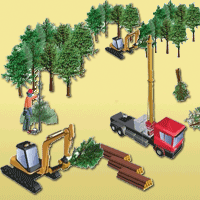
Biomasfor: an open-source holistic model for the assessment of sustainable forest bioenergy
iForest - Biogeosciences and Forestry, Volume 6, Issue 5, Pages 285-293 (2013)
doi: https://doi.org/10.3832/ifor0897-006
Published: Jul 01, 2013 - Copyright © 2013 SISEF
Research Articles
Abstract
This paper presents an open-source spatial analysis model (Biomasfor) that can quantify the availability of forest wood-energy biomass in the light of ecological and economic sustainability. Several multi-functionality parameters were evaluated to highlight the potential impact of biomass extraction on different forest functions. The multistep approach used and the model’s internal structure permit the use of the model with highly differentiated input datasets. The introduction of biomass demand evaluation allows the quantification of the wood-energy supply/demand. The analysis is focused on the province of Trento (northeastern Italian Alps). The results are based on a scenario evaluation characterised by several degrees of biomass extraction and by a sensitivity analysis of biomass price, as well as on a typology of mechanisation. The model outputs define a reduction in biomass availability with the introduction of technical, economic and multi-functionality parameters. Furthermore, considerations on territorial characteristics outline the importance of woodchip production as a means of avoiding carbon dioxide emissions and achieving low-impact reductions of the risk of fires. The model appears to be an effective tool in bioenergy planning, particularly for the following purposes: (i) the estimation of the biomass supply/demand ratio under different scenarios; (ii) a preliminary analysis of biomass quality; and (iii) the influence of local environmental, economic and logistical characteristics on biomass production.
Keywords
Spatial Analysis, Bioenergy, Open Source, Forest Multi-functionality, Holistic Models, GIS
Introduction
The assessment of the agroenergy chain is a fundamental issue in the field of renewable energies, in particular for the substitution of alternative energy sources for fossil fuels in rural areas ([18]). In this framework, the relevant topics are the evaluation of the bioenergy demand/supply ratio and the quantification of the impact of biomass on ecological and socio-economic parameters ([62]). Furthermore, the evaluation of the agroenergy chain must consider policy directives and the presence of fundings and regulation that can cause market distortion ([56]). The analysis of the biomass sector in holistic terms is quite complex given the proposed objectives and scale of results ([16]). Territorial and technological characteristics can be highly differentiated among study areas and could introduce variation into assessments of agroenergy ([21], [71]). The characteristics of the European region, particularly those of the Italian territorial area, coupled with the present forest landscape dynamics ([66], [67]) have suggested how the exploitation of wood-energy sources can achieve a high level of importance for bioenergy production in these areas (e.g., for a widespread relationship linking the agroforestry environment with the local population - [48], [42]). The variability of national forest areas in terms of geomorphology, species composition, facilities and socio-economic issues requires the use of flexible tools and Decision Support Systems (DSSs) to quantify the resources and to facilitate the communication between researchers, local stakeholders and policy makers in wood- energy planning activities as applied to the forest sector. A Geographic Information Systems (GIS) approach appears to represent an appropriate tool for attaining this goal.
Several studies have analysed GIS and spatial analysis instruments as tools for biomass chain evaluation at the European, national and local levels. Angelis-Dimakis et al. ([5]) classify energy availability in terms of potential availability (according to the gross energy of the source), theoretical availability (the harvestable fraction) and exploitable availability (based on ecological and economic sustainability criteria).
A state-of-the-art treatment of the topic of forest biomass availability at the European level was developed by Rettenmaier et al. ([49]) who analysed the methodological approaches and input datasets used for bioenergy estimation. These authors classified the analytic process according to biomass typology (ecological, technical, economical, sustainable), biomass sources (e.g., residues, stem, stumps) and spatial-temporal variables (e.g., scale of analysis, time frame). Geomatic applications for biomass resource evaluation have been implemented by different authors ([15], [32], [30]). For example, Gallaun et al. ([23]) have combined national forest inventory data and remotely sensed data to estimate the total increment and the above-ground biomass at the European level. The advantages and disadvantages of the application of geomatic procedures to bioenergy quantification have been analysed by Calvert ([12]).
Several studies consider the optimal location/allocation of resources, based primarily on economic and logistic parameters but also on policy constraints and sustainability evaluations ([72], [69], [38], [43], [3], [22], [33], [6]). Bush ([11]) by the use of multi-criteria evaluation and linguistic variables, introduces regional stakeholder preferences and planning guidelines as allocation criteria for Short Rotation Coppices (SRC).
Verkerk et al. ([70]) evaluated the potential supply of woody biomass from the forests of the EU in the light of multiple environmental, technical and social constraints. These calculations were based on the National Forest Inventories data implemented in the EFISCEN model. The quantification of the biomass supply/demand ratio has been achieved through widespread use of the calculations provided by the Woodfuel Integrated Supply/Demand Overview Mapping (WISDOM) approach ([36]).
The extraction of biomass components from forest areas (both the major components of the biomass - e.g., the stems - and other types of wood material - e.g., residues and stumps) has an impact on multifunctionality. This impact can be positive or negative depending on the local site characteristics (e.g., vegetation typology, geomorphology), silvicultural management and type of mechanisation. Berg et al. ([7]) developed a tool for sustainability impact assessment (ToSIA) of the whole forest wood chain, including economic, social and environmental indicators. Among the variety of possible influences resulting from such biomass extraction, the possible positive impacts include fire prevention, risk management and forest health ([47], [55]), greenhouse gas (GHG) emissions reduction ([75], [68]) and the addition of touristic-recreational value ([50], [63]). In contrast, an analysis of the literature stresses that negative impacts can potentially arise in the areas of soil fertility reduction ([17], [73], [4]), decreased soil and water protection ([1], [4]) and biodiversity losses ([51], [64]). Therefore, in a complex system such as the forest environment, it is important to evaluate the impact of biomass removal to prevent the overestimation of the bioenergy supply.
The general background developed for the analysis of applied GIS DSSs in the biomass sector stresses that the majority of the above mentioned papers refer to specific objectives and study areas. The application of these models to other case studies could be difficult because of differences in the datasets available. In addition, the model structure could complicate the implementation and evaluation of new variables and parameters, in particular if the model is based on proprietary software ([61]).
From this perspective, the implementation of the so-called holistic models (HMs) including the assessment of the bioenergy sector could be an intriguing new line of research. HMs focussed on bioenergy chain decision support and planning are not common in the literature. Among the case studies developed in recent years at the national level, an example of integration between landscape planning and the evaluation of bioenergy has been implemented through the PANDORA model ([24]). This model is able to incorporate concepts of thermodynamics, mathematical equilibrium and landscape analysis. The application of HMs requires the inclusion of a large number of parameters in the decision-making process; therefore, it is necessary to find a trade-off among the objectives of the analysis, the uncertainty allowable of the results and the feasibility of implementation of different scenarios.
In this framework, an open-source spatial analysis model (Biomasfor) that successfully overcomes the abovementioned limits will be specified. In the following paragraph, the model background will be examined. The characteristics of the Biomasfor model and an illustrative case study will then be presented.
Model background and integration of new components
The first version of the Biomasfor model was developed by Zambelli et al. ([77]). Their study implemented an estimate of the technical biomass extracted by a ground-based and cable crane system. The model computes the forest biomass with an approach based on the Free and Open Source Software for Geospatial (FOSS4G) framework ([76]). In the first version, the methodology combined the open-source GRASS software, PostGIS and the PostgreSQL object-relational database management system. In the present study, Biomasfor was implemented with economic and forest multifunctionality parameters following the approach used in Sacchelli et al. ([52]) and in Sacchelli et al. ([53]), respectively. In these two papers, the spatial analysis was based on proprietary software. To facilitate the installation of the software, the management of the tool and future development, the new Biomasfor version is based exclusively on GRASS GIS v. 7.0 ([41]).
Model implementation
Preliminary step
The Biomasfor model calculates the supply of forest energy-biomass for a defined territory.
Depending on the completeness of the input database available to the user, the model conducts a multi-step analysis that can yield estimates of ecological, technical, economic and sustainable bioenergy. By “ecological bioenergy”, we mean bioenergy based on a prescribed yield (e.g., in a Forest Management Plan) or on a periodic/annual increment (e.g., in a Forest Inventory). “Technical bioenergy” considers woody biomass obtained from a forest surface where extraction is possible given a particular level of mechanisation. The “economic bioenergy” is the part of the technical bioenergy that can be collected to supply heating plants or biomass logistic centres and that is associated with a positive net revenue for the entire production process. Finally, “sustainable bioenergy” introduces multifunctionality parameters and limits for biomass production.
The first step in model implementation is the dataset integration. Biomasfor automatically imports all variables according to the assigned column name (when variables are in shape file format - Tab. 1) and transforms them into a raster map with a specified pixel resolution. The input variable can be classified as mandatory (parameters strictly necessary for running the model) or optional. If the value of an optional variable is not available, a default value is used. This approach allows the model to process data even if certain parameter values are not available.
Tab. 1 - General input dataset and variable characteristics. (M): mandatory; (O): optional.
Variable | Description | Variable typology |
Name of attribute column |
---|---|---|---|
DTM (M) | Digital Terrain Model | ASCII GRID | - |
Main roads (M) | Main roads features | Shapefile | m_road |
Forest roads (M) | Forest roads features | Shapefile | f_road |
Total yield (M) | Prescribed yield or periodic/annual increment (m3) |
Shapefile | yield |
Yield of forest typology n (M) | Prescribed yield for forest typology n (m3) |
Shapefile | vol_typoln |
Forest management (M) | (1): high forest; (2): coppice | Shapefile | management |
Woodchip collection point(s), e.g., District Heating Plants (DHP, M) | Woodchip collection point | Shapefile | dhp |
Landing site (O) | Localization of landing sites | Shapefile | landing |
Forest treatment (O) | 1: final felling, 2: thinning | Shapefile | treatment |
Compartments (O) | Compartments boundary | Shapefile | compartment |
Roughness (O) | Roughness classification - (0): no rugged; (1): locally rugged; (2): partially rugged; (3): prevalently rugged | Shapefile | roughness |
Lakes (O) | Lakes features | Shapefile | lake |
Rivers (O) | Rivers features | Shapefile | river |
Mean tree diameter (O) | Average diameter (cm) | Shapefile | tree_diam |
Mean tree volume (O) | Average single tree volume (m3) | Shapefile | tree_vol |
Boundary (O) | Area to compute output variables (1, 2, ..., m - , e.g., Regions, Province, Municipality, etc) |
Shapefile | boundary |
Energy demand (O) | Annual bioenergy demand in DHP (MWh/y) | Shapefile | energy_dem |
Soil productivity (O) | Soil fertility category - (1): very low; (2): low; (3): medium; (4); high; (5): very high | Shapefile | soil_prod |
Soil texture (O) | Soil texture category - (1): not compacted; (2): medium compacted; (3): compacted | Shapefile | soil_text |
Soil depth (O) | Soil depth category - (1): superficial; (2): medium deep; (3): deep | Shapefile | soil_depth |
Soil compaction risk (O) | Soil compaction risk category - (1): low; (2): medium; (3): high; (4): very high; (9): no evaluation | Shapefile | comp_risk |
Fire risk index (O) | Fire risk index | Shapefile | fire_risk |
Protected areas (O) | Boundaries of protected areas: National, regional and provincial parks and reserves, and Natura 2000 sites (value 1 for protected areas, 0 otherwise) | Shapefile | protected |
Touristic value (O) | Suitability for recreational and touristic activity (value 1 for suitable areas, 0 otherwise) | Shapefile | touristic |
Each of the categorised bioenergy typologies (ecological, technical, economic and sustainable) represents a Biomasfor sub-model, as described in the following paragraphs.
Sub-models
Ecological bioenergy quantification
Biomasfor calculates ecological availability depending on yield, forest management and forest treatment. In the case of final felling or when the forest treatment typology is not specified, the total biomass is evaluated as a percentage of the prescribed yield expressed as cormometric volume (bark and stem without tops and branches). In the case of thinning intervention, the total bioenergy is derived from the whole tree (tops and branches, as well as the stem or a percentage of the stem). The analysis of field operations emphasises that the thinning of coppices is generally economically disadvantageous; therefore, only residues can be included in the computations included in the bioenergy calculations under this forest management approach.
Total bioenergy is finally quantified as the result of final fellings or on an annual basis according to the rotation period.
Technical bioenergy quantification
The model considers two types of forest processes: a ground-based extraction system and cable crane extraction (Fig. 1). The basis of the model is that the production of forest biomass is economically feasible with the Full Tree System (FTS) both in high forests and in coppices, in particular for the processing of tops and branches ([57]).
Fig. 1 - Example of forest production chain in high forests (source: ⇒ http://www.forestenergy.org/pages/images/ - [19], modified)
The mechanisation of extraction depends on the distance from the landing site, the slope and the terrain roughness as described in Zambelli et al. ([77]) and specified in the following case study. The integration of Biomasfor introduces additional forest chain organisation for thinning treatments and allows the parameter limits and the machinery typology to vary. The model can identify natural morphologies, such as morphometric features (e.g., pits, ridges, peaks), lakes and rivers, to calculate the actual extraction distances with the r.cost GRASS module. Full trees are extracted from the forest and delivered to the nearest landing site. Then, non-commercial material was chipped. Eventually, woodchips are delivered to their final destination (e.g., heating plants) by truck. In each evaluation unit (e.g., forest compartment), technical bioenergy can be considered to be spread uniformly over the entire surface or to be concentrated in accessible areas.
Economic bioenergy quantification
The economic biomass availability refers to the quantity of woodchips from accessible areas characterised by an economically feasible bioenergy chain. This definition implies that only areas with positive net revenues are considered.
Total revenues are estimated as the sum of the production of different assortments ([10]). More than one component can, in fact, be produced in a forest stand (e.g., roundwood, timber poles, woodchips).
The revenues R
obtained from the i-th pixel are calculated as (eqn. 1):
where n
is the number of a assortments in pixel i; Y
i is the total yield in pixel i; P
a,i is the percentage of a-th assortment in pixel i; p
a market price for a-th assortment.
For the v-th process phase and i-th forest pixel, the processing costs K
P were calculated as (eqn. 2):
where k
h,v,i is the hourly cost for v-th process phase in i-th forest pixel; p
v,i is the hourly productivity for v-th process phase in i-th forest pixel; Y
i yield for i-th forest pixel.
The hourly cost includes the machine and worker costs (see Appendix 1 - Tab. S1). The machine expense calculation are based on Miyata’s methodology ([37]). For each phase of the process, Biomasfor computes the hourly productivity based on the slope, tree characteristics, prescribed yield and extraction/transport distance (see Appendix 1, Tab. S2 - [60], [59], [35], [40], [58]). The delay times are also computed.
The direction expenses D
i, administrative costs Ad
i and interests I
i are calculated ([9]) to define the total cost KT,i (eqn. 3).
Finally, the total economic bioenergy Y
E is expressed by eqn. 4:
where Y
T,i is the ecological biomass availability in i-th forest pixel, and h
is the total forest pixels in study area.
To include the cost of moving machinery, the concept of “minimum harvestable volume” developed by Lubello ([34]) is considered. This concept involves the recognition that the harvesting of “small forest areas” with highly mechanized processes can fail to be economically feasible. For this reason, the authors calculate the break-even point (minimum harvestable volume) based on the total fixed costs of the machinery and the market price of the biomass components (eqn. 5):
where V
min is the minimum harvestable volume (m3), P
l is the price at landing (€/m3) and FC
m is the fixed costs of machinery (€).
The minimum harvestable area can be obtained using the average prescribed yield per unit of surface (e.g., m3/ha).
Sustainable bioenergy quantification
Biomass removal causes the potential depletion of a forest ecosystem but also allows potential enhancement. Biomasfor can estimate the decrease in the economic bioenergy availability to avoid a potential negative impact on the forest system and can also estimate the improvement in the social and environmental components due to the positive influences of biomass removal.
The non-living biomass that remains in the forest after harvesting operations is very relevant in terms of habitat and biodiversity conservation. Deadwood provides many important components of wildlife habitat and is important for seeds and other organisms, such as wood-inhabiting fungi ([64]).
From the perspective of fertility, biomass removal can influence the nutrient capital of the forest soil, the nutrient status and the growth of trees ([73]). The literature review authored by Abbas et al. ([1]) highlights the importance of the retention of tops and branches on the soil for hydrogeological protection and the maintenance of the carrying capacity of the site. In addition, water quality is influenced by soil compaction, which impacts water movement and increases surface runoff, erosion and the waterlogging of soil ([31]).
In this context, the following three indicators of negative impact are examined:
- site productivity reduction;
- soil and water protection reduction;
- biodiversity losses.
For each indicator and according to the local characteristics and the characteristics of mechanization, fixed biomass extraction limits were defined. Considerations of the maintenance of soil fertility yield removal limits based on the maximum rates for extracting stem and crown biomass during early thinning (see the current and medium mobilization scenario by [70]). Soil fertility does not appear to be strongly influenced by the extraction of logging residues over the short term ([74]); therefore, the removal of logging residues during final felling is permitted to a preset level of 90%. From a perspective of soil and water protection, the extraction limits are based on the maximum rates of extraction of stem and crown biomass during early thinning and on logging residues resulting from final felling (see the current and medium mobilization scenario by [70]). In particular characteristics such as slope, soil depth, soil texture and soil compaction risk were included in the analysis (Tab. 2). Restrictions on biomass removal to facilitate the maintenance of biodiversity are based on information in Sullivan et al. ([64]) and in Verkerk et al. ([70]) that generally favors the avoidance of residues removal from protected areas.
Tab. 2 - Extraction rate limits for multi-functionality criteria.
Multifunction criterion |
Variable | Description |
---|---|---|
Soil fertility maintenance |
Soil productivity | Thinning: 0% on very low and low fertility soils; 70% on other soils. Final felling: 90% on all soils. |
Soil and water protection |
Slope | Maximum extraction rate: 67% of residues up to 30% of slope and 100% over 30% and up to 100% of slope. 0% over 100% of slope. |
Soil depth | Maximum extraction rate: 0% of residues on superficial soils. | |
Soil texture | Maximum extraction rate: 0% of residues on compacted soils. | |
Soil compaction risk | Maximum extraction rate: 0% of residues on soil with very high compaction risk; 25% on soil with high compaction risk. | |
Biodiversity maintenance |
Protected areas | Maximum extraction rate: 0% of residues in protected areas. |
Fire risk reduction |
Fire risk index | Fire risk index was normalised on maximum single Country value (linear normalisation in the range 0-1). No biomass removal limits were set for fire risk reduction. |
Touristic-recreational valorization | Touristic value | No biomass removal limits were set for touristic-recreational valorization. |
CO2 emissions reduction |
CO2 emissions calculated for each forest process and energy production |
No biomass removal limits were set for CO2 emissions reduction |
Finally, three indicators of positive impact are evaluated:
- fire risk prevention
- touristic-recreational function improvement
- carbon dioxide emissions reduction.
According to forest typology, vegetation condition and climatic parameters, wood residues can represent a factor of risk for forest fires ([63]). The removal of woody debris and thinning material is, thus, a potential method of fire prevention ([55]).
The evaluation of fire risk prevention is based on the fire risk index calculated for EU countries ([54]). As stated by Schelhaas et al. ([54]) the risk of fire depends on different aspects of the danger associated with a fire (the moisture content of forest fuel, the rate of spread, the weight of fuel consumed and the intensity of the fire). Thus, it is difficult to relate biomass removal to reductions in the fire risk index. Agee & Skinner ([2]) assess the principles of fire resistance for dry forests in terms of the following factors: (i) reductions in the amount of surface fuel; (ii) increases in the height of the living crown; (iii) decreases in the density of the crown; and (iv) retaining large trees belonging to resistant species. Each of these principles is strictly dependent on local forest and geomorphological conditions. For the Italian conditions, due to the absence of specific studies, a weight equal to 30% has been assigned to biomass removal performed to reduce the fire risk index (see Tab. 2).
Scenic beauty, landscape variability and suitability of forest for recreational activity can be improved by extraction of after- felling residues ([65], [25]). For example, Ribe ([50]) stresses slash removal to be an important post-harvest practice in increasing beauty perception of tourists. Thus, 100% of residues extraction is hypothesized in order to strengthen, recreational function. Touristic improvement was measured as the sum of forest surface, with double function (productive and touristic), that have an economically feasible bioenergy production process.
An additional potential positive impact resulting from forest biomass use is the reduction of GHG emissions. In this framework, Biomasfor computes CO2 emissions for the whole forest process, the avoidance of CO2 emissions through the use of alternative fossil fuel and the resulting net balance. To develop a precautionary analysis, the total forest processing emissions are considered, not only the emissions associated with biomass production (chipping and woodchip transport). The net balance of the CO2 emissions is estimated in comparison with the equivalent energy of fossil fuels potentially used for heating. The fossil fuel emission coefficients are based on the literature ([20]), with the assumption that bioenergy and fossil fuel heating plants (diesel oil plants) are equally efficient. The coefficients inserted in the model for CO2 computation for each forest process are shown in Appendix 1, Tab. S3 ([44], [39], [28], [29]).
The details of the limits on residue extraction for each forest multi-functionality parameter are shown in Tab. 2.
Case study
The study area is the Province of Trento in the northeastern Italian Alps. The territory is characterized by strong variability in forest species composition and geomorphological conditions. These characteristics allow testing of the Biomasfor model in a widely differentiated area to include the variables that primarily influence biomass production. In addition, the demand for wood energy has been increasing rapidly in this area ([77]), and new tools are needed to assess the agroenergy chain and to quantify the supply of forest biomass.
Dataset and scenario assessment
The implementation of the dataset considers the sources for the variables listed in Tab. 3.
Tab. 3 - Dataset implemented for the case study.
Variable | Source |
---|---|
DTM | Territorial Informative System (SIAT), Province of Trento |
Main roads | Technical map, Province of Trento |
Forest roads | Forest and Fauna Office ([45]), Province of Trento |
Total yield | Forest Management Plan (PEFO), Province of Trento |
Yield per forest typology | Forest Management Plan (PEFO), Province of Trento |
Forest management | Forest Management Plan (PEFO), Province of Trento |
District Heating Plants (DHP) | Casini et al. ([13]) |
Forest treatment | Forest Management Plan (PEFO), Province of Trento |
Compartments | Forest Management Plan (PEFO), Province of Trento |
Roughness | Forest Management Plan (PEFO), Province of Trento |
Lakes | Technical map, Province of Trento |
Rivers | Technical map, Province of Trento |
Mean tree diameter | Forest Management Plan (PEFO), Province of Trento |
Mean tree volume | Forest Management Plan (PEFO), Province of Trento |
Provincial boundary | ISTAT (⇒ http://www.istat.it) |
Energy demand in DHP | Casini et al. ([13]) |
Soil productivity | Forest Management Plan (PEFO), Province of Trento |
Soil texture | Forest Management Plan (PEFO), Province of Trento |
Soil depth | Forest Management Plan (PEFO), Province of Trento |
Soil compaction risk | Houšková ([27]) |
Fire risk index | Schelhaas et al. ([54]) |
Protected areas | Portale Cartografico Nazionale (⇒ http://www.pcn.minambiente.it/PCNDYN/catalogowms.jsp?lan=it) |
Touristic value | Forest Management Plan (PEFO), Province of Trento |
The model outputs are based on scenario analysis (a base scenario and a three-variation scenario) with a spatial resolution of 40x40 m.
The analysis is initially focused on high forest because residue removal is more convenient in this forest type than in coppices, and because high forest is important in the entire forest chain in the Province of Trento. The structure of the Forest Management Plan (PEFO) database does not allow the estimation of the spatial distribution of thinning material but only includes the biomass from final felling. Woody material extraction has been initially hypothesized to occur via the nearest forest road because of the absence of a complete map of landing sites.
The percentage of tops and branches in high forest under Italian conditions usually varies between 10 and 30% of the cormometric volume, depending on the specific harvesting interventions and forest characteristics ([57], [8]). These values correspond to a range of 0.2-0.59 MWh/m3 of prescribed yield for a biomass moisture content of 40% (M40: current commercial moisture content).
A cautionary value of 0.3 MWh/m3 is set in the base scenario. The available mechanization level and a short-term increase in this level suggest the use of a skidder for ground-based extraction and of a medium-power cable crane system for aerial extraction.
The calculation of revenues is based on the unit prices for each forest category (firs, larix, Mountain pines, Arolla pine, beech and other broadleaves) and the typology of assortments (unique assortment, Arolla pine stem, shorts, packaging wood, first-class sawlog, larix first-class sawlog, timber pole, short sawlog, sawlog, larix sawlog). Market prices are reported in the database of the Chambers of Commerce, Industry, Handcraft and Agriculture of the Province of Trento ([14]) and refer to logs at the landing site. The base scenario biomass price is equal to 19.50 €/MWh, corresponding to approximately 55 €/t (the average market price for M40 woodchips).
The ecological, technical and economic values used for scenario assessment are shown in Tab. 4. In summary, variation is implemented in the following scenarios:
Tab. 4 - Parameters for the scenario assessment. (*): variation in respect to the base scenario.
Scenario | Residues rate (MWh/m3 of cormometric volume ) |
Extraction limits | Woodchip price (€/MWh) |
|||
---|---|---|---|---|---|---|
Machinery | Max. roughness degree |
Max. extraction distance (m) |
Slope (%) |
|||
Base | 0.3 | Medium power cable crane | No limits | 800 | 30.1-100 | 19.5 |
Skidder | 0-1 | 600 | 0-30 | |||
A | 0.49* | Medium power cable crane | No limits | 800 | 30.1-100 | 19.5 |
Skidder | 0-1 | 600 | 0-30 | |||
B | 0.3 | High power cable crane* | No limits | 1200* | 30.1-100 | 19.5 |
Forwarder* | 0-1 | 800* | 0-30 | |||
C | 0.3 | Medium power cable crane | No limits | 800 | 30.1-100 | 28.4* |
Skidder | 0-1 | 600 | 0-30 |
- scenario A: ecological parameter variation (increase in biomass extraction from 15 to 25% of cormometric volume for tops and branches);
- scenario B: technical parameter variation (increase in mechanization level using higher-power machinery characterized by higher hourly cost (Tab. S1), higher productivity (Tab. S2) and capable of a greater extraction distance);
- scenario C: economic parameter variation (increase in the sales price of biomass according to current market trends).
For each scenario, the ecological, technical and economic bioenergy availability are calculated. The provincial wood-energy supply/ demand ratio and the total net revenues for the entire forest chain are then quantified. Finally, the sustainable availability and the influence of biomass production on net CO2 emissions, fire risk reduction and recreational improvement are estimated.
Results and discussion
The results of scenario assessments are shown in Tab. 5. In the base scenario, the ecological availability is about 133 500 MWh/y, with decreases in technical bioenergy and economic bioenergy of 9% and 31%, respectively. The total revenue from the entire forest chain (sales of traditional assortments and woodchips) is 6 200 000 €/y. The sustainable bioenergy represents a 60% decrease relative to the ecological bioenergy: multifunctionality thus appear to be a fundamental parameter in terms of biomass production. The results underscore the substantial influence of the soil and water protection parameter, as well as of biodiversity and fertility maintenance, on bioenergy production. The relatively small decrease in the technical biomass highlights the good density of forest roads in the study region. The slope of the terrain is the primary influence on forest accessibility (the correlations between positive net revenues and the slope, extraction distance and roughness R
are equal to -0.36, -0.29 and -0.27, respectively). The analysis of the density of forest roads (26 m/ha) confirms that this value is higher than the national average of forest roads density in high forests. Moreover, an area whose road density is greater than 20 m per hectare is generally considered highly accessible ([26]).
Tab. 5 - Results os the four scenario assessments considered in the case study.
Parameter | Base scenario |
Scenario A |
Scenario B |
Scenario C |
---|---|---|---|---|
Ecological availability (MWh/y) | 133596 | 218207 | 133596 | 133596 |
Technical availability (MWh/y) | 121387 | 198266 | 123781 | 121387 |
Economic availability (MWh/y) | 92058 | 156937 | 88529 | 95042 |
Total revenues (€/y) | 6201907 | 7329056 | 5912112 | 7001438 |
Energy demand in DHP (MWh/y) | 178198 | |||
Fertility bioenergy (MWh/y) | 82853 | 141243 | 79676 | 85538 |
Soil and water protection bioenergy (MWh/y) | 70562 | 120179 | 67730 | 72803 |
Biodiversity bioenergy (MWh/y) | 77160 | 131139 | 74160 | 79494 |
Sustainable availability (MWh/y) | 53820 | 91342 | 51737 | 55395 |
Net avoided emission (t CO2/y) | 23575 | 44046 | 23288 | 24315 |
Fire risk (absolute value) | 938070 | |||
Reduced fire risk (absolute value and %) |
896873 (4.39%) | 894674 (4.63%) | 898633 (4.20%) | 895253 (4.56%) |
Recreational area (ha) | 98707 | 98707 | 98707 | 98707 |
Improved recreational area (ha) | 40214 | 41903 | 39251 | 41471 |
The use of wood residues for thermal energy production potentially prevents the emission of 23 500 t CO2/y. Biomass removal results in a low percentage of fire risk reduction (about 4%) due to local characteristics that produce a fire risk index lower than the national mean value. A total of 41% of the productive forest area is characterized by touristic-recreational improvement.
The scenario analysis shows an increase of the economic availability in Scenario A and in Scenario C (+3% and +2%, respectively). Scenario B appears less attractive than the base scenario (-3%) because the greater extraction distance and greater productivity of the hypothetical machinery cannot compensate for the higher hourly costs. In this case, the rate of biomass removal influences the outcome. For example, increasing the extraction of tops and branches from 15% of the cormometric volume to 22% produces an enhancement of economic efficiency equal to a 7% gain relative to the base scenario.
An additional important result is the halving of carbon dioxide emissions and the doubling of sustainable availability in Scenario A. The variation in the other parameters in Scenarios B and C is quite low.
The wood-energy supply/demand ratio considers the demand associated with the District Heating Plants (DHPs) of the Province of Trento ([13]). This ratio varies between 0.5 in Scenario B and 0.88 in Scenario A. Although the total demand is not satisfied by forest residues, it must be recognized that the main source of bioenergy for the DHPs is currently the sawmill residues (approximately 80%). Forest biomass can represent an integration of sawmill residues. Furthermore, biomass from thinning should be included in the forest energy chain assessment.
Finally, the biomass allotted to local residents for their residence rights should be excluded from the total bioenergy availability ([77]). The total amount of residues allotted to the residents is 68 000 MWh/y ([45]).
Potential future improvements and conclusions
The model developed in this study appears to be an appropriate DSS tool for the quantification of forest bioenergy availability. The multistep procedure yields an estimate of biomass for energy production that introduces ecological, technical, economic and social constraints based on a literature analysis and on local characteristics. The spatially based outputs allow the results to be identified in a georeferenced format and the values of the areas analyzed to be aggregated at the desired administrative level. This aspect should be particularly relevant for policymakers, allowing them to apply regulations and funds at the local level. The flexible structure of the tool allows its use in different study areas and for different available dataset. The implementation of the model in a single software system (GRASS GIS) facilitates the installation and management.
The scenario assessment framework defines not only the amount of biomass but also the supply/demand ratio and the economic added value for the entire forest chain according to the modeling of the input variables. The potential impact of the removal of woody residues is estimated both in negative terms and for environmental enhancement.
The term “holistic model” applied to Biomasfor does not mean that the results obtained are claimed to be exhaustive. Rather, the structure of the model and the open-source basis of the approach furnish the opportunity to develop further evaluations for each parameter. In particular, multifunctionality variables could be examined in depth and new input data introduced (e.g., additional forest production processes and functions such as new mechanization levels, impact analysis of regeneration or berry production). Field experiments now in progress will help verify the correspondence between real forest processes and the model outputs. Best practices and trade-off among different forest function could be evaluated by the application of multicriteria approach and the depiction of Pareto front and manifolds.
Future case studies could be performed with coppices and different forest treatments (final felling and thinning) to delineate not only the total amount of bioenergy but also the qualitative characteristics resulting from the origin of the biomass (e.g., stems, tops, branches, conifers, broadleaved species). This categorization will allow the quantification of biomass suitable for heating plants of different sizes and power characteristics.
To optimize the functionality of the model, the transport distances for woody material could be computed on the basis of the true demand/supply ratio for the DHPs or the biomass logistic centers. In the current version of Biomasfor, these distances represent the nearest collection points to the forest area. The application of GIS-based linear programming (LP) methodologies could overcome this limitation. The implementation of a user-friendly graphical interface will facilitate the application of the model by its end users. This work is based on a holistic concept that can be adopted in other fields of application following the same logic ([46]).
The Biomasfor model is available online for testing and integration at ⇒ http://sourceforge.net/p/biomasfor/code/ci/415ab11cda6fae799d77423a8cb400af780f9f09/tree/.
Acknowledgments
This work was funded by the BIOMASFOR project co-funded by the CARITRO Foundation through grant No. 101. The authors wish to acknowledge CARITRO, as well as the BIOMASFOR project partners, for their contribution to the research.
References
Gscholar
Gscholar
Gscholar
Gscholar
Gscholar
Gscholar
Gscholar
Gscholar
Gscholar
Gscholar
Gscholar
Gscholar
Gscholar
Gscholar
Gscholar
Gscholar
Gscholar
Authors’ Info
Authors’ Affiliation
GESAAF - Dipartimento di Gestione dei Sistemi Agrari, Alimentari e Forestali, Univ. di Firenze, p.le delle Cascine 18, I-50144 Florence (Italy)
P Zatelli
M Ciolli
DICAM - Dipartimento di Ingegneria Civile, Ambientale e Meccanica, Università di Trento, v. Mesiano 77, I-38123 Trento (Italy)
Corresponding author
Paper Info
Citation
Sacchelli S, Zambelli P, Zatelli P, Ciolli M (2013). Biomasfor: an open-source holistic model for the assessment of sustainable forest bioenergy. iForest 6: 285-293. - doi: 10.3832/ifor0897-006
Academic Editor
Luca Salvati
Paper history
Received: Nov 16, 2012
Accepted: Apr 02, 2013
First online: Jul 01, 2013
Publication Date: Oct 01, 2013
Publication Time: 3.00 months
Copyright Information
© SISEF - The Italian Society of Silviculture and Forest Ecology 2013
Open Access
This article is distributed under the terms of the Creative Commons Attribution-Non Commercial 4.0 International (https://creativecommons.org/licenses/by-nc/4.0/), which permits unrestricted use, distribution, and reproduction in any medium, provided you give appropriate credit to the original author(s) and the source, provide a link to the Creative Commons license, and indicate if changes were made.
Web Metrics
Breakdown by View Type
Article Usage
Total Article Views: 59521
(from publication date up to now)
Breakdown by View Type
HTML Page Views: 49821
Abstract Page Views: 2949
PDF Downloads: 5272
Citation/Reference Downloads: 79
XML Downloads: 1400
Web Metrics
Days since publication: 4351
Overall contacts: 59521
Avg. contacts per week: 95.76
Article Citations
Article citations are based on data periodically collected from the Clarivate Web of Science web site
(last update: Mar 2025)
Total number of cites (since 2013): 24
Average cites per year: 1.85
Publication Metrics
by Dimensions ©
Articles citing this article
List of the papers citing this article based on CrossRef Cited-by.
Related Contents
iForest Similar Articles
Research Articles
Making objective forest stand maps of mixed managed forest with spatial interpolation and multi-criteria decision analysis
vol. 6, pp. 268-277 (online: 01 July 2013)
Research Articles
Using self-organizing maps in the visualization and analysis of forest inventory
vol. 5, pp. 216-223 (online: 02 October 2012)
Review Papers
Carbon neutrality of forest biomass for bioenergy: a scoping review
vol. 16, pp. 70-77 (online: 05 March 2023)
Research Articles
Nutritional, carbon and energy evaluation of Eucalyptus nitens short rotation bioenergy plantations in northwestern Spain
vol. 9, pp. 303-310 (online: 05 October 2015)
Research Articles
Spatial distribution of aboveground biomass stock in tropical dry forest in Brazil
vol. 16, pp. 116-126 (online: 17 April 2023)
Research Articles
Tree volume modeling for forest types in the Atlantic Forest: generic and specific models
vol. 13, pp. 417-425 (online: 16 September 2020)
Commentaries & Perspectives
Benefits of a strategic national forest inventory to science and society: the USDA Forest Service Forest Inventory and Analysis program
vol. 1, pp. 81-85 (online: 28 February 2008)
Review Papers
Integration of forest mapping and inventory to support forest management
vol. 3, pp. 59-64 (online: 17 May 2010)
Research Articles
Geographic determinants of spatial patterns of Quercus robur forest stands in Latvia: biophysical conditions and past management
vol. 12, pp. 349-356 (online: 05 July 2019)
Review Papers
Open top chamber and free air CO2 enrichment - approaches to investigate tree responses to elevated CO2
vol. 3, pp. 102-105 (online: 15 July 2010)
iForest Database Search
Search By Author
Search By Keyword
Google Scholar Search
Citing Articles
Search By Author
Search By Keywords
PubMed Search
Search By Author
Search By Keyword