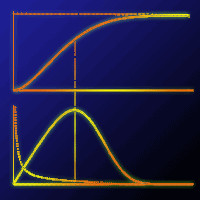
Properties and prediction accuracy of a sigmoid function of time-determinate growth
Róbert Sedmák (1-2), Lubomír Scheer (1)
iForest - Biogeosciences and Forestry, Volume 8, Issue 5, Pages 631-637 (2015)
doi: https://doi.org/10.3832/ifor1243-007
Published: Jan 13, 2015 - Copyright © 2015 SISEF
Research Articles
Abstract
The properties and short-term prediction accuracy of mathematical model of sigmoid time-determinate growth, denoted as “KM-function”, are presented. Comparative mathematical analysis of the function revealed that it is a model of asymmetrical sigmoid growth, which starts at zero size of an organism and terminates when it reaches its final size. The function assumes a finite length of the growth period and includes a parameter interpretable as the expected lifespan of the organism. Moreover, the possibility for growth curve inflexion at any age is possible, so the function can be used for modelling of S-shaped growth trajectories with various degree of asymmetry. These good theoretical predispositions for realistic growth predictions were empirically evaluated. The KM-function used in three and four-parameter forms was compared with three classical (Richards, Korf and Weibull) growth functions employing two parameterisation methods - nonlinear least squares (NLS) and Bayesian method. The evaluation was conducted on the basis of the tree diameter series obtained from stem analyses. The main empirical findings are: (i) if the minimisation of the prediction bias is required, the KM-function in three-parameter form in connection with Bayes parameterisation can be recommended; (ii) if the minimisation of root square error (RMSE) is required, the best short-term prediction results for a particular dataset were obtained with four-parameter Weibull function employing NLS parameterisation; (iii) moreover, three-parameter functions parameterised by Bayesian methods show a considerably smaller RMSE by 15-25% as well as smaller biases by 40-60% than four-parameter functions employing NLS. Overall, all analyses confirmed relative usefulness of the KM-function in comparison with classical growth functions, especially in connection with Bayesian parameterisation.
Keywords
Growth Function, Determinate Growth, Nonlinear Least Squares, Bayes, Prediction
Authors’ Info
Authors’ address
Lubomír Scheer
Faculty of Forestry, Technical University in Zvolen, T.G. Masaryka 24, 960 53 Zvolen (Slovak Republic)
Faculty of Forestry and Wood Sciences, Czech University of Life Sciences Prague, Kamýcká 1176, 165 21 Praha 6 - Suchdol (Czech Republic)
Corresponding author
Paper Info
Citation
Sedmák R, Scheer L (2015). Properties and prediction accuracy of a sigmoid function of time-determinate growth. iForest 8: 631-637. - doi: 10.3832/ifor1243-007
Academic Editor
Renzo Motta
Paper history
Received: Jan 15, 2014
Accepted: Oct 17, 2014
First online: Jan 13, 2015
Publication Date: Oct 01, 2015
Publication Time: 2.93 months
Copyright Information
© SISEF - The Italian Society of Silviculture and Forest Ecology 2015
Open Access
This article is distributed under the terms of the Creative Commons Attribution-Non Commercial 4.0 International (https://creativecommons.org/licenses/by-nc/4.0/), which permits unrestricted use, distribution, and reproduction in any medium, provided you give appropriate credit to the original author(s) and the source, provide a link to the Creative Commons license, and indicate if changes were made.
Web Metrics
Breakdown by View Type
Article Usage
Total Article Views: 52903
(from publication date up to now)
Breakdown by View Type
HTML Page Views: 43908
Abstract Page Views: 2323
PDF Downloads: 5279
Citation/Reference Downloads: 42
XML Downloads: 1351
Web Metrics
Days since publication: 3732
Overall contacts: 52903
Avg. contacts per week: 99.23
Citation Metrics
Article Citations
Article citations are based on data periodically collected from the Clarivate Web of Science web site
(last update: Mar 2025)
Total number of cites (since 2015): 2
Average cites per year: 0.18
Publication Metrics
by Dimensions ©
Articles citing this article
List of the papers citing this article based on CrossRef Cited-by.
References
Parameter estimation in the context of nonlinear longitudinal growth models. In: “Methods in Human Growth Research” (Hauspie RC, Cameron N, Molinari L eds). Series Cambridge Studies in Biological and Evolutionary Anthropology, vol. 39, Cambridge University Press, Cambridge, UK, pp. 198-220.
Gscholar
Bayes and empirical Bayes methods for data analysis. Texts in Statistical Science, Chapman & Hall/CRC, Boca Raton, FL, USA, pp. 440.
Gscholar
A growth and yield tables of main tree species in Slovakia. Slovak Academic Press, Bratislava, SK, pp. 325. [in Slovak]
Gscholar
Mathematical and statistical research of diameter structures of Slovak stands. Lesnícky časopis 3 (1): 39-74. [in Slovak]
Gscholar
Mathematical functions of forest growth. Estonian Agriculture Academy, Tartu, Estonia, pp.108.
Gscholar
Contribution to mathematical definition of the law of stand volume growth. Manuscript, Lesnická práce, Slovakia, pp. 339-379.
Gscholar
Problems of plant growth. Landwirtschaftliche Jahrbücher 53: 167-182. [in German]
Gscholar
Forestry dendrology. Technical University in Zvolen, Zvolen, Slovakia, pp. 347. [in Slovak]
Gscholar
Nonlinear regression. Wiley series in probability and statistics, John Wiley & Sons, Inc., New Jersey, USA, pp. 792.
Gscholar
Growth and yield modelling of beech trees and stands. Msc Thesis, Technical University in Zvolen, Zvolen, Slovakia, pp. 181.
Gscholar
Investigation of growth processes using first order differential equations. Mitteilungen der Baden-Württembergischen Forstlichen Versuchs und Forschungsanstalt 32: 1-109. [in German]
Gscholar
A note on population growth. Correspondence Mathematiques et Physiques 10: 113-121. [in French]
Gscholar
A statistical distribution of wide applicability. Journal of Applied Mechanics 18: 293-297.
Gscholar