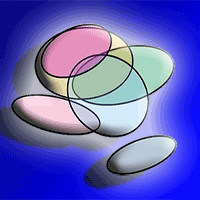
Development and evaluation of generalized fuel models for predicting fire behaviour in northern European heathlands
iForest - Biogeosciences and Forestry, Volume 17, Issue 2, Pages 109-119 (2024)
doi: https://doi.org/10.3832/ifor4394-017
Published: Apr 30, 2024 - Copyright © 2024 SISEF
Research Articles
Abstract
Northern European heathlands and moorlands dominated by Calluna vulgaris are internationally recognized for their conservation importance while also supporting traditional, low-intensity agriculture and game hunting. Managed burning plays an important role in maintaining these ecosystems but climate and land-use changes, including planned or unplanned transitions to forest and woodland, are now resulting in concerns about increasing wildfire frequency, intensity and severity. In combination with rapidly-changing regulations surrounding managed burning, this has highlighted the need to understand current and potential future fuel structures to effectively model fire behaviour and develop evidence-based regulations surrounding managed burning. We developed standardized heathland fuel descriptions and modeled associated fire behaviour for heathlands in the UK (England, Scotland) and Norway. Utilizing existing fuel and biomass data, we used cluster analysis to identify five distinct fuel models and assessed how they were represented across C. vulgaris life-stages, geographic locations and EUNIS habitat-types. We validated their independence by examining predicted fire rates of spread based across three representative fire weather scenarios. Fire rates of spread differed between C. vulgaris life stages, regardless of EUNIS community or country. Mature stage and taller building stage fuels produced the highest fire rates of spread and early, shorter building and pioneer stage fuels produced the lowest. Moss and litter fuel loads proved to be important determinants of fire rate of spread in a high-risk fire weather scenario. An understanding of links between fuel types and potential fire behaviour can be used to inform management and policy decisions. To aid in this, we used classification tree analysis to link fuel types to easily-observable characteristics. This will facilitate pairing the fuel models with fire behaviour prediction software to make evidence-based assessments of management fire safety and wildfire risk.
Keywords
Calluna vulgaris, Fuelbed, Managed Burning, Mire, Rate Of Spread, Rothermel, Wildfire, Peatland
Introduction
Fire is a fundamental disturbance process in many ecosystems around the world, including both naturally occurring wildfires and intentional managed burning ([57]). Managed and cultural fire, as well as wildfires, can be vital in sustaining many globally important fire-dependent ecosystems ([70]). Nevertheless, in the context of global climate change and with concern about the increasing frequency and severity of vegetation fires, it is vital we understand and forecast the behaviour and impact of both wild and managed fires ([23]). Evaluating how traditional forms of management affect fuel and landscape flammability and thus wildfire risk is a critical piece of this puzzle ([26]) with the development of fuel classification systems ([10]) also being vital.
In sub-boreal northern regions, including the peatlands and heathlands (hereafter collectively referred to as heathlands) of north-west Europe, there is growing concern about the effects of wildfires and increasing attention on the need for effective fire management policy and practice ([17], [35], [31]). Peat soils, including those found within the Calluna vulgaris (hereafter Calluna) - dominated heathlands of the UK and Norway, contain roughly 25% of Earth’s terrestrial carbon stock despite only covering 2-3% of its surface ([58]). Where heathland fires are severe, and especially where their peat soils are ignited, significant amounts of carbon can be released via burning ([16]). European heathlands are also of global, regional and national conservation significance ([62], [49]) and hold significant economic and cultural value associated with traditional agriculture and/or wild game hunting.
Traditional management on Calluna-dominated heathlands typically aims to burn small patches, mostly dominated by common heather, to create the mosaic of vegetation structures favoured by red grouse and/or to improve the quality of forage for sheep and deer ([20]). Such practices formed an important component of heathland land-use systems throughout northern Europe but have declined in many regions. Even in the UK, where burning remains common due to the economic importance of grouse shooting, there has been growing debate over whether it should be discontinued on deep peat soils due to concerns about the risk of management fire escapes ([4]), effects on water quality and carbon storage ([35]) and a growing interest in “rewildling” ([60]). Meanwhile, in other countries with similar vegetation types, such as Norway, increasing attention is being paid to the reintroduction of traditional fire use for both biodiversity and wildfire management goals ([48], [5], [26], [68]). Many Norwegian heathlands, for example, which were historically managed with both grazing and fire, have experienced a build-up of fuel due to a lack of management ([42]). This, in addition to drought events in recent summers and winters due to climate change ([53], [36]), has increased the risk for landscape-altering wildfire ([43]), necessitating attention to fuel management and landscape resilience ([69]). Such issues are likely to emerge throughout European heathlands ecosystems if grazing and managed fire continue to decline or are suppressed by policy changes ([20]).
Land managers, conservationists and policy makers need to be able to model both prescribed and wild fire behaviour to understand their potential impacts on above- and belowground fuel loads and carbon storage in the context of climatic and management change. In particular, there is a need to understand under what circumstances management fires might increase vs. decrease the risk of landscape wildfires. Doing so requires the generation of fuel type descriptions for use in fire modeling software and to simplify the number of different fuel structures that need to be represented in risk analyses ([9]). Variation in vegetation composition, productivity and biomass in northern European heathlands are broadly understood and align across environmental and successional gradients. Latitudinal gradients are broadly represented by lower biomass at northern sites as compared to their southern counterparts due to varying climatic and edaphic constraints on productivity ([25]). Successional gradients are typically described by the heathland management cycle ([20] - see Fig. S1 in Supplementary material) that transitions through a series of recognized Calluna structural stages ([30]) from young (pioneer) to old (degenerate), a process requiring 25 years or more. Later, unmanaged stands may transition to woodlands of Betula spp., Juniperus spp., Salix spp., Sorbus spp. and others ([46], [66], [31]) but these may retain an abundant Calluna understory for multiple decades.
Despite existing understanding of the ecological and structural variation in heathland vegetation types, researchers and managers currently lack fuel type descriptions that could be used to inform management and fire risk assessment. A number of tools are available to inform the latter, including systems based upon the Rothermel model ([3]). The Rothermel model has been applied in many other ecosystems around the world ([22], [64], [11]) and was recently demonstrated to perform tolerably well for predicting fire rate of spread in Calluna-dominated heathland fuels ([45]). Therefore, our overall aim was to reveal new knowledge of variation in Calluna-dominated heathland fuel structures and potential fire behaviour in the heathlands of north-west Europe. Specifically, we sought to: (i) generate new heathland fuel bed descriptions suitable for use with existing fire modeling software; (ii) evaluate how fuel characteristics and fuel bed types vary regionally and in relation to environmental and Calluna stand-age gradients; and (iii) assess how modeled fire behaviour differs across fuel bed types. Addressing these critical issues will provide managers and policy makers with the tools and knowledge to make efficient quantitative assessments of fire hazard on the ground.
Methodology
Data were compiled from several experiments, including projects covering four coastal dry heathlands in western Norway, seventeen sites spanning forest understories, heathlands, and blanket mires in Scotland and four heathland and blanket mire sites in England. The dataset captures dwarf-shrub (Calluna) - dominated heathland stands growing under a range of environmental conditions and management regimes and at a variety of times since fire (Tab. S1 in Supplementary material). All fuels information used resulted from destructive harvesting, though sampling frame size and shape varied between experiments (Tab. S1). Collected material was manually harvested either to the top of the peat or O-layer, or to the top of the layer of moss and litter layer that underlies most Calluna stands. Where the latter sampling method was followed moss and litter layer biomass was estimated based on the relationship between moss layer depth and mass established by Davies et al. ([13]). Vegetation samples were returned to the lab following harvesting where they were separated into various components and weighed following drying at 80 °C for 48 hours (Scottish and English samples) or 65 °C for 48 hours (Norwegian samples). The specific components that fuel samples were separated into differed depending on the original project for which they were collected.
To facilitate different analyses the data were systematically restructured (Fig. S2) to two sets of consistent fuel characteristics. The first was based on common characteristics: live woody (kg m-2), moss and litter (kg m-2), dead wood (kg m-2), and herbaceous (kg m-2) biomass, as well as canopy bulk density (kg m-3) and fuel bed depth (m). All collected woody fuels were < 25 mm in diameter. Data were then further simplified to the parameters required for development of fuel bed models and use with the Rothermel model ([59]) and its derived software tools ([27], [28], [2], [64]). Heathland fire risk and prescribed burning activity is greatest during the winter dormant season due to reduced shrub live fuel moisture contents accumulation of senesced herbaceous fuel and regulations that limit burning to between October and April ([19]). We therefore chose to focus our analysis on this period. Thus, for Rothermel analyses, all herbaceous fuels were combined with dead wood to create 1-hour fuels as most herbaceous fuels are dead during the winter and early spring. To understand the association between broad plant community structure and fuel characteristics, observations were assigned to EUNIS habitat classifications ([12]) with some heathlands further separated according to the British NVC system ([24]). Calluna stand stages describe recognizable age-related phases in the structural development of heathland vegetation and are often used by land-managers and ecologists as the basis for describing the ecological status of an area of vegetation (Fig. S1 in Supplementary material). Thus, successional changes with time since fire were described by assigning observations to stages of the Calluna cycle (as per [30]).
Evaluating patterns in fuel structure and fire behaviour
All statistical analyses were completed using R ver. 3.6.3 ([55]). Fuel types based on the common characteristics dataset (Fig. S2 in Supplementary material) were identified via hierarchical agglomerative cluster analysis and with the data standardized using variable maxima to ensure equal weight in analysis. Cluster analysis was completed using the “hclust” function with the Bray-Curtis Dissimilarity Index and Ward’s Linkage Method. Scree plots were then used to identify the appropriate number of final groups. We tested for significant differences in overall fuel structure between our identified fuel groups, as well as for differences associated with successional stage and broad plant community composition. Tests were completed using Permutational Multivariate Analysis of Variance (PERMANOVA - [1]). We utilized the “adonis2” function from the “vegan” package ([51]) to examine the effects of fuel cluster, Calluna stage and EUNIS community. The PERMANOVA was run on the standardized fuel structure data and using the Bray-Curtis dissimilarity index. We utilized 999 iterations and performed a marginal (i.e., “Type II”) test of the factors’ significance. We then performed pairwise PERMANOVA comparisons for any significant factors (“pairwise.perm.manova” function in the “RVAideMemoire” R package - [37]).
We subsequently sought to evaluate links between patterns in fuel structure and variability in potential fire behaviour within and between identified fuel clusters. We achieved this by visualizing the general positions of the fuel clusters, EUNIS communities and stages of the Calluna cycle within multivariate ordinations of fuel structure over which we also represented variation in potential fire behaviour. Visualizations were completed for three different scenarios representing high, medium and low fire risk. Part of this scenario development included assigning variable amounts of an observation’s moss/litter load to the 1-hour fuel category. While mosses can contribute to 1-hour fuel loadings ([19]), they often have very high fuel moisture contents and frequently see limited consumption except under the most severe conditions ([18]). This is particularly the case during times of the year when traditional management burning is completed and wildfire activity tends to peak (October - April). Fuel models for the high, medium, and low-risk scenarios were thus respectively assigned additional 1-hour fuels based on the 90th, 50th or 10th percentiles of moss/litter load for all observations in the associated, previously determined fuel cluster. When making such assignments we did not, however, allow the moss/litter load attributed to the 1-hour fuels to exceed the total moss/litter load recorded for a given observation. This method is conceptually similar to the principle employed in dynamic Rothermel fuel models in BehavePlus and Farsite ([3]), where proportionally more live herbaceous fuels are allocated to 1-hour fine dead fuels with increased herbaceous curing (reduced live herbaceous fuel moisture content). Increases in moss/litter fuel load will also be reflected in changes in fuelbed depth. We therefore used the empirical model linking moss/litter load and layer depth in Davies et al. ([13]) to convert the amount of added fuel to an equivalent depth and added this to the fuel bed depth of each fuel entry. We acknowledge that presently we do not have empirical evidence of the maximum extent to which layers of moss/litter contribute to flaming combustion and the spread of the fire front. The amounts we applied to the 1-hour fuels were based on existing evidence that dry beds of pleurocarpous mosses are highly flammable ([50], [65]) and qualitative observations during experimental fires and following wildfires. Our “high risk” scenario thus represents a worst-case scenario for fire behaviour based on a nearly fully dry moss/litter bed. The fuel scenario development process resulted in three separate fuel structure datasets, one for each of the scenarios. A separate NMDS was run on each of these datasets. The fuel structure data was standardized by variable maxima prior to analysis and we utilized the Bray-Curtis dissimilarity matrix to produce three-dimensional solutions. We used the “ordiellipse” function in the “vegan” R package to describe the general positions of the differing fuel clusters, Calluna stages and EUNIS communities within the ordinations. Rate of spread predictions were generated using the “ros” function in the “Rothermel” R package ([64]). To achieve this, we converted the fuel structure data for each observation into a “static” Rothermel fuel model (Fig. S2 in Supplementary material). Live woody fuel moisture content (FMC) and dead FMC were set at 60% and 15% for the high-risk scenario, 75% and 20% for the medium-risk scenario and 90% and 25% for the low-risk scenario. FMC values were selected based on values observed during experimental heathland fires ([14]) and to avoid non-starts (i.e., low rate of spread predictions skewed toward 0 m s-1). They do not to represent the full range of potential FMCs across heathlands ([15]). We set slopes to a constant 0% and windspeeds to a constant 5 m s-1 for all fire behaviour simulations to facilitate FMC comparisons away from the potential impacts of topography and variable wind. Other fuel physical and chemical characteristics (e.g., Heat of Combustion, Surface Area-to-Volume ratio) required by the Rothermel model were based on previously published data for heathland fuels ([40] - see also Tab. S2 in Supplementary material).
Testing the behaviour of generalized fuel models
Generalized fuel models were created by averaging characteristics within each of the fuel clusters previously identified. Potential fire rates of spread associated with the generalized fuel models were assessed using a representative range of variation in FMCs and wind speeds observed during heathland experimental fires in the UK ([14]). Specifically, five steps of live woody and dead FMC, respectively ranging from 60-88% (7% increments) and 15-23% (2% increments), were combined with ten steps of wind speed, ranging from 0-9 km hr-1 (1 km hr-1 increments) to create a suite of fifty fire weather scenarios. These fire weather scenarios were intended as a controlled investigation into the effects of changing FMC and windspeed, thus were selected to ensure that all fires started and their inputs could be compared effectively rather than using higher FMC values that were noted in modeling to produce non-starts. Rate of spread was predicted again using the “ros” function in the “Rothermel” R package. This variation in fire weather also allowed us to compare Rothermel fire behaviour predictions using a linear model, with the “lm” function in R. Predicted rate of spread was evaluated as a function of fuel model, wind speed, fuel moisture and their interactions. Statistical differences in fire behaviour between fuel models were then assessed using least-square-means (LSM), with the “lsmeans” function in the “lsmeans” R package ([41]). Fuel models that did not exhibit significant differences in fire behaviour were combined.
The final suite of generalized fuel models was evaluated using Classification and Regression Tree (CART) analysis with the aim of providing a fuel classification guide based on readily observable characteristics. To perform this, a random 20% of observations from the common characteristics data set were retained as a validation dataset, while the other 80% were analyzed using a recursive partitioning model with the “rpart” function in the “rpart” R package ([61]). This model included the effects of region (Scotland, Norway or England), EUNIS habitat, Calluna stage, moss layer depth (predicted from layer load where necessary) and fuel bed depth. A final predictive model was then generated for the partitioning model with the “predict” function in the “car” R package ([29]) using the validation dataset, following which the decision tree was pruned with the “train” function in the “caret” R package ([39]) using a train control of 10 and a tune length of 10.
To validate this final suite of fuel models, we utilized our decision tree to assign them to each of 27 experimental heathland burns ([14]) and for which pre-fire fuel structure and loading data had been collected. These burns were completed in spring or autumn when the moss layer fuel moisture was generally very high (72% to 552%). We therefore assigned moss/ litter to the 1-hour fuel category in the same way as for our previous low-risk fire weather scenario. Wind speed, and live and dead fuel moisture, were set based on values recorded for the given experiment. Rates of spread were predicted using the “ros” function in R. To understand how including the estimated available moss/litter load affected rate of spread predictions we also constructed fuel models without any addition of moss/litter. To compare the resulting predictions, we generated linear models of predicted vs. observed spread rates using the “lm” function in R. We then compared the slopes of each linear model to the 1:1 line of perfect agreement (LPA - [56]) where deviations of a model’s slope from the 1:1 line would reflect a potential for over- or under-prediction by our models. We also compared the position of each model’s 1:1 line to the 95% confidence intervals of each model. If the 1:1 line fell within the majority of a linear model’s confidence intervals, the observed and predicted values were deemed to not differ significantly from each other.
Results
The combined ecological dataset encompassed total fuel loads ranging from 0.23 kg m-2 (2.27 t ha-1) to 6.27 kg m-2 (62.66 t ha-1). On average, ericaceous live woody material (including Calluna and other dwarf-shrub species) represented 40 ± 21% of total fuel loads while herbaceous material was 7 ± 10%, dead fuel load was 8 ± 7% and the moss/litter layer was 45 ± 19%. Mature and degenerate Calluna stages were generally associated with the greatest total and woody fuel loads, and the greatest fuel bed depths (Fig. 1). The Erica-Ulex and Vaccinium-Calluna heaths (EUNIS = S42) were associated with the largest total fuel loads and fuel bed depths, however fuel characteristics were generally similar among all habitat types (Fig. 2). Longer times since fire in Norwegian fuels were associated with that region’s highest total and live woody fuel loads as well as the largest fuel bed depths (Fig. 3). Records from UK sites which experienced grazing, but where Calluna stage indicated they had not been recently burned, exhibited low fuel bed depths that were similar to Norwegian samples that had been burned three years previously. However, a direct comparison of the effect of time since fire between regions could not be made due to the lack of such data for the UK.
Fig. 1 - Boxplots comparing fuel characteristics across Calluna stages ([30]). UK entries included representation from England and Scotland. No fuel data collected in Norway were represented by the degenerate Calluna stage. English pioneer fuels were also absent from the UK set. The “mire” category is not a true Calluna stage but represents samples collected from Eriophorum-dominated blanket bogs. Box widths are relative sample sizes from N = 296 data entries.
Fig. 2 - Boxplots comparing fuel characteristics across EUNIS communities ([12]). UK entries included representation from England and Scotland. Missing boxplots indicates that no fuel data for that community was collected for a given country. Box widths are relative sample sizes from N = 296 data entries. Some EUNIS communities contained very few fuel entries, attributing to small boxes. The S421 community contains both the H12 and H12b NVC communities ([24]).
Fig. 3 - Boxplots comparing fuel characteristics across varying times since fire for samples collected in Norway. NB = no recent (< 30 years) history of burning. No definitive times-since-fire were available for UK samples. Box widths are relative sample sizes from N = 126 data entries.
Hierarchical cluster analysis identified six unique fuel types (Fig. S3 and Fig. S4 in Supplementary material). The first fuel type (“Mature”) contained fuel samples predominantly classified as mature stage Calluna, but also contained fuels at other Calluna stages with large live woody and moss/litter fuel loads, high fuel bed depths, and moderate canopy bulk densities. The second fuel type (L Build: “late building”) mostly included building stage Calluna fuels with notably large live woody fuel loads, but it also contained many mature stage fuels. While such observations had similar fuel loads to the Mature fuel type, they had lower fuel bed depths, higher canopy bulk densities and much lower moss/litter fuel loads. The third fuel type (T Build; “tall build”) contained mostly building stage Calluna fuels like the L Build group, but it was represented by samples with higher fuel bed depths, much lower canopy bulk densities and higher moss/litter and herbaceous fuel loads. A fourth fuel type was defined (E Build: “early building”) that was also comprised of mostly building stage fuels, but it had the lowest overall fuel bed depths and herbaceous fuel loads, moderate live and dead woody fuel loads, as well as the highest overall canopy bulk densities. The final two fuel types (Build+ and Pioneer+) were comprised mostly of fuels in the building and pioneer Calluna stages respectively, but with greater representation of mire fuel entries than the first four fuel types. These fuel types were relatively similar to each other with regards to fuel characteristics, as they contained: the lowest overall moss/litter and dead woody fuel loads, moderate herbaceous fuel loads, very low live woody fuel loads and moderately high canopy bulk densities. All fuel types contained fuels from England, Norway and Scotland.
There was clear separation in multivariate fuel structure as a function of fuel type cluster and Calluna stage (Fig. 4, Fig. 5). Mire fuel entries appeared to be randomly distributed through the ordinations. There were also substantial overlaps in EUNIS habitat types amongst all fuel entries (Fig. 4, Fig. 5) with only the Hiberno-Britannic Eriophorum-Calluna blanket bog habitat (EUNIS = Q1222) distinct from the others. Results from our PERMANOVA showed that fuel type clusters, EUNIS habitat code and Calluna stage all predicted a difference between their respective fuel entries (Tab. 1). Our pairwise PERMANOVA analyses showed that fuel entries differed between all comparisons of fuel clusters and all comparisons of EUNIS habitat codes except S421 (H12) v. S423 which are both Erica-Ulex and Vaccinium-Calluna heaths (Tab. S3 and Tab. S4 in Supplementary material). For Calluna stage comparisons, we found that all stages differed from each other in fuel characteristics other than for Building vs. Degenerate and Degenerate vs. Mature. Fuels in the building and pioneer Calluna stages, as well as mire fuels, tended to have higher bulk densities and lower 1-hour and live woody fuel loads than mature stage fuels. These differences resulted in higher modeled fire rates of spread for mature stage fuels (Fig. 4, Fig. 5), however several building stage samples produced rates of spread higher than even those of the mature stage. All Calluna stages were well-represented across the UK and Norway. Dry heathland community (EUNIS = S421; NVC = H12b) samples were most commonly associated with records from Norway and also tended to have greater fuel bed depths and lower fuel bulk densities, resulting in higher predicted rates of spread. The lowest rates of spread were associated with the blanket bog habitats (EUNIS = Q12).
Fig. 4 - NMDS analysis examining variation in heathland fuel structure for the high fire risk scenario. Shapes represent country of sampling. Fuels are visualized with regards to: A - Calluna stage ([30]); B - EUNIS (and NVC) community code ([24], [12]); C - the fuel clusters identified via hierarchical clustering; and D - predicted rates of spread. In panes B and C ellipses respectively enclose the centroid ± 1 standard deviation for the EUNIS communities and fuel clusters. Centroids for key fuel components are also shown in pane C including explicit dead fuels (DEAD: 90% moss, litter, and dead wood), live woody fuels (LW), all herbaceous fuels (HERB), fuel bed depth (FBD) and Calluna canopy bulk density (CBD).
Fig. 5 - NMDS analysis examining variation in heathland fuel structure for the low fire risk scenario. Shapes represent country of sampling. Fuels are visualized with regards to: A - Calluna stage ([30]); B - EUNIS (and NVC) community code ([24], [12]); C - the fuel clusters identified via hierarchical clustering; and D - predicted rates of spread. In panes B and C ellipses respectively enclose the centroid ± 1 standard deviation for the EUNIS communities and fuel clusters. Centroids for key fuel components are also shown in pane C including explicit dead fuels (DEAD: 10% moss, litter, and dead wood), live woody fuels (LW), all herbaceous fuels (HERB), fuel bed depth (FBD) and Calluna canopy bulk density (CBD).
Tab. 1 - PERMANOVA comparing the effects of fuel cluster (Mature, L Build, T Build, E Build, Build+ and Pioneer+), EUNIS habitat code (Q121, Q1221, Q1222, S421 (H12), S421 (H12b and S423) and Calluna stage (Pioneer, Building, Mature, Degenerate and Mire). (df): degrees of freedom; (SS): sum of squares; (R2): correlation value; (F): test statistic; (p): significance value for each test set against α = 0.05.
Predictor | df | SS | R2 | F | p |
---|---|---|---|---|---|
Fuel Cluster | 5 | 10.537 | 0.052 | 3.257 | 0.001 |
EUNIS Habitat | 4 | 2.929 | 0.014 | 1.132 | 0.004 |
Calluna Stage | 3 | 2.230 | 0.011 | 1.149 | 0.004 |
Residual | 282 | 182.450 | 0.901 | - | - |
Total | 295 | 202.593 | 1 | - | - |
The modeled fire rate of spread decreased substantially from high (Fig. 4) to medium (Fig. S5 in Supplementary material) to low (Fig. 5) fire risk scenarios. This was linked to increasing fuel moisture along with a reduced allocation of moss and litter fuels to the available 1-hour fuel class. The latter was especially the case within UK heathland and mire communities where deep pleurocarpous moss layers were generally present (typically represented by mature Calluna stages). Relative differences in fuel structure and modeled fire behavior between regions, vegetation communities and Calluna stages did not substantively differ across the fire risk scenarios considered. Predicted rates of spread differed between fuel types for all fire weather scenarios (Tab. 2). All fuel clusters were distinct with regards to fire behaviour apart from the pioneer and building stage Calluna fuels with many mire entries (Pioneer+ and Building+; Tab. 3). The lack of distinct differences in fire behaviour between these fuel types meant they were subsequently combined (P Build+; mostly building, with pioneer and mire entries), leading to a final set of five fuel models (Tab. 4). The highest fire rates of spread were predicted for the fuel types pertaining to mature stage Calluna (Mature) and tall building stage Calluna (T Build). Fuel types with more mire type vegetation (P Build+) and early building stage Calluna (E Build) had the lowest predicted fire rates of spread.
Tab. 2 - ANOVA comparing the effects of windspeed (0-9 km hr-1), dead FMC (15-23%), fuel type (Mature, L Build, T Build, E Build, Build+ and Pioneer+) and their interaction on rate of spread predictions. (F Value): the test statistic computed; (p): a significance value for each test set against α = 0.05. N = 300.
Response: Rate of Spread | df | F Value | p |
---|---|---|---|
Windspeed | 1 | 3993.68 | < 0.001 |
Dead FMC | 1 | 178.55 | < 0.001 |
Fuel Type | 5 | 257.06 | < 0.001 |
Windpeed × Dead FMC | 1 | 96.80 | < 0.001 |
Windspeed × Fuel Model | 5 | 140.70 | < 0.001 |
Dead FMC × Fuel Model | 5 | 6.89 | < 0.001 |
Windspeed × Dead FMC × Fuel Model | 5 | 3.70 | 0.003 |
Tab. 3 - Lsmeans analysis of a linear model for Rothermel rate of spread (m min-1) with fuel group as a factor. (lsmean): behaviour of the rate of spread dependent variable in response to the fuel group factor; (SE): error associated with each mean; (df): degrees of freedom; (Lower CL, Upper CL): 95% confidence limits of the analysis for each factor level; (Group): how each fuel group overlaps with regards to their lsmean.
Linear Model | lsmean | SE | df | Lower CL | Upper CL | Group |
---|---|---|---|---|---|---|
E Build | 4.36 | 0.223 | 276 | 3.76 | 4.95 | a |
Pioneer+ | 5.44 | 0.223 | 276 | 4.85 | 6.03 | b |
Build+ | 5.48 | 0.223 | 276 | 4.89 | 6.07 | b |
L Build | 7.55 | 0.223 | 276 | 6.96 | 8.14 | c |
T Build | 10.42 | 0.223 | 276 | 9.83 | 11.02 | d |
Mature | 13.67 | 0.223 | 276 | 13.08 | 14.26 | e |
Tab. 4 - Summary of final fuel types developed via hierarchical clustering analysis and assessment of variation in predicted rates of spread. P Build+ is a combination of Build+ and Pioneer+. Predicted rates of spread are from moisture contents and windspeeds used in our NMDS ordinations. All rates of spread were produced for the dormant oceanic dry season when herbaceous fuels are senesced and thus represent the peak fire risk period ([19]). Moss/litter fuel load is the available moss/litter based on fire risk scenario (high, medium, low) to add to 1-hour fuels.
Fire Risk Scenario |
Model Name | Model Type | 1-hour Fuel Load (kg m-2) |
Moss/Litter Fuel Load (kg m-2) |
Live Woody Fuel Load (kg m-2) |
Fuel Bed Depth (m) |
Predicted Rate of Spread (m min-1) |
---|---|---|---|---|---|---|---|
High | Mature | Static | 0.220 | 1.019 | 1.214 | 0.557 | 16.999 |
L Build | Static | 0.261 | 0.660 | 1.062 | 0.329 | 9.701 | |
T Build | Static | 0.212 | 0.761 | 0.259 | 0.381 | 12.387 | |
E Build | Static | 0.141 | 0.498 | 0.624 | 0.187 | 5.593 | |
P Build+ | Static | 0.157 | 0.390 | 0.266 | 0.203 | 6.314 | |
Medium | Mature | Static | 0.220 | 0.699 | 1.214 | 0.531 | 12.273 |
L Build | Static | 0.261 | 0.553 | 1.062 | 0.321 | 6.984 | |
T Build | Static | 0.212 | 0.655 | 0.259 | 0.373 | 9.915 | |
E Build | Static | 0.141 | 0.416 | 0.624 | 0.180 | 4.105 | |
P Build+ | Static | 0.157 | 0.292 | 0.266 | 0.198 | 4.790 | |
Low | Mature | Static | 0.220 | 0.485 | 1.214 | 0.514 | 6.764 |
L Build | Static | 0.261 | 0.281 | 1.062 | 0.300 | 2.259 | |
T Build | Static | 0.212 | 0.275 | 0.259 | 0.343 | 6.033 | |
E Build | Static | 0.141 | 0.214 | 0.624 | 0.170 | 1.565 | |
P Build+ | Static | 0.157 | 0.171 | 0.266 | 0.192 | 2.487 |
Our classification tree analysis yielded a relatively strong model (Fit = 0.62 - Fig. 6) with five final fuel models separated as a function of Calluna stage, country (Scotland vs. elsewhere) and fuel bed depth. Utilizing this decision tree to assign fuel models to experimental burns, we found that the slopes of both our linear models comparing predicted to observed values were not different from the slope of the 1:1 LPA (p = 0.70, R2 = 0.45 for the predictions without moss/litter included in the 1-hour fuels and p = 0.99, R2 = 0.50 for the predictions including moss/litter - Fig. S6 in Supplementary material). When moss/litter was included in the fuel model the 1:1 LPA fell entirely within the confidence intervals for the linear model of predicted vs. observed rate of spread.
Fig. 6 - Decision tree based on field-observable fuel structures and site attributes. Model fit: 0.62. FBD is fuel bed depth above the moss/litter layer.
Discussion
Utilizing heathland and peatland fuel samples collected from a diverse range of geographic regions, plant community types, and times-since fire, we identified five broad fuel types that displayed easily identifiable structural and ecological characteristics and different fire behaviour in response to changing fire weather. The resulting fuel models represent an array of fuel conditions during the dormant season, when fire risk is greatest ([19], [42]) for Calluna-dominated oceanic heathlands within the early building, late building, and mature stages of the Calluna cycle. Many of the structural stages and ecological communities utilized to classify fuels here can be readily recognized in the field and from aerial imagery ([71]), suggesting the potential to adopt these fuel models and our classification decision tree for evaluation of fire hazard and potential fire behaviour. However, regional variations in fuel characteristics, with regards to the age and ecology of Calluna-dominated communities are still poorly understood. Though we captured a relatively wide range of conditions, further research is necessary especially in the context of potential land-cover and land-use change. The encroachment of birch (Betula spp.), juniper (Juniperus communis) and conifers onto heathlands has been demonstrated to lead to significant alterations to vegetation, soils, and fuels ([47]). Abandonment of traditional land-use with associated fire management regimes is also a threat to heathland biodiversity and cultural services ([38]). Both of these processes will likely result in impacts on fuels and fire behaviour ([32]). We have limited knowledge of fuel conditions in such transitional habitats as well as in fuels resulting from restoration of drained or afforested peatlands. Additionally, dead biomass can rapidly increase following physiological drought resulting from both dry and/or very cold weather ([33], [6]). However, if late-successional heathlands are more susceptible to drought ([36]), then changes in traditional management regimes could further exacerbate fire risks and climate change impacts on heathlands.
Improved understanding of seasonal/phenological variation in fuels is also important - here the timing of sampling meant we could only make inferences about fuel characteristics during the dormant season. During the summer Calluna live fuel moisture content is considerably higher ([15]) and live herbaceous plants, also with high fuel moistures, can be abundant in some fuel types. Live fuel moisture has been observed to have a dampening effect on shrubland fire behaviour ([54]), but how this balance with potentially drier coarse dead fuels and moss/litter layers needs to be determined. Given that many peatland and heathland landscapes in north-west Europe find themselves at a critical juncture under the combined pressures of climatic, environmental, economic and policy changes ([25]), it is vital we expand our understanding of fuel conditions to allow for robust assessment of wildfire hazard across all seasons, land-use scenarios and edaphic settings.
Fuel loads we estimated were within the range of those recorded for other shrublands. For instance, Casals et al. ([8]) observed total fuel loads for a suite of CORINE Mediterranean shrubland biotypes to range from 0.02 to 3.93 kg m-2. Our shrubs loads (i.e., excluding the moss/litter layer) are, however, lower than those recorded for other fire-prone shrublands such as those found in Nova Scotia (3.17 ± 0.84 kg m-2 - [52]), the Fynbos of South Africa (2.5 kg m-2 - [67]) and at the lower end of values observed for chapparal shrublands of California (1.1 to 7.5 kg m-2 - [63]). The Calluna heathlands we observed were, however, distinct in their comparatively low fuel bed depth compared to their load; this will result in generally higher bulk densities than observed in other shrubland fuel types. Within our dataset there was, however, a clear distinction in the ecological and structural scope of fuels collected in the UK vs. Norway. For instance, Norwegian fuels tended to contain substantially more herbaceous plant material and to be taller with lower bulk density for the same Calluna stage. Fuel bulk density is known to be a fundamental control on fire spread in porous natural fuel beds ([59], [14]). Comparatively heavy grazing from sheep and deer is common in UK heathlands and biomass off-take can be high ([7]). Grazing is also known to alter patterns of Calluna growth and structure ([66]). Given that most of the sampled Norwegian sites were ungrazed during the scope of fuels collection, this could explain the greater fuel bed depths associated with fuel samples from that region. Regional variations in fuels could also be the result of environmental and microclimatic differences (e.g., exposure) between the sampling sites. UK fuels were mostly sampled in the Scottish Highlands and English uplands while Norwegian fuels came from lowland coastal heaths. Greater productivity in Norwegian sites could reduce stress and shift patterns of competition to favour more rapid vertical growth ([21]). Finally, stage-related differences in Norwegian and UK fuels could potentially be the result of variations in sampling practice between the UK and Norway researchers and/or differences in the way in which vegetation is qualitatively assigned to stages of the Calluna cycle. Furthermore, dead fuels in Calluna canopies were sorted and recorded for the UK but not for Norway, leading to a conservative estimate of dead fuel loads for Norway. This suggests a need for harmonized fuel sampling procedures and more consistent descriptions of sample ecology across the array of heathland ecosystems.
Heathland and peatland fuel structures are somewhat unusual due to the presence of large loads of acrocarpous, pleurocarpous, and Sphagnum mosses the composition of which varies with stand age and environmental conditions. Such fuels can comprise up to 90% of the total load present, especially in blanket bog habitats. During normal prescribed burning conditions, and large parts of the dormant season when wildfires are common, these layers have extremely high moisture contents (72% to 552% across the 27 experimental fires burned by [14]) and often show limited consumption ([18]). Mosses lack active mechanisms to control moisture loss and can therefore dry rapidly during periods of drought. We explored the effect of changing moss layer availability and our modeling suggested such fuels have the potential to contribute to significant increases in the rate of fire spread. Previous research tentatively linked the flammability of these fuels to both opportunity for accidental wildfires and increased risk of managed burns escaping ([19]). By comparing observations of fire rates of spread to predictions based on our fuel models, we show that utilizing the moss/litter layer as an active fire front predictor is viable. Our predictions were based on a low-risk scenario that reflected conditions during those burns, but the high agreement of our results to real-world observations also suggest that at least a proportion of these fuels actively contribute to fire spread and their drying may therefore significantly increase fire risk. Traditional burning practices acknowledge these risks and are normally conducted in periods when the ground is wet ([20]). As the climate warms regions such as the UK and Norway are expected to see higher temperatures and increased drought severity but also increased overall precipitation and fewer periods of very cold weather ([34]). Such patterns will significantly alter inter and intra-annual fuel moisture dynamics with consequences for wildfire risk and the availability of periods of suitable for management burning ([4], [44]). The results here highlight the need for extreme caution during periods when the moss and litter is dry enough to ignite as well as for more focus on data collection during the traditional growing season that will see more risky fire behaviour in the future due to climate change.
Conclusions
The differences in fuel structure and modeled fire behaviour revealed by our study provide two important considerations for future management and research. Firstly, age-related changes in the fuel structure across stages of the Calluna cycle lead to significantly more intense modelled fire behaviour as fuels accumulate and thus areas of unmanaged Calluna represent a greater wildfire hazard. This suggests that management burns may be one appropriate tool for reducing wildfire risk and associated threats to soils carbon stores and human lives and livelihoods. Secondly, mire (blanket bog) communities that are typically found on deep peat displayed some of the least intense modelled fire behaviour. Thus, peatland restoration that leads to raised water-tables, and a transition away from fuel structures associated with drier heathland conditions, may be associated with reduced wildfire hazard in the longer term. However, in the short-medium term we need better understanding of the characteristics of transitional fuel types which could, for instance, include larger amounts of dead material due to shrub mortality. Our results here, taken together with evidence that existing fire behaviour models could be applied to heathland fuels ([45]), suggests it would be prudent to develop management tools that aid in understanding how fuels impact fire behaviour. This could include fire behaviour nomograms ([22]) or the fuel structure decision tree we have provided. Producing meaningful guidance will, however, require attention to better understanding the wider array of heathland and peatland fuel conditions, heathland fuel moisture dynamics and the contribution to fire behaviour of heathland moss and litter layer fuels.
Author contributions
CDM-D co-conceived the study, designed and completed the statistical analysis, and led drafting the manuscript; GMD co-conceived the study, collected the Scottish and English data, advised on statistical analysis and assisted in drafting the manuscript; SVH led fieldwork that collected the Norwegian data, processed the fuel samples and contributed to editing the manuscript; PT co-led the development and financing of the LandPress project that facilitated the Norwegian research, collected Norwegian data, and contributed to editing the manuscript; LGV co-led the development and financing of the LandPress and DYNAMIC projects that facilitated the Norwegian research, collected Norwegian data, and contributed to editing the manuscript; VV co-conceived the Norwegian component of the study, co-led the development and financing of the LandPress and DYNAMIC project that facilitated the Norwegian research, and contributed to editing the manuscript.
Acknowledgements
Financial and in-kind support for this research was provided by the Natural Environment Research Council (Grant nos. NER/S/C/2001/06470 and NE/J006289/1), the Research Council of Norway (LandPress, Grant no. 255090; DYNAMIC, Grant no. 298993), NatureScot, The Scottish Government and The Game and Wildlife Conservation Trust. In-kind support and/or generous permission to access land was provided by the Heathland Centre at Lygra, Natural England, The National Trust, Yorkshire Water, United Utilities, NatureScot, Forestry and Land Scotland, Blackhill Estates, Ralia Enterprises, Finzean Estate, Phoines Estates, Birse Community Trust, and the Royal Society for the Protection of Birds. Additional field and scientific assistance was provided by Elaine Boyd, John Dold, Rut Domenech, Alan Gray, Alistair Hamilton, Mark Hancock, Angus MacDonald, Sophie Philbrick, Adam Smith and Colin Legg.
Additional data files
All data and code for this manuscript are available at ⇒ https://github.com/cdmdenvi/FuelModelCreation.
References
CrossRef | Gscholar
CrossRef | Gscholar
CrossRef | Gscholar
Gscholar
Gscholar
Online | Gscholar
Gscholar
Gscholar
Gscholar
Authors’ Info
Authors’ Affiliation
Department of Biology, Georgetown University, 37th and O Streets, NW, Washington, DC 20057 (USA)
School of Environment and Natural Resources, The Ohio State University, Kottman Hall, 2021 Coffey Road, Columbus, OH 43210 (USA)
Department of Landscape and Biodiversity, Norwegian Institute of Bioeconomy Research. Parkveien 15, 8860 Tjøtta (Norway)
Department of Biological Sciences, University of Bergen, PO Box 7801, N-5020 (Norway)
Corresponding author
Paper Info
Citation
Minsavage-Davis CD, Davies GM, Haugum SV, Thorvaldsen P, Guri Velle L, Vandvik V (2024). Development and evaluation of generalized fuel models for predicting fire behaviour in northern European heathlands. iForest 17: 109-119. - doi: 10.3832/ifor4394-017
Academic Editor
Davide Ascoli
Paper history
Received: May 30, 2023
Accepted: Apr 16, 2024
First online: Apr 30, 2024
Publication Date: Apr 30, 2024
Publication Time: 0.47 months
Copyright Information
© SISEF - The Italian Society of Silviculture and Forest Ecology 2024
Open Access
This article is distributed under the terms of the Creative Commons Attribution-Non Commercial 4.0 International (https://creativecommons.org/licenses/by-nc/4.0/), which permits unrestricted use, distribution, and reproduction in any medium, provided you give appropriate credit to the original author(s) and the source, provide a link to the Creative Commons license, and indicate if changes were made.
Web Metrics
Breakdown by View Type
Article Usage
Total Article Views: 520
(from publication date up to now)
Breakdown by View Type
HTML Page Views: 68
Abstract Page Views: 257
PDF Downloads: 186
Citation/Reference Downloads: 2
XML Downloads: 7
Web Metrics
Days since publication: 17
Overall contacts: 520
Avg. contacts per week: 214.12
Article Citations
Article citations are based on data periodically collected from the Clarivate Web of Science web site
(last update: Feb 2023)
(No citations were found up to date. Please come back later)
Publication Metrics
by Dimensions ©
Articles citing this article
List of the papers citing this article based on CrossRef Cited-by.
Related Contents
iForest Similar Articles
Short Communications
Upscaling the estimation of surface-fire rate of spread in maritime pine (Pinus pinaster Ait.) forest
vol. 7, pp. 123-125 (online: 13 January 2014)
Research Articles
Harmonized dataset of surface fuels under Alpine, temperate and Mediterranean conditions in Italy. A synthesis supporting fire management
vol. 13, pp. 513-522 (online: 13 November 2020)
Research Articles
Developing wildfire risk probability models for Eucalyptus globulus stands in Portugal
vol. 6, pp. 217-227 (online: 27 May 2013)
Research Articles
Tree encroachment dynamics in heathlands of north-west Italy: the fire regime hypothesis
vol. 3, pp. 137-143 (online: 27 September 2010)
Research Articles
Wildfire risk and its perception in Kabylia (Algeria)
vol. 11, pp. 367-373 (online: 04 May 2018)
Review Papers
Prescribed burning in Italy: issues, advances and challenges
vol. 6, pp. 79-89 (online: 07 February 2013)
Research Articles
Changes in vegetation diversity and composition following livestock removal along an upland elevational gradient
vol. 8, pp. 582-589 (online: 22 April 2015)
Research Articles
Wildfire and harvesting effects on carbon dynamics in an oak-pine mixed forest
vol. 13, pp. 435-440 (online: 16 September 2020)
Research Articles
Wildland fire typologies and extreme temperatures in NE Spain
vol. 10, pp. 9-14 (online: 01 November 2016)
Research Articles
Wildfire cause analysis: four case-studies in southern Italy
vol. 3, pp. 8-15 (online: 22 January 2010)
iForest Database Search
Search By Author
Search By Keyword
Google Scholar Search
Citing Articles
Search By Author
Search By Keywords
PubMed Search
Search By Author
Search By Keyword