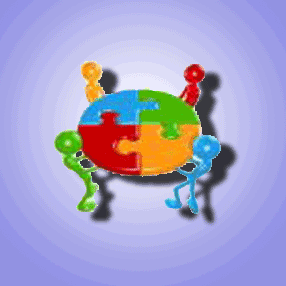
Usefulness and perceived usefulness of Decision Support Systems (DSSs) in participatory forest planning: the final users’ point of view
iForest - Biogeosciences and Forestry, Volume 9, Issue 3, Pages 422-429 (2016)
doi: https://doi.org/10.3832/ifor1356-008
Published: Jan 02, 2016 - Copyright © 2016 SISEF
Research Articles
Abstract
In recent decades, the focus of forestry Decision Support Systems (DSSs) has expanded to consider the social dimension of forestry and to support participatory decision-making. A large number of models and tools have become available to solve forest management planning problems. The Usefulness of a DSS depends on the range of tools that it incorporates, and many researches have been developed to evaluate DSSs using Usefulness as parameter. The assessment of Usefulness concerns the effectiveness of a DSS. Furthermore, most assessments take into account the degree of Perceived Usefulness, which is considered an indicator of the impact a system has on job performance. The present study focuses on the analysis of final users’ point of view on the Usefulness and Perceived Usefulness of DSSs in participatory forest planning. The research investigates how forest users’ characteristics and context influence their views on the potentialities of DSSs to enhance both the various phases of the participatory planning process (Usefulness) and job performance (Perceived Usefulness). The study is based on quantitative data collected through two questionnaires e-mailed to a sample of 150 DSSs end users. The questionnaires focused on Usefulness and on Perceived Usefulness topics, respectively. Results indicate that special attention must be given to motivating respondents with a clear explanation of the survey objectives when e-mailing questionnaires. Moreover, results show that, in general, respondents consider DSSs useful at each step of the participatory process, despite differences emerge among steps. The research also shows that respondents’ Perceived Usefulness of DSSs was higher before actually engaging with DSSs. Furthermore, the results highlight differences in Perceived Usefulness to improve job performance, suggesting that the use of DSSs may actually improve job performance more than expected. Specifically, results indicate that improving the technical descriptions of methodologies incorporated in a DSS may contribute to increasing the Perceived Usefulness. The information provided within this research contributes to the advancement of knowledge regarding the Usefulness of DSSs as perceived by forest stakeholders, which in turn supports the improvement of DSS architectures and the development of participatory processes in forest management planning.
Keywords
Forest Management, Decision Support Systems, Participatory Planning, Usefulness, Perceived Usefulness
Introduction
Decision support systems (DSSs) are computer systems designed to address complex decision-making processes ([39], [47], [62], [30]). Specifically, DSSs are a subset of computer-based Information Systems (IS), and may support the recording, processing and dissemination of information. Typically, the users are groups of individuals who act together in a field with a common purpose ([19]). The forestry literature reports several approaches to characterize DSSs used to address forest management planning problems ([58], [46], [45], [8], [23], [51], [52], [53], [63], [44]). According to Borges et al. ([9]), a forestry management planning DSS consists of a computerized tool with a graphic-based user interface that includes a data management module and a solution module - for example, a module that provides guidance and support so as to define the timing and location of forest management options. In recent decades, the focus of forestry DSSs has expanded to consider the new resource base and a broad range of forest functions and benefits. The social dimension of forestry has become more prominent, representing a key element of Sustainable Forest Management (SFM). The importance of involving stakeholders in the decision-making process - in particular in the definition of forest management strategies - has been underlined by several authors ([26], [57], [42], [15], [40], [11], [10]). As a consequence, participatory approaches to improve the planning processes using the views and experiences of stakeholders and experiential knowledge are often a highly valued component of forestry DSSs ([41]). Nevertheless, the decision-making processes may engage with scientific complexity and political and social uncertainty, becoming gradually more complicated ([32], [35], [49]).
In order to address these substantial changes in the decision-making context, a large number of models and tools have become available to support individual decision-making and to help address current and emergent forest management planning problems. DSSs in the forest sector typically include models and methodologies that simulate various forest variables to support the forecast of the outcomes of current decisions. Some DSSs also include the potentiality of inserting information about the preferences of decision-makers and participants ([44]). The possibility of developing DSSs incorporating techniques to help further decision-makers build their preferences, e.g., by providing them with information about trade-offs between criteria ([9]) is promising, as it may contribute to the effectiveness of participatory approaches. The Usefulness of a DSS thus depends on the range of tools that it incorporates.
Usefulness is defined as the total value a user perceives from using a new technology ([55]) and is a critical success factor for the effectiveness of a DSS application. As reported by Díez & McIntosh ([19]) the evaluation of an IS and the assessment of its usefulness are difficult tasks for researchers and technicians, as the basis and outcomes are strictly related to the context in which it is used. Typically, these tasks encompass both technical and implementation assessments. The former corresponds to testing the technical aspects of the IS, while the second focuses on the overall Usefulness of the system ([56]), that is, whether the implementation of the system satisfies users’ needs and expectations ([5]). Many theories and practical experiments have been established to evaluate IS through a post-implementation process using Usefulness as parameter. Examples include the Technology Acceptance Model (TAM - [14]) and its variants ([38]), the Theory of Planned Behaviour (TPB - [24]), the analysis of acceptability of a computer system ([48]), and the analysis of model quality ([54]).
The assessment of Usefulness concerns the effectiveness of a DSS and focuses both on its functionality and usability. In practice, the assessment of Usefulness generally includes meetings devoted to monitoring the participants’ interaction with the DSS. Most assessments also consider the degree of Perceived Usefulness (PU - [3]) and/or the Perceived Ease of Use (PEU). PU is an indicator of the impact a system has on work effectiveness and is defined as the degree to which a person believes that using a particular system may enhance his/her job performance ([14]). A DSS PU thus increases with the perception of a positive relationship between using the DSS and job performance. The PU is a key indicator of the TAM, widely used in the evaluation of an IS - e.g., King & He ([33]) reported references to TAM in 140 papers. The PU indicator is further considered by the Theory of Planned Behaviour (TPB - [60]) and other DSS Usefulness assessment models ([34]). DSS assessment studies suggest that PU and PEU are the two main critical success factors for the adoption and use of information technology ([14]). Before deciding to use a system, the user must perceive the system as being easy to use (Perceived Ease of Use) and as being useful, i.e., contributing to making his/her work easier (Perceived Usefulness - [65]).
PU has been used to assess DSSs in many fields. Examples for specific subjects include computer science ([1]); acceptance of a website ([31], [66]); Executive Information Systems ([4]); Enterprise Resource Planning systems ([2]); 3G mobile services ([59]); landscape and river basin management ([67]); agriculture and farming systems research ([47]); river rehabilitation ([29]); and information systems and managers’ performance ([20]). The case of forestry has been considered when assessing forest farmers’ intention of engaging in online trading ([37]), forest education tools ([36]) and DSSs for forest management ([21]). The final users’ PU and the way they see the Usefulness of forest DSSs are affected by several elements, such as the local socio-cultural and territorial context, the availability and culture of using DSSs, the final users’ background, and their profession ([2], [30], [16]). Italian and Finnish researchers’ and practitioners’ perception of DSS Usefulness in participatory forest planning has been analyzed by De Meo et al. ([16]). The present study has a dual purpose. Firstly, it will extend the research of De Meo et al. ([16]) to a wider geographical area. Secondly, it will focus not only on analyzing final users’ point of view on the Usefulness of DSSs in participatory forest planning, but also on the Perceived Usefulness. The emphasis is on investigating how forest users’ characteristics and context (e.g., country, age and background) influence their views on the potentialities of DSSs to enhance both the various phases of the participatory planning process (the Usefulness) and job performance (the Perceived Usefulness).
Materials and methods
Sampling and survey
The study is based on quantitative questionnaire data collected from February through May 2013. Two questionnaires were submitted by e-mail to a sample of 150 DSS end users. The first focused on Usefulness issues (U questionnaire), while the second addressed Perceived Usefulness topics (PU questionnaire). The sample was selected using an iterative process based on the principles of snowball sampling ([25], [27]): during the first iteration, DSS end users involved in the COST Action FP0804 (FORSYS) were selected, while during the second iteration, further DSS end users were identified.
The sample of 150 DSS end users was composed of 31 National Coordinators involved in the FORSYS COST Action, 56 Portuguese stakeholders (from Research Institutes, Agencies, Companies) involved in forest management and 63 DSS users contacted in the second stage of sample selection. The sample included: (i) professors and researchers at universities or research institutes; (ii) representatives of national or regional forestry centers; (iii) professionals in private associations; and (iv) representatives of state enterprises. They were divided into two main categories: professionals and researchers.
It is not possible to know the origins of DSS users contacted by peers in the second iteration, but from the evidence in the results it is supposed that most of them are from Europe. The geographic distribution of the 87 DSS users involved in the first iteration was: 79 from other European countries, 2 from Africa, 2 from North America and South America, and 1 from Asia and Oceania. In terms of gender, 81% of the sample was represented by males and 19% by females.
Respondents were required to return answers to the questionnaires by e-mail within six weeks. Practices reviewed by Dennis ([17]) were taken into account to avoid some problems related to auto-compiled questionnaire techniques, such as a large number of missing responses, delays in collection, and sample distortion due to auto-selection. In particular, follow-up reminder e-mails were sent after two weeks, and in some cases respondents were also contacted by phone about a week after the questionnaires had been sent.
The response rates to the U and PU questionnaires were 14% and 17%, respectively. Some authors ([66], [6], [43]) classify these response rates as low. However, for questionnaires sent by e-mail, the absolute number of responses is reported to be more significant than the rate ([18]). Hochman & Carberry ([28]) reported as significant findings from a survey with 23 responses. In the case of this research, a total of 46 responses were received: 25 and 21 responses to the PU and U questionnaires, respectively.
Questionnaires
The two questionnaires comprised seven (the U questionnaire) and six (the PU questionnaire) close-ended questions, chosen with the aim of keeping the structure simple. In some cases, the respondents had to choose from a list of predefined responses, while other questions were formulated to offer an n-options ranking scale for selection by respondents. The full content of both the U and PU questionnaires are reported in Appendix 1 and Appendix 2, respectively.
The U questionnaire comprises a general section focusing on the characteristics of the management planning problems faced by the respondents (Appendix 1). Specifically, respondents were asked to define the temporal and spatial scales of their forest management planning problems. A third question focuses on the users’ experiences using DSSs.
In the questionnaire focusing on U, two additional questions from De Meo et al. ([16]) were included. These questions investigate the potential usefulness of DSS in improving each stage of the participatory planning process. They divided this process into 6 steps and 13 phases (Appendix 1). Respondents were asked to indicate the usefulness of the DSS for each step and phase, indicating a value from “highly useful” to “not useful at all”. The optional response “I do not know” was also provided. The last part of the questionnaire deals with the socio-professional situation of the respondents, namely, their year and place of birth, their professional position and their role in participatory planning.
The PU questionnaire also comprises a general section concerning the management planning problems faced by the DSS end users and their experience using DSSs (Appendix 2). In addition, the PU questionnaire includes two questions to gauge the degree of Usefulness as perceived currently by the respondent (question 5) and perceived earlier before the actual engagement of the respondent with DSS (question 6). Respondents were also provided with the option “Unable to form an opinion”. Users were asked to respond to questions 5 and 6 by providing a score on a five-point Likert’s scale, according to their agreement or disagreement with six statements adapted from Davis ([14]) to measure user acceptance of computer tools. A low score indicates negative Perceived Usefulness, while a high score indicates positive perceptions.
Data Analysis
Voluntary responses received by e-mail were collected and stored in a spreadsheet. In order to analyze the agreement to the statements concerning the PU of DSSs (questions 5 and 6 reported in Appendix 1), respondents were divided into four groups (Tab. 1). This grouping took into account the users’ experience with DSS and the statement reference period (current period or period before engaging with the DSS). Group A included all respondents and referred to the current PU (all respondents answering question 5). Group B comprised respondents experienced with DSS, reporting their PU before engaging with DSS (question 6). In addition, among respondents to question 5, we separated respondents who had already engaged with DSS (group C) from those who had not (group D) to get their current PU.
Tab. 1 - Characteristics of respondents classified into homogeneous groups (see text for details).
Groups | Experience with DSSs | Reference period |
---|---|---|
A | yes + no | current |
B | yes | before using the DSS |
C | yes | current |
D | no | current |
To avoid random errors, the reliability of each group’s responses was analyzed by applying Cronbach’s alpha coefficient (α). This statistic was introduced by Cronbach ([13]) and is widely used in psychometric studies to test the internal consistency of multiple-item scales. Internal consistency describes the extent to which all the items in a test measure the same concept. The technique is based on the fact that the variance of the sum of a group of independent variables is the sum of their variances ([7]). Therefore, the variance of the sum will increase if the variables are positively correlated. Values of the coefficient range from 0 to 1, with higher values indicating greater reliability. When items in the scale are identical (thus perfectly correlated) then α = 1, while when items are independent, then α = 0. It is commonly accepted that a value of 0.8 provides a reasonable rate of reliability ([22], [61]). Differences among groups were analyzed with the aim of detecting whether experience with DSSs influenced PU. In particular, PU was analyzed by comparing data from the following pairs of groups: B-C (comparison of current PU and PU before engaging with DSSs from users with experience with DSS); C-D (comparison between current PU of users experienced with DSSs and users that have not yet engaged with DSSs); B-D (PU of users before engaging with DSSs). In order to ascertain whether experience with DSSs influences PU and to analyze pairwise differences among groups of respondents, a comparison by means, standard deviations and medians was carried out. In fact, since normal distribution in the various groups could not be guaranteed, a more in-depth statistical analysis was conducted using a non-parametric test. Data concerning PU, grouped by experience with DSSs and by statement reference period, were compared by means of the Wilcoxon’s test at p < 0.05 for significance. The test was carried out using the “wilcox.test” function in R statistical software system.
Results and discussion
Profile of the respondents
The profile characteristics of the respondents are shown in Tab. 2. Respondents were mainly from European countries, and in particular from Italy, Spain, Germany and Portugal (28, 16, 12 and 12%, respectively). Such distribution by nationality reflected the fact that most of the contacted DSS users were from European countries; furthermore, the Authors’ nationality is Italian and Portuguese and this may partly explain why the majority of responses are from Italy and Portugal.
Tab. 2 - Main characteristics of the respondents. (n/a): data not available.
Country | Age class |
Temporal scale |
Spatial scale |
Role in participatory planning |
Years of experience |
---|---|---|---|---|---|
Germany | 20-30 | long | stand | researcher | 6-10 |
31-40 | long | regional | facilitator-researcher | > 10 | |
41-50 | all | all | researcher | 6-10 | |
Italy | 41-50 | long | forest | researcher | n/a |
31-40 | long | stand | expert | n/a | |
41-50 | medium | forest | researcher | 1-5 | |
31-40 | medium | stand-forest | researcher | n/a | |
51-60 | medium-long | forest-regional | expert | n/a | |
41-50 | medium-long | stand-forest | expert | n/a | |
31-40 | long | forest | expert | > 10 | |
Lebanon | 31-40 | medium | regional | facilitator | n/a |
Netherlands | 41-50 | medium-long | forest-regional | expert-developer-facilitator | n/a |
Portugal | 31-40 | long | stand | facilitator | n/a |
41-50 | long | stand | facilitator | n/a | |
41-50 | long | forest | stakeholder | n/a | |
Slovenia | 41-50 | medium-long | forest | expert | > 10 |
31-40 | medium-long | all | expert | 1-5 | |
Spain | 31-40 | long | stand | forest manager | 1-5 |
20-30 | all | forest | facilitator | n/a | |
51-60 | medium | forest | stakeholder | n/a | |
31-40 | short | forest | expert | n/a | |
Sweden | 31-40 | long | forest | facilitator-expert | 1-5 |
Switzerland | 51-60 | medium-long | forest-regional | expert | n/a |
Ukraine | 41-50 | long | forest | researcher | n/a |
USA | 51-60 | all | all | facilitator-expert | > 10 |
Concerning their role in the participatory planning process, the respondents are mainly experts (32%), researchers (16%) or facilitators (16%). However, in some cases it was difficult to identify their main role because some respondents (36%) declared that they had mixed expertise (e.g., expert-facilitator, facilitator-researcher). Respondents between the ages of 31 and 50 years represented 76% of the whole sample. A total of 13 respondents reported experience with DSSs, while 12 reported no engagement with DSSs. There were approximately an equal number of the former in each of the three levels of experience (1-5, 6-10, >10 years).
Concerning the temporal scale of forest management, most respondents (44%) were involved in long-term planning (more than 10 years). Only 4% declared that they were involved in short-term planning. Concerning the spatial scale, 40% of the respondents declared that they were involved in forest-level planning, where the focus is the joint management of a set of stands in a forested landscape. Some respondents indicated experience with DSSs in more than one combination of temporal and spatial scales.
Usefulness
The U questionnaire was completed by 21 respondents, a response rate lower than that of the PU questionnaire. Respondents having experience with DSSs in participatory planning indicated the use of one to four DSSs. Seven interviewees declared that they had used only one DSS.
Respondents with little or no experience with DSSs in participatory planning were asked to specify the reason why they did not use DSSs (Tab. 3). Results seem to indicate that the options listed could not help to explain the motivation for not using DSSs. In fact, the option “Other: I do not know DDSs” was reported by seven respondents as the main reason.
Tab. 3 - Distribution of respondents expressing why they did not use DSSs.
Statement | Number of respondents |
---|---|
DSS available is too costly to use | 1 |
DSS available is too difficult to use | 1 |
DSS available does not include properties needed | 2 |
I cannot see the benefit DSS would provide for the planning task | 1 |
DSS is not needed for participatory planning I’m involved in | 1 |
Other, what?: I do not know DDSs | 7 |
The answers to the questionnaires provided information used to assess the usefulness of DSS at each step and each phase of the participatory processes (Tab. 4). In general terms, the respondents consider DSSs somewhat useful at every step. The items analyzed appear reliable for analysis purposes: in fact, the overall Cronbach’s alpha reliability coefficient achieved was 0.97 at a level of p = 0.05, indicating that the test used is free from random error and that the items have a high internal consistency.
Tab. 4 - Usefulness at each step and phase of the Participatory Planning Process.
Steps | Usefulness | Phases | Usefulness |
---|---|---|---|
Organization | 3.34 | a - Organizing the process (time frame, budget definition) | 2.92 |
b - Problem structuring (focussing on the problem situation, formulating a joint problem, raising awareness, assessing current status) | 3.77 | ||
Intelligence | 3.01 | c - Identifying stakeholders | 2.33 |
d - Defining criteria, goals and constraints | 2.71 | ||
e - Eliciting stakeholders’ preferences | 2.92 | ||
f - Eliciting decision makers’ preferences | 2.83 | ||
g - Gathering/identifying information/expert knowledge regarding the decision/problem | 3.38 | ||
h - Creating new information and knowledge | 3.71 | ||
i - Aggregating stakeholders’ preferences | 3.15 | ||
Design | 3.42 | j - Identifying alternatives | 3.38 |
k - Exploring alternatives (investigating, discussing, modifying) | 3.38 | ||
l - Illustrating effects | 3.50 | ||
Choice | 3.07 | m - Selecting the best option | 3.07 |
Monitoring | 3.50 | n - Achieving goals set by the stakeholders | 3.50 |
According to the respondents, the usefulness of DSSs is higher during the “monitoring” (average 3.50) and “design” (3.42) steps. Furthermore, the phases in which the use of DSSs is considered more useful are problem structuring (3.77) and creating new knowledge (3.71). By contrast, the step in which DSSs are not perceived as very useful is the intelligence step, and in particular the phases of “identification of stakeholders” (2.33) and “definition of criteria, goals and constraints” (2.71). The low value for identifying stakeholders implies that respondents do not see DSSs as useful in providing evidence to determine the key actors to be involved in the participatory process.
Our results showed that the use of DSSs during the Intelligence phase is critical, as confirmed by previous reports ([50], [16]). According to respondents, Intelligence seems the step with the lesser usefulness for DSSs. This fact highlights the likely importance of providing users with good examples of how DSSs could be used to facilitate the Intelligence step of participatory planning. On the other hand, if the low scores for DSS usefulness are due to a lack of proper DSSs for the Intelligence step, the main recommended action is to create such software.
Some respondents indicated that they only used DSSs in some phases of the participatory processes. In particular, they indicated that “organizing the process” (28% of responses), “identification of stakeholders” (20%) and “achievement of the goals set by the stakeholders” (12%) are the phases where the use of DSS is more difficult. In order to avoid such difficulties, some improvements are needed in the development of DSSs. In this context, Van Meensel et al. ([64]) showed how the approach used in developing DSSs may result in features that positively influence critical factors, such as selecting stakeholders, setting objectives and evaluating the DSS.
Answers provided by respondents might also explain their negative perception of DSS usefulness. In most cases, respondents stated that DSSs were not used either because the properties needed to support the participatory process are missing, or the respondents failed to see the benefit DSSs might provide. In fact, most answers list as prominent the reasons “DSS available does not include properties needed” and “I cannot see the benefit DSSs would provide for the planning task”. Both reasons are related to the organization step and its “identification of stakeholders” phase.
Perceived usefulness
The PU questionnaire was completed by 25 respondents. Each group of respondents obtained a significant Cronbach’s alpha reliability at 0.05 level (Tab. 5), showing that the test used is free from random error and it is reliable for analysis purposes. These results agree with those reported by Davis ([14]), Adams et al. ([1]), and Suki & Suki ([59]).
Tab. 5 - Cronbach’s alpha reliability values for each respondent group.
Groups | Cronbach’s alpha reliability |
---|---|
A | 0.92 |
B | 0.87 |
C | 0.93 |
D | 0.86 |
Concerning the average of the six statements, very few differences among the groups were detected, indicating that experience does not seem to influence PU (Tab. 6). Nevertheless, the highest average value is associated with the PU before engaging with DSSs given by respondents reporting experience with DSS (group B).
Tab. 6 - Average (Av), standard deviation (SD) and median values (Med) of Perceived Usefulness per statement for each group of respondents.
Statement | A | B | C | D | ||||||||
---|---|---|---|---|---|---|---|---|---|---|---|---|
Av | SD | Med | Av | SD | Med | Av | SD | Med | Av | SD | Med | |
1 | 1.17 | 1.19 | 2 | 1.00 | 1.21 | 1 | 1.08 | 1.20 | 2 | 1.11 | 0.87 | 1 |
2 | 1.33 | 0.98 | 2 | 1.58 | 0.67 | 2 | 1.46 | 0.83 | 1 | 0.89 | 0.70 | 2 |
3 | 0.92 | 1.16 | 1 | 1.08 | 1.00 | 1 | 1.00 | 1.08 | 1 | 1.22 | 0.63 | 1 |
4 | 1.25 | 0.87 | 2 | 1.25 | 0.87 | 2 | 1.25 | 0.87 | 2 | 1.22 | 0.79 | 1 |
5 | 1.08 | 1.16 | 2 | 1.25 | 0.97 | 2 | 1.17 | 1.06 | 2 | 1.11 | 0.87 | 1 |
6 | 1.42 | 0.67 | 2 | 1.67 | 0.65 | 2 | 1.54 | 0.66 | 2 | 1.33 | 0.82 | 2 |
Grand mean | 1.19 | 1.00 | 2 | 1.31 | 0.91 | 2 | 1.25 | 0.96 | 1 | 1.15 | 0.79 | 1 |
The statements associated with highest average PU value were “using DSSs improves/would improve my job performance” (statement number 2 in Tab. 6) and “I find/would find DSSs useful in my job” (statement number 6 in Tab. 6), given by respondents reporting experience with DSSs (groups A, B and C). As expected, the lowest average PU value was associated with statement 2 given by respondents with no experience using DSSs (group D).
The paired group comparison of PU by mean values shows small differences in terms of overall average values, reaching values around ± 0.1 (Tab. 7). Negative values indicate that the second group has a higher value. Nevertheless, the differences increase to higher values when the analysis focuses on individual statements. The highest differences occur in response to the statements “Using DSSs improves/would improve my job performance” (statement 2 in Tab. 7), “I find/would find DSSs useful in my job” (statement 6 in Tab. 7) and “Using DSSs in my job increases/would increase my productivity” (statement 6 in Tab. 7). The comparison between the current PU reported by the whole sample (Group A) and the PU before engagement with DSSs reported by respondents with experience using DSSs (Group B) highlighted that respondents perceived DSSs as being more useful before engaging with them. Moreover, respondents reported a decrease in PU in response to statements related to the impact of DSSs on performance, productivity, and job efficacy. Regarding current PU, the comparison between respondents with experience using DSSs (group C) and respondents who have not engaged with DSSs (group D) showed a high PU difference in response to “Using DSSs improves/would improve my job performance” (statement 2 in Tab. 7). By contrast, the value in response to “using DSSs in my job increases/would increase my productivity” (statement 3 in Tab. 7) was relatively high among respondents with no experience using DSSs. Concerning the other statements, values tended to be higher among respondents engaged with DSSs or showed differences very close to zero.
Tab. 7 - Pairwise comparisons by means of the Wilcoxon’s test of average Perceived Usefulness values per statement.
Statement | B-C | C-D | B-D |
---|---|---|---|
1 | -0.08 | -0.03 | -0.11 |
2 | 0.12 | 0.46 | 0.69 |
3 | 0.08 | -0.22 | -0.14 |
4 | 0.00 | 0.03 | 0.03 |
5 | 0.08 | 0.06 | 0.14 |
6 | 0.13 | 0.21 | 0.33 |
Grand mean | 0.06 | 0.10 | 0.16 |
The Mann-Whitney-Wilcoxon test showed that the data were not statistically different when comparing pairwise groups in B-C (p-value = 0.5967), C-D (p-value = 0.6338), or B-D (p-value = 0.2536). Results indicate that no significant differences in perception were due to experience with DSSs.
Conclusions
The response rate achieved in this study confirms the evidence reported by Dennis ([17]), who suggested that when e-mailing questionnaires, attention must be paid to motivating additional potential respondents by clearly explaining the objectives of the research. Nonetheless, both questionnaires achieved a high Cronbach’s alpha reliability value, showing that they are free from random error and that the methodology is robust.
In general, respondents consider DSSs useful. Nevertheless, PU was higher before actually engaging with DSSs. This suggests that either respondents’ expectations were unrealistic or the DSS development project failed to meet the users’ needs. Results highlighted further differences in PU in terms of improving job performance. In this case, the PU reported by groups A, B and C is higher than the PU reported by group D, suggesting that DSS use may actually improve job performance more than was expected. This outcome confirms the results by Kamis et al. ([31]) who reported that, in general, DSS use may increase perceived usefulness directly or through perceived ease of use.
The main reasons indicated to explain why DSSs were not used in some phases during a participatory planning process were “available DSS does not include properties needed” and “DSSs are not believed to provide benefits for the planning task”. Cox ([12]) and Newman et al. ([47]) examined and reviewed the main problems in adopting DSSs in agriculture. According to our results, they suggested that DSS process models may be inadequate in terms of distinguishing practitioners’ matters. The latter hypothesized that the benefits of many DSSs are unclear in the development stage. Therefore, the system does not meet the need of any group of users. Nevertheless, as a general rule, DSSs appeared useful at each step of the participatory process, although differences did emerge among steps. Problem structuring, monitoring the achievement of goals set by the stakeholders and creating new information and knowledge were the steps at which the use of DSS seems to be more helpful. By contrast, DSSs were considered less useful in identifying stakeholders, defining criteria, goals and constraints, and eliciting decision-maker’s preferences. According to Sheppard & Meitner ([57]), enhancing the technical descriptions of models and methodologies incorporated in a DSS might help increase the PU of a DSS.
The main limit of this research is the small number of respondents. This limitation is firstly due to the fact that very few DSS final users are actively involved in participatory planning at present. However, the qualitative analysis reported here may contribute to the advancement of knowledge on the usefulness of DSSs as perceived by forest stakeholders.
Our results suggest that DSS developers should pay close attention to users’ perception in order to increase both the usefulness and PU of DSSs. In particular, the development of software that can overcome constraints in the intelligence phases is needed.
Given the rapid development in participatory forest planning, further investigation is required to improve methodologies aimed at effectively collecting up-to-date information about DSS final users’ opinions and perspectives. This is influential for the definition of approaches to enhance DSS architectures/structures and development processes, thus fostering the successful use of DSSs in forest management planning participatory processes.
Acknowledgements
This paper is a result of a short-term scientific mission (STSM) developed for the Working Group on participatory processes of the COST-action FP0804-Forest Management Decision Support Systems (FORSYS).
The authors have contributed to this paper in equal parts.
References
Online | Gscholar
CrossRef | Gscholar
Online | Gscholar
Gscholar
Online | Gscholar
Gscholar
CrossRef | Gscholar
Gscholar
Gscholar
Gscholar
Gscholar
Gscholar
Gscholar
Gscholar
Gscholar
Gscholar
Gscholar
Gscholar
Gscholar
Gscholar
Gscholar
CrossRef | Gscholar
Authors’ Info
Authors’ Affiliation
Consiglio per la Ricerca in Agricoltura e l’analisi dell’economia agraria, Forest Monitoring and Planning Research Unit (CRA-MPF), p.zza Nicolini 6, I-38123 Villazzano di Trento (Italy)
Forest Research Centre, School of Agriculture, University of Lisbon, Apada da Ajuda, 1349-017 Lisboa (Portugal)
Consiglio per la Ricerca in Agricoltura e l’analisi dell’economia agraria, Agrobiology and Pedology Research Center, p.zza M. D’Azeglio 30, I-50121 Firenze (Italy)
Corresponding author
Paper Info
Citation
Pastorella F, Borges JG, De Meo I (2016). Usefulness and perceived usefulness of Decision Support Systems (DSSs) in participatory forest planning: the final users’ point of view. iForest 9: 422-429. - doi: 10.3832/ifor1356-008
Academic Editor
Luca Salvati
Paper history
Received: May 20, 2014
Accepted: Aug 20, 2015
First online: Jan 02, 2016
Publication Date: Jun 01, 2016
Publication Time: 4.50 months
Copyright Information
© SISEF - The Italian Society of Silviculture and Forest Ecology 2016
Open Access
This article is distributed under the terms of the Creative Commons Attribution-Non Commercial 4.0 International (https://creativecommons.org/licenses/by-nc/4.0/), which permits unrestricted use, distribution, and reproduction in any medium, provided you give appropriate credit to the original author(s) and the source, provide a link to the Creative Commons license, and indicate if changes were made.
Web Metrics
Breakdown by View Type
Article Usage
Total Article Views: 41118
(from publication date up to now)
Breakdown by View Type
HTML Page Views: 34438
Abstract Page Views: 2136
PDF Downloads: 3599
Citation/Reference Downloads: 15
XML Downloads: 930
Web Metrics
Days since publication: 3037
Overall contacts: 41118
Avg. contacts per week: 94.77
Article Citations
Article citations are based on data periodically collected from the Clarivate Web of Science web site
(last update: Feb 2023)
Total number of cites (since 2016): 7
Average cites per year: 0.88
Publication Metrics
by Dimensions ©
Articles citing this article
List of the papers citing this article based on CrossRef Cited-by.
Related Contents
iForest Similar Articles
Research Articles
Public participation GIS to support a bottom-up approach in forest landscape planning
vol. 6, pp. 347-352 (online: 29 August 2013)
Research Articles
Communicating spatial planning decisions at the landscape and farm level with landscape visualization
vol. 7, pp. 434-442 (online: 19 May 2014)
Research Articles
Public participation in sustainable forestry: the case of forest planning in Slovakia
vol. 7, pp. 414-422 (online: 19 May 2014)
Editorials
Spatial information and participation in socio-ecological systems: experiences, tools and lessons learned for land-use planning
vol. 7, pp. 349-352 (online: 19 May 2014)
Research Articles
Application of indicators network analysis to support local forest management plan development: a case study in Molise, Italy
vol. 5, pp. 31-37 (online: 27 February 2012)
Review Papers
Public participation: a need of forest planning
vol. 7, pp. 216-226 (online: 27 February 2014)
Research Articles
A Decision Support System for trade-off analysis and dynamic evaluation of forest ecosystem services
vol. 11, pp. 171-180 (online: 19 February 2018)
Research Articles
A model of shrub biomass accumulation as a tool to support management of Portuguese forests
vol. 8, pp. 114-125 (online: 27 July 2014)
Technical Advances
Forest planning and public participation: a possible methodological approach
vol. 5, pp. 72-82 (online: 12 April 2012)
Technical Advances
A WebGIS tool to support forest management at regional and local scale
vol. 16, pp. 361-367 (online: 13 December 2023)
iForest Database Search
Search By Author
Search By Keyword
Google Scholar Search
Citing Articles
Search By Author
Search By Keywords
PubMed Search
Search By Author
Search By Keyword