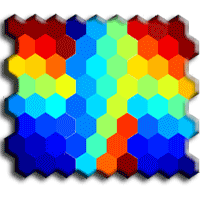
Using self-organizing maps in the visualization and analysis of forest inventory
D Klobucar (1) , M Subasic (2)
iForest - Biogeosciences and Forestry, Volume 5, Issue 5, Pages 216-223 (2012)
doi: https://doi.org/10.3832/ifor0629-005
Published: Oct 02, 2012 - Copyright © 2012 SISEF
Research Articles
Abstract
A lot of useful data on forest condition can be gathered from the Forest Inventory (FI). Without the help of data analysis tools, human experts cannot manually interpret information in such a large data set. Conventional multivariate statistical analyses provide results that are difficult to interpret and often do not represent the information in a satisfactory way. Our goal is to identify an alternative approach that will enable fast and efficient interpretation and analysis of the FI data. Such interpretation and analysis can be performed automatically with a clustering method, but all clustering methods have some shortcomings. Therefore, our aim was also to provide information in a form suitable for fast and intuitive visualization. Kohonen’s Self Organizing Map (SOM) is an alternative approach to data visualization and analysis of large multidimensional data sets. SOM provides different possibilities and our experiments are presented with component matrices of individual stand parameters and label matrices. In forming data clusters, we experimented with hierarchical and non hierarchical clustering methods. Our experiments showed that SOM provides useful information in a form suitable for data clustering and data visualization. This enables an efficient analysis of large FI data sets at different analysis scales. Clustering results obtained with SOM and two clustering algorithms are in accordance with ground truth. We have also considered the efficiency of SOM component matrices by visual comparison and correlation among structural parameters and by determining contributions of individual stand parameters to clustering input data. SOM application in visualization and analysis of stand structural parameters enables gathering quickly and efficiently holistic information on the current condition of forest stands and forest ecosystem development. Therefore we recommend the application of Kohonen’s SOM for visualization and analysis of FI data.
Keywords
Forest Inventory, Stand Structural Parameters, Self-organizing Maps, Forest Data Visualization, Neural Networks
Authors’ Info
Authors’ address
Hrvatske sume Ltd., Croatian National Forestry Agency, Ljudevita F. Vukotinovica 2, 10000 Zagreb (Croatia)
Faculty of Electrical Engineering and Computing, University of Zagreb, Unska 3, 10000 Zagreb (Croatia)
Corresponding author
Paper Info
Citation
Klobucar D, Subasic M (2012). Using self-organizing maps in the visualization and analysis of forest inventory. iForest 5: 216-223. - doi: 10.3832/ifor0629-005
Academic Editor
Marco Borghetti
Paper history
Received: May 15, 2012
Accepted: Sep 14, 2012
First online: Oct 02, 2012
Publication Date: Oct 30, 2012
Publication Time: 0.60 months
Copyright Information
© SISEF - The Italian Society of Silviculture and Forest Ecology 2012
Open Access
This article is distributed under the terms of the Creative Commons Attribution-Non Commercial 4.0 International (https://creativecommons.org/licenses/by-nc/4.0/), which permits unrestricted use, distribution, and reproduction in any medium, provided you give appropriate credit to the original author(s) and the source, provide a link to the Creative Commons license, and indicate if changes were made.
Web Metrics
Breakdown by View Type
Article Usage
Total Article Views: 57097
(from publication date up to now)
Breakdown by View Type
HTML Page Views: 48296
Abstract Page Views: 2770
PDF Downloads: 4619
Citation/Reference Downloads: 47
XML Downloads: 1365
Web Metrics
Days since publication: 4316
Overall contacts: 57097
Avg. contacts per week: 92.60
Citation Metrics
Article Citations
Article citations are based on data periodically collected from the Clarivate Web of Science web site
(last update: Feb 2023)
Total number of cites (since 2012): 11
Average cites per year: 0.92
Publication Metrics
by Dimensions ©
Articles citing this article
List of the papers citing this article based on CrossRef Cited-by.
References
Forest tree mortality simulation in uneven-aged stands using connectionist networks. In: Proceedings of the “International conference on engineering applications of neural networks” (Liljenström H, Bulsari AB eds). Stockholm (Sweden), 16-18 June 1997, pp. 341-348.
Gscholar
Exploratory data analysis by the SOM: structures of welfare and poverty in the world. In: Proceedings of the 3rd “International conference on neural networks in the capital markets” (Abu-Mostafa A-PN, Moody Y, Weigend A eds). Singapore 1996, pp. 498-507.
Gscholar
Estimation of stands parameters from IKONOS satellite images using textural features. In: Proceedings of the 7th International Symposium on “Image and signal processing and analysis” (Loncaric S, Ramponi G, Sersic D eds). Dubrovnik (Croatia) 4-6 September 2011, pp. 491-496.
Gscholar
Self-organizing maps (3rd edn). Series in Information Sciences, vol. 30. Springer, Berlin, Germany.
Gscholar
Numerical ecology. Elsevier Science BV, Amsterdam, The Netherlands, pp. 870.
Gscholar
Dendrométrie. Ecole Nationale des Eaux et Forêts, Louis-Jean GAP, Paris, France, pp. 350.
Gscholar
Recent application of artificial neural networks in forest resource management: an overview. In: Proceedings of the Meeting “Environmental decision support systems and artificial intelligence” (Corté U, Srnchez-Marrc M eds). Orlando (FL - USA) 18 July 1999. AAAI Press, Palo Alto, CA, USA, pp. 15-22.
Gscholar
Survey and comparison of quality measures for SOM. In: Proceedings of the 5th “Workshop on data analysis” (Paralic J, Pölzlbauer G, Rauber A eds). Vysoké Tatry (Slovakia) 24-27 June 2004. Elfa Academic Press, pp. 67-82.
Gscholar
Ecological modelling with self-organising maps. In: Proceedings of the International Congress on “Modelling and simulation; integrative modelling of biophysical, social and economic systems for resource management solutions” (Post DA ed). Townsville (Australia) 14-17 July 2003, pp. 759-764.
Gscholar
Prirasno prihodne tablice. Sumarski Institut Jastrebarsko, Zagreb, Croatia, pp. 103. [in Croatian]
Gscholar
Finding profiles of forest nutrition by clustering of the SOM. In: Proceedings of the Workshop on “Self-organizing maps”. Kitakyushu (Japan) 11-14 September 2003, pp. 243-248.
Gscholar
SOM Toolbox for Matlab 5 Documentation. Helsinki University of Technology, Helsinki, Finland.
Gscholar
The visualization capability of SOMs to detect deviation in distribution control. TUCS Technical Report 153, Centre for Computer Science, Turku, Finland.
Gscholar