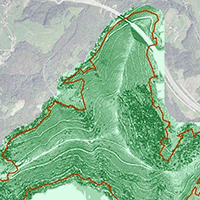
Large scale semi-automatic detection of forest roads from low density LiDAR data on steep terrain in Northern Spain
Covadonga Prendes (1) , Sandra Buján (2), Celestino Ordoñez (3), Elena Canga (1)
iForest - Biogeosciences and Forestry, Volume 12, Issue 4, Pages 366-374 (2019)
doi: https://doi.org/10.3832/ifor2989-012
Published: Jul 05, 2019 - Copyright © 2019 SISEF
Research Articles
Abstract
While forest roads are important to forest managers in terms of facilitating the exploitation of wood and timber, their role is far more multifunctional. They permit access to emergency services in the case of forest fires as well as acting as fire breaks, enhance biodiversity, and provide access to the public to enjoy recreational activities. Detailed maps of forest roads are an essential tool for better and more timely forest management and automatic/semi-automatic tools allow not only the creation of forest road databases, but also enable these to be updated. In Spain, LiDAR data for the entire national territory is freely available, and the capture of higher density data is planned in the next few years. As such, the development of a forest road detection methodology based on LiDAR data would allow maps of all forest roads to be developed and regularly updated. The general objective of this work was to establish a low density LiDAR data-based methodology for the semi-automatic detection of the centerline of forest roads on steep terrain with various types of canopy cover. Intensity and slope images were generated using the currently available LiDAR data of the study area (0.5 points m-2). Two image classification approaches were evaluated: pixel-based and object-oriented classification (OBIA). The LiDAR-derived centerlines obtained with the two approaches were compared with the real centerlines which had previously been digitized in the field. The road width, type of surface and type of vegetation cover were also recorded. The effectiveness of the two approaches was evaluated through three quality indicators: correctness, completeness and quality. In addition, the accuracy of the LiDAR-derived centerlines was also evaluated by combining GIS analysis and statistical methods. The pixel-based approach obtained higher values than OBIA for two of the three quality measures (correctness: 93% compared to 90%; and quality: 60% compared to 56%) as well as in terms of positional accuracy (± 5.5 m vs. ± 6.8 for OBIA). The results obtained in this study demonstrate that producing road maps is among the most valuable and easily attainable products of LiDAR data analysis.
Keywords
GIS, Pixel-based Classification, OBIA, Quality Measures, Forest Roads Network, Accuracy Assessment
Authors’ Info
Authors’ address
Elena Canga 0000-0003-2132-9991
CETEMAS, Centro Tecnológico y Forestal de la Madera, Área de Desarrollo Forestal Sostenible. Pumarabule, Carbayín Bajo s/n 33936 Siero (Spain)
Laboratorio do Territorio (LaboraTe), Universidad de Santiago de Compostela. C/ Benigno Ledo Campus Universitario 27002 Lugo (Spain)
Departamento de Explotación de Minas, Grupo de Investigación en Geomática y Computación Gráfica (GEOGRAPH), Universidad de Oviedo, 33004 Oviedo (Spain)
Corresponding author
Paper Info
Citation
Prendes C, Buján S, Ordoñez C, Canga E (2019). Large scale semi-automatic detection of forest roads from low density LiDAR data on steep terrain in Northern Spain. iForest 12: 366-374. - doi: 10.3832/ifor2989-012
Academic Editor
Agostino Ferrara
Paper history
Received: Nov 05, 2018
Accepted: Apr 15, 2019
First online: Jul 05, 2019
Publication Date: Aug 31, 2019
Publication Time: 2.70 months
Copyright Information
© SISEF - The Italian Society of Silviculture and Forest Ecology 2019
Open Access
This article is distributed under the terms of the Creative Commons Attribution-Non Commercial 4.0 International (https://creativecommons.org/licenses/by-nc/4.0/), which permits unrestricted use, distribution, and reproduction in any medium, provided you give appropriate credit to the original author(s) and the source, provide a link to the Creative Commons license, and indicate if changes were made.
Web Metrics
Breakdown by View Type
Article Usage
Total Article Views: 43246
(from publication date up to now)
Breakdown by View Type
HTML Page Views: 36755
Abstract Page Views: 2765
PDF Downloads: 3138
Citation/Reference Downloads: 3
XML Downloads: 585
Web Metrics
Days since publication: 2098
Overall contacts: 43246
Avg. contacts per week: 144.29
Citation Metrics
Article Citations
Article citations are based on data periodically collected from the Clarivate Web of Science web site
(last update: Mar 2025)
Total number of cites (since 2019): 15
Average cites per year: 2.14
Publication Metrics
by Dimensions ©
Articles citing this article
List of the papers citing this article based on CrossRef Cited-by.
References
Análisis de imágenes basado en objetos (OBIA) y aprendizaje automático para la obtención de mapas de coberturas del suelo a partir de imágenes de muy alta resolución espacial: aplicación en la Unidad de Demanda Agraria n° 28, cabecera del Argos [Object-based image analysis (OBIA) and automatic learning for obtaining land cover maps from very high spatial resolution images: application in the Agrarian Demand Unit No. 28, head of the river Argos]. PhD thesis, Geography Department, University of Murcia, Murcia, Spain, pp. 204. [in Spanish]
Online | Gscholar
The design and implementation of an integrated geographic information system for environmental applications. Photogrammetric Engineering and Remote Sensing 61: 1393-1404.
Gscholar
Fourth national forest inventory, Asturias. General Directorate of Rural Development and Forest Policy, Ministry of Agriculture, Food and Environment, Madrid, Spain, pp. 58.
Gscholar
Evaluating automated road extraction in different operational modes. In: Proceedings of the Conference “Algorithms and Technologies for Multispectral, Hyperspectral, and Ultraspectral Imagery XV”. Orlando (FL, USA) 14-16 Apr 2009. SPIE Defense, Security, and Sensing, vol. 7334, no. 73341A.
CrossRef | Gscholar
Linear feature extraction with 3-D LSB-snakes. In: “Automatic Extraction of Man-Made Objects from Aerial and Space Images (II). Monte Verità (Proceedings of the Centro Stefano Franscini Ascona)” (Gruen A, Baltsavias EP, Henricsson O eds). Birkhäuser, Basel, Switzerland, pp. 287-298.
CrossRef | Gscholar
Sistema cartográfico nacional [National cartographic system]. Instituto Geográfico Nacional, Comunidades Autónomas de Cantabria, País Vasco / Euskadi y Comunidad Foral de Navarra, Spain. [in Spanish]
Gscholar
FUSION/LDV: a software for LiDAR data analysis and visualization. USDA Forest Service, Pacific Northwest Research Station, Seattle, WA, USA.
Gscholar