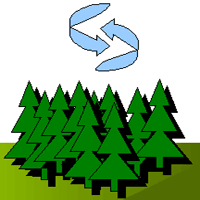
Ozone flux modelling for risk assessment: status and research needs
iForest - Biogeosciences and Forestry, Volume 2, Issue 1, Pages 34-37 (2009)
doi: https://doi.org/10.3832/ifor0485-002
Published: Jan 21, 2009 - Copyright © 2009 SISEF
Short Communications
Collection/Special Issue: Cost Action E29 Meeting 2008 - Istanbul (Turkey)
Future Monitoring and Research Needs for Forest Ecosystems
Guest Editors: Marcus Schaub (WSL, Birmensdorf, CH)
Abstract
In this paper, some shortcomings involved in the modelling of ozone fluxes in the context of local-scale risk assessment are discussed, especially as related to the data collected within the International Co-operative Programme on Assessment and Monitoring of Air Pollution Effects on Forests (ICP Forests). An enhanced monitoring strategy, that would provide a sounder basis for the development, validation and application of risk assessment modelling tools, is also suggested.
Keywords
Introduction
In Europe, the development of air pollution abatement strategies is founded on the effects-based approach, which includes the ozone-induced plant injury as one of the key effects to be minimized. Within this framework, the risk of ozone damage to vegetation is related to numerical exposure and dose indices ([17], [12], [14]). Both types of index are presently defined within the risk assessment methodology adopted within the Convention of Long-range Transboundary Air Pollution of the United Nations Economic Commission for Europe ([17]). However, there is increasing evidence for the superior biological basis of the dose approach ([7]).
The exposure and dose indices differ in that exposure can be evaluated from ozone concentration data alone, while for the calculation of ozone dose the stomatal uptake by vegetation must be modelled. In this paper, we will discuss some shortcomings involved in the modelling of ozone fluxes in the context of local-scale risk assessment, especially as related to the data collected within the International Co-operative Programme on Assessment and Monitoring of Air Pollution Effects on Forests (ICP Forests - [18]). We will also suggest an enhanced monitoring strategy that would provide a sounder basis for the development, validation and application of risk assessment modelling tools.
Flux modelling principles
The dose-based ozone risk indicator (AFstY, Accumulated stomatal Flux above a threshold Y) introduced within the UNECE risk assessment methodology ([17]) can be written as (eqn. 1):
where Fst is the stomatal ozone flux per projected leaf area (PLA) to sunlit leaves at the canopy top and Y is the threshold stomatal flux per PLA (in nmol m-2 s-1). AFstY is calculated from hourly values of Fst (denoted by i), so Δt = 1 h; N denotes the number of hours to be included in the calculation period, which corresponds to the growing season. The stomatal flux in eqn. 1 is defined as (eqn. 2):
where c
i is the hourly ozone concentration, hveg is vegetation height and gst,i is the effective stomatal conductance (here referred to as “effective” because it also depends on the conductances of the leaf boundary layer and the external plant surfaces, see [14]). The stomatal conductance is modelled using the DO3SE (Deposition of Ozone and Stomatal Exchange) model, which is based on a multiplicative plant species-specific parametrization representing the stomatal responses to environmental and phenological factors ([2], [17]). Thus the stomatal flux depends on two components, a concentration and a stomatal conductance, both of which are equally significant for the flux.
Measuring concentration
As indicated by eqn. 1 and eqn. 2, the stomatal flux for AFstY, by definition, is to be calculated from hourly-averaged ozone concentration data. These data could be obtained from a standard ozone analyser that is based on UV absorption photometry; this is the reference method defined in the EU Directive on ambient ozone ([3]). Within ICP Forests, passive sampling is defined as an option for concentration measurements ([15]) and, being relatively inexpensive and easy to deploy in the field, is widely used at the ICP Forests Level II monitoring plots across Europe ([18]). A comparison of passive samplers against the reference method at some ICP Forests sites demonstrated the feasibility of this method but also showed the associated methodological uncertainty ([10]).
A fundamental property of passive sampling is the time-averaging of the measurement. As a typical sampling time for ozone is two weeks ([15]), the concentration data obtained have a low temporal resolution as compared to the definition of many air quality indicators, and especially so for AF st Y. Thus the hourly data must be derived from the measured mean (14-d or so) concentration by using a statistical technique with additional meteorological ([5]) or topographical ([6]) data. This requires a significant amount of prior (hourly) calibration data, and unavoidably further uncertainty is introduced in the modelled hourly values, as exemplified by the results of Gerosa et al. ([4]). Moreover, it is questionable to what extent the correlation between high ozone concentrations and environmental factors limiting stomatal uptake, which was a major motivation for the flux-based approach in the first place, can be simulated by this approach.
According to the monitoring recommendations of ICP Forests, the passive samplers are to be located in an open field near, but outside, the forest at a 2-4-m height ([15]). This contrasts the definition of stomatal flux (eqn. 2), which should be calculated using the tree-top concentration (at hveg ~ 20 m). Consequently, the measured concentration must be transformed to the correct reference height (Fig. 1). This can be accomplished by using a flux-gradient model that relates the vertical concentration profile to wind speed, surface roughness and the bulk surface conductance of ozone deposition ([17]). In principle, this kind of model is founded on a micrometeorological theory which assumes horizontal homogeneity. However, this assumption is not fulfilled very well within the present experimental configuration. Furthermore, neutral atmospheric stability, constant surface conductance and independence of the 20-m concentration of the underlying vegetation were employed as necessary assumptions by Schaub et al. ([11]), who modelled ozone doses using the ICP Forests monitoring data.
Fig. 1 - Schematic presentation of the height transformation for estimating the canopy-top concentration.
Modelling stomatal conductance
The modelling of stomatal conductance with DO3SE depends on data on wind speed, photosynthetically active radiation, air temperature and air humidity at hveg, and data on soil moisture ([2], [17]). According to UNECE ([16]), the recommended height for wind measurements is 10 m, while for most of the other meteorological variables it is 2 m. This means that the measured data should be transformed to h veg, using a micrometeorological flux-gradient relationship similar to that employed for the vertical concentration profile. In addition, since soil moisture status is not measured at the ICP Forests monitoring plots, it needs to be modelled based on water budget principles when applying the DO3SE model ([11]).
A general problem related to flux modelling is the limited validation of models, and here we can note several issues. Firstly, in many cases there are little independent data available for statistically sound cross-validation. This results in conceptual problems concerning the distinction between model calibration and validation, as is evident from the evaluations of the canopy-scale version of DO3SE ([13]).
Secondly, field experiments are seldom designed from the point of view of the characteristics of a certain model, so the data provided by these efforts may be far from optimal for model validation. For example, the observational data may not cover a full range of environmental conditions, as encountered when running the model for a complete growing season, or all the necessary input data, such as soil moisture, are not measured at all ([13]). In particular, the partitioning between stomatal and non-stomatal fluxes would be essential for evaluating flux models. At the canopy scale, this would require measurements of water vapour exchange and/or xylem sap flow. Finally, air quality monitoring programmes, such as that run at the ICP Forests Level II monitoring plots, provide little support for the validation of flux models, as the ozone (or water vapour) flux is not measured.
Discussion
While the passive sampling technique for measuring ozone concentrations offers many advantages over continuous monitoring, especially in a remote and complex forest environment, we have here identified several uncertainty sources specific to the application of this technique to the modelling of ozone fluxes. Some of these issues arise from the monitoring recommendations provided by ICP Forests. All the concentration-related uncertainties discussed above would be avoided, if the ozone concentration was measured at the canopy top using a UV absorption analyser providing hourly-resolved data. In addition, the location of meteorological sensors is not ideal for flux modelling.
In general terms, it can be argued that we are dealing with a trade-off situation as regards the ozone monitoring strategy. On the one hand we have the inexpensive alternative relying on passive sampling; on the other, we could replace this by the costly continuous monitors. The inexpensive alternative makes it possible to run an extensive measurement network. In this case, however, the application of flux-based risk indicators, which should gradually replace the concentration-based indices, entails a large number of additional calculation steps with associated simplifying assumptions. The overall uncertainty involved in these simplifications remains to be quantified. The alternative based on continuous monitors is too costly to be implemented across the existing ICP Forests network. In addition, measurements of ozone deposition fluxes would be needed for model development and validation.
In order to avoid the straightforward trade-off outlined above, an alternative approach could be adopted. We suggest establishing, in addition to the existing Level II sites, a small number of well-equipped measurement sites, “supersites” or “Level III sites”, that would provide data specifically for the flux-based risk assessment purposes. For the calculation of F st and AF st Y, the canopy-top ozone concentration (possibly with vertical within-canopy profiles) and all the input data needed for DO3SE would be measured at these sites on an hourly basis. For further development and validation of DO3SE and other flux models, ozone and water vapour fluxes would be measured above the canopy using the micrometeorological eddy covariance technique, possibly enhanced by sap flow and shoot-scale gas exchange measurements. To overcome the financial and logistic restrictions, all this could be accomplished in practice by collaborating with the existing flux measurement stations (“flux towers”) run across Europe, mainly within large-scale projects such as CarboEurope ([1]) and NitroEurope ([8]) (Fig. 2). These stations constitute a potential framework for the supersites, providing the necessary infrastructure and expertise, extensive measurement programmes and data bases, as well as a direct connection to flourishing research on atmosphere-biosphere exchanges (e.g., [9]).
Fig. 2 - The flux measurement network of the CarboEurope project ([1]).
Acknowledgements
Discussions with Lisa Emberson (SEI-York), Dave Simpson (EMEP MSC-W) and Mika Aurela (FMI) are highly appreciated.
References
Gscholar
CrossRef | Gscholar
Online | Gscholar
Online | Gscholar
Online | Gscholar
Authors’ Info
Authors’ Affiliation
Finnish Meteorological Institute, Climate Change Research, P.O. Box 503, FI-00101 Helsinki, Finland
Corresponding author
Paper Info
Citation
Tuovinen J-P (2009). Ozone flux modelling for risk assessment: status and research needs. iForest 2: 34-37. - doi: 10.3832/ifor0485-002
Academic Editor
Marcus Schaub
Paper history
Received: Mar 13, 2008
Accepted: Dec 09, 2008
First online: Jan 21, 2009
Publication Date: Jan 21, 2009
Publication Time: 1.43 months
Copyright Information
© SISEF - The Italian Society of Silviculture and Forest Ecology 2009
Open Access
This article is distributed under the terms of the Creative Commons Attribution-Non Commercial 4.0 International (https://creativecommons.org/licenses/by-nc/4.0/), which permits unrestricted use, distribution, and reproduction in any medium, provided you give appropriate credit to the original author(s) and the source, provide a link to the Creative Commons license, and indicate if changes were made.
Web Metrics
Breakdown by View Type
Article Usage
Total Article Views: 49795
(from publication date up to now)
Breakdown by View Type
HTML Page Views: 41663
Abstract Page Views: 2995
PDF Downloads: 4131
Citation/Reference Downloads: 64
XML Downloads: 942
Web Metrics
Days since publication: 6006
Overall contacts: 49795
Avg. contacts per week: 58.04
Article Citations
Article citations are based on data periodically collected from the Clarivate Web of Science web site
(last update: Mar 2025)
Total number of cites (since 2009): 1
Average cites per year: 0.06
Publication Metrics
by Dimensions ©
Articles citing this article
List of the papers citing this article based on CrossRef Cited-by.
Related Contents
iForest Similar Articles
Research Articles
A comparison between stomatal ozone uptake and AOT40 of deciduous trees in Japan
vol. 4, pp. 128-135 (online: 01 June 2011)
Research Articles
Prediction of ozone effects on net ecosystem production of Norway spruce forest
vol. 11, pp. 743-750 (online: 15 November 2018)
Research Articles
Soil drench of ethylenediurea (EDU) protects sensitive trees from ozone injury
vol. 4, pp. 66-68 (online: 05 April 2011)
Research Articles
Effects of abiotic stress on gene transcription in European beech: ozone affects ethylene biosynthesis in saplings of Fagus sylvatica L.
vol. 2, pp. 114-118 (online: 10 June 2009)
Review Papers
Monitoring the effects of air pollution on forest condition in Europe: is crown defoliation an adequate indicator?
vol. 3, pp. 86-88 (online: 15 July 2010)
Research Articles
Ambient ozone phytotoxic potential over the Czech forests as assessed by AOT40
vol. 5, pp. 153-162 (online: 25 June 2012)
Short Communications
QA/QC activities and ecological monitoring in the Acid Deposition Monitoring Network in East Asia (EANET)
vol. 2, pp. 26-29 (online: 21 January 2009)
Research Articles
Changes in the proteome of juvenile European beech following three years exposure to free-air elevated ozone
vol. 4, pp. 69-76 (online: 05 April 2011)
Research Articles
A new approach to ozone plant fumigation: The Web-O3-Fumigation. Isoprene response to a gradient of ozone stress in leaves of Quercus pubescens
vol. 1, pp. 22-26 (online: 28 February 2008)
Research Articles
Long-term monitoring of air pollution effects on selected forest ecosystems in the Bucegi-Piatra Craiului and Retezat Mountains, southern Carpathians (Romania)
vol. 4, pp. 49-60 (online: 05 April 2011)
iForest Database Search
Search By Author
Search By Keyword
Google Scholar Search
Citing Articles
Search By Author
Search By Keywords