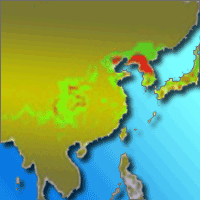
Estimating the distribution characters of Larix kaempferi in response to climate change
iForest - Biogeosciences and Forestry, Volume 13, Issue 6, Pages 499-506 (2020)
doi: https://doi.org/10.3832/ifor3570-013
Published: Nov 01, 2020 - Copyright © 2020 SISEF
Research Articles
Abstract
A better understanding of the distribution of suitable habitats of Larix kaempferi and its environmental constraints is crucial to know how global climate change will affect its growth and future dynamics. We simulated global suitable distribution areas of L. kaempferi under current and future climates, using different representative concentration pathway (RCP) scenarios, to evaluate the main factors affecting its geographical distribution. The results showed that under current climate conditions the suitable distribution areas of L. kaempferi are concentrated in Europe and Asia, followed by North America. The “Germany-Sweden-Britain” (19.42% of the total worldwide area) and “China-Japan-North Korea” (43.11%) regions are the cores for L. kaempferi distribution. The suitable distribution area for L. kaempferi is large in China (33.75% of the total area). The suitable distribution areas in Asia, Europe, and China decreased and shifted northward in the RCP scenarios. The main climatic factors affecting the distribution of L. kaempferi were the annual mean temperature, mean temperature of the coldest quarter, annual mean precipitation, and precipitation in the driest month. L. kaempferi could adapt or move to higher latitudes/altitudes to cope with climate change. Our results contribute to the introduction, cultivation, and management of L. kaempferi and potentially of other deciduous gymnosperms.
Keywords
Suitable Distribution Areas, Maxent Model, Environmental Variable, RCPs, Cultivation and Management
Introduction
Species survival and forest ecosystems are increasingly changing due to the rapid increase in global temperature ([39]). Change in temperature is one of the most important factors impacting on species distribution, along with invasive pests, disease, and land use change. There was a dozen of important tree species whose range has been altered by disease/pests or the sprawl of urbanization/agriculture ([7]). Changes in species distribution are becoming more and more common, in particular latitudinal and altitudinal changes in the northern regions ([22]).
Predicting changes in the potential distribution areas of species under global climate change has become a topic of interest in current research ([28], [7]), and is essential both to assess the impacts of climate change on forests and develop adaptive forest management strategies ([43], [44], [37]).
Previous studies have demonstrated that temperature and precipitation influence plant physiology ([23]), thereby affecting ecological processes ([28]) and ultimately leading to changes in the geographical distribution of plant species worldwide ([7], [19]). For example, the plant metabolic rate is directly influenced by increasing temperatures, resulting in major changes in their growth, development, reproduction and geographic distribution ([17]). Differences in precipitation regimes are reflected in the distribution of plant species along polar or altitudinal isopleths ([29]). In addition, the interaction between vegetation and climate must be taken into account to understand the adaptability and response of vegetation to climatic factors ([22]).
Determining the distribution of species and their potential establishment in climate-friendly habitats is basic for many ecosystem management activities ([43], [37]). Recently, species distribution models (SDMs) have been used to understand the impacts of global climate change on the potential distribution areas of various species ([29], [43]) as well as for biodiversity conservation purposes ([20], [17]). The commonly used SDMs include generalized linear models, classification regression trees, generalized additive models (GAMs - [9]), genetic algorithm-based rule combination prediction models (GARPs), niche models (Bioclim), and maximum entropy models (Maxent - [24]). These models are different and cannot be used for most tree species, as the location of study areas, research scales, environmental variables and spatial resolutions, different ecological characteristics of the species, and the sample size can affect the results. However, in the last deceade the Maxent model has been widely used in the study of the potential distribution areas of suitable habitats of species ([15]). This model is particularly suitable for predicting the geographical distribution of species with small sample sizes or partial sample data ([24]).
Larix kaempferi (Lamb.) Carr is an important woody species in fast-growing plantation in China, and it is also the main forest tree species of alpine coniferous forests in northeastern China, Inner Mongolia forest area, and north and southwest China ([3]). Larix kaempferi has a straight trunk, high growth rate, and good wood quality ([31], [22]). It is endemic to Japan, and native to the central mountainous region of the Honshu Island ([25]). The boundaries of L. kaempferi distribution are Mt. Zao in Miyagi Prefecture in the north and east, Mt. Hakusan in the west, and Mt. Tengu and Mt. Yamazumi of the Akashi Mountain Range in the south, and the eight representative provenances are Kawakami-Higashiyamanashi, Yatsugatake, Asama, Fuji, Minami-Alps (The South Japan Alps), Nikko, Kita-Alps (The North Japan Alps), and Kiso. L. kaempferi grows well in these areas, and artificial selection, hybridization, and development of new varieties are being conducted ([11]). L. kaempferi was introduced and cultivated in Europe, North America, and Asia, in the early 19th century ([31], [18], [25]).
The successful introduction and cultivation of plant species depends on the availability of environmental conditions suitable for its growth ([12]). In recent years, studies on L. kaempferi forests have been conducted on the physical and chemical properties of soil ([14]), the growth processes and structural features of stands in different areas ([42], [8]), the characteristics of the understory plant diversity ([16]), and the productivity and ecological adaptability ([5]). However, the effects of climate change on the distribution of L. kaempferi have not been documented. Therefore, it is necessary to explore the future potential distribution areas of L. kaempferi under different climate change scenarios.
The present study combined GIS with the Maxent model to assess the characteristics of suitable distribution areas for L. kaempferi under a changing climate. Our objectives were to: (i) simulate the suitable distribution area of L. kaempferi in the current and future climate scenarios with different representative concentration pathways (RCPs); and (ii) determine the main climatic factors affecting the distribution pattern of L. kaempferi with different altitude gradients. The results of this study are expected to provide significant theoretical background to guide the cultivation and effective management of L. kaempferi.
Materials and methods
Distribution data of L. kaempferi
L. kaempferi is a full-sun tree, with strong adaptability to climate, growing in regions of 35° 20′-38° 10′ N, 136° 45′-140° 30′ E, with altitude 1200-2500 m a.s.l. ([3]), where the annual average temperature is 2.5-12.0 °C and annual precipitation is 500-1400 mm ([3]). The growth rate is higher in sites with high air humidity and high precipitation ([12]). It has limited cold tolerance, and is sensitive to soil fertility and moisture ([31]). The current global distribution data of L. kaempferi was obtained from the Global Biodiversity Information Database (GBIF - ⇒ http://www.gbif.org/), the National Herbarium of America (⇒ http://www.nmnh.si.edu/botany), and the Chinese Herbarium (CVH - ⇒ http://www.cvh.ac.cn/) specimen information platforms.
Some 108 L. kaempferi plots (40 × 25 m, o.1 ha) were established in three typical cultivation areas for the species ([21], [4]): (i) the north subtropical sub-high mountain area (Changlinggang Forest Farm, Hubei); (ii) the warm temperate middle mountain area (Xiaolongshan Forest Farm, Gansu); (iii) the mid-temperate low mountain area (Liaoning Dagujia Forest Farm). Plots were grouped in 3 age classes: (I) young forests (<20 years); (ii) middle-aged forest (20-30 years); (iii) pre-mature forest (30-40 years). In each plot, the diameter at the breast height (DBH), tree height, first living branch height, crown width (four sides) were measured for all trees. Morever, coordinate information (latitude, longitude and altitude) of each tree was collected (Tab. 1).
Tab. 1 - Main statistics of site and environmental factors at the 108 plots analyzed.
Factor | Warm temperate zone |
Northern subtropic zone |
Mid-temperate zone |
|||
---|---|---|---|---|---|---|
Mean | SE | Mean | SE | Mean | SE | |
Age | 17.64 | 1.76 | 21.66 | 1.06 | 23.67 | 2.21 |
DBH | 11.52 | 1.15 | 18.98 | 0.94 | 16.62 | 1.07 |
Density | 1912 | 182 | 740 | 82 | 1154 | 105 |
Longitude | 106.01 | 0.04 | 109.89 | 0.03 | 124.92 | 0.03 |
Latitude | 34.24 | 0.02 | 30.77 | 0.01 | 42.47 | 0.01 |
Altitude | 1647 | 17 | 1747 | 8 | 380 | 10 |
A specific literature search was also carried out on current L. kaempferi worldwide distribution ([19]). We investigated the peer-reviewed literature on L. kaempferi under rapid climate changes, finding a total of 83 publications, including 181 scope-limited observations of the dynamic changes of larch. We obtained records from a total of 253 sites worldwide, including both natural and introduced L. kaempferi distributions (Fig. 1). To determine the potential scope adjustment factors beyond climate change, we also retrieved from each study the following information: start year, end year, total study area, number of tree species, and number of studies on L. kaempferi in each country.
Data analyses
To reflect the climate data of the current period, we obtained data regarding 19 climate variable from the WorldClim database (⇒ http://worldclim.org/), with a spatial resolution of 2.5′, over the period 1950-2000. Moreover, we generated climate data using the IDW (inverse distance weight) spatial interpolation method in GIS, including observation data from meteorological stations around the world ([10]). Climate data for the year 2070 was generated using the CCSM4 climate system model ([40]), with greenhouse gas emission scenarios for four typical concentration targets: RCP2.6, RCP4.5, RCP6.0, and RCP8.5. These scenarios represented an increase of 2.6, 4.5, 6.0, and 8.5 W m-2, respectively, of the radiative force in the year 2100. RCP2.6 is a warming mitigation scenario, RCP4.5 and RCP6.0 are stable scenarios, while RCP8.0 is a high greenhouse gas emission scenario, which correspond to an increase in the mean global temperature of 1.6, 2.4, 2.8, and 4.3 °C, respectively ([32], [40]). The environmental variable data was processed using the software ArcGIS® ver. 10.2 (ESRI, Redlands, CA, USA), and the layer data of each environment was obtained for model prediction. The mapping data was sourced from the DIVA-GIS website (⇒ http://www.diva-gis.org/) and the National Basic Geographic Information System (⇒ http://nfgis.nsdi.gov.cn/).
The software Maxent v. 3.3.3 (⇒ https://biodiversityinformatics.amnh.org/open_source/maxent/ - Phillips et al. ([27]) was used to identify the potential distribution areas of L. kaempferi. To this purpose, 70% of the available data was randomly selected for model training, 15% was used for model verification, and 15% was used for model fitting. Model accuracy was evaluated using the receiver operating characteristic (ROC) curve, which was plotted with specificity (1-specificity) and sensitivity (1-omission rate). The AUC value (the area enclosed by the ROC curve) does not depend on the threshold. The magnitude of the AUC value reflects the predictive ability of the model ([6]). The larger the AUC value, the better the accuracy of model predictions. When 0.7<AUC<0.8 was classified as useful predictive, 0.8<AUC<0.9 as good predictive, and AUC>0.9 as highly predictive ([33], [38]). The Jackknife method was used to test and evaluate the effects of various environmental factors on the potential distribution areas of L. kaempferi. The distribution threshold was calculated by randomly selecting 10% of the data in the training set. The output distribution value was a logistic, and the output was in ASCII format. The maximum number of iterations was set to 5.000, and the random operation was set to 15 times. The final average was used to evaluate the effect of the model. The calculated result showed that the distribution threshold of the model was 0.3092, i.e., the presence of L. kaempferi in areas showing values of 0.3092 or less is a small probability event. Therefore, these areas were regarded as unsuitable for L. kaempferi cultivation and discarded. A distribution threshold of 0.3092-0.5083 indicated a suitable distribution area (SA), while values in the range 0.5083-0.9624 indicated the most suitable distribution area (MSA). The final prediction results were then imported into ArcGIS® v. 10.2 software to prepare maps using the “Reclass” tool, and the surface area of the different suitable regions was calculated using the “Raster Reclassify” tool.
We employed the contribution rate of environmental variables, the displacement value, and the Jackknife method to assess the importance of environmental variables in delineating the geographic patterns of potential distribution areas for L. kaempferi ([16]). The contribution rate increased the gain value by gradually correcting the coefficient of a single essential factor, and the gain value was assigned to the environment variable that determined this essential factor. The replacement value was obtained by randomly replacing the value of the environmental variable in the training point set, and determining the reduction in the degree of the training AUC value. Jackknife created a new model by sequentially using or excluding a variable, and the difference in regularized training gain, test gain, and AUC value of the model was used for evaluating model performance.
The probability distribution obtained with the largest entropy is considered as the optimal distribution by Maxent model, using non-random relationships between environmental factors, such as climate, altitude, and vegetation types, at species distribution sites or research areas under constraints. This can predict the suitable distribution areas of a species, and construct a spatial distribution model at the geographic scale. Model prediction results, in combination with GIS, can be used to obtain an intuitive map of the suitable distribution areas for the species.
Results
Spatial differentiation of L. kaempferi under temporal climate conditions
The result of model fitting showed a high accuracy of the model predictions using Maxent (Fig. 2).
The result showed that the establishment of L. kaempferi is increasing (i.e., advance or infilling) in 56% of the research sites, in 14% is decreasing (i.e., recession or thinning), while no change was detected for 30% of the analyzed sites.
The suitable (SA) and most suitable (MSA) distribution areas for L. kaempferi occupied a global area of 334.18×104 km2 and 58.35×104 km2, respectively (Fig. 3). The potential distribution area in Europe was the largest, accounting for 52.00% (SA) and 40.67% (MSA) of the total area, respectively. This was followed by Asia, with the suitable and most suitable distribution areas covering 46.67% and 57.34% of the total area, respectively. However, Africa and South America did not show any potential distribution areas for L. kaempferi (Tab. 2).
Fig. 3 - The global potential distribution area for Larix kaempferi under current climate conditions.
Tab. 2 - The potential global suitable areas of Larix kaempferi. (SA): suitable area (distribution threshold: 0.3092-0.5083); (MSA): most suitable area (distribution threshold: 0.5083-0.9624). For more details, see text.
Region | SA | MSA | Total |
---|---|---|---|
(×104 km2) | |||
Europe | 173.80 | 23.73 | 197.53 |
Asia | 155.95 | 33.46 | 189.41 |
North America | 3.33 | 1.07 | 4.60 |
Oceania | 1.10 | 0.09 | 1.19 |
Global | 334.18 | 58.35 | 392.73 |
The potential distribution areas for L. kaempferi, including both suitable and most suitable distribution areas, were mainly concentrated in Europe and Asia. In Europe, these areas principally are present in Britain, Ireland, Italy, the Netherlands, France, Poland, Sweden, and Germany, centering in the “Germany-Sweden-Britain” region, each accounting for 9.07%, 5.23%, and 5.12% of the total areas, respectively. In Asia, the suitable and most suitable distribution areas were mainly located in Japan, South Korea, North Korea, and China, centering in “China-Japan-North Korea” region, accounting for 33.75%, 6.23% and 3.13% of the total areas, respectively. In other continents, no significant suitable distribution areas were found. However, some regions scattered across the United States (North America), Canada (North America), Australia (Oceania), and New Zealand (Oceania) were found to be somewhat suitable for L. kaempferi cultivation (Fig. 3).
China presented the main distribution areas for L. kaempferi in Asia. The suitable and most suitable distribution areas occupy 115.24×104 km2 and 17.29×104 km2, accounting for 34.48% and 29.63% of the total area, respectively. These areas were mainly located in Jilin, southeastern Liaoning, western Hubei, Hebei, central and eastern Sichuan, Chongqing, Shandong, southwestern Shaanxi, southeastern Gansu, Guizhou, and southeastern Heilongjiang. The most suitable distribution areas in Chinese region within “Dabashan-Wushan” were concentrated in Sichuan (15.24%), Shaanxi Province (12.91%) and Hubei (9.58%), accounting for 37.73% of the most suitable distribution areas in China. The most suitable distribution areas within “Liaodong Peninsula-Hebei” were located mainly in Liaoning (31.94%) and Hebei (11.92%), representing for 43.86% of the most suitable distribution areas of China (Fig. 4).
Fig. 4 - The potential distribution area for Larix kaempferi in China under current climate condition.
Spatial differentiation of L. kaempferi in different RCP scenarios
The distribution of L. kaempferi exhibited different degrees of variations under different RCPs scenarios (Fig. 5). Compared to the current climate condition, the suitable distribution areas (SA) for L. kaempferi increased by 2.81%, 4.81%, 3.80%, and 3.25% in the four RCPs scenarios, respectively. However, the most suitable distribution area (MSA) decreased by 14.85%, 15.10%, and 6.46% in RCP2.6, RCP4.5, and RCP6.0 scenarios, respectively, while it increased by 1.40% in RCP8.5 scenario.
Fig. 5 - Variation of the global potential distribution area predicted for Larix kaempferi under different RCPs.
The suitable and most suitable distribution areas of L. kaempferi in Africa and South America also have not found under the four RCPs scenarios (Tab. 3). The potential distribution area in North America increased under the four RCPs scenarios, mainly in the coastal areas of western United States and southwestern Canada. The potential distribution areas showed an increase, particularly in RCP8.5, indicating that the range of suitable distribution area for L. kaempferi in the United States and Canada will expand in case of the four RCPs scenarios (Fig. 5, Tab. 3).
Tab. 3 - The potential global suitable area of Larix kaempferi under different RCPs scenarios. (RCPs): representative concentration pathways; (SA): suitable area (distribution threshold: 0.3092-0.5083); (MSA): most suitable area (distribution threshold: 0.5083-0.9624). For more details, see text.
RCPs scenarios |
Regions (×104 km2) | |||||||||||
---|---|---|---|---|---|---|---|---|---|---|---|---|
Europe | Asia | North America | Oceania | South America | Africa | |||||||
SA | MSA | SA | MSA | SA | MSA | SA | MSA | SA | MSA | SA | MSA | |
RCP2.6 | 185.57 | 17.68 | 145.07 | 31.27 | 11.23 | 0.84 | 1.24 | 0.09 | 0.48 | 0 | 0 | 0 |
RCP4.5 | 181.95 | 14.54 | 147.96 | 31.68 | 19.12 | 3.46 | 0.98 | 0.09 | 0.82 | 0 | 0 | 0 |
RCP6.0 | 181.76 | 16.84 | 144.07 | 33.72 | 19.09 | 3.17 | 1.34 | 0.08 | 0.89 | 0 | 0 | 0 |
RCP8.5 | 176.12 | 14.31 | 138.19 | 35.72 | 27.7 | 7.97 | 1.32 | 0.1 | 1.83 | 0.01 | 0 | 0 |
Current | 173.8 | 23.73 | 155.95 | 33.46 | 3.03 | 0 | 1.1 | 0.09 | 0.07 | 0 | 0 | 0 |
The potential distribution area of L. kaempferi in Asia showed a decreasing trend under the four RCPs scenarios (Tab. 3). The suitable distribution area decreased mainly in China, North Korea, and Japan, however, the most suitable distribution area increased in Russia, suggesting that the suitable distribution area of L. kaempferi in Asia would shift northward under the four RCPs scenarios (Fig. 5, Tab. 3).
Suitable distribution areas of L. kaempferi in the European region exhibited varying trends under the four RCP scenarios (Tab. 3). The potential distribution areas in Finland and Sweden, in northern Europe, exhibited a gradual increase, indicating that the suitable distribution area of L. kaempferi has a tendency to move northward in Europe (Fig. 5, Tab. 3).
The potential distribution area of L. kaempferi did not exhibit marked changes in Oceania under the four RCPs scenarios (Tab. 3). The distribution area increased slightly, except in the RCP8.5 scenario, and the most suitable distribution area also showed a slight increase (Fig. 5, Tab. 3).
The suitable distribution area of L. kaempferi in China decreased by 8.49%, 4.39%, 7.81%, and 8.80% in RCP2.6, RCP4.5, RCP6.0, and RCP8.5 scenarios, respectively (Fig. 6). In addition, the most suitable distribution area further decreased by 9.16%, 17.52%, 2.95% and 1.34% in the four RPCs, respectively.
Fig. 6 - The potential distribution area for Larix kaempferi in China under different climate RCP scenarios.
Our results indicated that the suitable and most suitable areas of L. kaempferi will reduce and shift northeast at varying degrees in China (Fig. 6). Nearly half of the most suitable distribution areas would disappear due to climate change. For example, only 0.77×104 km2 persisted in the RCP8.5 scenario in Hubei province (Fig. 6). Conversely, the most suitable distribution area in Liaoning Province would increase to 6.25×104 km2 (Fig. 6), indicating that Liaoning province is the most suitable area for L. kaempferi under the different RCPs.
Response to environmental variables
The annual average temperature (Bio-1) was the main climatic factor affecting the distribution pattern of L. kaempferi, with a contribution rate of 28.5% (Tab. S1 in Supplementary material). This was followed by precipitation of the driest month (Bio-14), the monthly mean temperature difference between day and night (Bio-2), and the coldest quarterly mean temperature (Bio-11). The cumulative contribution rate of these six variables was 83.1%, indicating that these six variables mostly contribute to the variations in distribution pattern. The environment variable with the highest permutation value was the coldest quarter average temperature (Bio-11), with a value of 56.90%, followed by the average annual precipitation (Bio-12), indicating that these two variables were particularly important in the delineation of suitable distribution areas of L. kaempferi.
The results of the Jackknife test showed that when considering individually, the three variables with the highest regularization training gain and test gain were the coldest seasonal mean temperature (Bio-11), the annual mean temperature (Bio-1), and the driest seasonal mean temperature (Bio-9 - see Fig. S1 in Supplementary material). The three variables with the highest AUC values were the annual average temperature (Bio-1), the coldest seasonal average temperature (Bio-11), and the coldest monthly minimum temperature (Bio-6). This suggests that these variables reflect significant information, as the regularization training gain, test gain and AUC value exhibit little change. The three variables with the most reduction were the seasonal variation coefficient of precipitation (Bio-15), isotherm (Bio-3), and the hottest month maximum temperature (Bio-5), further suggesting that these variables contain more information than other variables.
The main factors affecting the geographical distribution of L. kaempferi were temperature (annual average temperature, coldest seasonal average temperature) and precipitation (annual average precipitation, driest month precipitation). We found a significant difference in the trends of centroid changes for precipitation between the early and late growth periods for this species. Receding L. kaempferi occurred near the centre of the envelopes, where conditions were becoming warmer and wetter. For the North American continent, the number of studies was limited, but suggested that L. kaempferi was tracking wetter conditions, and that changes in precipitation and temperature trends were variable among these sites.
Discussion
Since the 1980s, the overall distribution range of L. keampferi has changed as a consequence of climate warming, though with a varying degree in Europe and Asia. This may be attributed to the difference in climatic conditions ([30]). Asia has a temperate monsoon climate, with typical temperate continental climate characteristics ([2]). The average temperature of the coldest month in the north is below 0 °C, and in the south is close to 20 °C ([2]). The climate was characterized by cold and dry winters, and high temperatures and rain in summer ([2]). This was consistent with the biological characteristics of L. kaempferi, which is cold-tolerant and prefers slightly cold climate ([3]). In addition, variations in species range are becoming more common in the north with increasing latitude and altitude ([36]). Climate change, human disturbances, competitive releases, and ecological characteristics of individual species may contribute to new and unexpected overlaps in species ranges, and to impediments in predicting range-bound changes in surrounding trees ([7]).
L. kaempferi may be particularly sensitive to climate change ([26]). A large-scale study of climatic responses of L. kaempferi found that the global distribution is sensitive to the several environmental conditions, such as altitude, latitude, temperature, and moisture ([22], [1]), leading to regional differences in the geographic distribution of the species ([19]). In the context of global warming, forest tree species have been found to expand to higher altitudes ([34], [19]). In the present study, the distribution of L. kaempferi in North America, Canada, Oceania Australia and New Zealand, was found to be relatively small. In the past 30 years, the average annual temperature changes in low-altitude areas have either increased or decreased by about 3 °C. This suggests that temperature is a dominant factor limiting the distribution and growth of L. kaempferi, compared to other environmental variables. Geographical differences in the distribution-temperature response indicate that decreasing temperatures in high-altitude forests limits the distribution of L. kaempferi. The potential distribution areas of L. kaempferi in China were located mainly in Jilin, southeastern Liaoning, western Hubei and Hebei provences. This is similar to the potential distribution area obtained in previous studies ([18], [25], [35]).
Direct and/or indirect effects of drought may lead to significant changes in local microclimatic conditions ([22], [13]). Due to temperature-induced drought stress, decline of tree lines and thinning of forests are becoming more frequent, thus leading to a reduction in the distribution of L. kaempferi in the south, as well as to its increase in the north ([23]). Larch can tolerate harsh conditions in permafrost environments, regulate soil moisture intake under humid conditions, and benefit from the water released by the melting of ice-rich permafrost under drought conditions ([19]). Therefore, the impact of environmental changes on L. kaempferi forests will ultimately depend on the balance between temperature and precipitation. Based on the native L. kaempferi distribution trends and current distribution-climate response patterns, we determined the distribution gradient of L. kaempferi forests along the altitudinal gradient, i.e., the distribution areas increased at high altitudes and declined at low altitudes.
Our results predict that the suitable distribution areas of L. kaempferi will respond differently to climate change in Europe, Asia and North America under the four RCP scenarios ([35], [23]). Of these, the potential distribution areas would increase in North America, those in Asia would decrease and shift northward, while those in Europe would fragment and shift northward, compared to the present conditions. Africa and South America did not show any potential distribution areas for L. kaempferi in the current (Tab. 2) and future climate scenarios (Tab. 3). The reason is that Larix kaempferi is limited mainly by temperature, with a survival temperature range of 2.5-12 °C ([18]), while temperatures in Africa and South America are too high for the species. Although changes in temperature are expected under future climate scenarios, still the anticipated climate in the above continents will not meet the survival conditions of Larix kaempferi ([4]).
Our result showed that the main factors affecting the suitable distribution area of L. kaempferi were the annual average temperature and average annual precipitation. Extreme climates (coldest average temperature, driest monthly precipitation) were also the dominant factor affecting the distribution of L. kaempferi. Thus, extreme climate may lead to local discontinuity in species distribution ([41]).
Conclusion
In this study, we modeled the changes in the suitable distribution areas of L. kaempferi based on climatic factors. Most populations appear to be expanding their distribution northward. Our results can help planning the introduction and cultivation of L. kaempferi, thus avoiding economic losses caused by blind introduction, and increasing the yield of L. kaempferi. However, the predictions of this study were based only on the environmental conditions of the distribution area, being the genetic variation of the species and anthropogenic effects not considered here. Uncertainty about how these factors will change over time exist, so further studies on the patterns of L. kaempferi expansion and the underlying processes are required. Future research will include the improvement of prediction model as well as related research aimed to further characterize the future distribution of L. kaempferi, e.g., by carrying out cultivation tests in suitable distribution areas, thereby confirming the area suitable for introduction and cultivation of L. kaempferi.
The spatial dynamics of L. kaempferi over time indicate that the global warming will contribute to the species’ expansion, as it can adapt or shift to higher latitudes/altitudes. These results can aid in prioritizing geographic regions and/or larch species for further studies on their altitudinal/geographical response to climate change, in order to better understand how these deciduous gymnosperms adapt to global climate change.
Acknowledgment
We would like to thank all the researchers who made their data available for this study. This research was funded by the National Key Research and Development Program of China (2017YFD0600401).
References
Gscholar
Gscholar
Gscholar
Gscholar
Gscholar
Gscholar
Gscholar
Gscholar
Gscholar
CrossRef | Gscholar
Authors’ Info
Authors’ Affiliation
Jiapeng Shen
Dongsheng Chen 0000-0002-2135-8381
Xiaomei Sun
Shougong Zhang 0000-0001-9832-2558
State Key Laboratory of Tree Genetics and Breeding, Key Laboratory of Tree Breeding and Cultivation of the State Forestry Administration, Chinese Academy of Forestry, Beijing 100091 (China)
Jiapeng Shen
Dongsheng Chen 0000-0002-2135-8381
Xiaomei Sun
Shougong Zhang 0000-0001-9832-2558
Research Institute of Forestry, Chinese Academy of Forestry, Beijing 100091 (China)
Hubei Academy of Forestry, Wuhan, Hubei 430075 (China)
Corresponding author
Paper Info
Citation
Wu C, Shen J, Chen D, Du C, Sun X, Zhang S (2020). Estimating the distribution characters of Larix kaempferi in response to climate change. iForest 13: 499-506. - doi: 10.3832/ifor3570-013
Academic Editor
Maurizio Marchi
Paper history
Received: Jul 05, 2020
Accepted: Aug 18, 2020
First online: Nov 01, 2020
Publication Date: Dec 31, 2020
Publication Time: 2.50 months
Copyright Information
© SISEF - The Italian Society of Silviculture and Forest Ecology 2020
Open Access
This article is distributed under the terms of the Creative Commons Attribution-Non Commercial 4.0 International (https://creativecommons.org/licenses/by-nc/4.0/), which permits unrestricted use, distribution, and reproduction in any medium, provided you give appropriate credit to the original author(s) and the source, provide a link to the Creative Commons license, and indicate if changes were made.
Web Metrics
Breakdown by View Type
Article Usage
Total Article Views: 29622
(from publication date up to now)
Breakdown by View Type
HTML Page Views: 26024
Abstract Page Views: 1341
PDF Downloads: 1865
Citation/Reference Downloads: 6
XML Downloads: 386
Web Metrics
Days since publication: 1364
Overall contacts: 29622
Avg. contacts per week: 152.02
Article Citations
Article citations are based on data periodically collected from the Clarivate Web of Science web site
(last update: Feb 2023)
Total number of cites (since 2020): 1
Average cites per year: 0.25
Publication Metrics
by Dimensions ©
Articles citing this article
List of the papers citing this article based on CrossRef Cited-by.
Related Contents
iForest Similar Articles
Research Articles
Local ecological niche modelling to provide suitability maps for 27 forest tree species in edge conditions
vol. 13, pp. 230-237 (online: 19 June 2020)
Research Articles
Predictive capacity of nine algorithms and an ensemble model to determine the geographic distribution of tree species
vol. 15, pp. 363-371 (online: 20 September 2022)
Research Articles
Distribution factors of the epiphytic lichen Lobaria pulmonaria (L.) Hoffm. at local and regional spatial scales in the Caucasus: combining species distribution modelling and ecological niche theory
vol. 17, pp. 120-131 (online: 30 April 2024)
Research Articles
Predicting impacts of climate change on forest tree species of Bangladesh: evidence from threatened Dysoxylum binectariferum (Roxb.) Hook.f. ex Bedd. (Meliaceae)
vol. 10, pp. 154-160 (online: 25 May 2016)
Research Articles
Spatial modeling of the ecological niche of Pinus greggii Engelm. (Pinaceae): a species conservation proposal in Mexico under climatic change scenarios
vol. 13, pp. 426-434 (online: 16 September 2020)
Research Articles
Universal reaction norms for the sustainable cultivation of hybrid poplar clones under climate change in Italy
vol. 15, pp. 47-55 (online: 08 February 2022)
Research Articles
Predicting the impacts of climate change on the distribution of Juniperus excelsa M. Bieb. in the central and eastern Alborz Mountains, Iran
vol. 11, pp. 643-650 (online: 04 October 2018)
Research Articles
Modelling diameter distribution of Tetraclinis articulata in Tunisia using normal and Weibull distributions with parameters depending on stand variables
vol. 9, pp. 702-709 (online: 17 May 2016)
Research Articles
Distribution and habitat suitability of two rare saproxylic beetles in Croatia - a piece of puzzle missing for South-Eastern Europe
vol. 11, pp. 765-774 (online: 28 November 2018)
Research Articles
Environmental niche and distribution of six deciduous tree species in the Spanish Atlantic region
vol. 8, pp. 214-221 (online: 28 August 2014)
iForest Database Search
Search By Author
Search By Keyword
Google Scholar Search
Citing Articles
Search By Author
Search By Keywords
PubMed Search
Search By Author
Search By Keyword