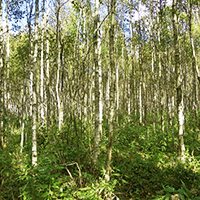
Comparing land use registry and sample based inventory to estimate forest area in Podlaskie, Poland
iForest - Biogeosciences and Forestry, Volume 10, Issue 1, Pages 315-321 (2017)
doi: https://doi.org/10.3832/ifor2078-009
Published: Feb 23, 2017 - Copyright © 2017 SISEF
Research Articles
Abstract
The Land and Property Register (LPR) of Poland contains information on land use for the entire country. Additionally, a sample-based National Forest Inventory (NFI) provides statistical data for forest areas and detailed information on numerous forest parameters. However, until 2014 NFI plots were established only on areas classified as forest in the LPR. In this article, we present results of an estimation of forest area by extending the NFI measurements on all theoretical points in a grid, and compare the results with LPR information for one province (the Podlaskie voivodeship). At each point, we assess land use with recent aerial photographs and verify the ambiguous points in the field. Forest area in Poland is increasing due to afforestation and natural expansion of forest. Delays in the updating process of the LPR, and unwillingness of the owners to agree to reclassification of their land, have led to an underestimation of overall forest area. Our results demonstrate that forest area estimates made by the improved NFI are higher than those based upon the LPR. The modified NFI may be an appropriate tool for monitoring forest area changes in Poland.
Keywords
Land Cover, Afforestation, Natural Expansion of Trees, Land and Property Register, National Forest Inventory
Introduction
Forests cover almost 4 billion hectares globally, which corresponds to 31 percent of the world’s total land area ([18]). Reporting about forests and their diversity requires a clearly defined system of indicators at various spatial scales ([28], [30]), based directly on tree attributes or area features ([46]) and collected at regular time intervals. Forest assessment systems still need to be developed, particularly on national and global levels ([33], [4], [9]).
Forests are generally defined by the type of land use or land cover they include, or as an administrative unit ([24]). The definition of a forest is determined by the main goal of assessment ([27], [44]). Differences between national definitions, and those used by international organisations, result in mismatches between the forest areas reported on a national level and country statistics ([24]). Therefore, harmonisation of inventory systems and applied definitions may constitute a better solution than standardisation of forest data ([44], [15], [40]). However, such processes can be difficult to implement due to high costs, national conditions and varying information requirements ([22]). Thus, developing appropriate methods for converting national data to that which can be merged into international systems ([35], [41]) may be the only solution.
In most European countries, basic information about forests that is used for formulation of national forest policies and forest ecosystem monitoring comes from National Forest Inventories (NFIs - [15], [13], [40]). Lawrence et al. ([23]) noted that almost all countries that participated in COST Action E43 (“Harmonisation of National Forest Inventories in Europe: Techniques for Common Reporting”) use the percentage of crown cover and minimum forest area as basic parameters for forest definition. In some countries, the minimum tree height and minimum forest width are also used. Of the 27 European countries that took part in this process, only eight use crown cover threshold and minimum area definitions consistent with the Food and Agriculture Organization of the United Nations’ reference definition (10% crown cover and 0.5 ha minimum area - [8]) that is applied within COST Action E43 ([44]).
NFIs estimate forest area by: (1) assessment of field plots or points; (2) interpretation of plot or point locations (inside or outside the forest) using aerial photographs; or (3) assessment of maps ([23]). Because NFI plots are smaller than the minimum area used to define a forest ([13]), classification of land as forest or non-forest requires additional measurements from the area surrounding sample plots.
The continuum from treeless to fully treed vegetation types ([32]), which is manifested in diverse tree cover and composition ([19]), complicates the classification of land areas. Gabler et al. ([13]) proposed two approaches for classifying NFI sample plots as forest or non-forest: (1) a point decision based on the analysis of surrounding area of predefined shape and size; or (2) a point decision based on delineation of the surrounding land into polygons of forest and non-forest land. Using reference areas for crown cover estimation reduces assessment uncertainties ([25]). However, Kleinn ([19]) noted that the crown cover criterion is only reasonable for open forests (without contact between tree crowns). For closed forests, when a visible demarcation between forest and non-forest exists, a sample point can be defined as outside or inside the forest area ([19]).
In 2005, an assessment of forest resources at a national level was initiated in Poland, based on a systematic grid of fixed-area sample plots ([29], [38]). We emphasise that until 2014 the NFI’s sample plots were only established in those grid points that were classified as forest in the Land and Property Register (LPR). LPR is a public register, maintained by local government authorities, based on geodetic and cartographic elaborations. The frequency of LPR updating has not been defined. Delays in the reclassification of afforested land, as well as the natural expansion of trees on abandoned agricultural lands, have led to areas meeting the criteria for forested land but not being registered in the LPR as forest. The goal of this paper is to study the usability of LPR for forest area estimation and to propose an improved method for monitoring of forest area in Poland.
Materials and methods
Definition of forests in Poland
Polish law defines forest as a land with forest vegetation (with trees, shrubs and herbaceous ground cover) covering an area of at least 0.1 ha. Forest land can be temporarily unstocked as a result of forest management or natural disasters. Lands related to forestry (such as forest roads and nurseries) are also included in the forest area. Elements of forest structure, such as the minimum tree height or canopy cover are not highlighted in this definition; nevertheless, acknowledgement of an area as a forest strictly depends upon its land use classification as defined by the Land and Property Register ([16]). Generally, forest land is protected by law, and deforestation is only allowed with the consent of the Minister of Environment.
Since the Second World War, a vast increase in forest area as a result of afforestation and natural succession has occurred in Poland. Almost 1.5 million hectares of wasteland (unproductive marshy or sandy sites) and agricultural land was afforested between 1945 and 2012 ([7]). Due to fragmentation of land ownership, very narrow parcels were often planted with trees. In the definition of a forest used for reporting under the United Nations Framework Convention on Climate Change (UNFCCC), a minimum width of 10 m is required for an area to be classified as forest, except in areas with small properties. However, 0.1 hectares has been kept as the minimum area threshold ([20]). Under the UNFCCC, only those forests included in LPR are reported. The minimum width of 20 meters recommended by the FAO ([8]) for an area to be classified as forest has only been implemented for afforestation achieved with European Agriculture Guidance and Guarantee Funds (EAGGF). The minimum area for this afforestation (0.3 hectares) is also lower than the 0.5 ha FAO threshold.
Reclassification of land from non-forest to forest, particularly that resulting from the natural expansion of trees on abandoned lands, is accomplished using criteria implemented in the State Forests for distinguishing between stocked and unstocked areas. For trees older than 20 years, the stocking index criterion (the growing stock per ha compared to yield table values) is applied. For 21-40 year-old stands, the stocking index cannot be less than 0.4, whilst for older stands, the index cannot be less than 0.3. For the youngest stands (under 20 years old) the criterion of tree occurrence is used, where there should be at least 50% trees compared to the planting density. However, afforested areas established with EAGGF funds are reclassified from non-forest to forest land (in LPR) after 4-5 years if at least 70% of the planted trees are alive. For natural succession, a threshold of 50% is used.
Object of the study
The NFI methodology implemented in Poland uses a 4×4 kilometre grid with clusters consisting of five plots ([29], [38]). Under this scheme, there are almost 99.000 theoretical sample points in Poland. Out of this number, we analysed the land cover of 6429 NFI points located in northeast Poland (Fig. 1) within the area of the Podlaskie voivodeship (province).
The total area of the Podlaskie voivodeship is 2.018.702 hectares, of which 1.991.100 is land area ([6]). According to the Central Statistical Office database, at the end of 2012, forest covered 628.400 hectares of the study area, or 31.1% of the total area and 31.6% of the land area of the voivodeship ([7]). Approximately 68% of the forests within the study area are public and managed by the State Forest Holding. The proportion of private forests (32.0%) is higher than average for Poland (18.4%).
Within the first five-year cycle of the NFI (2005-2009 - NFI I), 1927 sample plots were established in the forest area of the voivodeship. Our study commenced during the second cycle of NFI (NFI II), therefore the total number of plots for 2nd cycle was calculated as the sum of: (1) the measured real data from the first three years of NFI II; and (2) the number of plots planned to be measured during 2013-2014. The second number was assessed in 2012, by examination of land use category in LPR at theoretical NFI points, by the body responsible for NFI field assessment. As the result, 1995 sample points were classified as located on forest land, according to the Polish definition, 68 forest plots from NFI I have now been classified as non-forest, and 136 non-forest points in NFI I are now identified as being in forest, according to the LPR.
Photographic interpretation and field survey
Analysis of land cover within the study area was conducted in 2012-2013 independently from the Land and Property Register records (Fig. 2). Firstly, the 6429 NFI points in the Podlaskie voivodeship were plotted on an orthophotograph (RGB, 0.25 m pixel size) maintained by the Geodesy and Cartography Documentation Centre. Source aerial images were taken between 2002 and 2010, but the vast majority of the study area (approximately 85%) was covered with images taken in 2006 and later. Some images, especially along the Belarus border, were acquired before 2006 or came from satellite imagery.
Forested areas in Poland are generally closed forests. Thus, it was usually possible to clearly define the location of sample points as inside or outside of forested land. There were only few points located close to the forest borders. In those cases, a decision was made based on position of tree tops or stumps, without field verification. For the sample points located inside the wooded land, the area of small forest patches was estimated using QGIS software. The minimum area threshold of the forest (0.1 ha for the national definition and 0.5 ha for the FAO definition) was verified.
Any doubtful points that resulted from unsatisfactory orthophotographic quality or a lack of closed forest character were selected for further examination. These include the areas with possible agricultural land use, such as fruit tree plantations or urban land use.
Assessment of temporarily unstocked areas (resulting from natural disturbances or forest management practises such as clear-cutting) was made using auxiliary data from the NFI and 23 forest divisions located within the Podlaskie voivodeship. When a doubtful point was located on an area described as temporarily unstocked forest land, it was classified as a forest. Neither remote sensing data, satellite observations, nor aerial photos are sufficient to assess forest cover trends ([43]).
Any doubtful points, not recognised as unstocked forest land, were selected for field assessment, which was performed in the second half of 2012. Some areas that were inaccessible at that time of year (boggy, marshy sites), mainly located in Biebrza National Park, were assessed in 2013.
Classification of sample points as forest or non-forest land during field verification utilised visual evaluation of the crown cover, with thresholds referring to criteria by which to distinguish between stocked and unstocked areas in the State Forest Holding on a minimum area of 0.1 ha (see section “Definition of Forest in Poland”). Although many precise instruments have been developed to measurement crown (canopy) cover ([17], [21]), they are relatively time-consuming in practice ([14], [34], [11], [21]). This impracticality makes methods applicable to scientific studies rather unreliable for routine inventories ([34]). For example, measuring crown cover in sample plots using a densiometer ([17], [11]) or Cajanus tube ([34], [21]) is impractical and ineffective in the context of defining large areas as forest and non-forest to aid in decision-making.
Statistical assessment
Forest area was estimated as a product of the study area and proportion of points located in the forest. The area of the voivodeship is well known from official statistics.
Proportion of forest was estimated using a binominal distribution approach, as follows ([5] - eqn. 1):
where hat{p} is the estimated proportion of forest, n is the number of clusters, mi is the number of points in cluster i and fi is the number of forest points in cluster i.
The standard error of the proportion of forest was estimated to be the square root of the sampling variance, calculated with a formula appropriate for cluster sampling ([5], [12] - eqn. 2):
Using normal approximation ([5]), 95% confidence limits for the true proportion of forest (P) were calculated as follows (eqn. 3):
where t is the normal deviate corresponding to confidence probability.
Results
Photographic interpretation
As a result of the photographic interpretation, 2066 points were classified as forest (according to minimum forest area and canopy cover applied in Poland), 4233 as non-forest, and 130 for field checking (Tab. 1). From the 68 sample plots established during NFI I (2005-2009) and classified as non-forest using LPR during NFI II (2010-2014), 57 plots were classified as forest based upon land cover assessment in the photographs. Accordingly, from the 136 points classified as non-forest in NFI I and as forest in NFI II using the LPR data, 36 points were found to be non-forest and 14 were designated for field verification. Our analysis also revealed 76 points, located on the always non-forest LPR land, with tree cover satisfying the forest definition. Examples of areas classified as forest during photographic interpretation are presented in Fig. 3.
Tab. 1 - Results of NFI points classification based on photographic interpretation and field assessment in relation to NFI I-II.
Forests defined by LPR | Photographic interpretation (with support of auxiliary information) |
Field assessment | |
---|---|---|---|
NFI I (2005-2009) |
NFI II (2010-2014) |
||
1927 - forest | 1859 - forest (remeasured) | 1847 - forest | 3 - forest 1 - non-forest |
4 - for field verification | |||
8 - non-forest | |||
68 - non-forest (removed) | 57 - forest | 2 - forest 1 - non-forest |
|
3 - for field verification | |||
8 - non-forest | |||
4502 - non-forest |
136 - forest (new plots) |
86 - in forest | 12 - forest 2 - non-forest |
14 - for field verification | |||
36 - non-forest | |||
4366 - non-forest | 76 - forest | 70 - forest 39 - non-forest |
|
109 - for field verification | |||
4181 - non-forest | |||
Total number of points | 2066 - forest | 87 - forest 43 - non-forest |
|
130 - for field verification | |||
4233 - non-forest |
Fig. 3 - NFI’s cluster no. 151144. Sample points 3-5 are classified as forest based on photographic interpretation. Only plot 3 belongs to forest land within the LPR and thus it was measured during the NFI II (a 30 year-old silver birch and black alder stand in 2013). Points 4 and 5 are recorded as agricultural land in LPR. In 2008 (NFI I) all of the points were recorded as non-forest in LPR.
We found that 10 out of 2066 points were placed in patches of forest with an area less than 0.5 hectares, i.e., they are not forests based on the FAO definition.
We should emphasise that some of the points classified as non-forest (14 of 1995 measured in NFI II) are located on areas recorded in LPR as forest. This discrepancy may have been due to the lack of division of land parcels into various land use classes in the LPR, in situations where forest and agricultural or pastoral lands are located in the same land parcel (Fig. 4).
Fig. 4 - NFI’s sample plot no. 1301565, located in a parcel described as forest in the LPR. Red lines denote LPR parcel boundaries.
As a result of photographic interpretation, 130 sample points were selected for field verification. According to the LPR, these points are located in: forest areas (18), woodlands (18), pastures (15), wastelands (28), agricultural lands (46), and other areas (5).
Field assessment
During field assessment, 87 out of the 130 points were classified as forest (Tab. 1). In three cases, the area of these forest patches was lower than 0.5 hectares.
In general, three categories of forest were distinguished on lands classified as non-forest in the LPR, depending upon their origins and current status. The first category includes stands previously classified as woodlands (land with a crown cover or stocking index that does not fulfill the national definition of forest) that have not yet been reclassified as forests in the LPR (Fig. 5). The second category includes naturally afforested stands of birch, alder, pine or spruce, on wastelands and abandoned agricultural lands (Fig. 6). The last category includes stands resulting from planting, which have not yet been recorded in the LPR. Such stands are usually relatively young (Fig. 7).
Fig. 5 - An approximately 70 year-old black alder (Alnus glutinosa L. Gaertn.) stand in Biebrza National Park in an area classified in the LPR as a woodland.
Fig. 6 - An approximately 25 year-old downy birch (Betula pubescens Ehrh.) stand in Biebrza National Park in an area classified in the LPR as a wasteland.
Fig. 7 - An approximately 30 year-old Scots pine (Pinus sylvestris L.) stand that afforested an area classified in the LPR as agricultural land.
Four of the 130 sample points assessed in the field were not recognised as forest, but could be classified as “other land with tree cover” according to the FAO ([8]) categories. This definition derives from their use for purposes other than forestry (such as cemeteries and urban or industrial areas).
Statistical assessment
Based upon photographic interpretation and field assessment, 2153 sample points (33.5% of the total points from the NFI grid in the study area) were classified as forest.
These numbers correspond to a total forest area of 676.300 ha, which is nearly 48.000 ha more than the official LPR-based estimate. The standard error of estimated forest percentage using eqn. 2 is 1.1% (note that the forest proportion was converted to a percentage by multiplying by 100). Thus, there is a 95% probability that the true percentage of forest area is between 31.3% and 35.7% (631.900 and 720.700 ha). The lower limit of the confidence interval is still 3500 ha higher than the officially estimated forest area of the voivodeship.
Using the FAO’s minimum area for a forest (0.5 hectares), 2140 of the NFI points met the definition of a forest. This corresponds to a forest percentage of 33.3% ± 1.1% (standard error).
Discussion and conclusions
Forests, similar to other ecosystems, are significantly influenced by human impact ([45]). The net change in forest area from 1990-2015 is estimated to have been -5.1 million hectares per year around the world ([18]). In contrast, the forest area in Europe has been increasing steadily since the 1990s, and the net change is estimated to be 0.8 million hectares per year during the last 25 years ([18]).
The forest area in Poland increased by approximately 485.000 hectares between 1990 and 2012, but the area of afforestation during this period was only 315.000 hectares ([16]). Two main processes likely cause this inconsistency in forest area balance: (1) reclassification of afforestation made before 1990; and (2) natural expansion of forests on abandoned agricultural lands. Our results show that forest area is still underestimated in Poland, because of these reasons.
The percentage of forest land in the Podlaskie voivodeship estimated in our studies (based only on land cover and land use) is approximately 2.4 percentage units higher than official (LPR) data states (33.5% and 31.1%, respectively). This difference refers to Polish thresholds of minimum crown cover, applied within our study. Using the FAO criterion for canopy cover, forest area in Poland could be even greater.
Defining a forest as an area with a minimum 10% canopy cover, as in the FAO ([8]) definition, is justified by the significant diversity of forest ecosystems that range from closed to open. Because of ecological zones and natural timberlines, a low threshold is applicable to forests throughout the world ([42]). Forests in Poland, except for some marshy sites, are closed. For this reason, we chose a higher threshold for crown cover in this study than the FAO proposal. Consequently, some areas in the initial stages of colonising abandoned agricultural land were not included in forest area in our assessment. However, some forest patches (13 points in our study) could not fulfill the minimum area threshold proposed by FAO.
We observed that the accuracy of assessment is highly influenced by the selection of a suitable estimator. Using formulas for simple random sampling (SRS), with the same number of sample points and share of forested area, the standard error of the forest percentage would be almost 50% less than the error assessed by a cluster sampling estimator (0.6%) for the study area. Application of SRS formulas for cluster sampling is not advisable, due to the possibility of significant underestimation of the true standard error ([5], [36]).
The spontaneous appearance of trees on abandoned agricultural lands resulting from the decline in traditional agriculture, and accompanying changes in socioeconomic conditions, has been observed all over the world ([39], [1], [3]). The phenomenon is also observed in Poland, however its extent is unknown ([37]). These changes prompt questions regarding the definition of forest and classification of land. Forest land recorded in the Land and Property Register in Poland is protected by law and special permission is required for deforestation, whilst abandoned agricultural land with tree cover can be converted to arable land according to good practice guidelines for agricultural land management. In general, land owners are not interested in removal of trees from abandoned lands, however there is still an unwillingness to reclassify such lands in LPR.
We additionally observed that the problem of defining land as a forest occurs in the case of disasters or specific disturbances. For example, flooding caused by the damming activity of beavers results in conversion of terrestrial habitats to aquatic ones ([31]). In our opinion, classification of such areas should be made independently for each case, taking into account the spatial extent and intensity of disturbance.
Results of our study highlight the need for implementing a new system for estimation of forest area in Poland. In most European countries, as well as in China, New Zealand, and the USA, forest areas are assessed within NFIs based upon systematic sampling plots ([23]). Furthermore, NFIs are key components of greenhouse gas removal/emission reporting under the UNFCCC and its Kyoto Protocol ([2], [10], [26], [3]). Extension of the Polish NFI to assess land cover on all points of the NFI grid is recommended for monitoring forest area changes in Poland, including for UNFCCC purposes.
Acknowledgments
This work was conducted with funds from the Polish State Forests Holding.
References
Gscholar
Gscholar
CrossRef | Gscholar
Gscholar
Authors’ Info
Authors’ Affiliation
Piotr Budniak
Marcin Mionskowski
Grzegorz Zajaczkowski
Department of Forest Resources Management, Forest Research Institute, Braci Lesnej 3, 05-090 Raszyn (Poland)
Natural Resources Institute Finland, PO Box 68 (Yliopistokatu 6), FI-80101 Joensuu (Finland)
Department of Natural Forests, Forest Research Institute, Park Dyrekcyjny 6, 17-230 Bialowieza (Poland)
Corresponding author
Paper Info
Citation
Jablonski M, Korhonen KT, Budniak P, Mionskowski M, Zajaczkowski G, Sućko K (2017). Comparing land use registry and sample based inventory to estimate forest area in Podlaskie, Poland. iForest 10: 315-321. - doi: 10.3832/ifor2078-009
Academic Editor
Piermaria Corona
Paper history
Received: Apr 07, 2016
Accepted: Oct 12, 2016
First online: Feb 23, 2017
Publication Date: Feb 28, 2017
Publication Time: 4.47 months
Copyright Information
© SISEF - The Italian Society of Silviculture and Forest Ecology 2017
Open Access
This article is distributed under the terms of the Creative Commons Attribution-Non Commercial 4.0 International (https://creativecommons.org/licenses/by-nc/4.0/), which permits unrestricted use, distribution, and reproduction in any medium, provided you give appropriate credit to the original author(s) and the source, provide a link to the Creative Commons license, and indicate if changes were made.
Web Metrics
Breakdown by View Type
Article Usage
Total Article Views: 39576
(from publication date up to now)
Breakdown by View Type
HTML Page Views: 34030
Abstract Page Views: 1800
PDF Downloads: 2980
Citation/Reference Downloads: 20
XML Downloads: 746
Web Metrics
Days since publication: 2631
Overall contacts: 39576
Avg. contacts per week: 105.30
Article Citations
Article citations are based on data periodically collected from the Clarivate Web of Science web site
(last update: Feb 2023)
Total number of cites (since 2017): 8
Average cites per year: 1.14
Publication Metrics
by Dimensions ©
Articles citing this article
List of the papers citing this article based on CrossRef Cited-by.
Related Contents
iForest Similar Articles
Research Articles
Land use inventory as framework for environmental accounting: an application in Italy
vol. 5, pp. 204-209 (online: 12 August 2012)
Research Articles
Afforestation monitoring through automatic analysis of 36-years Landsat Best Available Composites
vol. 15, pp. 220-228 (online: 12 July 2022)
Research Articles
Carbon storage and soil property changes following afforestation in mountain ecosystems of the Western Rhodopes, Bulgaria
vol. 9, pp. 626-634 (online: 06 May 2016)
Review Papers
Integration of forest mapping and inventory to support forest management
vol. 3, pp. 59-64 (online: 17 May 2010)
Research Articles
Assessment of land sensitivity to degradation using MEDALUS model - a case study of Grdelica Gorge and Vranjska Valley (southeastern Serbia)
vol. 15, pp. 163-170 (online: 07 May 2022)
Commentaries & Perspectives
Benefits of a strategic national forest inventory to science and society: the USDA Forest Service Forest Inventory and Analysis program
vol. 1, pp. 81-85 (online: 28 February 2008)
Research Articles
Future land use and food security scenarios for the Guyuan district of remote western China
vol. 7, pp. 372-384 (online: 19 May 2014)
Research Articles
Estimation of stand crown cover using a generalized crown diameter model: application for the analysis of Portuguese cork oak stands stocking evolution
vol. 9, pp. 437-444 (online: 02 December 2015)
Technical Reports
Deforestation, land conversion and illegal logging in Bangladesh: the case of the Sal (Shorea robusta) forests
vol. 5, pp. 171-178 (online: 25 June 2012)
Research Articles
Landsat TM imagery and NDVI differencing to detect vegetation change: assessing natural forest expansion in Basilicata, southern Italy
vol. 7, pp. 75-84 (online: 18 December 2013)
iForest Database Search
Search By Author
Search By Keyword
Google Scholar Search
Citing Articles
Search By Author
Search By Keywords
PubMed Search
Search By Author
Search By Keyword