories. At very low railroad densities (0.001 - 0.1 km/km2) the model has an underestimation for the fire occurrence of 48.3 % and at higher railroad densities (> 0.2 km/km2) an overestimation of more than 25%. Fig. 3 indicates that the model underestimates fire ignitions for municipalities with the category “no forest roads” (FORROAD/TOTAREA) quite high (54.5%), whereas the predicted fire ignitions for municipalities with a higher density of forest roads (> 1 km/km2) was comparable to the observed number of forest fires. As regards to the comparison of predicted vs. observed fire ignitions for the hiking trail densities (HIKETRAIL/TOTAREA), it becomes evident that the model underestimates the fire occurrence at low hiking trail densities and shows reasonable results for higher densities (> 1 km/km2). Only at high densities (> 2 km/km2) the model overestimates fire ignitions (> 40%), though the number of records is small in this category (Fig. 4).
Tab. 7 - Evaluation of the fire danger map classification with the validation dataset.
Fire danger classes | Forest area (%) |
Ignitions observed (%) |
Average density (fires/100 km) |
---|---|---|---|
Very low (0 - 0.19) | 24.6 | 9.0 | 1.16 |
Low (0.2 - 0.50) | 31.6 | 12.0 | 1.20 |
Medium (0.51 - 0.65) | 27.6 | 13.5 | 1.54 |
High (0.66 - 0.87) | 13.2 | 12.0 | 2.85 |
Very high (0.88 - 1.0) | 3.0 | 3.5 | 3.71 |
Fig. 2 - Fire ignition in relation to railroad density for original and model dataset (RAIL/TOTAREA).
Fig. 3 - Fire ignitions in relation to forest road density for original and model dataset (FORROAD/TOTAREA).
Fig. 4 - Fire ignitions in relation to for hiking trail density (HIKETRAIL/AREA) for original and model dataset.
All tested models confirm the high significance of socio-economic variables related to infrastructure for forest fire ignition in Austria. In partucilar, the variables railroad density (RAIL/TOTAREA), density of forest roads (FORROAD/TOTAREA) and hiking trail density (HIKETRAIL/TOTAREA) were found to be significant in all models.
Two maps have been generated with the selected model with the aim of illustrating model predictions for all municipalities which data were included in this analysis. Fig. 5 displays the spatial distribution of the forest fire danger at the level of municipalities, and Fig. 6 reports the model prediction for the forest areas only. The estimated probabilities have been classified in five fire danger classes. In Tab. 5 it can be observed that the majority of the forest areas is classified in the very low to medium fire danger classes (83.8 %); only 16.2 % of the forest area comprises a high to very high forest fire danger. Some 15.5% of all fire ignitions observed are located in the very high and high danger classes, and the very low and low danger class comprises 21% of all ignitions observed. The average ignition density per 100 km2 of forest area is in the high fire danger class 2.85 and for the very high fire danger 3.71, respectively.
Discussion
The logistic regression carried out in this study provides a model based on a set of socio-economic factors potentially affecting forest fire ignition, in addition to the driving factors of climate and fuel. The logistic model obtained correctly classified 63.1 % of the calibration and 59.5 % of the validation dataset by using the midpoint (0.5) of the logistic function, which is customarily used in most analyses ([46]). Depending on the fire management strategies and objectives, a different level of probability could be used to estimate forest fire danger. Comparing the medium to high fire danger areas of the model output with the known fire “hot spot” areas in Austria from a previous study (districts of Neunkirchen in Lower Austria, in Kapfenberg in Styria as well as the region around Villach and Arnoldstein/Spittal in Carinthia - [59]), we found that the midpoint of the Pearson correlation represents the actual fire danger problem in Austria quite well. The average density of the observed fire ignitions of the validation data (3.7 fires/100 km2) in the highest fire danger class is higher than the overall Austrian average.
The dominance of infrastructural variables implies that an easier access to forest areas through infrastructures may lead to an increased fire ignition risk in the future. The influence of agriculture, forestry and tourism on fire ignition seems to be enhanced by the easier accessibility of areas through infrastructure.
The railroad density per total area of a municipality (RAIL/TOTAREA) is related to development processes and its accessibility. Railways have often been related to accidental or negligence fires due to braking trains, broken brake shoes as well as repair works along railway tracks ([36], [11], [4], [46]). On one hand, Cardille et al. ([11]) assume that railroad density is influencing the ignition potential and frequency. On the other hand, the authors argue that railroad density together with road density would strongly affect the probability of a fire being reported in an area by increasing both residents and visitors.
The density of hiking trails per total area of municipality (HIKETREAIL/TOTAREA) indicates easy accessibility of forest areas both for tourists and local inhabitants. According to Romero-Calcerrada et al. ([52]) walking around recreational or camping areas is related to an increased susceptibility of forest areas to fire: the short the walking distance around a densely populated area the higher the likelihood of fire for a relatively small area. According to other studies ([62], [11]), negligence and other destructive activities such as arson may well be connected to this variable.
The density of forest roads per municipality (FORROAD/TOTAREA) is linked to the accessibility to forestry machinery and to enhanced human activity in forests such as timber harvesting and slash burning. Uhl & Buschbacher ([58]) and Fredericksen & Putz ([24]) identified these activities as potential causes for fire ignition and associated them with an increased susceptibility of forests to fire. Also Gossow & Frank ([29]) argue that salvage harvesting carried out when wind blows can lead to subsequent burns as a consequence of inappropriate engineering techniques. Another problem in this context is the simultaneous use of forest roads for timber harvest and tourist activities (such as hiking or mountain biking), which leads to a higher ignition probability.
In this study variables connected to infrastructure - especially railroads, hiking trails and forest roads - seem to be of great significance for forest fire ignition in Austria. Contrary to other studies such as Vega-Garcia et al. ([62]), Cardille et al. ([11]) or Sebastián-López et al. ([54]) road density was the only infrastructural predictor not significant in the model.
Unlike other regions such as Mediterranean countries, agricultural variables were not found to play a major role for ignition of forest fires in Austria (see [62], [11], [28], [54], [46]). In this study, factors such as agricultural machinery or the high partitioning of agricultural properties (which are considered to be relevant for wildfire ignition by other studies as a consequence of agricultural burnings - [46]) were not found to be of significance for Austria. Similarly, customary burning related to the persistence of livestock under traditional management was not found to be of relevance for Austrian conditions. These practices are legally banned in general; only a pilot program in Carinthia tries to improve the grazing capacity and quality for domestic livestock by alpine burning practices ([38]). However, these practices seem to be only of local relevance and cannot be generalized for Austrian conditions. Additionally, factors related to fire prevention activities, socioeconomic changes, land use abandonment or an increasing urbanization, land use disputes or unemployment rates ([28], [42], [9], [54], [46]) do not seem to have relevance for forest fire ignition in Austria. Nonetheless, it is worth noticing that only forest fire ignitions have been considered in this study, while fire ignitions on agricultural land have been excluded.
As tourism is still one of the most important income sources in Austria ([55]) the increasing number of visits and outdoor activities might lead to an increased ignition on a regional level in the future. Although studies such as Guyette & Dey ([31]), Cardille et al. ([11]) or Guyette & Spetich ([32]) have found a clear connection between factors related to population density and fire danger, these factors were not found to be relevant in the Austrian context. Other international studies confirm that population density play only a minor role as for forest fires ([9]). Variables related to population density were not significantly related to fire danger in this study. We assume that the probability for fire ignition is underestimated in areas with a low density of variables relevant for fire ignition. In this context the studies of Zumbrunnen et al. ([68]) have emphasized the non-linear nature of the relationships between fire occurrence and anthropogenic drivers. As road density was no longer correlated with fire occurrence above a certain threshold in two cantons of Switzerland, the authors concluded that expected future increase and spatial concentration of the human population may not result in a further increase in fire risk.
Contrary to the findings of other studies ([13], [46]), a high number of forest fires is predicted for areas with a low density of variables significant for fire ignition. Although a high average density of forest fire ignitions per forest area occurs in the highest fire danger class, the probability of fire occurrence was found to be higher in districts further away from areas densely populated or with a high density of infrastructure. According to Brosofske et al. ([9]) the explanation might be that arsonists seek especially remote areas to avoid capture. Moreover, the authors argue that the detection of forest fires might be lower in remote areas due to a lower density of infrastructure and therefore a generally low level of human activity in forests. These hypotheses might partly hold for the mountain forests in Austria. In general, a high level of fire detection is assumed as the average size of burned areas of most fires in the database is small. This assumption is supported by findings of Cardille et al. ([11]) who found that the low number of forest fire ignitions in areas with a high density of infrastructure and population strongly enhance the probability of a fire being reported.
Conclusions
In this study the logistic regression technique has been chosen to model forest fire ignition using relevant socio-economic factors as predictors. No meteorological parameters, topographic elements or fuel conditions were included since most of these factors require a fine-scale analysis, such as the province level. Our results prove that socio-economic variables found to be significant in other areas more prone to fire ignition risk ([13], [46]) have little relevance under the extant conditions in Austria, since many of these factors either do not exist or have minor impact for forest fire ignition risk.
The construction of the model was based on a logistic regression analysis. This method is more advisable than multiple regression, where the assumption of normality is often not met. We tested different logistic models to analyze and compare relevant socio-economic factors and to identify potential trends within the variables. Variable selection in the colinearity-exclusions process is always subjective and sometimes leading to different results. However, we found that the selected variables underlying the five models reflected the Austrian conditions fairly reasonable.
The results obtained in this investigation may be improved through the application of techniques less sensitive to correlations and nonlinear relationships among independent variables as well as the non-parametric distribution showed by most of the independent variables ([23], [46]). Performances of the model could be further improved by carrying out spatially explicit models such as the geographically weighted regression ([23]). First attempts using Geographic Weighted Regression have provided interesting results in the European Mediterranean ([39], [40]). The logistic probabilities would allow human factors to be integrated with meteorological factors ([5]) and fuel conditions. Statistical or physical models could be used for integration of factors in order to build an integrated fire danger model ([46]).
So far, records on wildfires and forest fires have not been documented consistently by the respective agencies, municipalities and fire brigades in Austria. The dataset underlying this study has been established in the last four years and the process of data assembly is still ongoing. Similar to other studies, the dataset still contains uncertainties regarding the size and location of forest fires and it is still incomplete because of the decentralized documentation of fire records ([59], [21]). For the above reasons and the overall small dataset available, in this study we did not consider the causes underlying forest fire ignition in Austria. Therefore, the analysis carried out can be seen as the first step of this research.
Acknowledgments
This research has been partly conducted within the frame of the Austrian Forest Research Initiative (AFFRI), which is funded by the Austrian Science Funds (FWF) - reference number L539-N14, and within the European Project ALP FFIRS (Alpine Forest Fire Warning System), which is funded by the European Regional Development fund of the Alpine Space Program - reference number 15-2-3-IT.
References
Gscholar
Gscholar
Gscholar
Gscholar
Gscholar
CrossRef | Gscholar
Gscholar
Gscholar
Online | Gscholar
Gscholar
Gscholar
Gscholar
Gscholar
Online | Gscholar
Gscholar
Gscholar
Gscholar
Gscholar
Gscholar
Gscholar
Gscholar
Authors’ Info
Authors’ Affiliation
Department of Landscape, Spatial and Infrastructure Sciences, Institute of Surveying, Remote Sensing and Land Information, University of Natural Resources and Life Sciences, Peter Jordan Str. 82, A-1190 Vienna (Austria)
Department of Integrative Biology and Biodiversity Research, Institute of Wildlife Biology and Game Management, University of Natural Resources and Life Sciences, Peter Jordan Str. 82, A-1190 Vienna (Austria)
Corresponding author
Paper Info
Citation
Arndt N, Vacik H, Koch V, Arpaci A, Gossow H (2013). Modeling human-caused forest fire ignition for assessing forest fire danger in Austria. iForest 6: 315-325. - doi: 10.3832/ifor0936-006
Academic Editor
Marco Borghetti
Paper history
Received: Dec 18, 2012
Accepted: Apr 14, 2013
First online: Jul 16, 2013
Publication Date: Dec 02, 2013
Publication Time: 3.10 months
Copyright Information
© SISEF - The Italian Society of Silviculture and Forest Ecology 2013
Open Access
This article is distributed under the terms of the Creative Commons Attribution-Non Commercial 4.0 International (https://creativecommons.org/licenses/by-nc/4.0/), which permits unrestricted use, distribution, and reproduction in any medium, provided you give appropriate credit to the original author(s) and the source, provide a link to the Creative Commons license, and indicate if changes were made.
Web Metrics
Breakdown by View Type
Article Usage
Total Article Views: 59238
(from publication date up to now)
Breakdown by View Type
HTML Page Views: 47785
Abstract Page Views: 4565
PDF Downloads: 5078
Citation/Reference Downloads: 93
XML Downloads: 1717
Web Metrics
Days since publication: 4085
Overall contacts: 59238
Avg. contacts per week: 101.51
Article Citations
Article citations are based on data periodically collected from the Clarivate Web of Science web site
(last update: Feb 2023)
Total number of cites (since 2013): 43
Average cites per year: 3.91
Publication Metrics
by Dimensions ©
Articles citing this article
List of the papers citing this article based on CrossRef Cited-by.
Related Contents
iForest Similar Articles
Research Articles
Contribution of anthropogenic, vegetation, and topographic features to forest fire occurrence in Poland
vol. 15, pp. 307-314 (online: 23 August 2022)
Research Articles
The economic value of fire damages in Tuscan agroforestry areas
vol. 14, pp. 41-47 (online: 14 January 2021)
Research Articles
Assessing the performance of fire danger indexes in a Mediterranean area
vol. 11, pp. 563-571 (online: 01 September 2018)
Research Articles
Historical fire ecology and its effect on vegetation dynamics of the Lagunas de Montebello National Park, Chiapas, México
vol. 14, pp. 548-559 (online: 01 December 2021)
Research Articles
Harmonized dataset of surface fuels under Alpine, temperate and Mediterranean conditions in Italy. A synthesis supporting fire management
vol. 13, pp. 513-522 (online: 13 November 2020)
Research Articles
Post-fire effects and short-term regeneration dynamics following high-severity crown fires in a Mediterranean forest
vol. 5, pp. 93-100 (online: 30 May 2012)
Research Articles
Differences of fire activity and their underlying factors among vegetation formations in Greece
vol. 6, pp. 132-140 (online: 08 April 2013)
Research Articles
Analysis of factors influencing deployment of fire suppression resources in Spain using artificial neural networks
vol. 9, pp. 138-145 (online: 19 July 2015)
Research Articles
Near zero mortality in juvenile Pinus hartwegii Lindl. after a prescribed burn and comparison with mortality after a wildfire
vol. 12, pp. 397-402 (online: 31 July 2019)
Short Communications
Upscaling the estimation of surface-fire rate of spread in maritime pine (Pinus pinaster Ait.) forest
vol. 7, pp. 123-125 (online: 13 January 2014)
iForest Database Search
Search By Author
Search By Keyword
Google Scholar Search
Citing Articles
Search By Author
Search By Keywords
PubMed Search
Search By Author
Search By Keyword