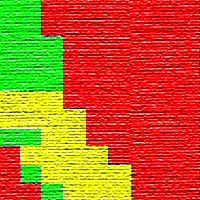
Soil and forest productivity: a case study from Stone pine (Pinus pinea L.) stands in Calabria (southern Italy)
iForest - Biogeosciences and Forestry, Volume 4, Issue 1, Pages 25-30 (2011)
doi: https://doi.org/10.3832/ifor0559-004
Published: Jan 27, 2011 - Copyright © 2011 SISEF
Research Articles
Abstract
The objective of this study was to determine whether edaphic and/or topographic variables may be used as predictors of site productivity in Stone pine stands in Calabria (southern Italy). To accomplish this goal, a linear discriminant rule was developed using data from 16 pure Stone pine stands, grouped into three different classes based on the mean dominant height annual growth. The discriminant rule was based on three linear models (one for each class) that jointly predicts site class for a given stand. To test the accuracy of the proposed method, cross-validation was carried out by developing 16 alternative discriminant rules (excluding the analyzed data). Predictors tested were edaphic (texture, pH, organic matter) and topographic (altitude and slope) variables. The model obtained allow to discriminate poorest sites accurately (100% of sites were correctly re-classified using the discriminant functions obtained). In more productive areas, sites were correctly re-classified in the 33.33% of cases, while in intermediate sites the correct classification was equal to 50%. Our discriminant rule classifies correctly the poorest stands, suggesting that site index in plain site soils strongly depends on clay percentage. Overall, the edaphic model obtained classifies plots into the correct site index class 61.11% of cases, which is considered an acceptable value for these kinds of studies.
Keywords
Clay, Pinus pinea, Site index, Site productivity, Topographic factor
Introduction
Stone pine (Pinus pinea L.) is one of the most important species in southern Europe widely distributed along Mediterranean coastal areas, from Portugal to Syria. Stone pine has important ecological, landscape, recreation and soil conservation uses covering over 600 thousand hectare mainly in Spain, Italy, Portugal, France, Morocco and Tunisia. In Italy the main products are pine nuts, wood and truffles. However, both soil-site and local studies focused on plantations are scarce. Young planted stands in southern Italy need silvicultural treatment in order to achieve managerial objectives (mainly environmental protection and production), to avoid permanent productivity declines.
Site index, defined as a species-specific measure of actual or potential forest productivity, has been widely used as forest productivity surrogate because it is an important parameter in forest growth and yield modeling. Site index can be estimated through site data, stand data, or both combined. Usually, in operational forestry, site index is estimated by measuring stand variables (dominant height and age). However, in some cases (young stands, high-graded stands, etc.) appropriate stand variables are not available. Studies based on soil-growth relationship (soil-site method) have been carried on for a wide range of species elsewhere (e.g., [16], [20], [25], [27], [42], [5]), but very few soil-site studies have been reported for Stone pine ([6]). Site index is a variable surrogate of the net stem growth that can be harvested in traditional forestry. As net stem growth harvested is roughly a 10% of the Gross Primary production (GPP) in the temperate forests ([29]), site index serves also as proxy of GPP linking forest growth and yield science with production ecology. Site index perform better in monospecific, even aged, single-layered forests and plantations as young planted Stone pine stands.
Soil-site studies based on topographic and edaphic factors are utilized to estimate site index when stand data as dominant height and age are not available or not suitable for this purpose. Altitude, slope, aspect ([20], [27], [17]) and geographic position ([27]) are the topographic factors commonly used (Jokelaet al. 1988, [27], [17]) to estimate site index. It is common knowledge that topography, in the forest ecosystem, influences local microclimates by changing the pattern of precipitation, temperature and relative humidity ([36], [40]) and significantly affects soil physical and chemical characteristics and soil organic matter trend, strictly related to the dynamic and ecology of the soils.
Topographic factors are usually utilized in combination with edaphic factors. Soil texture and nutrients levels are commonly used to estimate site index ([1], [20], [37], [27]), though the wide range of nutrients and analytical methods make difficult to compare the results. On the other hand, it is well known that nutrients explain a low percentage of height increment ([1]). For this reason, some authors avoid the use of nutrients to estimate site index ([33]). As multicollinearity among soil properties related to site index can also cause problems in regression analysis ([24], [38]), discriminant analysis ([16], [5], [6]) have been used.
Many conceptual models exist for defining the biotic, abiotic, and cultural practices that influence forest productivity ([35], [8], [26]). Morris & Miller ([26]) described forest productivity as a function of plant potential, climate, soil-site quality, and catastrophes. Computer modelling has been used to account for these factors, but models are imperfect and sometimes unsatisfying, because of scaling issues ([30]).The ability to make a compelling field evaluation of soil properties and site productivity would be an important achievement.
The objective of this study is to determine if edaphic and/or topographic variables can be used as predictors of site productivity in Stone pine stands in Calabria (southern Italy). To accomplish this objective, a discriminant rule was developed by using data from 16 pure Stone pine stands. Three site productivity classes have been developed based upon mean dominant height annual growth and the discriminant functions included both edaphic and topographic variables.
Materials and methods
Site description
The study area is located in the Calabrian Apennines, southern Italy, Lat 39° 50’ - 53’ Long 15° 55’ - 16° 16’ (Fig. 1). Mean annual temperature is 15 °C, mean temperature of the coldest month is 7.4 °C, and mean temperature of the warmest month is 24.6 °C. Mean annual precipitation is 799 mm; summer precipitation is 102 mm, with a dry period of 46 days. The natural vegetation is dominated by Holm oak (Quercus ilex L.) and Hop hornbeam (Ostrya carpinifolia Scop.), sharing dominance with other broadleaves.
Fig. 1 - Walter-Lieth climate diagram and location map showing plots situation in Castrovillari, Calabria Apennine, southern Italy (Lat 39° 50’-53’ Long 15° 55’-16°16’). The natural vegetation is dominated by Holm oak (Quercus ilex L.) and Hop hornbeam (Ostrya carpinifolia Scop.), sharing dominance with other broadleaves.
To represent the silvicultural variability of the area studied, 16 circular plots of 706 m2 (Tab. 1) were randomly established in four different areas reforested during 1948-1970 in Calabria. In sloping terrain soil preparation was carried out in small terraces and direct seeding was done in late summer. Terrace length varied from 1500 to 2500 linear meters per hectare, with a distance between them of about 4 meters. In these stands pre-commercial and commercial thinning operations rarely have been executed. The canopy cover ranges from 85 up to 100%. Tab. 2 and Tab. 3 show the main characteristics of the plots analyzed.
Tab. 1 - Main edaphic, topographic and soil site characteristics of Stone pine plots analyzed to study site index estimation in Calabria (southern Italy).
Plot | Age | Altitude (m a.s.l.) |
Aspect | Parent material | Soil type |
---|---|---|---|---|---|
Castrovillari | |||||
CA1 | 45 | 700 | W | Calcareous breccias | Pachic Hapludolls |
CA2 | 45 | 680 | W | Calcareous breccias | Pachic Hapludolls |
CA3 | 45 | 600 | SW | Calcareous breccias | Pachic Hapludolls |
CA4 | 45 | 500 | SW | Calcareous breccias | Pachic Hapludolls |
Frascineto | |||||
FR1 | 55 | 420 | SW | Dolomite | Pachic Hapludolls |
Morano | |||||
MO1 | 35 | 800 | W | Dolomite | Pachic Hapludolls |
MO2 | 35 | 840 | W | Dolomite | Pachic Hapludolls |
MO3 | 36 | 820 | W | Dolomite | Pachic Hapludolls |
MO4 | 36 | 820 | W | Dolomite | Pachic Hapludolls |
MO5 | 36 | 840 | W | Dolomite | Pachic Hapludolls |
Papasidero | |||||
PA1 | 40 | 360 | NE | Calcareous Dolomite | Pachic Hapludolls |
PA2 | 38 | 460 | NE | Calcareous Dolomite | Typic Hapludolls |
PA3 | 38 | 485 | NE | Calcareous Dolomite | Typic Hapludolls |
PA4 | 38 | 485 | NE | Calcareous Dolomite | Typic Hapludolls |
PA5 | 40 | 530 | SW | Calcareous Dolomite | Typic Hapludolls |
PA6 | 40 | 490 | S | Calcareous Dolomite | Lithic Hapludolls |
Tab. 2 - Stand level characteristics of Stone pine plots analyzed to study site index estimation in Calabria (southern Italy). QMD: quadratic mean diameter; H0: dominant height; MDHG: mean dominant height annual growth.
Parameters | Basal area (m2/ha) |
Volume (m3/ha) |
N (trees/ha) |
QMD (cm) |
H0 (m) |
MDGH (m) |
---|---|---|---|---|---|---|
Mean | 21.3 | 116.3 | 612.7 | 20.9 | 8.8 | 0.22 |
Maximum | 44.6 | 315.8 | 877 | 27.7 | 12 | 0.27 |
Minimum | 10.1 | 45 | 353 | 17 | 5.2 | 0.04 |
Standard deviation | 8.7 | 63 | 158 | 3.2 | 1.5 | 0.12 |
Tab. 3 - Chemical and physical soil properties (clay, sand and silt content, organic matter amount, pH, cation exchangeable capacity, and calcium amount) and topographic variables (altitude, slope, and depth) used to develop the discriminant rule to estimate the site index class in Stone pine (Pinus pinea) in Castrovillari (Calabrian Apennines, southern Italy).
Variable | Mean | STD | Max | Min |
---|---|---|---|---|
Sand (%) | 56.75 | 19.94 | 74 | 23 |
Silt (%) | 32.69 | 22.74 | 68 | 11 |
Clay (%) | 10.56 | 3.86 | 19 | 6 |
Ca (meq/100g) | 37.63 | 13.24 | 50 | 10 |
Organic Matter (%) | 4.3 | 0.19 | 4.57 | 4.07 |
Depth (cm) | 75.08 | 10.08 | 95 | 60 |
Slope (%) | 22.81 | 13.16 | 45 | 10 |
Altitude (s.l.m.) | 615 | 169.22 | 840 | 360 |
CEC (meq/100g) | 23.08 | 11.30 | 55.3 | 14.0 |
pH | 8.08 | 0.24 | 8.31 | 7.3 |
Soil samples
Soil samples (0-10 cm depth) were used to describe the characteristic of forest soils in accordance with the methodology proposed by Jokela et al. ([20]), particularly because the changes caused by environmental or topographic factors are more prominent and relevant (e.g., on organic matter and soil biochemical properties) on the upper layer of the soils.
Soil samples were taken from the first 10 cm for each soil profile and analyzed independently. The samples were brought to the laboratory on the same day and kept in the refrigerator at 4 °C for up to 24 h until processing. Prior to soil analysis, all the samples were air-dried, sieved (< 2 mm), and visible roots were removed. Particle size analysis was carried out by the hydrometer method, using sodium hexametaphosphate as a dispersant ([4]); pH was measured in distilled water and 1 M KCl using a 1:2.5 (soil:water) suspension; organic carbon was determined by dichromate oxidation ([41]), and it was converted to organic matter by multiplying the percentage of carbon by 1.72. Soil total nitrogen was measured by the Kjeldahl method, and cation exchange capacity (CEC) was measured using the barium chloride-triethanolamine method ([23]). Available P was determined by the Bray II method ([7]). Exchangeable K+ was extracted with 1 M NH4OAc, and determined using a flame photometer. Electrical conductivity (mmhos/cm) was measured using the solution extracted from a saturated paste ([18]). Exchangeable cations Ca2+, Mg2+, K+ and Na+ were extracted with 1 M ammonium acetate (pH=7) and determined by atomic absorption spectrometer.
Data analysis
Site productivity classes
Three site productivity classes have been developed based upon mean dominant height annual growth (MDHG). Stands age range from 35 to 45 years. Class I was defined when MDHG is equal to or more than 0.25 meters, class II was defined when MDHG is less than 0.25 meters and over 0.19 meters and class III was defined when MDHG is equal to or less than 0.19 meters.
Discriminant models
The examined discriminant functions include edaphic and topographic variables, with the following general structure (eqn. 1):
where β0, β1, …, βn are the obtained coefficients, Xi s are values of the n variables used as predictors.
To obtain site index classification by using soil and topographic variables, classificatory discriminant analysis was applied. The original data set has been used to both define and validate the discriminant functions. The use of the same data set to obtain and validate discriminant models involves a bias in site misclassification, called apparent error rate, and generates an “optimistic” estimate of the accuracy of the method. To minimize the above bias, cross-validation was carried out using a discriminant function computed from all other observations to classify each observation (see [19] - pp. 217-285 for more details).
Departure from normal distribution of the independent variables considered was verified by the Shapiro-Wilk test, and Pearson’s correlation coefficients among variables were calculated. Different transformations of the original variables have been applied to avoid skewed distributions of the variables considered. To this purpose, CORR, DISCRIM and UNIVARIATE procedures of the SAS® Statistical Package were used.
Results
Tab. 3 summarizes the physical and chemical properties of the soils analyzed in this study. Soils differed mainly in soil texture (clay amount), in pH and CEC values. Indeed, the soils with a larger content of clays had a greater CEC, pH values and Ca content. No significant differences in organic matter content were detected among the soils analyzed. To test the differences in soil properties among the above classes, one-way ANOVAs (analysis of variance) were applied and class means were compared by Tukey’s test (data not shown). Variables considered for discriminant analysis were normally distributed (Tab. 4) and weakly correlated (Tab. 5). Edaphic variables showing significant departure from normal distribution were not considered for further analysis.
Tab. 4 - Shapiro and Wilks normality test of edaphic variables (original and transformed variables). (a): the normal variables selected (p > 0.01). Untransformed variables (X) have been preferably selected when possible.
Variable | X | √(X) | √(X+0.5) | Ln(X+1) | Log(X) |
---|---|---|---|---|---|
Sand (%) | 0.0007 | 0.0007 | 0.0007 | 0.0007 | 0.0007 |
Silt (%) | 0.0009 | 0.0020 | 0.0019 | 0.0048 | 0.0053 |
Clay (%) | 0.0340 a | 0.0954 | 0.0922 | 0.1395 | 0.1427 |
Ca (meq/100g) | 0.0070 | 0.0027 | 0.0028 | 0.0009 | 0.0009 |
Organic matter (%) | 0.0005 | 0.0005 | 0.0005 | 0.0005 | 0.0005 |
Depth (cm) | 0.0014 | 0.0011 | 0.0011 | 0.0008 | 0.0008 |
Slope (%) | 0.0052 | 0.0132 a | 0.0129 | 0.0222 | 0.0233 |
ALT (m.a.s.l.) | 0.0436 a | 0.0651 | 0.0651 | 0.0881 | 0.0882 |
CEC (meq/100g) | 0.0007 | 0.0030 | 0.0029 | 0.0088 | 0.0096 |
pH | 0.0001 | 0.0001 | 0.0001 | 0.0001 | 0.0001 |
Tab. 5 - Pearson’s correlation coefficients among edaphic variables selected as predictors.
Variables | Clay | √(Slope) | ALT |
---|---|---|---|
Clay | 1.0000 | -0.40276 | 0.61941 |
√(Slope) | - | 1.00000 | -0.62665 |
ALT | - | - | 1.0000 |
Selected predictors for discriminant analysis were clay and slope (after squared root transformation) but not altitude, due its high correlation with other variables (Tab. 6).
Tab. 6 - Coefficients of the linear discriminant functions obtained for site index class estimation of Stone pine stands in Calabria (Southern Italy).
Variables | Site index class | ||
---|---|---|---|
I | II | III | |
Constant | -26.438 | -24.199 | -14.992 |
Clay | 1.654 | 1.439 | 1.342 |
√(slope) | 6.768 | 6.766 | 4.813 |
Plots reclassification into classes using the discriminant functions obtained showed an error rate ranging from 66.67 % (class I) to 0 % (class III - Tab. 7). Overall, stands were misclassified in 38.89% of the cases using the selected predictors and the discriminant functions obtained. The pattern of relationship between the factors considered and the site productivity shows that the lower is the slope, the more important is the texture (Fig. 2) for determining the site index (SI) class. Where slope is over 35%, sites are always classified in class III, while in areas with lower slope clay content strongly influences the classification of the site. Stands on calcareous soils showed no chlorotic or decay clues, but a very low growth in comparison with stands on siliceous soils (Tab. 8).
Tab. 7 - Error rates (%) by site index class for the selected edaphic discriminant rule in Stone pine stands in Calabria (Italy). Error rates have been obtained by cross-validation (see text for more details).
Actual Site Index Class |
Predicted Site Index Class | ||
---|---|---|---|
I | II | III | |
I | 33.33 | 50.00 | 16.67 |
II | 16.67 | 50.00 | 33.33 |
III | 0.00 | 0.00 | 100.00 |
Fig. 2 - Site index classification using clay and slope as classificatory variables for Stone pine stands in Castrovillari (Calabrian Apennines, southern Italy).
Tab. 8 - Mean annual increment in different planted Stone pine stands in Italy. (*): Mean height.
Age (years) |
N (trees/ha) |
Ho (m) |
BA (m2/ha) |
V (m3/ha) |
MAI (m3/ha) |
Substrata | Region | Souce |
---|---|---|---|---|---|---|---|---|
30-40 | - | - | - | 128-180 | 3.6-5.6 | sandstone | Bosco Bellia (Sicily) | [11] |
30-40 | 700-500 | 11-12.6 | 30-32.8 | 165-209 | 8.2-8.7 | sandy | Cecina (Tuscany) | [2] |
35-45 | 350-190 | - | - | 205-383 | 4.8-7.7 | sandy | S.Rossore (Tuscany) | [10] |
30-40 | 711-485 | 12.1-13.4* | 25.7-27.8 | 174-228 | 5.8-5.7 | sandy | Feniglia (Tuscany) | [22] |
45 | 450-763 | 10-12 | 25.1-35.7 | 162-238 | 3.6-5.3 | sandy | Litorale veneto (Veneto) | [14] |
40-50 | 500-350 | 12.6-14.1 | 32.8-34.9 | 209-247 | 8.7-8.6 | sandy | Cecina (Tuscany) | [2] |
40-50 | 485-349 | 13.4-14.4* | 27.8-26.8 | 228-249 | 5.7-4.9 | sandy | Feniglia (Tuscany) | [22] |
40-50 | 544-752 | 9.4-13.5* | 17.5-42.9 | 91-300 | 1.7-7.5 | granite | La Maddalena (Sardinia) | [13] |
40-50 | - | 13.7-16.1* | - | 193-376 | 4.8-6.5 | sandy | Central Italy | [12] |
35-45-55 | 353-877 | 5.2-12.0 | 10.1-44.6 | 45.0-315.8 | 1.0-5.7 | calcareous | Pollino (Calabria) | Present work |
As mentioned before, the error rate using the discriminant functions obtained was lower for poorest sites. On the other hand, a low error rate is more important in the high site index classes. Errors in site index estimation for a high-productivity stands generate a higher bias in the growth and yield predictions than for a low-productivity stand. However, as our discriminant rule classify 100 % of the poorest stands correctly, so it can be used to identify the worst sites to establish Stone pine plantations, helping foresters to avoid these areas as plantation sites.
Discussion and conclusion
In this study, discriminant rules useful to classify Stone pine stand productivity in Calabria (southern Italy) have been developed. In summary, the discriminant model obtained classifies plots into the correct site index class with an overall rate of 61.11 %, which is considered an acceptable value for these kinds of studies.
Models based on discriminant functions using edaphic and climatic factors as site index predictors may help forest managers to classify stand productivity when information on site productivity is not available. Their use may be particularly helpful for site index assessment of young stands, where site index curves cannot be used, as well as for assessing the suitability of an area to Stone pine plantations. However, foresters should accurately plan soil analyzes needed for site index assessment, because their high costs in terms of money and time. Furthermore, some cautions have to be considered to this regards. Our study is based on soil samples from well-established forest stands, and the potential productivity of a stand planted on bare soils may be somewhat hardly evaluated using the models obtained here. Based on our results and the above considerations, priority should be given to low clay sites if there are no other limiting factors.
The use of discriminant functions allows to classify any observations based on its Euclidean distance from two or more groups of observations established a priori, using one or more predicting variables. On the other hand, discriminant analysis does not allow to determine causal relationship among productivity, soil and topographic factors. However, results from this study can help to identify patterns of relationship among soil, topographic factors and forest productivity.
Different statistical methods have been used to find relationship between these site variables and site index: discriminant analysis ([5], [6], tree classification models ([39]), multiple regression ([42]) or principal components analysis ([31]).
In areas showing high silvicultural and environmental variability, the use of models for site class prediction seems more appropriate than using regression models. Verbyla & Fisher ([39]) showed the advantages of using classification methods instead of linear regression analysis when different site-index discriminant rules have been developed in the past ([16], [5], [6]). Moreover, in a highly variable environment, it is easier to assess site class than an exact site index. Our target stands in Calabria show a small silvicultural variability (origin from plantation with no thinning since 20 to 30 years) and great differences in environmental conditions. In the northern temperate zone, slope is an important topographic factor influencing local site microclimate ([32]), as a consequence of the amount of solar radiation received. Solar radiation influences soil temperature and water availability, which in turn affect soil chemical properties. In this investigation slope and soil texture (in terms of clay) were the most relevant variables affecting site productivity.
Wang ([42]) stated that: (1) many nutrients affect tree growth and the limiting nutrient(s) may vary with site; (2) there are complex interactions between nutrients not described by linear relationships; (3) chemical analysis may not give an accurate picture of nutrient availability for tree growth; and (4) usually the nutrient cycle is not considered. The site index in plain sites soils depends strongly on clay percentage. Studying Stone pine stands in southern Spain, Bravo-Oviedo & Montero ([6]) also found a clear association between clay and poor sites, showing that the presence of clay causes bad drainage and aeration of the soil limiting the growth of this species. Our findings are in accordance with the results obtained for Stone pine stands by Boisseau ([3]), Prada et al. ([28]), Bravo-Oviedo & Montero ([6]) and for other Mediterranean forests by Bravo & Montero ([5]).
Bravo-Oviedo & Montero ([6]) stated that the assessment of forest productivity based on soil properties is greatly facilitated when a mature stand is located on reforested soil type, with low parental rock flow, scarce differentiation in horizons and low variability of soil attributes. Previous studies on Mediterranean forests ([5], [6]) have stated that discriminant analysis with site variables, as texture and elevation, is appropriate in stands of Scots pine and Stone pine.
Both regional and local studies are needed in the Mediterranean basin, due to its large ecological variability, as well as to the differences in management background among the different areas. Local case studied may have great significance across the Mediterranean region, where the need of cheap and straightforward management tools may be have important fallout in forest and landscape management.
References
Gscholar
Gscholar
Gscholar
Gscholar
Gscholar
Gscholar
Gscholar
Gscholar
Gscholar
Gscholar
Gscholar
Gscholar
Gscholar
Gscholar
Gscholar
Gscholar
Gscholar
Gscholar
Gscholar
Gscholar
Gscholar
Authors’ Info
Authors’ Affiliation
Sustainable Forest Management Research Institute, University of Valladolid-INIA, avda. de Madrid 57, E-34004 Palencia (Spain)
R Mercurio
M Sidari
A Muscolo
Department of Agricultural and Forest Systems Management, Faculty of Agriculture, University “Mediterranea” (Italy)
Corresponding author
Paper Info
Citation
Bravo F, Lucà M, Mercurio R, Sidari M, Muscolo A (2011). Soil and forest productivity: a case study from Stone pine (Pinus pinea L.) stands in Calabria (southern Italy). iForest 4: 25-30. - doi: 10.3832/ifor0559-004
Paper history
Received: May 03, 2010
Accepted: Dec 17, 2010
First online: Jan 27, 2011
Publication Date: Jan 27, 2011
Publication Time: 1.37 months
Copyright Information
© SISEF - The Italian Society of Silviculture and Forest Ecology 2011
Open Access
This article is distributed under the terms of the Creative Commons Attribution-Non Commercial 4.0 International (https://creativecommons.org/licenses/by-nc/4.0/), which permits unrestricted use, distribution, and reproduction in any medium, provided you give appropriate credit to the original author(s) and the source, provide a link to the Creative Commons license, and indicate if changes were made.
Web Metrics
Breakdown by View Type
Article Usage
Total Article Views: 57105
(from publication date up to now)
Breakdown by View Type
HTML Page Views: 48151
Abstract Page Views: 3460
PDF Downloads: 3923
Citation/Reference Downloads: 24
XML Downloads: 1547
Web Metrics
Days since publication: 5252
Overall contacts: 57105
Avg. contacts per week: 76.11
Article Citations
Article citations are based on data periodically collected from the Clarivate Web of Science web site
(last update: Mar 2025)
Total number of cites (since 2011): 15
Average cites per year: 1.00
Publication Metrics
by Dimensions ©
Articles citing this article
List of the papers citing this article based on CrossRef Cited-by.
Related Contents
iForest Similar Articles
Research Articles
Relationship between environmental parameters and Pinus sylvestris L. site index in forest plantations in northern Spain acidic plateau
vol. 9, pp. 394-401 (online: 16 January 2016)
Research Articles
Assessment of age bias in site index equations
vol. 9, pp. 402-408 (online: 11 January 2016)
Research Articles
Potential impacts of regional climate change on site productivity of Larix olgensis plantations in northeast China
vol. 8, pp. 642-651 (online: 02 March 2015)
Research Articles
Relationship between microbiological, physical, and chemical attributes of different soil types under Pinus taeda plantations in southern Brazil
vol. 17, pp. 29-35 (online: 28 February 2024)
Research Articles
Site quality assessment of degraded Quercus frainetto stands in central Greece
vol. 8, pp. 53-58 (online: 12 May 2014)
Research Articles
The spread of the non-native pine tortoise scale Toumeyella parvicornis (Hemiptera: Coccidae) in Europe: a major threat to Pinus pinea in Southern Italy
vol. 11, pp. 628-634 (online: 04 October 2018)
Research Articles
The effect of soil conditions on submountain site suitability for Norway spruce (Picea abies Karst.) in Central Europe
vol. 16, pp. 210-217 (online: 31 July 2023)
Research Articles
Effect of forwarder multipassing on forest soil parameters changes
vol. 15, pp. 476-483 (online: 24 November 2022)
Short Communications
Growth of Stone pine (Pinus pinea L.) European provenances in central Chile
vol. 10, pp. 64-69 (online: 29 August 2016)
Research Articles
The effect of clear-cut age on soil organic carbon and nitrogen indices in Scots pine (Pinus sylvestris L.) stands
vol. 18, pp. 146-153 (online: 09 June 2025)
iForest Database Search
Search By Author
Search By Keyword
Google Scholar Search
Citing Articles
Search By Author
Search By Keywords
PubMed Search
Search By Author
Search By Keyword