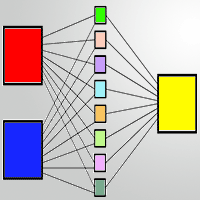
Artificial intelligence associated with satellite data in predicting energy potential in the Brazilian savanna woodland area
João Victor Nobre Carrijo (1) , Eder Pereira Miguel (1), Ailton Teixeira Do Vale (1), Eraldo Aparecido Trondoli Matricardi (1), Thiago Campos Monteiro (2), Alba Valéria Rezende (1), Jonas Inkotte (1)
iForest - Biogeosciences and Forestry, Volume 13, Issue 1, Pages 48-55 (2020)
doi: https://doi.org/10.3832/ifor3209-012
Published: Feb 05, 2020 - Copyright © 2020 SISEF
Research Articles
Abstract
The use of artificial intelligence to generate information of the savanna’s energy capacity may support sustainable management of those areas. We assessed the efficacy of artificial neural networks (ANNs) combined with satellite data to estimate the energy potential (Pe) for cerradão, a dense savannah-like vegetation type in Brazil. We conducted a forest inventory for measuring dendrometric variables and sampling woody materials and barks in a cerradão area in the state of Tocantins, Brazil. The Pe of cerradão biomass was estimated based on the observed higher calorific power and drier biomass values. Six vegetation indices were retrieved from a RapidEye image and tested for correlation to choose the optimum vegetation index for biomass modeling. The basal area and the Normalized Difference Vegetation Index were used as predictors in the Pe modeling. We estimated an average of 19.234 ± 0.411 GJ ton-1 and 19.878 ± 1.090 GJ ton-1 for higher heating values of the wood species and barks, respectively, and an average Pe of 1022.660 GJ ha-1. The best ANN showed an error of 11.3% by using a structure of two, eight, and one neurons in the input layer, in the hidden layer, and in the output layer, respectively, as well as activation functions of the tangential and sigmoidal types. The validation tests showed no significant difference between the observed and ANN-predicted values. Based on our results, we concluded that Pe can be efficiently predicted by combining ANNs and remotely sensed data, which ultimately is a promising tool for forest sustainable management of the cerrado ecosystems.
Keywords
Artificial Neural Networks, Cerrado, Higher Heating Value, Biomass, Modelling, Forestry
Authors’ Info
Authors’ address
Eder Pereira Miguel 0000-0001-6259-4594
Ailton Teixeira Do Vale
Eraldo Aparecido Trondoli Matricardi 0000-0002-5323-6100
Alba Valéria Rezende
Jonas Inkotte 0000-0001-6151-6658
Department of Forestry, Faculty of Technology, University of Brasília, Brasília, 70910-900 (Brazil)
Department of Forestry and Forest Technology, Federal University of Paraná, Av. Prefeito Lothário Meissner, 632 - Jardim Botnico, Curitiba, 80210-170 (Brazil)
Corresponding author
Paper Info
Citation
Carrijo JVN, Miguel EP, Teixeira Do Vale A, Matricardi EAT, Monteiro TC, Rezende AV, Inkotte J (2020). Artificial intelligence associated with satellite data in predicting energy potential in the Brazilian savanna woodland area. iForest 13: 48-55. - doi: 10.3832/ifor3209-012
Academic Editor
Carlotta Ferrara
Paper history
Received: Aug 05, 2019
Accepted: Nov 26, 2019
First online: Feb 05, 2020
Publication Date: Feb 29, 2020
Publication Time: 2.37 months
Copyright Information
© SISEF - The Italian Society of Silviculture and Forest Ecology 2020
Open Access
This article is distributed under the terms of the Creative Commons Attribution-Non Commercial 4.0 International (https://creativecommons.org/licenses/by-nc/4.0/), which permits unrestricted use, distribution, and reproduction in any medium, provided you give appropriate credit to the original author(s) and the source, provide a link to the Creative Commons license, and indicate if changes were made.
Web Metrics
Breakdown by View Type
Article Usage
Total Article Views: 33059
(from publication date up to now)
Breakdown by View Type
HTML Page Views: 28481
Abstract Page Views: 1834
PDF Downloads: 2255
Citation/Reference Downloads: 1
XML Downloads: 488
Web Metrics
Days since publication: 1610
Overall contacts: 33059
Avg. contacts per week: 143.73
Citation Metrics
Article Citations
Article citations are based on data periodically collected from the Clarivate Web of Science web site
(last update: Feb 2023)
Total number of cites (since 2020): 2
Average cites per year: 0.50
Publication Metrics
by Dimensions ©
Articles citing this article
List of the papers citing this article based on CrossRef Cited-by.
References
Propriedades energéticas da madeira e do carvão vegetal de Cenostigma macrophyllum: subsídios ao uso sustentável [Wood and charcoal energy properties of Cenostigma macrophyllum: subsidies to sustainable use]. Pesquisa Florestal Brasileira 38: 1-9. [in Portuguese]
CrossRef | Gscholar
Redes neurais artificiais: teoria e aplicações [Artificial neural network: theory and applications] (2nd edn). LTC, Rio de Janeiro, Brazil, pp. 226. [in Portuguese]
Gscholar
Applied regression analysis (3rd edn). John Wiley and Sons, New York, USA, pp. 407.
Gscholar
Fitossociologia da vegetação arbórea [Phytosociology of tree vegetation]. In: “Biogeografia do bioma Cerrado: vegetação e solos da Chapada dos Veadeiros” [Cerrado biome biogeography: vegetation and soils of Chapada dos Veadeiros] (Felfili JM, Rezende AV, da Silva Jr. MC eds). Editora Universidade de Brasília, Finatec, Brasília, Brazil, pp. 45-96. [in Portuguese]
Gscholar
Econometria básica [Basic econometrics] (5th edn). AMGH, New York, USA, pp. 924. [in Portuguese]
Gscholar
Redes neurais: princípios e prática [Neural networks: principles and practice] (2nd edn). Bookman, Porto Alegre, Brazil, pp. 900. [in Portuguese]
Gscholar
Forest mensuration (3rd edn). John Wiley, New York, USA, pp. 402.
Gscholar
Caracterização dos lenhos de cerne, alburno e transição de jatobá (Hymenaea sp.) visando ao agrupamento para fins de secagem convencional [Characterization of heartwood, sapwood and transition wood of jatoba wood (Hymenaea sp.) aiming at its grouping in conventional kiln drying]. Scientia Forestalis 36: 279-284. [in Portuguese]
Gscholar
Aspectos socioambientais da produção de carvão vegetal de origem nativa em uma área de cerrado em Jerumenha, Piauí/Brasil [Social and environmental aspects of the charcoal production of native origin in an area of savannah in Jerumenha, Piauí, Brazil]. Espacios 37: 18. [in Portuguese]
Gscholar
Modelos para estimativa de variáveis florestais com a utilização de imagens multiespectrais [Models of forest variables estimation using multispectral images]. Pesquisa Florestal Brasileira 37: 171-181. [in Portuguese]
CrossRef | Gscholar
Densidade e poder calorífico como base para prevenção de incêndios florestais sob linhas de transmissão [Density and calorific power as a basis for forest fires prevention under transmission lines]. Nativa 3: 10-15. [in Portuguese]
CrossRef | Gscholar
Redes neurais artificiais para a modelagem do volume de madeira e biomassa do cerradão com dados de satélite [Artificial neural network for modeling wood volume and aboveground biomass of tall Cerrado using satellite data]. Pesquisa Agropecuaria Brasileira 50: 829-839. [in Portuguese]
CrossRef | Gscholar
Amostragem de discos e uso de equações para estimar a densidade básica da madeira em diversas fitofisionomias [Disc sampling and use of equations for estimate the basic wood density in several phytophysiognomies]. Ciência Florestal 28: 1615-1626. [in Portuguese]
CrossRef | Gscholar
Caracterização energética de pellets in natura e torrificados produzidos com madeira residual de Pinus [Energy characterization of fresh and torrified pellets produced from Pinus waste wood]. Pesquisa Florestal Brasileira 35: 435. [in Portuguese]
CrossRef | Gscholar
Poder calorífico da madeira e de resíduos lignocelulósicos [Calorific value of wood and wood residues]. Biomassa & Energia 1: 173-182. [in Portuguese]
Gscholar
Densidade básica e potencial energético de espécies lenhosas do Cerrado do estado de Tocantins [Basic density and energy potential of woody species of the Cerrado of Tocantins state]. Doctoral thesis, Forestry Department, Universidade de Brasília, Brasília, Brazil, pp. 66. [in Portuguese]
Gscholar
Redes neurais artificiais para estimar a densidade básica de madeiras do cerrado [Artificial neural network to estimate the basic density of cerrado wood]. Pesquisa Florestal Brasileira 38: 1-10. [in Portuguese]
CrossRef | Gscholar
Perfil florístico e estrutural do componente lenhoso em seis áreas de cerradão ao longo do bioma Cerrado [Structural and floristic profile of the woody component of six cerradão areas]. Acta Botanica Brasilica 26: 328-341. [in Portuguese]
CrossRef | Gscholar
Estimativa da altura de eucalipto por meio de regressão não linear e redes neurais artificiais [Eucalyptus height estimation by nonlinear regression and artificial neural networks]. Revista Brasileira de Biometria 33: 556-569. [in Portuguese]
CrossRef | Gscholar
Diverse relationships between forest growth and the Normalized Difference Vegetation Index at a global scale. Remote Sensing of Environment 187: 14-29.
CrossRef | Gscholar
Genetics of wood production. Springer-Verlag, Berlin, Germany, pp. 337.
Gscholar