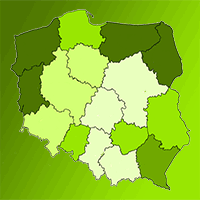
Contribution of anthropogenic, vegetation, and topographic features to forest fire occurrence in Poland
iForest - Biogeosciences and Forestry, Volume 15, Issue 4, Pages 307-314 (2022)
doi: https://doi.org/10.3832/ifor4052-015
Published: Aug 23, 2022 - Copyright © 2022 SISEF
Research Articles
Abstract
Climate is one of the main causes of forest fires in Europe. In addition, forest fires are influenced by other factors, such as the reconstruction of tree stands with a uniform species composition and increasing human pressure. At the same time, the increasing number of fires is accompanied by a steady increase in the number and quality of spatial information collected, which affects the ability to conduct more accurate studies of forest fires. The appropriate use of spatial information systems (GIS) together with all the collected information on fires could provide new insights into their causes and, in further steps, allow the development of new, more accurate predictive models. The objectives of the study were: (i) to estimate the probability of fire occurrence in the period 2007-2016; (ii) to evaluate the performance of the developed model; (iii) to identify and quantify anthropogenic, topographic and stand factors affecting the probability of fire occurrence in forest areas in Poland. To achieve these objectives, a statistical model based on a logistic regression approach was built using the nationwide forest fire database for the period from 2007 to 2016. The information in the database was obtained from the Polish State Forest Information System (SILP). Then it was supplemented with spatial, topographic and socio-economic information from various spatial and statistical databases. The results showed that fire probability is significantly positively affected by population density and distance from buildings. In addition, the further the distance from roads and railways, watercourses and water objects or the edge of the forest, height above sea level, and steep slopes, the lower is the fire probability. Analysis of spatial, ecological and socio-economic factors provides new insights that contribute to a better understanding of fire occurrence in Poland.
Keywords
Forest Fires, Logistic Regression, Variables Selection, Anthropogenic Factors
Introduction
Forest fires are a major cause of forest environmental degradation and an important contributor to global carbon emissions ([28]). Our current understanding of the causes of forest fires may have a fundamental impact on the stability of forest ecosystems. Due to the increase in the number and severity of fires that has been observed for many years ([28]), the damage caused by large fires in particular is increasingly recognized as a factor affecting forest ecosystems ([2]). Forest fires negatively affect soil properties and microclimate and are one of the most important forest disturbances ([24]). In Central Europe, large fires are rare, partly due to forest characteristics and the long tradition of fire suppression ([2]). However, the increasing number of small fires in recent years has led to the recognition of forest fires as an important factor influencing the ecosystem in this region, and research questions on this topic have become extremely important ([1], [2]).
Poland is classified as one of the countries with average forest fire risk in the European Union ([14]). However, according to the European Forest Fire Information System of the Joint Research Center at Ispra, Italy ([33]), it ranks third in terms of the average annual number of fires (n=7188) after Portugal and Spain. The peculiarity of fires in Poland is their large number on a relatively small area ([37], [38]). The causes of forest fires are often difficult or impossible to determine, but in Poland the probability of natural ignition is low. Therefore, direct and indirect human influence on the causation of forest fires is most often considered and seems to be the dominant factor ([37]). According to the data of the National Forest Fire Information System, 41.2% of fires are caused by arson, 14.2% by human carelessness, while in 40.3% of fires, the cause remained unknown ([42]).
It is not only in Poland that human factors are of great importance in evaluating the fires occurrence. Accidents/negligence or arson are also cited as one of the main causes of forest fires in many other European countries ([33]). The European Fire Database indicates that this type of cause was reported for about 70% of all fires. In Central Europe the situation is similar. In general, according to Ganteaume et al. ([15]), 97.1% of fires in Europe are directly or indirectly caused by humans when all European regions are considered. The research conducted so far has largely made it possible to identify the human factors that influence the fire occurrence. The first models only dealt with demographic indicators and forest availability ([11]). As technical capabilities have developed, spatial dependencies between fires and factors such as distance from roads and railroads, valuable natural features, recreation areas, and buildings have also been studied ([12], [30]). In recent years, socioeconomic variables such as unemployment rate and population density have also been considered in modeling fire probability ([24]). To date, numerous statistical models have been used to model fire occurrence. The most common is the binary logistic regression method. According to Costafreda-Aumedes et al. ([11]), it is widely used because of its relative ease of application and understandability. Fire occurrence modeling has also been performed using machine learning methods, such as Random Forest ([30]), Support Vector Machines ([17]), or Generalized Additive Models ([29]). Jain et al. ([22]) noted that since 1990, more than 300 articles have been published using machine learning in modeling fire occurrence. Costafreda-Aumedes et al. ([11]), who analyzed 152 papers in the field, showed that the modeling methods themselves were not identical. The researchers used both binary (absence or presence of fire) and numeric (number of fires) as dependent variables. This illustrates the wide variety of research and the complexity of the topic. The relationship between the factors and fire occurrence also depends strongly on the region. It is necessary to identify the local factors that determine the occurrence of fires.
Due to the increase of forest fires in Poland in recent years, it is important to learn about additional groups of factors that may influence the fire occurrence ([37], [38]). The studies conducted so far in Poland have mainly used weather, climate, and tree stand variables to model fire occurrence ([38]). The degree of fire occurrence is determined at 9:00 a.m. and at 1:00 p.m. based on meteorological parameters (air temperature, relative humidity, and total daily precipitation) and the moisture content of pine litter, which is an indicator of combustible material due to the dominant proportion of pine trees in Polish forests. Based on the values of meteorological parameters predicted by the numerical weather prediction model (COSMO) and the expected moisture content of combustible material, the forest fire occurrence is also predicted up to 24 hours in advance ([38]).
So far, spatial, topographic, and socio-economic factors have been largely ignored in research in Poland. Considering this, and taking into account previous research results from other European countries ([1], [2], [30]), which present different results for different research areas, it is reasonable to conduct similar research for Poland ([38]).
The aim of the study was: (i) to estimate the probability of fire occurrence over the period 2007-2016; (ii) to evaluate the performance of the model; (iii) to identify and quantify anthropogenic, topographic and stand factors affecting the probability of fire occurrence in forest areas in Poland.
Material and methods
Study area
The study area included Polish State Forests (7354.8 thousand ha, 76.9% of all forests in the country). The analyses were performed on the basis of data on forest fires collected between Jan 1, 2007 and May 31, 2016 (Fig. 1). According to Statistics Poland, 29.6% of the country’s area was forested in 2017, with significant regional differences (from 21.5% in Lódzkie Voivodeship to 49.3% in Lubuskie Voivodeship). Statistically, there were 0.24 ha of forest per inhabitant, ranging from 0.09 ha in Šlaskie Voivodeship to 0.7 ha in Lubuskie Voivodeship ([35]). As shown by the statistical data of the National Forest Fire Information System, about 85% of the forest area in Poland is susceptible to fire ([25]). The threat of such a large area of Polish forests to fire is mainly due to the following factors: the predominance of conifers (68.4%), including pines, which are particularly vulnerable to fire; the large share of the poorest coniferous habitats (49.8%); climate change characterised by weather anomalies; the increasing availability and penetration of forests by humans for recreational and commercial purposes.
Fig. 1 - Polish forests (A), location of forest fires in Polish State Forests (1.01.2007 - 31.05.2016) (B) and regional variability of forest area per inhabitant (C).
Forest fires database and stand factors
Information on forest fires was obtained from the Polish State Forest Information System (SILP). In the analyzed period (Jan 01, 2007 to May 31, 2016 - Fig. 2), a total of 25.660 fires were registered. Each fire was identified by its coordinates, date of occurrence, cause of fire (e.g., arson), and forest stand description (habitat, type of ground cover, dominant tree species, proportion of dominant tree species, age and average height of dominant tree species, second dominant tree species, vertical structure, understory information) according to the applicable standard of the European Forest Fire Information System ([32]). The dataset does not include fires in forests with other ownerships (e.g., private forests, national parks). Only fires of anthropogenic origin with a specific cause for their occurrence were selected for further analysis (n=18.167).
Fig. 2 - Number of forest fires in each year of the studied period. Data for 2016 come only from January-May.
Topographic factors
The location of each fire in relation to topography was compiled based on freely available geospatial data (see Tab. S1 in Supplementary material). The topographic variables: elevation above sea level, terrain aspect, and slope were obtained from Shuttle Radar Topography Mission (SRTM) data. The average accuracy of the SRTM elevation model for Europe is 6.2 m. According to Karwel ([23]), the absolute accuracy of SRTM data for Poland is 3.6 m for flat areas and 4.1 m for areas with slopes from 2° to 6°. A plot aspect originally expressed as azimuth in degrees was converted into numerical values according to the approach proposed by Socha ([34]).
Spatial factors
The planar distance of the fire from the nearest railroad tracks, roads, fire access roads, surface water bodies, and rivers was determined based on the OpenStreetMap and Forest Digital Map combined databases. Two other freely available data sources, CORINE Land Cover 2012 and protected areas layer (nature reserve, landscape park, protected landscape area) of the Polish General Directorate of Environmental Protection, allowed determining the distance of the fire from urban areas and protected natural objects. The distance to the nearest 1 km grid (number of people>0) with demographic data from Statistics Poland was also calculated.
Socio-economic data
Municipality level data (level 2 according to Local Administrative Unit - LAU), such as population density (persons km-2), net migration rate (number of persons), number of persons living in urban areas, unemployment rate (%), average per capita income (PLN person-1), were extracted from the Statistics Poland Local Data Bank and aggregated to the forest district level. Aggregation was done by calculating the weighted average of each characteristic for the given forest districts, where the weight corresponded to the share of the municipality area in the total area of the forest district.
Spatial analyses
The data on fires contained information on the location of their occurrence, so it was possible to use the “Extract Values to Points” tool of ArcGis® ver. 10.5 (ESRI, Redwoods, CA, USA) to obtain variables from grids that contained data on topography. The distance of the fire to the selected spatial objects was calculated using the “Near” tool in ArcGis®. Statistical information was assigned to the forest fire layer based on the combination of forest districts and attribute tables of the forest fire layer according to the spatial identifier (Fig. 3). Similar statistics were also assigned to randomly generated points where no fire was detected (n = 20.394).
Fig. 3 - Spatial information assigned to fires and random points. (SRTM): Shuttle Radar Topography Mission; (OSM/LMN): OpenStreetMap/Forest Digital Map; (CLC): CORINE LandCover.
Statistical analyses
Preliminary statistics analysis
In the first step of data processing, a content analysis of the collected material were conducted, including elimination of incomplete observations from the database, aggregation of some variables, i.e., type of habitat, share of species in a stand, age of the species, etc., and measuring scales. Then preliminary statistical analysis were performed, i.e., eliminated variables with a low degree of variation (level of v = 0.10), eliminated variables that were poorly correlated with the dependent variable, and finally selected variables for study using forward step regression. The values of the Spearman’s correlation coefficients between these variables were calculated (α = 0.05). At this stage of selecting characteristics (variables) for the study, statistically non-significant variables were eliminated. Tab. S2 (Supplementary material) provides estimates of the basic characteristics of the distribution (mean, standard deviation, coefficient of variation, asymmetry, kurtosis) for the remaining characteristics. In estimating the parameters of the logit model, the final selection of characteristics (17 in total) was made using forward stepwise regression. The descriptions and definitions of the quantitative and qualitative variables are given in Tab. S1 (Supplementary material).
Qualitative variables were coded as dummy variables after aggregation of detailed values (indicator coding, see [20]). The group of units for which all other predictors are zero is called the reference group. The reference group for the interpretation of the model parameters was arbitrarily determined ([10]). This was a group of records for pine stands growing on oligotrophic sites (species_1, habitat_1).
The logit model
In analyzing the empirical material, the use of different statistical models for qualitative binomial variables (fire/no fire) was tested. First, the usefulness of the following models was analyzed: logit, probit, LPM (Linear Probability Model), and Poisson and Cox regression models. Since Cox models are concerned with estimating the duration of the dependent variable and Poisson regression models are models based on a variable that is a counter, they were not used. The content analysis supported by the statistical analysis showed that the best model for the analysis of the empirical material is the logistic regression model. In the study, we used logit models for qualitative dependent variables in the single-equation econometric model ([10], [5]). The dichotomous qualitative dependent variable Y was assigned one of two values: 0 - no fire, 1 - occurrence of fire. A total of 54 variables expressed in different measurement scales were used as independent variables (predictors, X in the model). The cumulative distribution function of the logistic distribution (the S-curve) in the model used for the analysis has the following form (eqn. 1, eqn. 2):
where pi is probability of the event occurrence, and Zi = xi β. In the logistic distribution with the expected value of 0 and variance equal to π2/3, the probability density function has the following form (eqn. 3):
and the inverse function to the F function is (eqn. 4):
This expression is called logit, and the model a logit model. Logit is a logarithm of the odds ratio of Yi variable to have and not to have the value of 1 (log-odds ratio). For pi = 0.5, logit = 0, for pi <0.5 logit is negative and for pi > 0.5 logit is positive. The final form of the logit model used is the followig (eqn. 5):
Estimation of the logit model parameters was done using maximum likelihood method (MLM) in the GRETL software package ([26]).
The Maximum Likelihood (MWW) method is a commonly used estimation method for models of various types in large samples. It is primarily convenient, available in all statistical packages that use some version of the numerical algorithm to estimate the parameters (the Newton-Raphson method or the scoring method, depending on the package), and satisfies a number of desired theoretical properties. The MNW estimator is a consistent, asymptotically effective, and unbiased parameter as it achieves the Rao-Cramer’s lower bound and is invariant.
The results of the estimation have been interpreted for marginal effects of X conversion to pi value (sensitivity of pi probability to exogenous variables) according to the following formula (eqn. 6):
Estimated marginal effects shown in the Tab. 1 are interpreted as an increase of probability with an increase of each variable by one unit above its average level, which is called MEM (marginal effects at the mean). Apart from MEM, it is also possible to estimate MER (marginal effects at representative value) and AME (average marginal effects).
Tab. 1 - Estimated marginal effects of the logit model. Prediction accuracy: y = 1 - n11/n1; y = 0 - n00/n0; Odds ratio(OR) = (n11·n00)/(n01·n10), OR>1 denotes better classification. ROC (receiver operating characteristic) curve - minimisation of the α+β sum.
Observed | Predicted | Total | |
---|---|---|---|
Ŷ = 1 | Ŷ = 0 | ||
Y=1 | n11 | n10 | n1. |
Y=0 | n01 | n00 | n0. |
Total | n.1 | n.0 | N |
The results of the estimation have also been interpreted for the odds ratio defined as follows ([21], [19] - eqn. 7):
The advantage of the logit model is the possibility of interpretation of convenient parameter eβ using the “odds ratio” term, which is a ratio of probability of the event to occur and probability that it does not occur. In the case of the intercept, eβo value is usually interpreted as an odd of the phenomenon occurrence in the reference group (all variables and their products equal zero).
Probability of the fire occurrence was estimated using the following formula (eqn. 8):
Model fit measures and parameter interpretation
In general, there are two approaches to measure the choice of the right model. According to Allison ([4]), the first is to obtain a measure of how well the dependent variable can be predicted based on the independent variables. The other is to test whether the model needs to be more complex, i.e., whether it needs additional nonlinearities and interactions to represent the data satisfactorily.
Three popular large-sample significance tests based on MNW were used. These tests are: Likelihood ratio test (LR), Wald test, and Rao scoring test (also known as Lagrange multiplier test - LM). In all three tests, the null hypothesis assumes that the parameters of the model meet the overall condition g(θ) = 0m × 1. A special case is the linear condition Rθ - r = 0m × 1. The m lines of the vector g(θ) (and matrices R and r) denotes the number of independent conditions imposed on the parameters of the model that the condition g(θ) = 0 is not fulfilled.
The goodness-of-fit of the model to empirical data was performed using the following measures: (i) the McFadden pseudo-R2 coefficient based on the likelihood ratio (eqn. 9):
where Lww is the likelihood function maximum, if the function is maximised with all parameters, while Lp is the maximum under maximisation under the following condition βi = 0 for i = 1, 2, …, k ([27]); thus, the measure is based on the comparison of the full model and the model reduced to the intercept only; (ii) the Maddala coefficient R2 ([27]) which illustrate the share of the correctly predicted cases in all cases (% - eqn. 10):
It shows a combination of both types of errors for different c-value thresholds, i.e., the relationship between the 1-α level (the proportion of true positives that are correctly identified, the proportion of correct y=1 predictions, referred to as sensitivity) on the vertical axis and the β level (the proportion of true negatives that are correctly identified, the proportion of correct y=0 predictions, referred to as specificity) on the horizontal axis.
After estimating the parameters of the logit model using the maximum likelihood method (MLM), which maximizes the logarithm of the likelihood with respect to the model parameters ([20]), the predictor variables were selected using forward stepwise regression, taking into account the significance of the partial Snedecor F value and the values of the partial correlation coefficients ([13]). A collinearity test between predictors (independent variables) was performed using the VIF (Variance Inflation Factor) coefficient and Belsley-Kuh-Welsch collinearity diagnostics.
In all the models obtained, the coefficients marked “β” are slope coefficients. They are interpreted as the change (increase or decrease, depending on the sign of the coefficient) in the dependent variable [logit(y) in our case] when a given predictor changes by one unit or one class.
For dummy variables, their slopes can be interpreted in terms of the intercept, assuming that all other predictors are constant. A positive slope value means that the log likelihood of fire in forest intercepts is increasing for a given dummy variable. A negative slope value means the opposite trend.
Results
Model summary
The summary of the logit model MLM estimation is shown in Tab. 2. The collinearity test revealed no significant correlation between the independent variables. The VIF coefficient was less than 2.3 in all cases examined. The Belsley-Kuh-Welsch collinearity diagnostic also revealed no collinearity between the variables (the sum of the variance proportion columns is 1.0). All model parameters are statistically significant, and the model has a high predictive accuracy of 99.8%. The parameters of the resulting model are listed in Tab. 3.
Tab. 2 - Summary of the logit model MLM estimation
Statistics | Value |
---|---|
Average dependent variable | 0.4869 |
Standard deviation | 0.4998 |
McFadden R2 | 0.9891 |
Sensitivity | 0.9968 |
Specificity | 0.9984 |
AUC | 0.9998 |
Number of correctly predicted cases | 37.214 (99.8%) |
Tab. 3 - Parameters of the logit model. For the explanation of variables, see text.
Variable | β coeff. |
Std. Error |
Z | P>z | Odds ratio |
Prob. | Marginal effect |
---|---|---|---|---|---|---|---|
intercept | 0.2754 | 0.3253 | 0.8464 | 0.3973 | 1.3170 | 0.5684 | - |
habitat_8 | -2.1868 | 0.6847 | -3.1940 | 0.0014 | 0.1123 | 0.1009 | -0.0077 |
habitat_9 | -1.9395 | 0.4628 | -4.1900 | <0.0001 | 0.1438 | 0.1257 | -0.0047 |
species_2 | -0.9857 | 0.4154 | -2.3730 | 0.0177 | 0.3732 | 0.2718 | -0.0016 |
species_3 | -2.0216 | 0.7371 | -2.7420 | 0.0061 | 0.1324 | 0.1170 | -0.0064 |
species_6 | -3.8933 | 1.9607 | -1.9860 | 0.0471 | 0.0204 | 0.0200 | -0.0416 |
population_density | 0.0012 | 0.0003 | 3.6990 | 0.0002 | 1.0012 | 0.5003 | 0.0000 |
distance_road | -0.0116 | 0.0025 | -4.5600 | <0.0001 | 0.9885 | 0.4971 | 0.0000 |
distance_railway | -0.0002 | 0.0000 | -6.7280 | <0.0001 | 0.9998 | 0.4999 | 0.0000 |
distance_buiding | 0.0192 | 0.0019 | 9.9740 | <0.0001 | 1.0194 | 0.5048 | 0.0000 |
asl | -0.0034 | 0.0011 | -3.0540 | 0.0023 | 0.9966 | 0.4992 | 0.0000 |
slope | -0.3183 | 0.0317 | -10.0500 | <0.0001 | 0.7274 | 0.4211 | -0.0003 |
aspect | 0.3410 | 0.0296 | 11.5100 | <0.0001 | 1.4064 | 0.5844 | 0.0004 |
distance_water | -0.0008 | 0.0002 | -5.3200 | <0.0001 | 0.9992 | 0.4998 | 0.0000 |
distance_edge | -0.0156 | 0.0019 | -8.1910 | <0.0001 | 0.9845 | 0.4961 | 0.0000 |
population_grid | 0.0018 | 0.0003 | 6.3600 | <0.0001 | 1.0018 | 0.5004 | 0.0000 |
cover_5 | -1.2335 | 0.4127 | -2.9890 | 0.0028 | 0.2913 | 0.2256 | -0.0022 |
share_2 | 1.9055 | 0.9144 | 2.0840 | 0.0372 | 6.7230 | 0.8705 | 0.0009 |
Stand factors
The analysis results presented in Tab. 3 refer to the reference level (intercept), where pine stands (species_1) with dominant (> 50%) share of pine (share_1) on dry and fresh coniferous and mixed coniferous sites (habitat_1) and with leaf litter on the groud (cover_1) were assumed, which is an example of the most flammable forest type in Poland ([6]). The estimated positive parameters of the logit model (column β-coefficient in Tab. 3), apart from the intercept, show that the increase of these factors, i.e., share_2, by on unit, favors the occurrence of fire compared to the reference level.
The studies conducted showed that among the factors studied, the ratio of the probability of fire occurrence was most strongly associated with the stands where oaks have a dominant share (share_2). Fire outbreak on montane broadleaved, mixed broadleaved and riparian sites, and mixed coniferous, broadleaved and mixed broadleaved sites (habitat_8 and habitat_9) is much less likely than on the reference sites (10% and 13% vs. 57%, respectively). The main tree species, oak (species_2), beech (species_3), and alder (species_6), have a much lower probability of fire occurrence than pine forests (reference group) unless the proportion of oak in the species composition (share_2) exceeds 50%.
Factors that may reduce the likelihood of fire occurrence (value of the logit function) and consequently the likelihood of fire in relation to the intercept (i.e., pine stands in coniferous forest sites) are the main tree species and habitat.
Compared to coniferous forest sites, the probability of fire occurrence, is lower at sites such as: montane broadleaved, mixed broadleaved, riparian sites, upland mixed coniferous, broadleaved, mixed broadleaved sites and montane forest sites. For the remaining sites, the probability of fire occurrence is not significantly different from the probability for coniferous forest sites. Compared to the reference species group 1 (pines and larch), the significant decrease in the model value of fire probability was found for species group 2 (oaks). Species group 3 (European beech) have an even lower value of fire probability, and the lowest value is characteristic of black alder and grey alder stands, although the difference is very small compared to beech. For the other species groups, the logit value is not significantly different from the value observed in pine stands (group 1). In addition, the probability of fire is also influenced by the soil cover. A significantly lower modelled value of the fire probability was found for a heavily turfed cover (cover_5).
Topography and human factors
The fire probability is significantly positively affected by “population_density”, “population_grid” and “distance_buiding” (the more people and the greater the distance from buildings, the higher the probability - Tab. 3). In addition, the greater the distance from roads and railroads (“distance_road”, “distance_railway”), watercourses and water objects or forest edge (“distance_water”, “distance_edge”), the higher the elevation (“asl”) and the steeper the slope, the lower the fire probability. The obtained results also show that the probability of fire outbreak is also high when the transformed aspect is increased by one unit. An increase in fire occurrence ratio was also found for the distance of buildings and population density (odds ratio > 1), with a decrease in the case of the distance of roads and railroads (odds ratio < 1). However, in all the above cases, the probability of fire outbreak was at a similar high level.
Discussion
Selection of the statistical model
There are two acceptable approaches to statistical modeling in the literature: traditional data modeling and algorithmic modeling ([7]). The first approach is mainly concerned with the construction and verification of linear and non-linear regression models (logistic, Cox, Poisson, etc.), while the second approach is mainly used for machine learning methods (neural networks, decision trees, etc.). In the first approach, the model built on the whole sample is used, and after assessing the quality of the built model using a wide range of tools, it forms the basis for dependency analysis and prediction. In the second approach, model validation is performed on the basis of an independent data set (validation of the model built in the first stage according to the principle applied in traditional data modeling). In our case, it was decided to build the model using the concepts and tools of the data modeling culture, so no additional dataset was extracted for validation. The premise to remain within the framework of the "classic" tools was the multiplicity of objects in relation to the number of variables (attributes) describing the objects ( records ) and the possibility of a broader interpretation of the obtained results. Extracting the validation set would reduce the accuracy of the model parameters estimation.
Relationships between human factors and occurrence of forest fires
Anthropogenic factors that cause increases in fire probability are inextricably linked to human activities in forested areas ([24]). These factors include agricultural, recreational, and manufacturing activities and result in constant pressure on the forest ecosystem ([24]). Our study has shown that population density, distance from buildings, railways and roads are particularly important anthropogenic factors that determine the probability of fire. Anthropogenic ignition is related to both the motivation of people who set fires and regional differences in important socio-economic factors that cause fires, such as land use, population density, and presence of infrastructure ([12]).
A significant effect of population density on fire probability was also demonstrated by Milanović et al. ([30]). In a study conducted in Portugal, Catry et al. ([8]) pointed out that over 70% of fires occur in areas with a population density greater than 100 people per km2 and 85% occur within 500 m of buildings. In Portugal, 70% of all fires occurred in municipalities with more than 100 persons km-2, although they represent only 21% of the territory ([9]). The studies conducted so far in Poland have shown that the average number of inhabitants per 1000 ha of forest area is the only anthropogenic parameter that significantly distinguishes the occurrence of forest fires ([36]). According to the results of Alexandrian & Gouiran ([3]), some fires also started near individual buildings in large forest areas, far from major settlements and roads. Man-made fires are not always the result of arson ([15]). They also result from the lack of education and basic knowledge in forest protection, which means that forest fires can be caused, for example, by unattended fires, burning of waste, etc. Population density as a factor influencing fire occurrence is not surprising, since most fires are caused by human activities.
Our research has confirmed that as distance from roads increases, the probability of fire decreases. This is a consequence of the greater availability of forest land, which leads to more intensive use by society ([31], [11]). Gerdzheva ([16]) emphasizes that one of the reasons could be human behavior, especially the lack of awareness of the dangers to forest ecosystems, such as irresponsible discarding of burning matches and cigarette butts from vehicles. The growing accessibility of the forest (near roads) increase the probability of occurrence of a high-energy stimulus that can trigger a fire ([38]). This situation corresponds in particular to urban forests, where high forest penetration increases the number of fires.
A more detailed analysis of the impact of human activities on fire occurrence would be possible thanks to the use of real data on tourist/car traffic in forest areas. Taking into account current technologies and data (e.g., from mobile phones and social media - [43]) such analyzes could greatly improve the possibility of drawing conclusions in this regard.
Relationships between topography and probability of forest fires
The probability of fire occurrence is also influenced by factors related to landform, such as elevation, slope, aspect, and topographic position. These factors are partly responsible for shaping soil and climatic conditions in a given area and consequently affect vegetation (fuel). In our studies, fire probability decreases with increasing mean elevation above sea level and with increasing slope. This may be related to both forest availability and population distribution ([2], [24]). It seems reasonable to state that the lower the elevation, slope, and differentiation of topography, the greater the human activities that may primarily increase the probability of fire. This dependence may vary in individual areas, and the effects of terrain elevation or slope on fire occurrence should be estimated by considering the particular conditions in a given area. Numerous studies have demonstrated that aspect is an important factor in fire probability ([40]). It is related to the difference in solar radiation on slopes and thus to the lower humidity and higher temperatures on southern slopes, which can lead to the initiation of forest fires ([2]).
Relationships between stand factors and probability of forest fires
According to the previous study of Brach & Kaczmarowski ([6]), stands with dominant share of pine (> 50%) on coniferous sites (dry, fresh, mixed) and with leaf litter on the ground, was assumed to be the most combustible forest type in Poland. In this study, this specific forest type is used as a reference point in the analysis of vegetation contribution to the forest fire occurrence probability. Forest fire occurrence was positively associated with stands in which oaks have a dominant share and it was more likely then in reference site. Oak forests have a higher diversity ([39]) and therefore offering to the local community many services that attracting more people to the forest leading to the higher fire incidence ([44]). Contrary, forest fire occurrence was negatively associated with the majority of forest site types and it was much less likely than on the reference site. In accordance with our findings, study of González et al. ([18]) shows that mixed or broadleaved dominated stands are less affected by fire. The decrease in fire occurrence is more pronounced at montane forest sites similarly to González et al. ([18]) and Milanović et al. ([30]). Considering the vegetation contribution to the forest fire occurrence at the tree species level, the main tree species, oak, beech, and alder have a much lower probability of fire occurrence than the reference group, which can be attributed to the higher flammability of the pine forests ([41], [1]). In general, the probability of fire can be also influenced by the soil cover where the ignition is usually initiated ([38]). In this study, fire ignition was negatively associated with a heavily turfed cover.
Conclusions
Our analysis provided new information on fire occurrence in Polish forests. The presented method made it possible to reveal the factors influencing forest fire occurrence in Poland. Results of this study can be used by the bodies responsible for forest management in Poland, especially the State Forests, to take measures aimed at changing the approach to the classification of forest areas according to the category of fire risk, which is established every 10 years. Until now, the classification took into account an only one anthropogenic factor, namely the number of people per 0.01 km2 of forest area. The presented analysis has shown that there are many other factors that increase the probability of fire and that can be easily included in the classification. The results of this work can help to better understand and adapt future investments in forest fires prevention systems. More accurate models will allow to better place fire prevention monitoring, properly design tourism infrastructure, and last but not least, properly adjust species composition. Moreover, the results of this work can initiate further scientific research on the probability patterns of forest ignition in Poland (predictive model).
References
Gscholar
Gscholar
Gscholar
Gscholar
Gscholar
Gscholar
Gscholar
Gscholar
Gscholar
Gscholar
Gscholar
Gscholar
Gscholar
Gscholar
Gscholar
Gscholar
Gscholar
Gscholar
CrossRef | Gscholar
Gscholar
Authors’ Info
Authors’ Affiliation
Department of Geomatics, Forest Research Institute, Sekocin Stary ul. Braci Lesnej 3, 05090 Raszyn (Poland)
Prevent Fires Foundation, Warszawa, ul. Drawska 29A/56, 02-202 Warszawa (Poland)
Department of Econometrics and Statistics, Institute of Economy and Finances, Warsaw University of Life Sciences - SGGW (Poland)
Department of Applied Informatics, Institute of Information Technology, Warsaw University of Life Sciences - SGGW (Poland)
Department of Forest Management, Dendrometry and Forest Economics, Institute of Forest Sciences, Warsaw University of Life Sciences - SGGW (Poland)
General Directorate of the State Forests, ul. Grójecka 127, 02-124 Warszawa (Poland)
Ryszard Szczygiel 0000-0001-8008-7430
Forest Fire Protection Laboratory, Forest Research Institute, Sekocin Stary, ul. Braci Lesnej 3, 05-090 Raszyn (Poland)
Chair of Forest Protection, University of Belgrade Faculty of Forestry, 11030 Belgrade (Serbia)
Department of Forest Protection and Wildlife Management, Faculty of Forestry and Wood Technology, Mendel University, 61300 Brno (Czech Republic)
Corresponding author
Paper Info
Citation
Ciesielski M, Balazy R, Borkowski B, Szczesny W, Zasada M, Kaczmarowski J, Kwiatkowski M, Szczygiel R, Milanovic S (2022). Contribution of anthropogenic, vegetation, and topographic features to forest fire occurrence in Poland. iForest 15: 307-314. - doi: 10.3832/ifor4052-015
Academic Editor
Davide Ascoli
Paper history
Received: Dec 29, 2021
Accepted: Jun 19, 2022
First online: Aug 23, 2022
Publication Date: Aug 31, 2022
Publication Time: 2.17 months
Copyright Information
© SISEF - The Italian Society of Silviculture and Forest Ecology 2022
Open Access
This article is distributed under the terms of the Creative Commons Attribution-Non Commercial 4.0 International (https://creativecommons.org/licenses/by-nc/4.0/), which permits unrestricted use, distribution, and reproduction in any medium, provided you give appropriate credit to the original author(s) and the source, provide a link to the Creative Commons license, and indicate if changes were made.
Web Metrics
Breakdown by View Type
Article Usage
Total Article Views: 26295
(from publication date up to now)
Breakdown by View Type
HTML Page Views: 23068
Abstract Page Views: 1989
PDF Downloads: 980
Citation/Reference Downloads: 4
XML Downloads: 254
Web Metrics
Days since publication: 955
Overall contacts: 26295
Avg. contacts per week: 192.74
Article Citations
Article citations are based on data periodically collected from the Clarivate Web of Science web site
(last update: Mar 2025)
Total number of cites (since 2022): 10
Average cites per year: 2.50
Publication Metrics
by Dimensions ©
Articles citing this article
List of the papers citing this article based on CrossRef Cited-by.
Related Contents
iForest Similar Articles
Review Papers
Monitoring the effects of air pollution on forest condition in Europe: is crown defoliation an adequate indicator?
vol. 3, pp. 86-88 (online: 15 July 2010)
Research Articles
Near zero mortality in juvenile Pinus hartwegii Lindl. after a prescribed burn and comparison with mortality after a wildfire
vol. 12, pp. 397-402 (online: 31 July 2019)
Technical Reports
Air pollution regulations in Turkey and harmonization with the EU legislation
vol. 4, pp. 181-185 (online: 11 August 2011)
Research Articles
Bioaccumulation of long-term atmospheric heavy metal pollution within the Carpathian arch: monumental trees and their leaves memoir
vol. 17, pp. 370-377 (online: 27 November 2024)
Editorials
COST Action FP0903: “Research, monitoring and modelling in the study of climate change and air pollution impacts on forest ecosystems”
vol. 4, pp. 160-161 (online: 11 August 2011)
Research Articles
Modeling human-caused forest fire ignition for assessing forest fire danger in Austria
vol. 6, pp. 315-325 (online: 16 July 2013)
Research Articles
Dust collection potential and air pollution tolerance indices in some young plant species in arid regions of Iran
vol. 12, pp. 558-564 (online: 17 December 2019)
Commentaries & Perspectives
Clean air policy under the UNECE Convention on long-range transboundary air pollution: how are monitoring results “translated” to policy action
vol. 2, pp. 49-50 (online: 21 January 2009)
Research Articles
First results of a nation-wide systematic forest condition survey in Turkey
vol. 4, pp. 145-149 (online: 01 June 2011)
Editorials
Adaptation of forest ecosystems to air pollution and climate change: a global assessment on research priorities
vol. 4, pp. 44-48 (online: 05 April 2011)
iForest Database Search
Search By Author
- M Ciesielski
- R Balazy
- B Borkowski
- W Szczesny
- M Zasada
- J Kaczmarowski
- M Kwiatkowski
- R Szczygiel
- S Milanovic
Search By Keyword
Google Scholar Search
Citing Articles
Search By Author
- M Ciesielski
- R Balazy
- B Borkowski
- W Szczesny
- M Zasada
- J Kaczmarowski
- M Kwiatkowski
- R Szczygiel
- S Milanovic
Search By Keywords
PubMed Search
Search By Author
- M Ciesielski
- R Balazy
- B Borkowski
- W Szczesny
- M Zasada
- J Kaczmarowski
- M Kwiatkowski
- R Szczygiel
- S Milanovic
Search By Keyword