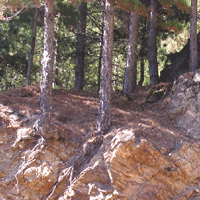
Ecological and anthropogenic drivers of Calabrian pine (Pinus nigra J.F. Arn. ssp. laricio (Poiret) Maire) distribution in the Sila mountain range
iForest - Biogeosciences and Forestry, Volume 8, Issue 4, Pages 497-508 (2014)
doi: https://doi.org/10.3832/ifor1041-007
Published: Nov 10, 2014 - Copyright © 2014 SISEF
Research Articles
Abstract
The most well-known and vast Calabrian pine forests are in the Sila mountain range, southern Italy. In this paper, present-day distribution of Calabrian pine in the Sila district was analyzed and compared with forest maps dating back to 1935 in order to assess the changes in land use. Main ecological and anthropogenic factors affecting Calabrian pine forest distribution were investigated by logistic regression models to identify the most important predictors of Calabrian pine persistence, expansion, and transition over the period 1935-2006. In 2006, the area covered by Calabrian pine forests and mixed Calabrian pine-beech forests was 36 100 ha and 20 221 ha, respectively. Overall, pine forest area increased by 38% between 1935 and 2006. Logistic regression revealed that Calabrian pine distribution in the Sila district was affected by both ecological (bioclimate, soil, and elevation) and anthropogenic factors (management, fire). Based on our results, four different potential dynamics of Calabrian pine stands are discussed in the perspective of the sustainable management and conservation of this important mountain forest habitat.
Keywords
Ecological Factors, Forest Management, Land Use Changes, Forest Dynamics
Introduction
The Mediterranean basin is one of the major plant diversity hotspots and among the richest in endemism over the world ([37], [43]). In Europe, 80% of all endemic plants are Mediterranean species ([8], [21]), and Mediterranean forests are exceptionally rich in endemics: 201 of the 290 woody species and subspecies (shrubs and trees) in Europe are found exclusively or preferentially under Mediterranean bioclimates ([49]).
Around the Mediterranean Basin, changes in land-use and different forms of forest utilization over several millennia have shaped diverse landscape mosaics with different and often impacting consequences on biodiversity ([57], [8], [7]). Forest distribution in Mediterranean landscapes is characterized by diverse environmental conditions in relation to climate, soils, and geology, as well as frequency and intensity of disturbance, both natural and anthropogenic. In the last decades, rapidly changing environmental conditions coupled with even more rapid changes in socio-economic conditions, especially in rural and mountain areas, are posing new challenges for the conservation of forest habitats ([32], [15]). Thus, an accurate knowledge base of ecological and anthropogenic drivers affecting the vegetation distribution is necessary for forest planning and for designing vegetation models ([56], [24], [29]). This knowledge is particularly important in highly sensitive areas such as in mountain territories, and especially within the Natura 2000 network, where habitat and species conservation is a mandatory goal.
Laricio pine (Pinus nigra J.F. Arn. ssp. laricio (Poiret) Maire), a sub-specific entity of the collective species Pinus nigra, includes 2 varieties: Corsican pine (var. Corsicana) and Calabrian pine (var. Calabrica - [14]). Corsican pine is the dominant species between 1000 and 1800 m a.s.l. on the mountains of Corsica, where it covers approximately 45000 ha, of which 21000 ha are pure Corsican pine stands ([30]).
Calabrian pine is endemic to southern Italy with a natural range extending from Calabria to Sicily. Calabrian pine forests (Cod. Eunis, G3.55, Calabrian Pinus laricio forest; Cod. CORINE Biotopes, 42.65, Calabrian laricio pine forests) are a priority habitat according to the Natura 2000 Directive (Cod. 9530* (Sub)-Mediterranean pine forests with endemic black pines - [19], [59]).
During the last ice age (the Würm period in the Alps) Calabrian pine covered vast areas of the Apennines. In the Boreal period, Calabrian pine expansion slowed down due to competition with silver fir (Abies alba Mill.) and beech (Fagus sylvatica L.) at higher altitudes, and with oaks at lower altitudes ([4]), restricting pine distribution to the less favorable site conditions ([58]). At the end of this period, Calabrian pine started to expand again into areas where human activities (primarily forest clearing and fire) destroyed the forest cover and caused extensive soil degradation. A pedo-stratigraphic study by Dimase et al. ([17]) showed that periods of reduced soil erosion alternated with periods of intense erosion that coincided with intensive agriculture and grazing, which occurred in the 12th to 13th centuries, then again in the 15th to 16th centuries, and finally after the 18th century (Fig. 1).
Fig. 1 - Outline of a pedostratigraphic section near Lake Cecita of the Sila Plateau (modified from [17]). (a) Sediments of the main sedimentation phases (1, 2, 3, 4). (b) Soils developed during the period between the end of each phase of sedimentation and the beginning of the following phase.
Today, Calabrian pine grows in Sicily in fragmented areas on the slopes of Mount Etna between 1000 and 2000 m a.s.l., covering approximately 3000 ha ([47], [5]). In Calabria it grows on the Sila and Aspromonte mountains, covering approximately 114 000 ha, of which more than 50% are pure stands of both natural and artificial origin. The latter originated from extensive reforestation projects carried out between 1950 and 1970 following specific State laws ([26], [27]).
The largest area covered by Calabrian pine is in the Sila mountain range, where this species characterizes the forest landscape. Together with soil conservation and watershed protection, Calabrian pine has an important role in the local forest economy. In public properties (townships and State forests), management of Calabrian pine has usually been based on various types of clear felling (strip or patch), whereas on private properties, pine stands have generally been managed according to traditional and locally developed forms of selection cutting, which have contributed to the maintenance of pure pine stands with complex structures ([13]). More recently, in State forests within the Sila National Park, management has been limited to felling only dead or dying trees. In these forests, and in other areas where for various reasons active management of Calabrian pine stands has stopped, broadleaved trees are spreading under the older pine trees ([25]). Thus, the primary environmental factors determining the occurrence and competitiveness of Calabrian pine are strongly influenced by anthropogenic activity.
In order to understand the ecological and anthropogenic factors driving Calabrian pine distribution in the Sila mountain range, we address 4 objectives: (1) to analyze present-day Calabrian pine distribution; (2) to determine forest cover changes in the period 1935-2006; (3) to characterize climate and soil conditions where Calabrian pine grows; and (4) to identify the most significant variables that have led to species’ persistence, expansion or transition in the last 70 years. We outline the potential dynamics of Calabrian pine forests for the near future in relation to changes in ecological and anthropogenic drivers. Our aim is to provide a tool for the decision-making process in forest planning, specifically where conservation of pine habitat is the main management objective.
Material and methods
Study area
The Sila mountain range (16° 20′ 9.5″ - 16° 45′ 44.4″ E, 39° 00′ 30.9″ - 39° 33′ 45.4″ N) covers an area of approximately 1302 km2 (Fig. 2), and is conventionally divided into 3 geographic zones named, from north to south, Sila Greca, Sila Grande, and Sila Piccola, respectively. The Sila Greca lies in the northern part of the mountain range, and is characterized by very steep slopes and altitudes ranging between 900 and 1650 m a.s.l. In the past, Calabrian pine was planted mostly at elevations higher than 900 m a.s.l. The Sila Grande is a vast area extending from 1100 up to 1928 m a.s.l. on Mount Botte Donato. The Sila Piccola is located in the southern part of the mountain range at altitudes between 1200 and 1765 m a.s.l. and incorporates Mount Gariglione. The Sila mountain range is characterized by wide, almost flat or slightly sloping areas, modeled in a Paleozoic substratum of igneous-metamorphic rock or in a Miocenic deposit during the Pliocene ([18], [54], [35], [40]).
Data
Present-day forest cover was obtained from Corine Land Cover 2000 map (CLC 2000). We used the fourth thematic level for maps of Italy at a nominal scale of 1:100.000, which consist of 23 forest classes ([9]). A forest map produced by the National Forest Service in 1935 at a scale of 1:100.000 ([39]) was used for land cover change analysis.
To identify the main drivers of change in land cover, we analyzed elevation, aspect, slope, climate, and soil type. Elevation data were extracted from a digital terrain model (DTM) available for Italy with a pixel size of 250 m ([6]). Aspect and slope were computed from the DTM. The climate was analyzed using monthly temperature and precipitation maps with a 250 m pixel size. Monthly data were elaborated at the national scale by using locally calibrated regressions from a database of 403 weather stations distributed across Italy with a 30-year time series ([6]). A bioclimate map for the study area was generated from monthly data ([50]). We used the historical dataset (1921-2010) of monthly and yearly precipitations from 19 weather stations distributed across the study area to provide additional information on climate conditions ([38]). Because temperature was only recorded on 5 of these weather stations, it was estimated for the remaining stations by a local regression equation between elevation and temperature ([12]). For each station, we derived climatic diagrams and the following bioclimatic indexes: thermicity index, continentality index, ombrothermic index, and compensated index ([50]).
Soil types according to the USDA soil classification system ([53]) were extracted from a soil map (1:250.000) produced by the Calabrian Regional Agency for Development and Services in Agriculture ([2]). Ancillary soil information from local studies was also used to interpret soil data ([16], [33], [34], [51]).
Forest distribution and analysis of land-cover change
Shape and classification of forest polygons extracted from the CLC 2000 map were verified by visual interpretation of digital orthophotos acquired in 2006. We discriminated between pure and mixed forests using 75% of total crown cover as the threshold and the minimum Corine Land Cover (CLC) mapping unit. After this procedure, no forest changes were detected in the study area between 2000 and 2006. We then reclassified the CLC into 9 forest classes: Calabrian pine forest (both plantations and natural stands); mixed Calabrian pine-beech forest (pure natural pine stands alternating with beech on small areas); beech forest with sparse Calabrian pine (beech forest with different sized and shaped pine groups); beech forest; mixed fir-beech forest; chestnut forest; other coniferous forest; other broadleaved forest; and non-forest. In this way, we produced a forest map for the Sila mountain range updated to 2006.
The present-day distribution of forest classes was compared with the forest distribution reported in 1935 by the National Forest Service. A cross-tabulation analysis was used to detect land cover changes ([11]). We applied a map generalization procedure to reduce the geometric and thematic discrepancies between the historical and the present-day forest maps ([46], [45]). First, a thematic generalization was applied to each forest map by merging the initial forest classes into 7 classes: Calabrian pine forest; mixed fir-beech forest; beech forest; chestnut forest; other coniferous forest; other broadleaved forest; and non-forest. Then we performed successive spatial aggregations from 50 m up to 400 m using a majority-rule aggregation procedure to identify the best pixel size for change analysis following the method of Petit & Lambin ([46]). After this procedure, a pixel size of 250 m was used for cross-tabulation analysis.
Relationship between forest classes and ecological factors
Preliminary analysis
Geographical information system tools (overlay and summary) were used to produce graphs and scatter-plots for a visual interpretation of the ranges of ecological variables. Analyses were carried out for every ecological factor with all forest classes. Graphs were created to interpret the relationships between single ecological variables and forest classes. Scatter-plots were used to analyze the bi-dimensional relationships between elevation and aspect.
Factors driving Calabrian pine distribution
To identify the explanatory variables affecting Calabrian pine distribution we used a binary logistic regression analysis. This type of statistical model is used to describe the relationship between a binary (presence-absence or 1-0) dependent variable (i.e., land cover change vs. no land cover change), and a set of continuous or categorical independent, or explanatory, variables ([28]). For instance, Poljanec et al. ([48]) used a logistic regression model to analyze changes in the distribution of European beech in Slovenia between 1975 and 2005 in terms of selected site, stand, and management variables. Mon et al. ([41]) used a logistic regression to examine the factors influencing deforestation and forest degradation in production forests of the central Bago Mountain area in Myanmar. Monzón-Alvarado et al. ([42]) employed logistic regression analysis to assess the likelihood of deforestation following forest fires in Laguna del Tigre National Park, Guatemala. Getahun et al. ([22]), Newman et al. ([44]), Schweizer & Matlack ([55]) used logistic regressions to study socio-economic factors and environmental variables driving land cover changes. In addition, several authors ([3], [1], [10]) used logistic regressions to identify the most important biophysical and human variables explaining the spatial occurrence of fire ignitions.
In our case, 3 logistic regressions were performed after analysis of land-use change to identify the most important explanatory variables for Calabrian pine persistence, expansion, and transition during 1935-2006 (Tab. 1).
Tab. 1 - Dependent and independent variables used in the binary logistic regression. (*): HD_HPD = Humic Dystrudept, and Humic Psammentic Dystrudept; HD_TD = Humic Dystrudept and Typic Dystrudept; TD_HD = Typic Dystrudept and Humic Dystrudept; HPD_HD_TD = Humic Psammentic Dystrudept, Humic Dystrudept and Typic Dystrudept; HLD_HD_RO = Humic Lithic Dystrudept, Humic Dystrudept and Rock outcrop; HPD_HD_RO = Humic Psammentic Dystrudept, Humic Dystrudept and Rock outcrop.
Role | Variables | Source | Variable type | Notes |
---|---|---|---|---|
Dependent | Calabrian pine persistence | Analysis of land-use change (this study) | Binary | 1 = yes; 0 = no |
Calabrian pine expansion | Analysis of land-use change (this study) | Binary | 1 = yes; 0 = no | |
Calabrian pine transition | Analysis of land-use change (this study) | Binary | 1 = yes; 0 = no | |
Independent | Bioclimate | Own elaboration from monthly temperature and precipitation maps ([6]) | Binary | 1 = oceanic Mediterranean; 2 = temperate oceanic |
Elevation | DTM ([6]) | Ordinal | Reclassified into 100 m intervals | |
Aspect | Own elaboration from DTM | Categorical | 1 = N; 2 = NE; 3 = E; 4 = SE; 5 = S; 6 = SW; 7 = W; 8 = NW; 9 = flat |
|
Slope | Own elaboration from DTM | Ordinal | Reclassified into 20% intervals | |
Soil type* | Soil map ([2]) | Categorical | 1 = HD_HPD; 2 = HD_TD; 3 = TD_HD; 4 = HPD_HD_TD; 5 = HLD_HD_RO; 6 = HPD_HD_RO |
The vector data format was transformed into a raster data format using a pixel size of 250 m. Then we established a systematic, 1-km sampling network over the study area, and obtained a 916-pixel sample, which reduced the effect of spatial autocorrelation in the spatial distribution of observation points ([31], [41], [55]). Only the pixels that were candidates for the type of event (persistence, expansion, or transition) were selected for the regression analysis. Specifically, in the pine-persistence model only pixels that were Calabrian pine stands in 1935 and 2006 were selected; in the pine-expansion model only pixels that were non-pine stands in 1935 and pine stands in 2006 were considered; and in the pine-transition model only pixels that were pine stands in 1935 and non-pine stands in 2006 were used. Thus, there were n = 182, n = 154, and n = 128 candidate pixels for the persistence model, the expansion model, and the transition model, respectively. A binary dependent variable was created for each model, with value 1 for candidate pixels representing the type of change under examination, and 0 for an equal number of randomly selected non-candidate pixels. The attributes of the independent variables for each candidate and non-candidate pixels were extracted by spatial analysis tools and exported to SPSS® v. 20 for statistical analysis.
High collinearity between the independent variables was verified by the variance inflation factor (VIF), and the tolerance was verified using linear regression ([41]). High collinearity occurs when tolerance is less than 0.2, or VIF is greater than 4 ([20]). High collinearity was not detected in our study.
The independent variables were included in a logistic regression using the forward stepwise algorithm (entry 0.50, removal 0.10), based on the maximum likelihood criterion used to reduce the number of independent variables to the most explicative ([3], [42], [1]). We examined Wald’s statistics to identify the relative importance of the contributions of explanatory variables ([41], [1]). The odds ratios of significant parameters (p<0.05) were used to facilitate model interpretation ([36], [52]). Odds ratios > 1 (or < 1) indicate an increased (or a decreased) likelihood of occurrence of the event ([41], [1]). The goodness-of-fit was tested using the Hosmer-Lemeshow test ([48], [10]). If the goodness-of-fit test statistic was > 0.05, we considered that there was no difference between the observed and the predicted values, indicating that the model’s estimates fit the data to an acceptable level ([23]).
Results
Forest distribution
The distribution of forest classes in the Sila mountain range are presented in Fig. 3a. At present, Calabrian pine forests (both plantations and natural stands) were found to cover 28% of the studied area, while 10% was covered by beech forests with sparse Calabrian pine (Tab. 2). Most Calabrian pine forests grew in the Sila Grande (37% of forest area); the remaining pine forests were almost equally distributed between the Sila Greca (55% of forest area) and the Sila Piccola (27% - Fig. 4).
Tab. 2 - Forest classes in the Sila mountain range.
Forest class | Sila Greca | Sila Grande | Sila Piccola | Total | ||||
---|---|---|---|---|---|---|---|---|
ha | % | ha | % | ha | % | ha | % | |
Calabrian pine forest | 7 348 | 39 | 20 722 | 26 | 8 030 | 25 | 36 100 | 28 |
Mixed Calabrian pine-beech forest |
1 495 | 8 | 14 059 | 18 | 4 668 | 15 | 20 221 | 16 |
Beech forest with sparse Calabrian pine |
791 | 4 | 7 257 | 9 | 5 125 | 16 | 13 173 | 10 |
Beech forest | 0 | 0 | 10 908 | 14 | 9 883 | 31 | 20 791 | 16 |
Mixed fir-beech forest | 0 | 0 | 0 | 0 | 1 187 | 4 | 1 187 | 1 |
Chestnut forest | 258 | 1 | 1 621 | 2 | 394 | 1 | 2 273 | 2 |
Other coniferous forest | 86 | 0 | 511 | 1 | 0 | 0 | 597 | 0 |
Other broadleaved forest | 3 357 | 18 | 788 | 1 | 217 | 1 | 4 362 | 3 |
Non-forest | 5 583 | 30 | 23 190 | 29 | 2 689 | 8 | 31 462 | 24 |
Total | 18 918 | 100 | 79 056 | 100 | 32 193 | 100 | 130 166 | 100 |
Fig. 4 - Distribution of the most important forest classes (for each class, the area is a percentage of total forest class area) into geographical zones, elevation, bioclimate, and soil (see Tab. 1 for soil type description).
Pine plantations and natural pine forests were not distinguished from each other in our study. Nevertheless, field observations and literature data show that natural pine forests grow between 800-1000 m a.s.l., and 1500-1600 m a.s.l., while plantations were located between 900 and 1200-1300 m a.s.l., as a consequence of the reforestation activities carried out from 1950 to 1970 ([26]).
Mixed Calabrian pine-beech forests and beech forests with sparse Calabrian pine were spatially adjacent to pure pine forests. These 2 forest classes reflect different beech forest dynamic stages: the former occurred where beech was partially replaced by pine due to intensive cuts in the past; the latter intermingled with pure beech forests especially in the southern, western, and central areas of the Sila mountain range. In the Sila Greca and the Sila Grande, beech forests with sparse Calabrian pine covered 6% and 13% of forest area, respectively; the area covered by this forest class in the Sila mountain range was equal to 13% of the total forest area (Tab. 2).
Pure beech forests prevailed in the southern area and in the western and central areas from 1400-1500 m a.s.l. up to the mountain top (Fig. 4). In the Sila Grande and the Sila Piccola, beech forests covered 20% and 33% of the forest area, respectively; the area covered by beech in the Sila mountain range was equal to 21% of the total forest area (Tab. 2).
The most important area for spontaneous fir vegetation was in the Sila Piccola (Tab. 2), where mixed fir-beech forests spread over 1100 ha (4% of forest area in the Sila Piccola), at elevation ranging from 1200 and 1600 m a.s.l. (Fig. 4).
Land cover changes
At present, the area covered by Calabrian pine forests and mixed Calabrian pine-beech forests is equal to 36 100 ha and 20 221 ha, respectively (Tab. 2). In 1935, the total area covered by pine was 40 888 ha. Overall, pine forest area has increased 38% between 1935 and 2006. The cross-tabulation analysis (Tab. 3) shows that most of these new pine forests (approx. 65%) occurred in the previously non-forested class because of reforestation projects after World War II ([27]). However, pine forests have also replaced other forest classes (approx. 35%), especially beech, chestnut, and other broadleaved forests. It is also worth noting that 46% of the current pine forest area was present in 1935, indicating the persistence of this forest class in the Sila mountain range (Fig. 5). Additionally, cross-tabulation analysis showed that during 1935 to 2006, parts of pine forests changed to other classes: about 46% changed into beech forest, 6% into other forest classes, and 46% were transformed into other land uses.
Tab. 3 - Forest cover changes of most important forest classes (in hectares) between 1935 (columns) and 2006 (rows).
2006 | 1935 | |||||||
---|---|---|---|---|---|---|---|---|
Calabrian pine |
Other coniferous |
Beech | Chestnut | Other broadleaved |
Mixed fir-beech |
Non-forest | Total | |
Calabrian pine | 26 006 | 0 | 4 181 | 844 | 5 738 | 0 | 19 756 | 56 525 |
Other coniferous | 19 | 0 | 0 | 0 | 56 | 0 | 525 | 600 |
Beech | 6 756 | 0 | 158 383 | 944 | 2 638 | 0 | 7 806 | 33 981 |
Chestnut | 200 | 0 | 263 | 144 | 475 | 0 | 1 213 | 2 294 |
Other broadleaved | 725 | 0 | 0 | 94 | 2 531 | 0 | 963 | 4 313 |
Mixed fir-beech | 0 | 0 | 988 | 0 | 181 | 0 | 6 | 1.175 |
Non-forest | 7 181 | 0 | 1 600 | 306 | 3 469 | 0 | 18 722 | 31 278 |
Total | 40 888 | 0 | 228 694 | 2 331 | 15 088 | 0 | 48 991 | 130 166 |
Climate
Calabrian pine forests grew in an oceanic Mediterranean bioclimate from the lower limit (800 to 900 m a.s.l.) up to 1200 m a.s.l., and in a temperate oceanic bioclimate at higher elevations. Thermotype and ombrotype for 19 weather stations in the Sila mountain range are reported in Tab. 4.
Tab. 4 - Thermotype and ombrotype for 19 weather stations distributed across the study area. (E): Elevation; (P): average yearly precipitation; (T): average yearly temperature; (It): 10(T + M + m), where M = mean of maximum temperature of the cold months, and m = mean of minimum temperature of the cold months; (Ic): Tmax - Tmin, where Tmax = mean temperature of the warm months, and Tmin = mean temperature of the cold months. (Iov3): Ppsv3 / Tpsv3, where Ppsv3 = mean precipitation in June, July and August, and Tpsv3 = mean temperature in June, July and August; (Iov4): Ppsv4 / Tpsv4, where Ppsv4 = mean precipitation in May, June, July and August, and Tpsv4 = mean temperature in May, June, July and August). (*): 1 = oceanic Mediterranean, 2 = Temperate oceanic; (**): 1 = Meso-Mediterranean higher, 2 = Supra-Mediterranean medium, 3 = Supra-Mediterranean higher, 4 = Mountain higher, 5 = Supra-Mediterranean lower, 6 = Mountain lower. (***): 1 = Humid higher, 2 = Humid lower, 3 = Iper-humid lower.
Weather station |
E (m a.s.l.) |
P (mm) |
T (°C) |
Rivas Martinez | Bioclimate* | Thermotype** | Ombrotype*** | |||
---|---|---|---|---|---|---|---|---|---|---|
It | Ic | Iov3 | Iov4 | |||||||
Parenti | 830 | 1394 | 11.9 | 196.9 | 17.7 | 1.3 | - | 1 | 1 | 1 |
Pinutello C.C. | 1005 | 1136 | 11.1 | 162.4 | 17.9 | 1.7 | - | 1 | 2 | 2 |
S. Giovanni in Fiore | 1050 | 1153 | 10.7 | 151.3 | 17.9 | 1.7 | - | 1 | 2 | 2 |
Cecita (ex Acquacalda) | 1180 | 1083 | 8.9 | 113.4 | 17.0 | 1.6 | - | 1 | 3 | 2 |
Stratalati C.C. | 1200 | 1324 | 9.7 | 118.6 | 18.0 | 1.2 | - | 1 | 3 | 1 |
Savuto C.C. | 1205 | 1355 | 9.7 | 117.5 | 18.0 | 1.3 | - | 1 | 3 | 1 |
Lorica | 1290 | 1229 | 9.1 | 98.4 | 18.1 | 2.0 | 2.5 | 2 | 4 | 1 |
Camigliatello Silano | 1291 | 1631 | 9.3 | 100.5 | 18.1 | 2.0 | 2.9 | 2 | 4 | 3 |
Quaresima C.C. | 1300 | 1577 | 9.0 | 96.2 | 18.1 | 2.0 | 3.3 | 2 | 4 | 3 |
Nocelle | 1322 | 1205 | 8.9 | 91.3 | 18.1 | 2.0 | 2.6 | 2 | 4 | 1 |
Longobucco | 770 | 1258 | 12.8 | 215.1 | 17.7 | 1.3 | - | 1 | 1 | 2 |
Bocchigliero | 870 | 1257 | 12.1 | 192.6 | 17.7 | 1.3 | - | 1 | 5 | 2 |
Fiorenza | 1126 | 1272 | 10.3 | 135.2 | 18.0 | 2.1 | - | 2 | 6 | 2 |
Casa Pasquale | 1246 | 1398 | 9.4 | 108.3 | 18.1 | 1.8 | 2.3 | 2 | 4 | 1 |
Casa Jolanda | 1250 | 1615 | 9.4 | 107.4 | 18.1 | 2.2 | - | 2 | 4 | 3 |
Barberano C.C. | 1280 | 1346 | 9.2 | 100.7 | 18.1 | 2.2 | - | 2 | 4 | 1 |
Trepidò | 1295 | 1305 | 9.0 | 97.3 | 18.1 | 1.8 | 2.3 | 2 | 4 | 1 |
Vivoli C.C. | 1300 | 1311 | 9.0 | 96.2 | 18.1 | 1.8 | 2.9 | 2 | 4 | 1 |
Sculca | 1358 | 1318 | 8.6 | 83.2 | 18.1 | 1.8 | 2.8 | 2 | 4 | 1 |
The average yearly precipitation exceeded 1000 mm with an increasing trend from the northern and eastern areas to the southern area of the Sila mountain range. The maximum precipitation was 1631 mm. The mean monthly precipitation between October and April exceeded 100 mm; most precipitation (>70-75%) occurred between autumn and spring (Fig. 6). Snow stayed on the ground for 6 months at elevations > 1400 to 1600 m a.s.l., especially in cool aspects ([38]).
Fig. 6 - Climate diagrams of 6 weather stations: Cecita, Longobucco, San Giovanni in Fiore, Camigliatello Silano, Vivoli, and Trepidò.
The mean temperature ranged between 12.8 and 8.6 °C. The mean temperature at the lower (900 m a.s.l.) and higher limits (1650 m a.s.l.) of pine growth were 11.9 and 6.5 °C, respectively. The mean monthly temperature between January and March varied between -2.6 and 0 °C, while the mean monthly temperature in July and August ranged from 16 to 18 °C. Between November and April, the daily temperature reached a minimum value of -10 °C, while in summer reached a maximum of 30 to 32°C.
Soil
Pine forests grew on different soils (Fig. 3b) depending on parent material, slope, and human impact. In the northern and eastern zones, pine forests were found on steep slopes on soils from altered granitic rocks of the following soil types (USDA soil classification system): Humic Psammentic Dystrudept, Humic Dystrudept and Rock outcrop (HPD_HD_RO). The HPD were thin soils with an A-Cr profile, a scarce skeleton with a coarse texture, acidic, a low water reserve, and fast drainage. The HD_RO were moderately deep soils with an Oi-A-Bw-Cr profile, a skeleton varying from scarce to common with moderately coarse texture, acidic, a high water reserve, and good drainage ([2]). These soil types were also present in some areas of the northern and eastern zones.
In the central zone, Calabrian pine was found on soils originated on moderate slopes from altered granitic rocks of the following soil type: Humic Psammentic Dystrudept, Humic Dystrudept, and Typic Dystrudept (HPD_HD_TD). The 2 most common profiles were: (i) Oi-A-Bw-Cr, moderately deep with a skeleton varying from scarce to common; and (ii) Oi-A-Bw-BC-Cr, moderately deep with a common skeleton, a medium coarse texture, acidic, a moderate water reserve, and good drainage ([2]).
In the southern zone, Calabrian pine grew on slight slopes on soils originated from metamorphic rocks: Typic Dystrudept and Humic Dystrudept (TD_HD); and Humic Lithic Dystrudept, Humic Dystrudept, and Rock outcrop (HLD_HD_RO). In the TD_HD soils, the most common profiles were: (i) A-Bw-C, moderately deep, with frequent skeleton, high stoniness, medium texture, acid to sub-acid, moderate water reserve, and good drainage; (ii) Oi-A-Bw-BC, moderately deep, with common skeleton, medium texture, acidic, water reserve from moderate to high, and good drainage. The HLD_HD_RO had very thin soils with an A-R profile, frequent skeleton, coarse texture, acidic, very low water reserve, and fast drainage ([2]).
Correlation between forest classes and ecological variables
The relationships between each ecological variable (geographic zones, elevation classes, bioclimate, and soil types) and forest classes are shown in Fig. 4. Scatter-plots reported in Fig. 7 depict the relationships between elevation and aspect: above 1600 m a.s.l., Calabrian pine forests were located mainly on warm aspects, whereas below 800 m a.s.l., mixed Calabrian pine-beech forests and beech forests with sparse Calabrian pine were present mainly in cool aspects at all elevations. Mixed fir-beech forests were present only in cool aspects.
Fig. 7 - Scatter-plots depicting the relationships between elevation in m above sea level (m a.s.l.) and aspect (degrees): (a) Calabrian pine forest, (b) Mixed Calabrian pine-beech forest, (c) Beech forest with sparse Calabrian pine, (d) Beech forest, and (e) Mixed fir-beech forest.
The logistic regression analysis shows that among the 5 independent variables describing the study area, bioclimate, elevation, and soil type were the variables best explaining the persistence of Calabrian pine. The Hosmer-Lemeshow test revealed that the model accounted for significant portion of variance for the pine persistence in 1935-2006 (Tab. 5). All explanatory variables were significant (p<0.05) except the soil type HPD_HD_TD, with an odds ratio < 1, indicating that the probability of persistence of pine decreased from the oceanic Mediterranean bioclimate to higher elevations, in soil types HD_HPD, HD_TD, TD_HD, and HLD_HD_RO. Based on the Wald’s values, the most important factor for the probability of pine-persistence was elevation, followed by bioclimate and soil type HLD_HD_RO.
Tab. 5 - Results of the binary logistic regression (n = 364) explaining pine-persistence during 1935-2006 (see Tab. 1 for independent variable description). (B): coefficient; (SE): standard error; (Wald): Wald’s statistics; Exp (B): exponential of B coefficient.
Independent variable |
B | SE | Wald | p-value | Exp (B) or Odds ratio |
---|---|---|---|---|---|
Constant | 7.568 | 1.233 | 37.699 | 0.000 | 1934.750 |
Bioclimate (1) | -2.322 | 0.475 | 23.888 | 0.000 | 0.098 |
Elevation | -0.469 | 0.085 | 30.438 | 0.000 | 0.625 |
Soil (HD_HPD) | -0.977 | 0.449 | 4.745 | 0.029 | 0.376 |
Soil (HD_TD) | -2.267 | 0.647 | 12.275 | 0.000 | 0.104 |
Soil (TD_HD) | -1.543 | 0.360 | 18.328 | 0.000 | 0.214 |
Soil (HPD_HD_TD) | -0.235 | 0.345 | 0.464 | 0.496 | 0.790 |
Soil (HLD_HD_RO) | -1.658 | 0.361 | 21.073 | 0.000 | 0.190 |
Hosmer-Lemeshow test | 0.595 | - | - | - | - |
For Calabrian pine expansion, the logistic regression indicates that the most important predictors were elevation and soil type. The Hosmer-Lemeshow test shows that the model fit was acceptable (Tab. 6). All explanatory variables were significant (p<0.05). An odds ratio < 1 for elevation indicates that the greater the altitude the less probability of expansion of pine; and an odds ratio > 1 for soil type indicates that the likelihood of expansion increased in the soils HD_HPD, HD_TD, TD_HD, HPD_HD_TD, and HLD_HD_RO. Based on Wald’s statistics, the most important factor for the likelihood of expansion was elevation, followed by soil type TD_HD.
Tab. 6 - Results of the binary logistic regression (n = 308) explaining pine-expansion during 1935-2006 (see Tab. 1 for independent variable description). (B): coefficient; (SE): standard error; (Wald): Wald’s statistics; Exp (B): exponential of B coefficient.
Independent variable | B | SE | Wald | p-value | Exp (B) or Odds ratio |
---|---|---|---|---|---|
Constant | 7.110 | 1.104 | 41.464 | 0.000 | 1224.098 |
Elevation | -0.644 | 0.089 | 52.812 | 0.000 | 0.525 |
Soil (HD_HPD) | 2.529 | 0.661 | 14.627 | 0.000 | 12.535 |
Soil (HD_TD) | 2.107 | 0.636 | 10.955 | 0.001 | 8.221 |
Soil (TD_HD) | 2.293 | 0.471 | 23.662 | 0.000 | 9.906 |
Soil (HPD_HD_TD) | 1.303 | 0.491 | 7.046 | 0.008 | 3.681 |
Soil (HLD_HD_RO) | 1.388 | 0.468 | 8.785 | 0.003 | 4.006 |
Hosmer-Lemeshow test | 0.908 | - | - | - | - |
For Calabrian pine transition, only elevation (p<0.05) was included in the model with an odds ratio > 1, i.e., the probability of transition increased with altitude. However, in this case the Hosmer-Lemeshow test indicates that the model did not fit the data adequately (Tab. 7).
Tab. 7 - Results of the binary logistic regression (n = 256) explaining pine-transition during 1935-2006 (see Tab. 1 for independent variable description). (B): coefficient; (SE): standard error; (Wald): Wald’s statistics; Exp (B): exponential of B coefficient.
Independent variable | B | SE | Wald | p-value | Exp (B) or Odds ratio |
---|---|---|---|---|---|
Constant | -4.096 | 1.054 | 15.101 | 0.000 | 0.017 |
Elevation | 0.289 | 0.074 | 15.387 | 0.000 | 1.335 |
Hosmer-Lemeshow test | 0.000 | - | - | - | - |
Discussion and conclusions
Despite forest changes between 1935 and 2006, Calabrian pine covers today extensive areas in the Sila mountain range. Our results show that the most important ecological drivers of Calabrian pine distribution are elevation, soil type and bioclimate. The probability of pine-persistence during the period 1935-2006 is significantly correlated with all of these explanatory variables. As shown in Fig. 8, pine-persistence prevails below 1400 m a.s.l., in a temperate oceanic bioclimate on thin and very eroded soils with low water-holding capacity (HPD_HD_RO), or on moderately deep soils with high water content and good drainage (HPD_HD_TD). Above 1600 m a.s.l., depending on aspect and climatic restrictions Calabrian pine is rare (Fig. 7).
Fig. 8 - Calabrian pine changes during 1935-2006 (for each type of change, the area is a percentage of the total change) in relation to elevation, bioclimate, and soil (see Tab. 1 for soil type description).
The likelihood of pine-expansion is significantly correlated with elevation and soil type. In the last 70 years, pine-expansion occurred especially below 1400 m a.s.l., on thin and very eroded soils with low water-holding capacity (HLD_HD_RO), and on moderately deep soils with good drainage, and water-holding capacity from moderate (TD_HD) to high (HPD_HD_TD - Fig. 8). Below 1400 m a.s.l., reforestation has been the main cause of Calabrian pine expansion ([26], [27]).
Pine transition seems related to elevation, though our model prediction for transition did not adequately fit the data. Nonetheless, our results shows that transition prevails above 1200 m a.s.l. in moderately deep soils with high water content (HPD_HD_TD).
In the Sila mountain range there is historical evidence that intense soil erosion following forest destruction for agriculture and grazing started between the first and second millennium BC, with the Bruzi occupation ([17]). Thus, Calabrian pine spreading, which was initiated in that period, can be associated to human-induced soil degradation. The presence of pine stands in many beech dominated forests, and the presence of mixed fir-beech forests is probably an example of the forest type characterizing the Sila mountain range before the destruction performed first by the Bruzi and then by the Romans. Beech forests with sparse Calabrian pine and pure pine forests can be considered as a simplified form of the mixed forest due to human impact: indeed, where human pressure was stronger (large clear cuts in beech forests and repeated fires) pine replaced beech, and then pine was favored by the silvicultural systems used to manage pine forests (such as clear felling).
Based on our results, 4 potential dynamics can be outlined for Calabrian pine forests in the Sila mountain range. These dynamics are driven by ecological (elevation, bioclimate, and soil) and anthropogenic factors.
Below 1200 m a.s.l., in an oceanic Mediterranean bioclimate:
- (A) In thin and very eroded soils, with coarse texture, fast drainage, low cation-exchange capacity, and low water-holding capacity (HPD_HD_RO), pure Calabrian pine forests grow at the lower limit of their distribution. Under these conditions, Calabrian pine is expected to dominate also in the future following both management or disturbance factors such as fire (Fig. 9a).
- (B) In moderately deep soils with a scarce to common skeleton, moderately coarse texture, acid pH, high water content, and good drainage (HPD_HD_TD), pure Calabrian pine forests may be maintained by forest management methods such as clear felling or the small group selection method described by Ciancio et al. ([13]). Absence of management will favor instead the evolution towards pure or mixed broadleaved forests dominated by oaks. However, disturbances like wildfire might affect this forest dynamic, favoring natural pine regeneration and thus perpetuating the pine forest (Fig. 9b).
Fig. 9 - Potential Calabrian pine forest dynamics according to ecological (bioclimate, elevation, and soil), and anthropogenic (forest fire and forest management) drivers in the Sila mountain range: (a) pure Calabrian pine forest naturally originated: (b) Calabrian pine forest that might evolve into pure or mixed broadleaved forest dominated by oaks; (c) Calabrian pine plantations; and (d) Calabrian pine forest naturally originated that can be either maintained or transformed in beech dominated forest or in mixed fir-beech forest, according to forest management methods (see Tab. 1 for soil type description).
Above 1200 m a.s.l., in a temperate oceanic bioclimate:
- (C) In thin and very eroded soils, with coarse texture, fast drainage, low cation-exchange capacity, and low water-holding capacity (HLD_HD_RO), pure and natural Calabrian pine forests curently prevail (Fig. 10a), and will probably be prevailing also in the future following either management or fire occurrence (Fig. 9c).
- (D) In moderately deep soils with common skeleton and a superficial stoniness, medium texture, acid to sub-acid pH, moderate water-holding capacity and good drainage (TD_HD), pure Calabrian pine forests can be maintained by appropriate forest management methods ([13]), or by disturbance factors such as fire. Absence of management will instead favor the evolution towards beech dominated forests or mixed fir-beech forests (Fig. 9d).
Fig. 10 - (A) Pure naturally originated Calabrian pine forest; (B) beech regeneration in a Calabrian pine forest (Photo: F. Iovino).
Forest dynamics corresponding to (A) and (B) are expected for pine forests growing both in the northern and eastern sectors of the Sila mountain range (Fig. 11). In these sectors, extensive reforestation with pines was carried out in the past, while pine regeneration was favored by repeated fires. Forest dynamics corresponding to (C) and (D) prevail in the southern and western sectors of the Sila mountain range (Fig. 11), where pine forests are strongly influenced by management, or conversely by its absence. However, the tendency to evolve towards beech-dominated stands is very strong. Indeed, beech regeneration is quickly replacing pine where pine forests have not been managed in the last 40 to 50 years (Fig. 10b). In such cases, appropriate silvicultural systems must be used to favor pine regeneration for conservation purposes.
Fig. 11 - Potential Calabrian pine forest dynamics (including mixed Calabrian pine-beech forest) according to ecological (bioclimate, elevation and soil), and anthropogenic (forest fire and forest management) drivers in the Sila mountain range (see also Fig. 9).
In conclusion, identifying potential Calabrian pine dynamics on the basis of ecological and anthropogenic factors can help forest managers define priorities for forest planning, such as landscape and habitat conservation, and management goals for pine plantations in a forest-priority habitat of community importance. Furthermore, our results may help understanding the possible response and adaptation potential of Calabrian pine to changes both in management and in environmental factors, such as expected climate change.
Acknowledgments
We thank four anonymous reviewers for their helpful suggestions and comments on an early version of the manuscript.
References
Gscholar
Gscholar
Gscholar
Gscholar
Gscholar
Gscholar
Gscholar
Gscholar
Gscholar
Gscholar
Gscholar
Gscholar
Gscholar
Gscholar
Gscholar
Gscholar
Gscholar
Gscholar
Gscholar
Gscholar
Gscholar
Gscholar
CrossRef | Gscholar
Gscholar
Authors’ Info
Authors’ Affiliation
Francesco Iovino
Dipartimento di Ingegneria Informatica, Modellistica, Elettronica e Sistemistica, Università della Calabria, Arcavacata di Rende, Cosenza (Italy)
Susanna Nocentini
Dipartimento di Gestione di Sistemi Agrari, Alimentari e Forestali, Università degli Studi di Firenze, Firenze (Italy)
Dipartimento Gestione dei Sistemi Agrari e Forestali, Università degli Studi Mediterranea di Reggio Calabria, Reggio Calabria (Italy)
Istituto per i Sistemi Agricoli e Forestali del Mediterraneo, Consiglio Nazionale delle Ricerche, Rende, Cosenza (Italy)
Corresponding author
Paper Info
Citation
Nicolaci A, Travaglini D, Menguzzato G, Nocentini S, Veltri A, Iovino F (2014). Ecological and anthropogenic drivers of Calabrian pine (Pinus nigra J.F. Arn. ssp. laricio (Poiret) Maire) distribution in the Sila mountain range. iForest 8: 497-508. - doi: 10.3832/ifor1041-007
Academic Editor
Renzo Motta
Paper history
Received: May 20, 2013
Accepted: Jul 19, 2014
First online: Nov 10, 2014
Publication Date: Aug 02, 2015
Publication Time: 3.80 months
Copyright Information
© SISEF - The Italian Society of Silviculture and Forest Ecology 2014
Open Access
This article is distributed under the terms of the Creative Commons Attribution-Non Commercial 4.0 International (https://creativecommons.org/licenses/by-nc/4.0/), which permits unrestricted use, distribution, and reproduction in any medium, provided you give appropriate credit to the original author(s) and the source, provide a link to the Creative Commons license, and indicate if changes were made.
Web Metrics
Breakdown by View Type
Article Usage
Total Article Views: 52257
(from publication date up to now)
Breakdown by View Type
HTML Page Views: 44871
Abstract Page Views: 2523
PDF Downloads: 3709
Citation/Reference Downloads: 24
XML Downloads: 1130
Web Metrics
Days since publication: 3734
Overall contacts: 52257
Avg. contacts per week: 97.96
Article Citations
Article citations are based on data periodically collected from the Clarivate Web of Science web site
(last update: Feb 2023)
Total number of cites (since 2015): 17
Average cites per year: 1.89
Publication Metrics
by Dimensions ©
Articles citing this article
List of the papers citing this article based on CrossRef Cited-by.
Related Contents
iForest Similar Articles
Review Papers
Large-scale effects of forest management in Mediterranean landscapes of Europe
vol. 6, pp. 342-346 (online: 29 August 2013)
Short Communications
The Polish landscape changing due to forest policy and forest management
vol. 2, pp. 140-142 (online: 30 July 2009)
Research Articles
Future land use and food security scenarios for the Guyuan district of remote western China
vol. 7, pp. 372-384 (online: 19 May 2014)
Research Articles
Historical fire ecology and its effect on vegetation dynamics of the Lagunas de Montebello National Park, Chiapas, México
vol. 14, pp. 548-559 (online: 01 December 2021)
Research Articles
Influence of site conditions and land management on Quercus suber L. population dynamics in the southern Iberian Peninsula
vol. 15, pp. 77-84 (online: 14 March 2022)
Research Articles
Is cork oak (Quercus suber L.) woodland loss driven by eucalyptus plantation? A case-study in southwestern Portugal
vol. 7, pp. 193-203 (online: 17 February 2014)
Editorials
Adaptation of forest landscape to environmental changes
vol. 2, pp. 127 (online: 30 July 2009)
Research Articles
Evaluation of urban forest landscape health: a case study of the Nanguo Peach Garden, China
vol. 13, pp. 175-184 (online: 02 May 2020)
Research Articles
Communicating spatial planning decisions at the landscape and farm level with landscape visualization
vol. 7, pp. 434-442 (online: 19 May 2014)
Research Articles
Scale dependency of the effects of landscape structure and stand age on species richness and aboveground biomass of tropical dry forests
vol. 16, pp. 234-242 (online: 23 August 2023)
iForest Database Search
Search By Author
Search By Keyword
Google Scholar Search
Citing Articles
Search By Author
Search By Keywords
PubMed Search
Search By Author
Search By Keyword